Using AI for qualitative data analysis has transformed how researchers approach extracting insights from qualitative data. It has opened new opportunities for efficiency, accuracy, and deeper insights. Traditional methods of qualitative data analysis often involved manual transcription, coding, and analysis,ย which were time-consuming and prone to errors. AI changes the game by automating repetitive tasks and offering powerful tools for data interpretation.
In this guide, weโll explore how AI enhances qualitative research and the key concepts and tools driving this transformation. Whether you’re a researcher, a market analyst, or a business leader seeking actionable insights, AI tools can provide the scalability and precision needed in todayโs data-driven world. From transcription to thematic analysis and visualization, the possibilities are vast.
We’ll break down the technical jargon, show you how AI tools can simplify your workflow, and spotlight key players in this field. By the end, youโll understand how AI integrates into qualitative research to uncover patterns, trends, and narratives that shape decision-making.
Analyze & Evaluate Calls. At Scale.
Understanding the Basics of AI for Qualitative Data Analysis
Qualitative data analysis involves interpreting non-numerical data like interviews, focus group transcripts, and open-ended survey responses. Unlike quantitative data, which focuses on numbers, qualitative research seeks to understand themes, emotions, and context.
Traditional vs. AI-Driven Qualitative Analysis
Traditionally, researchers manually transcribed recordings, categorized themes through coding frameworks, and analyzed results using qualitative methodologies like grounded theory or thematic analysis. While these methods offer rich, in-depth insights, they are often slow, prone to human error, and difficult to scale. In 2025, the increasing availability of AI tools is helping researchers overcome these challenges.
AI-driven analysis revolutionizes this process by automating transcription, coding, and sentiment analysis.
One of the primary reasons AI is now essential for qualitative research is the growing volume of data researchers have to process. Tools powered by natural language processing (NLP) and machine learning (ML) algorithms can quickly process large volumes of data, identify patterns, and even detect hidden themes. For example, AI algorithms can cluster responses into categories or analyze sentiment trends across datasets, providing insights faster than manual methods.
In addition to improving efficiency, AI tools also enhance accuracy. By eliminating human bias and providing objective, data-driven analysis, AI ensures that insights are derived more consistently. Lastly, with the rise of remote work and virtual research methods, AI tools have become more critical. Researchers now need solutions that allow them to collaborate, share insights, and maintain data privacy across multiple teams.
Key Terms & Concepts in AI Qualitative Data Analysis
Text Analysis
AI-based text analysis involves processing written or transcribed data to identify underlying patterns, themes, and meanings. For instance, in customer feedback data, AI can highlight common complaints or praises by scanning the text for frequently used words, phrases, or sentiment indicators. It works by breaking text into tokens (words or phrases) and applying algorithms to identify relationships, meaning, or context.
Sentiment Analysis
Sentiment analysis goes beyond identifying themes to determine the tone or emotional weight of the data. AI can classify text as positive, negative, or neutral based on the language used. For example, in a product review, โThe design is great but the battery life is awfulโ would be classified as a mixed sentiment with specific polarity tags for “design” and “battery life.”
Thematic Analysis
This involves discovering and categorizing recurring themes across datasets. AI tools automatically identify these themes by clustering similar phrases or ideas, helping researchers understand dominant narratives by leveraging natural language processing (NLP) and clustering algorithms. These algorithms analyze data contextually, grouping similar ideas for faster pattern recognition.
- Latent Dirichlet Allocation (LDA): This topic modeling technique identifies underlying themes across text-based datasets.
- Text Embeddings: Tools use embeddings to map related ideas, grouping them by semantic similarity to extract deeper meaning.
AI tools can group customer feedback into actionable themes like “pricing concerns,” “feature requests,” and “customer service issues.” These insights can guide product development or marketing strategies. AI-powered thematic analysis also reduces manual errors and makes the process scalable for large datasets, such as thousands of customer feedback entries or interview transcripts.
Coding
Coding in qualitative research means tagging segments of data with labels or categories for analysis. AI speeds this process by automatically assigning codes based on predefined rules or learned patterns. For example, when analyzing interview transcripts, AI can label parts of the text as โchallenges,โ โopportunities,โ or โrecommendationsโ without manual effort.
