How to Generate Accurate Transcript From Interview Recordings
As a marketer, you definitely understand the immense value of tapping into the authentic voice of your customers and target audience especially through every transcript from interview sessions with them.
Yet traditional market research methods like surveys often fall short of truly capturing the depth of consumer perspectives, behaviors, and unfiltered opinions.
This is where interview data becomes marketing’s secret weapon. By conducting in-depth interviews, you create a space for open-ended discussion where customers can freely share their experiences, pain points, needs, and desires in their own words. But the value trapped inside the average interview transcript is often underutilized.
With limited human bandwidth, many marketing teams are forced to take a shallow approach when analyzing any transcript from interview sessions with their customers. They end up settling for partial or disjointed insights rather than fully capitalizing on the data’s potential.
Missed Opportunities in Contemporary Interview Transcript Analysis
– Overlooking key drivers for new product/service innovation
– Misreading evolving audience segments and preferences
– Failing to identify critical usability flaws or client success blockers
– Downplaying root issues fueling customer churn and dissatisfaction
– Prioritizing sub-optimal messaging strategies and creative approaches
The consequences? Marketing dollars wasted on off-target campaigns, product lines not resonating with real needs, and customer experiences not matching true expectations. However, a new era of artificial intelligence (AI) is finally empowering marketers to maximize their qualitative research investments at scale.
Through the use of advanced natural language processing (NLP) and machine learning capabilities, marketing teams can rapidly surface impactful insights buried across pages of interview transcripts. AI transcription tools like Insight7 has made it possible for you to generate actionable insights from thousands of interview and make the most of your customer data.
What you Will Gain From This Article
A lot, obviously. But for starters we’ll dive (emphasis on the “dive”) into the best AI techniques to perform transcript analysis throughout the marketing lifecycle – from product strategy and UX research to brand health monitoring, customer experience programs, and beyond. We’ll cover AI workflows to streamline key marketing deliverables like:
– Analyzing transcripts at scale
– Clustering customer segmentation and use cases
– Detecting emerging trends, root causes, and interconnected themes
– Extracting high-impact customer quotes and testimonials
– Quantifying sentiment to gauge success metric
– Auto-generating insight reports, visualizations, and presentations
With the power of AI working alongside your team, you can finally tap into the full depth of customer truth hidden in your interview transcripts. Let’s explore how.
The Value (and Limitations) of Analyzing Transcript from Interview Sessions Traditionally
As any experienced market researcher knows, in-depth qualitative interviews are an incredibly rich source of insight into the hearts and minds of customers. By providing a forum for open-ended discussion and freeform responses, interview transcripts illuminate authentic perspectives that can be difficult to uncover through multiple choice surveys or rigid questionnaires alone.
But it is a known fact that humans have inherent limitations in our capacity to manually process and synthesize these unstructured interview datasets at scale. Traditional qualitative analysis methods create bottlenecks:
Manual Coding Constraints
The first major hurdle that slows analysis is manually coding and labeling relevant quotes from transcripts. Categorizing text into hierarchical codes like “Product Feedback > Feature Requests” or “Brand Sentiment > Competitive Comparisons” is essential to organize insights. Yet this line-by-line code application is massively time-consuming.
Even for efficient analysts, coding a single 1-hour interview can take 3-5 hours of focus work. Scale that across hundreds or thousands of transcripts needed for comprehensive audience understanding, and coding constraints quickly become barriers.
Lack of Quantifiable Grounding
Attempting to avoid the coding bottleneck, some market researchers take a more anecdotal approach – manually excerpting subjective quotes that stick out to them individually. However, without systematic coding and organization, it becomes difficult to quantify the true statistical importance of any observation.
Furthermore, distinctions between high-level themes, niche segmentation, and idiosyncratic one-off comments get muddled without structured data. Prioritizing which insights deserve investment gets subjective and contentious.
Missed Nuances & Connections
Even with diligent coding, human cognition is limited in our ability to instantly detect nuanced relationships and intersecting themes across thousands of quotes and data points. We risk missing subtle undertones or common threads that could represent high-impact opportunities.
Without AI assistance, we’re confined to linear thinking rather than rapidly uncovering non-obvious insights. Keywords and basic Boolean searches are poor proxies for the sophisticated exploration techniques made possible by AI.
Reporting Roadblocks
Finally, marketers frequently struggle to quickly package and socialize their qualitative findings beyond static PowerPoint reports or quote spreadsheets. Transforming transcripts into clear narratives with solid evidence trails and persuasive data visualization becomes a lengthy chore.
Stakeholders lack direct transparency into the underlying quotes and reasoning justifying recommendations. As a result, marketing strategies and investment decisions get anchored in incomplete evidence rather than capitalizing on the full qualitative dataset.
How AI Transforms the Analysis of Transcript From Interview Recordings
To overcome these constraints and unlock the transformative power of qualitative interviews, AI offers marketing teams a comprehensive solution to accelerate and streamline transcript analysis from beginning to end:
Automated, Intelligent Transcript Coding
AI models can automatically apply custom code taxonomies across a full transcript from interview sessions with human-level accuracy, massively accelerating the coding process.