Clustering
Clustering groups data points with similar characteristics into clusters without pre-labeled categories. For example, AI might group interview responses into categories like โpositive experiences,โ โnegative feedback,โ and โneutral commentsโ based on linguistic patterns or keywords. This helps identify natural groupings within large datasets.
Natural Language Processing (NLP)
NLP enables AI to understand, interpret, and respond to human language. It combines computational linguistics with machine learning to process and analyze large amounts of text or speech data. In qualitative research, NLP can extract key information, summarize content, or even translate between languages while maintaining context and nuance.
Machine Learning Algorithms
These are the backbone of AI-driven qualitative analysis. Machine learning enables AI to learn patterns from data and improve over time. For instance, an AI tool analyzing survey responses might start recognizing new themes or adapting its coding as more data is processed. Examples include supervised learning (where models are trained on labeled data) and unsupervised learning (like clustering).
Data Preprocessing
This step ensures data is clean, consistent, and ready for analysis. It involves removing duplicates, fixing errors, and formatting text for processing. For instance, transcriptions often contain filler words (“um,” “like”), which preprocessing can remove to streamline analysis. Effective preprocessing minimizes errors during later stages of AI processing.
Scalability
Scalability refers to the ability of AI tools to handle growing amounts of data without compromising speed or accuracy. Traditional qualitative analysis might falter with 50+ interview transcripts, but AI tools thrive in such scenarios, processing datasets of virtually any size while maintaining consistency in analysis.
Data Visualization
AI tools enhance qualitative data by transforming insights into visuals that are easier to comprehend and present. Visualizations are critical for engaging stakeholders and facilitating data-driven decisions. For example:
- Segmentation Diagrams: Divide data into distinct groups or personas based on common characteristics. For example, AI can analyze interview data to segment users into personas like “budget-conscious shoppers” or “tech-savvy customers.”
- Journey Maps: Visualize the customerโs experience across touchpoints, integrating qualitative insights like emotions or pain points. For example, using AI, a journey map can include qualitative data such as customer frustrations (“long wait times”) and satisfaction points (“easy checkout”).
- Mind Maps: Showcase hierarchical relationships or themes derived from data, offering an intuitive way to organize findings. For example, a mind map could show “customer feedback” branching into “service issues,” “product suggestions,” and “delivery concerns.”
- Interactive Dashboards: Allow users to explore qualitative insights dynamically, filtering data by categories such as demographics or time periods. For example, a dashboard might let you analyze customer complaints by region, product category, or sentiment.
- Word Clouds: Highlight the most frequently mentioned words or phrases. These are useful for a quick, surface-level understanding of trends. For example, a word cloud from customer interviews might emphasize terms like “delivery,” “price,” and “support.”
These tools make complex data easier to digest and ensure stakeholders focus on key takeaways rather than getting lost in raw data.
Extract insights from Customer & Employee Interviews. At Scale.
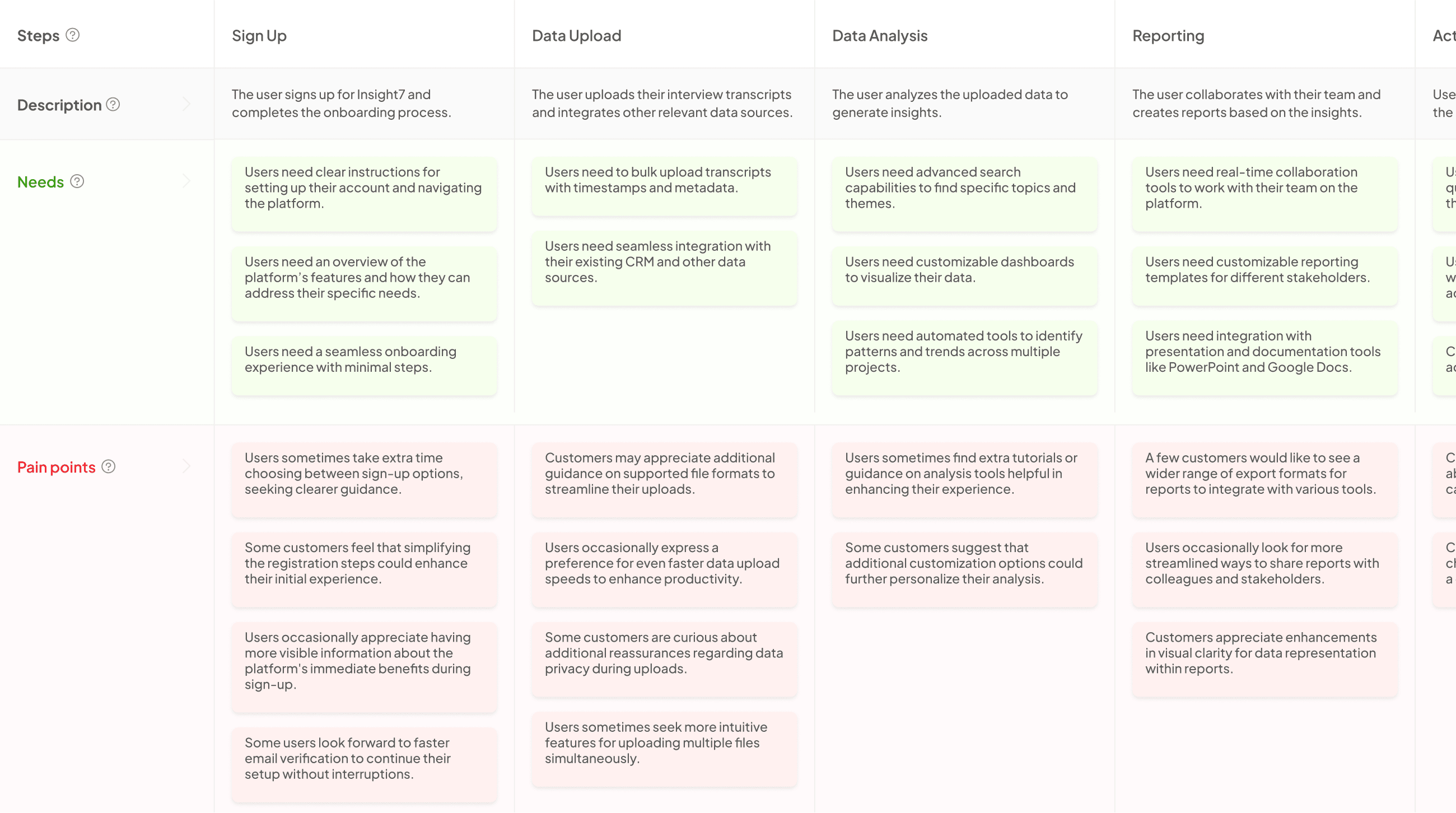
Cross-Case Analysis and Data Synthesis
Cross-case analysis compares data from multiple sources to identify overarching patterns or discrepancies. AI tools excel in this domain, merging diverse datasets like interview transcripts, survey responses, and focus group recordings into a unified framework. Example techniques enhanced by ai are:
- Triangulation: AI integrates findings from multiple sources, ensuring conclusions are credible and holistic. For example, combining focus group insights and social media data to validate trends.
- Segmentation Analysis: AI can identify and visualize demographic or behavioral segments in datasets. For example, segmenting customer feedback by location and age group to understand different preferences.
- Pattern Recognition: Advanced AI algorithms uncover recurring trends across cases. For example, Identifying that “ease of use” and “value for money” consistently rank as top satisfaction drivers across multiple product categories.
These technologies enable researchers to focus more on interpreting findings and less on repetitive tasks. AI complements human intuition with efficiency and precision.
Top 5 AI Tools for Qualitative Data Analysis in 2025
AI tools have become indispensable for qualitative research, each tailored to address specific challenges. Below, weโll explore five leading tools to highlight how they transform data analysis. These AI qualitative data analysis tools not only make the qualitative analysis process more seamless but also ensure the security of sensitive research data.
- Insight7
- Delve
- MaxQDA
- Nvivo
- Sonix
1. Insight7 – Best for End-to-End Qualitative Analysis and Reporting
Insight7 is a powerful AI qualitative data analysis tool designed specifically for qualitative research. ย It automatically extracts key themes, summary, insights, highlight and sentiment from up to 100 interviews at once.
Designed with user-friendly features and enterprise-grade security, Insight7 enables organizations to turn qualitative data into actionable insights effortlessly. The platform’s no-code design makes it accessible to users without technical expertise, while its project-based repository ensures that all research materials are stored and organized efficiently.
It offers a comprehensive solution that covers everything from transcription to advanced data analysis and reporting. With Insight7, researchers can easily analyze interviews, focus groups, and other unstructured qualitative data to generate actionable insights.
Key Features
- Automated Qualitative Data Analysis: Insight7 automatically analyzes transcripst, identifying key themes and patterns without the need for manual coding.
- Bulk Transcription Capability: Users can upload multiple files for transcription at once, drastically reducing the time needed to prepare qualitative data for analysis.
- Comprehensive Reporting: Insight7 generates detailed reports that include advanced data visualizations and key findings, making it easier for researchers to present their insights.
- Enterprise-Grade Data Security: The platform adheres to industry-standard security measures, such as GDPR and SOC2 compliance, ensuring that sensitive data remains protected.
- Customizable Insight Deliverables: Researchers can create customized reports, journey maps, mind maps, and personas based on the analysis of their qualitative data.
- Collaboration and Sharing: Teams can easily share insights and collaborate on projects in real time, improving the overall workflow.
Benefits:
Insight7 stands out for its ability to handle end-to-end qualitative research. Researchers can upload their transcripts, perform automated analysis, and receive comprehensive reportsโall from a single platform. This saves valuable time and ensures a smoother research process. Additionally, the enterprise-grade data security features make Insight7 ideal for organizations that need to ensure the privacy of sensitive research data.
Extract insights from interviews, calls, surveys and reviews for insights in minutes
2. Delve
Delve is a user-friendly qualitative data analysis platform designed for teams who want to move quickly from raw data to insightsโwithout complex software. Itโs ideal for applied researchers, UX teams, and students who need flexible coding support and real-time collaboration.
While not as automated as Insight7, Delve uses AI to assist researchers during manual tagging, helping uncover emerging themes and patterns as coding progresses.
Key Features:
- Streamlined Coding Process: Tag and categorize segments using a drag-and-drop interface thatโs easy to learn.
- AI-Assisted Theme Identification: Automatically suggests recurring themes based on previous tagging behavior.
- Real-Time Collaboration: Multiple users can code and comment on transcripts simultaneously.
- Project Organization: Organize interviews and focus groups by project folders for better data management.
Benefits:
Delve is a perfect entry point for teams that want to retain control over coding while still getting a productivity boost from AI. Its collaborative features make it especially useful for remote research teams or academic groups working on thesis projects or program evaluations.
3. MAXQDA – Best for Mixed Methods and Visual-Driven Research
MAXQDA is a professional software for qualitative and mixed methods data analysis. While not exclusively AI-driven, it incorporates machine learning features to assist with various aspects of qualitative research. It’s suitable for researchers across multiple disciplines, including social sciences, marketing, and education.
By using MAXQDA, researchers can efficiently organize, analyze, and visualize diverse types of data, from text and audio to images and videos. Its AI-assisted features help streamline the coding process and uncover patterns in large datasets.
Key Features
- AI-assisted coding: Suggests codes based on existing coding patterns and content.
- Automated text analysis: Includes sentiment analysis and topic modeling features.
- Mixed methods functions: Integrates qualitative and quantitative data analysis.
- Transcription support: Offers tools for transcribing audio and video data.
- Visualization tools: Provides various options for visualizing coding and analysis results.
- Advanced query tools: Allows complex Boolean searches and retrieval of coded segments.
Benefits:
MAXQDA is a go-to tool for researchers in social science, education, and marketing fields who need to manage diverse data types and generate sophisticated visual outputs. While it has a steeper learning curve, the payoff is powerfulโespecially for grant-funded projects or academic publications.
4. NVivo – Best for Large-Scale Thematic & Sentiment Analysis
NVivo is a comprehensive qualitative data analysis software designed for researchers, academics, and professionals working with rich text-based and multimedia information. It employs AI-driven features to streamline the analysis of interviews, focus group discussions, surveys, and other data sources.
Using NVivo, researchers can efficiently organize, analyze, and gain insights from large volumes of unstructured data. NVivo’s AI capabilities enable automatic coding suggestionsย and theme identification, significantly reducing the time spent on manual coding and allowing researchers to focus on deeper analysis and interpretation. While NVivo has a learning curve, its extensive documentation and support resources make it manageable for most users
Key Features
- AI-powered analysis: Utilizes machine learning algorithms for automated coding suggestions and theme identification.
- Automated insights: Speeds up the identification of themes and patterns across multiple data sources.
- Sentiment analysis: Measures the overall sentiment of textual data, helping researchers understand emotional tones in responses.
- Visualization tools: Offers word clouds, concept maps, and other visual representations to aid in data exploration and presentation.
- Query tools: Offers advanced query capabilities for complex data analysis and hypothesis testing.
Benefits:
NVivo is built for depth and flexibility. While it requires onboarding and some training, it shines in long-term, large-scale projects where researchers need both breadth and nuance. Itโs perfect for grant-funded studies or policy evaluations that demand rigor and repeatability.
5. Sonix – Best for Fast, Accurate Transcription with Keyword Tagging
Sonix is an AI transcription platform that helps researchers quickly convert interviews, podcasts, or video calls into clean, searchable text. It supports over 30 languages and integrates keyword tagging to speed up qualitative analysis.
Although Sonix doesn’t offer full thematic analysis like Insight7 or NVivo, itโs a powerful companion tool for teams who need reliable, multilingual transcription as a first step.
Key Features
- AI transcription: Provides fast, highly accurate transcription of audio and video recordings.
- Multi-language support: Transcribes content in various languages, making it ideal for global research projects.
- Keyword extraction: Automatically highlights keywords and key topics for faster analysis.
- Collaborative editing: Enables teams to collaborate on transcript reviews and share insights.
- Export options: Allows exporting transcripts into multiple formats for further analysis.
Benefits:
Sonix is best used alongside deeper analysis platforms. For researchers conducting international or multilingual interviews, it saves hours in transcription time and creates a clean foundation for further coding or sentiment tagging in tools like MAXQDA or Insight7.
Qualitative Research Tools: Table Overview
Tool | Key Features | Best Suited For | Pros | Cons |
---|---|---|---|---|
Insight7 | AI qualitative data analysis, bulk transcription, customizable reporting, data security | Marketing research, content creation, strategic messaging | No-code interface, secure data, handles large datasets | Not suited for quantitative research |
Delve | Streamlined coding, AI-assisted theme identification, collaborative analysis | Teams needing quick insights from qualitative data | Easy-to-use interface, real-time collaboration | Limited support for advanced data visualization |
MAXQDA | AI-assisted coding, mixed methods integration, transcription support | Researchers across disciplines (social sciences, education, marketing) | Robust tools for qualitative and quantitative analysis | Steep learning curve for new users |
NVivo | AI-powered coding, sentiment analysis, data visualization | Academics, social researchers, large-scale projects | Advanced visualization tools, extensive query capabilities | More complex, can be overwhelming for small teams |
Sonix | Fast AI transcription, automated tagging, multi-language support | Global research teams, interviews, podcasts | Accurate transcription, multi-language capabilities | Primarily a transcription tool, lacks advanced analysis features |
These AI tools are reshaping qualitative research by automating time-consuming tasks, uncovering deeper insights, and enabling more efficient analysis of large datasets. Each tool offers unique features that cater to different aspects of the qualitative research process, from data collection and transcription to analysis and visualization.
Analyze & Evaluate Calls. At Scale.
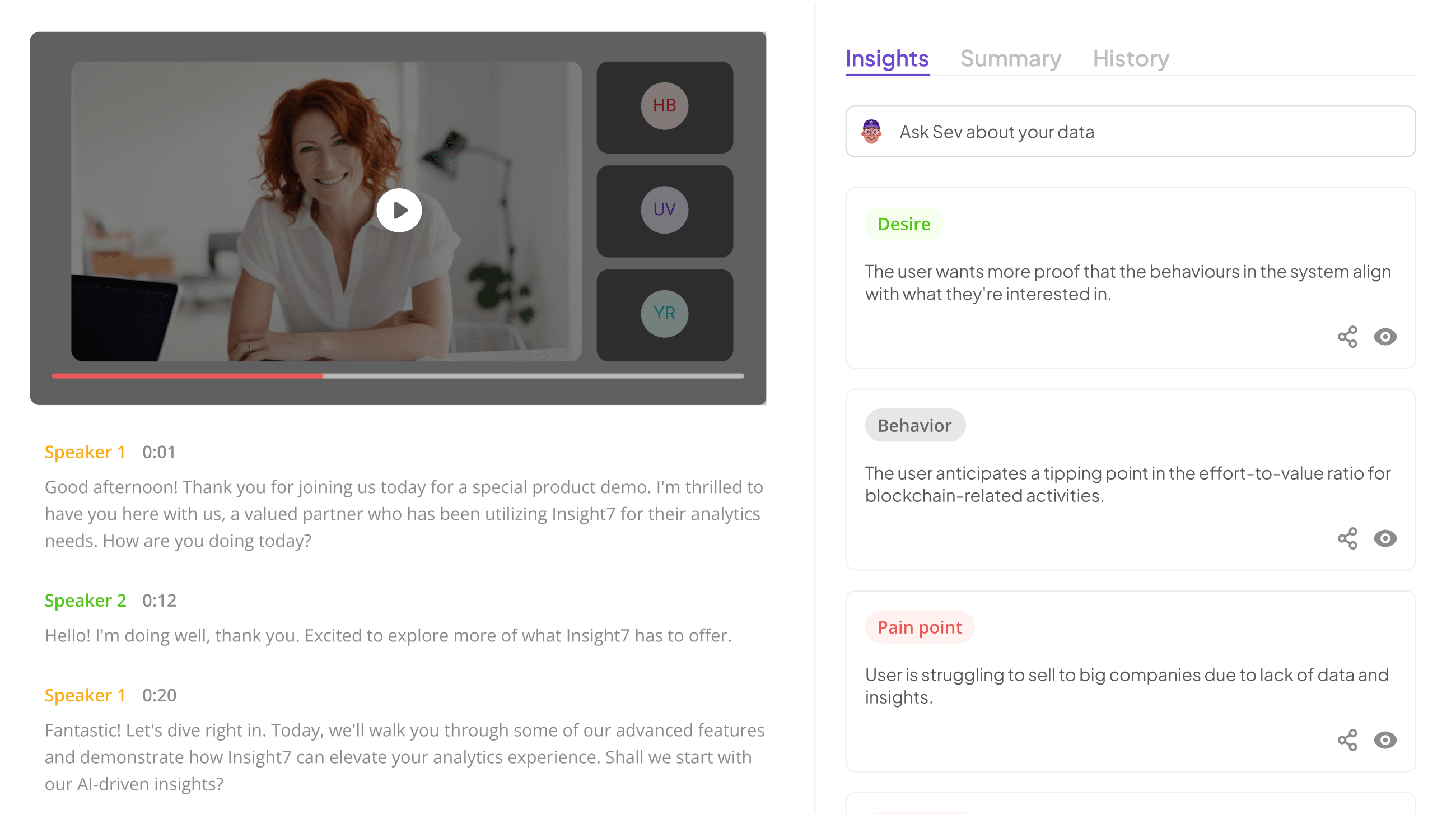
How to Choose the Right AI Tool for Qualitative Data Analysis
Choosing the right AI-powered tool for qualitative analysis starts with one key principle: match the toolโs strengths to your research goals and workflow complexity. Not every platform will suit every teamโso itโs critical to evaluate your specific needs before investing time or budget.
1. Define Your Research Objectives
Begin by identifying the primary goal of your analysis. Are you trying to:
- Transcribe interviews?
- Extract themes and patterns?
- Visualize journey maps or personas?
- Compare sentiment across datasets?
For instance, if you want a fully automated, end-to-end platform, Insight7 is ideal. If youโre looking to manually code with AI-assisted suggestions, a tool like Delve might be more appropriate.
A UX team running 40 customer interviews will benefit more from Insight7โs bulk analysis than from a manual-heavy platform like NVivo.
2. Consider Your Teamโs Skill Level
Ease of use is criticalโespecially if your team includes non-researchers or if youโre on a tight deadline. Platforms like Insight7 and Delve offer no-code, intuitive interfaces perfect for teams without technical backgrounds. In contrast, NVivo and MAXQDA are better suited for teams with research experience or academic training.
3. Evaluate Data Types and Inputs
Think about what kind of data youโre working with:
- Text only?
- Multimedia (audio/video/images)?
- Surveys, interviews, or social media inputs?
For rich media, choose tools like NVivo or MAXQDA that support multimedia analysis. If your main input is voice or video calls, Sonix offers a fast, multilingual transcription foundation.
4. Prioritize Security and Compliance
If you’re handling sensitive information (e.g., health data, customer interviews), make sure your tool meets compliance standards like:
- GDPR
- SOC2
- Data encryption
Insight7, for example, is built with enterprise-grade data security and is compliant with global privacy standardsโmaking it a strong choice for enterprise and healthcare settings.
5. Look for Customization and Output Flexibility
The best tools let you tailor insights for different stakeholders. If you need customizable reports, exportable visuals, or branded dashboards, prioritize platforms like Insight7 or MAXQDA that allow you to shape deliverables to match audience needs.
Tip: Always test a demo version first. Itโs the easiest way to assess whether the platform matches your real-world use case and integrates into your workflow smoothly.
Common Pitfalls and Challenges of AI in Qualitative Data Analysis
AI has brought transformative potential to qualitative data analysis, but its adoption isnโt without hurdles. Understanding these pitfalls and how to navigate them ensures researchers extract maximum value from AI-driven tools.
Data Security Concerns
One of the most significant challenges is ensuring data privacy and security, especially when handling sensitive customer or market research data. Tools like Insight7 mitigate these concerns by adhering to GDPR and SOC2 compliance standards and using AWS for secure data storage, offering enterprise-grade security.
Ethical Considerations in Analysis
AI systems can unintentionally introduce biases into analysis, leading to skewed insights. Researchers must carefully train and monitor AI algorithms to minimize bias. Combining automated results with human review ensures findings remain accurate and contextually relevant.
Contextual Understanding
AI excels at identifying patterns, but it often struggles with nuanced interpretation. For example, sarcasm, cultural context, or colloquial expressions may be misinterpreted. To counteract this, tools like Insight7 enable human validation of AI-generated insights, ensuring that qualitative rigor is maintained.
Integration and Usability
Another challenge lies in integrating AI tools with existing workflows. Researchers often encounter compatibility issues with legacy systems or face steep learning curves. Opting for user-friendly platforms that support project-based research repositories, like Insight7, simplifies this process, enabling seamless collaboration across teams.
Strategies to Overcome These Challenges
- Data Security Measures: Always choose tools that offer robust encryption and compliance with data protection regulations.
- Human Oversight: Combine AI-powered insights with human expertise to ensure accurate, actionable results.
- Training and Onboarding: Invest in proper training to integrate AI tools into existing workflows effectively.
Tips for Successful AI-Driven Qualitative Data Analysis
Making the most of AI tools requires a thoughtful approach to their implementation and use. Here are some actionable strategies to maximize effectiveness:
- Align AI Tools with Research Goals: Before selecting an AI tool, clearly define your research objectives. For example, if the focus is on uncovering customer sentiment, prioritize tools with advanced sentiment analysis and thematic coding features.
- Combine Human Expertise with AI Insights: AI can process vast amounts of data quickly but lacks the intuition and depth of human researchers. Use AI to surface patterns and trends, then apply human judgment to interpret and contextualize findings.
- Leverage Collaboration Features: Modern platforms like Insight7 allow teams to collaborate and share insights seamlessly, making it easier to align on findings and recommendations. Collaborative tools also ensure everyone has access to updated project data in real time.
The Future of AI in Qualitative Data Analysis:
Will AI Tools Replace Researchers?
A common misconception is that AI will replace human researchers. While AI excels at pattern recognition and data processing, it cannot replicate the contextual understanding and empathy researchers bring. For example, interpreting the emotional subtext of an interview often requires human insight.
AI as a Tool, Not a Replacement
AI tools are designed to assist, not replace, researchers. By automating mundane tasks, AI enables researchers to focus on strategic activities like hypothesis testing and designing innovative methodologies. Insight7 exemplifies this by offering features such as bulk transcription and customizable insight deliverables, allowing users to save time while delivering high-quality results.
The Importance of Human Expertise
Human expertise remains indispensable in qualitative research. AI outputs must be validated, interpreted, and aligned with broader research objectives. Researchers bring the critical thinking necessary to contextualize AI-generated insights.
Industry Trends
The role of researchers will evolve as AI becomes an integral part of the workflow. For instance, researchers will need to develop skills in AI tool integrations and interpreting advanced data visualizations like interactive charts or network diagrams.
How Researchers Can Collaborate with AI
Practical Collaboration
Using AI tools effectively requires researchers to adopt a collaborative mindset. AI can identify patterns or themes across large datasets, providing a foundation for researchers to interpret and refine. For instance, tools that use machine learning algorithms for thematic analysis can group responses into meaningful categories, leaving researchers to evaluate these groupings against project goals.
Integrating AI into the workflow also involves iterative processes. Researchers can guide AI by refining the focus of analysis, training the system with additional inputs, or providing feedback on initial results, ensuring AI output aligns with the studyโs objectives.
AI for Deeper Insights
AI tools often excel at uncovering hidden relationships in qualitative data. By analyzing datasets holistically, AI can identify trends, correlations, and anomalies that might escape a human researcherโs notice. For example, AI can reveal subtle shifts in customer sentiment over time or identify recurring themes in customer feedback, providing richer insights than manual analysis.
However, these insights are most valuable when paired with human expertise. Researchers add depth to the data by interpreting nuanced contexts and aligning insights with the studyโs purpose.
New Skills for Researchers
As AI continues to evolve, researchers must develop complementary skills to maximize its potential. These skills include understanding how to use AI-powered platforms, interpreting AI-generated results, and ensuring ethical considerations in data usage. Additionally, researchers should familiarize themselves with AI’s capabilities and limitations to better integrate these tools into their workflows.
The Human Touch in Research
Despite AIโs advances, qualitative research fundamentally relies on human interpretation. AI can analyze data, but it cannot replace the empathy, intuition, and domain expertise that researchers bring. By using AI to enhance efficiency and accuracy, researchers can allocate more time to storytelling, strategy development, and drawing connections that resonate with stakeholders.
Analyze & Evaluate Calls. At Scale.
Conclusion: Unleashing the Power of AI in Qualitative Data Analysis
AI is no longer a future concept in qualitative researchโitโs a current necessity. From automating repetitive tasks like transcription to uncovering deep, hidden patterns across massive datasets, AI tools are transforming how we analyze conversations, interviews, and unstructured feedback.
Researchers, strategists, product teams, and evaluators now have access to platforms that donโt just save timeโthey enhance accuracy, reduce bias, and turn raw insights into strategic advantage.
Among all tools explored, Insight7 stands out as a complete, enterprise-ready solution. With its automated analysis engine, robust security infrastructure, and customizable deliverables, it empowers teams to move faster, think deeper, and deliver smarter insights at scale.
If youโre evaluating interviews, discovery calls, stakeholder feedback, or focus group dataโInsight7 enables you to analyze, visualize, and act on findings with confidence.
With AI qualitative tools, you can:
- Surface customer sentiment at scale
- Build journey maps and personas from real feedback
- Analyze 100+ interviews in hoursโnot weeks
- Drive strategic product, messaging, or policy decisions with real evidence
Ready to elevate your qualitative research?
Try Insight7 and experience the power of AI-powered analysis for yourself.