Multichannel transcripts from sources like web interviews, audio calls, video focus groups, and more are automatically transcribed using speech recognition and converted into analyzable text data.
Natural language processing models then intelligently suggest applicable codes as human analysts validate the recommendations, incrementally improving the AI coding accuracy over time.
Theme & Trend Discovery
Once coded, AI techniques like topic modeling, clustering, and unsupervised learning can rapidly uncover hidden thematic patterns buried across the interview transcript dataset.
Areas of intersecting interest between audiences, consistent pain points and friction drivers, as well as emerging new trends are automatically surfaced for analysis rather than relying on randomized human discovery.
AI models quantify inter-code relationships, establish hierarchies, and detect subtle naunces that may otherwise go unnoticed — for example, uncovering a new sub-segment of customers with distinct channel preferences or user journey divergences.
Customer segmentation
In addition to high-level thematic findings, AI also illuminates customer segmentation details and nuanced persona profiles organically present in the qualitative data.
By clustering common quotes and perspectives, marketers can accelerate understanding of the finest micro trends or niche audiences within their target markets. Verbatim language offers authentic color to enhance personas.
Similarly, custom AI models can isolate and classify specific types of commentary around features, UX friction areas, or branding resonance to efficiently harvest insights for more tactical marketing activations.
Intelligent Insight Synthesis
Another key advantage of AI is its ability to synthesize evidence and automatically generate written findings in a coherent narrative structure.
AI tools can ingest coded interview transcripts along with key outputs like sentiment analysis, trend models, quote banks, and more. Abstractive summarization then generates ingestible, human-like summaries articulating the core insights in plain language for stakeholders.
These AI-generated insight reports pull in visualizations, exemplar quotes, supporting data points, and marketing-specific recommendations as needed – all in a seamlessly designed deliverable format. They offer a shareable starting point for iteration and socialization.
Interactive Transcript Exploration
Crucially, AI also powers advanced ways to dynamically explore and navigate the original transcript dataset from any angle. Teams aren’t confined to linearly dissecting monolithic reports.
Intelligent search allows querying on conceptual topics beyond basic keywords, surfacing relevant quotes in context from across fragmented conversations. Clustering visualizations reveal intersecting code relationships and granular audience facets for drill-down analysis.
Recommendations models proactively surface insights catered to each user’s role and areas of responsibility by learning their specific interests and priorities over time through feedback loops. The possibilities for extracting personalized value are limitless.
Step-by-step Process For Analyzing Transcript From Interview Recordings
The process of analyzing interview transcript is pretty straightforward. However, the quality of transcript is determined by a couple of factors including the quality of video or audio being transcribed and the transcription tool used.
While the quality of the transcript analysis for the most part is determined by the clarity of the transcript and the AI tool used. It is basically garbage-in-garbage-out for lack of a better word. But following the steps below will determine a better outcome and help you generate accurate insights from your transcript.
Organizing and Cleaning Transcripts
The first step in generating actionable insights from interview transcripts is organizing and cleaning the data. This involves transcribing audio recordings accurately, formatting the text for readability, and removing any irrelevant or redundant information. Structuring the interview transcript in a systematic manner helps marketers streamline the analysis process and focus on extracting meaningful insights.
Identifying Themes and Patterns in the Transcript From Interview Sessions
With clean and organized interview transcript at hand, the next step is to identify recurring themes and patterns within the data. This can be achieved through techniques such as coding, categorization, and thematic analysis using AI transcription tools. Through the systematic tagging of segments of text related to specific topics or ideas, you can uncover common trends, preferences, and pain points among interview participants.
Analyzing Tone and Emotion
While the words spoken in an interview are essential, so too is the tone and emotion conveyed by the participants. By paying attention to non-verbal cues, such as pauses, laughter, or changes in pitch, marketers can glean insights into the emotional drivers behind consumer behavior. Sentiment analysis tools can also be employed to quantify the overall mood of the conversation and identify areas of positivity or negativity.
Context Consideration
In addition to analyzing the content of interview transcripts, it’s crucial to consider the broader context in which the conversations took place. External factors such as cultural norms, economic conditions, and industry trends can significantly influence the perspectives shared by participants. However, contextualizing the data within its larger framework enables marketers gain a deeper understanding of the underlying drivers shaping consumer attitudes and behaviors.
Turning Insights into Action/Strategy
The true value of interview transcripts lies in their ability to inform actionable strategies and decisions. Once key insights have been identified and analyzed, the next step is to translate these findings into concrete actions. This may involve refining marketing messaging, developing targeted campaigns, or optimizing product offerings to better align with consumer needs and preferences.
Automated Transcription and Speech Recognition The first fundamental requirement for working with interview data is converting recorded audio or video files into machine-readable text transcripts for further analysis. While manual transcription is an option, automated speech recognition software powered by AI can dramatically accelerate this time-intensive process.
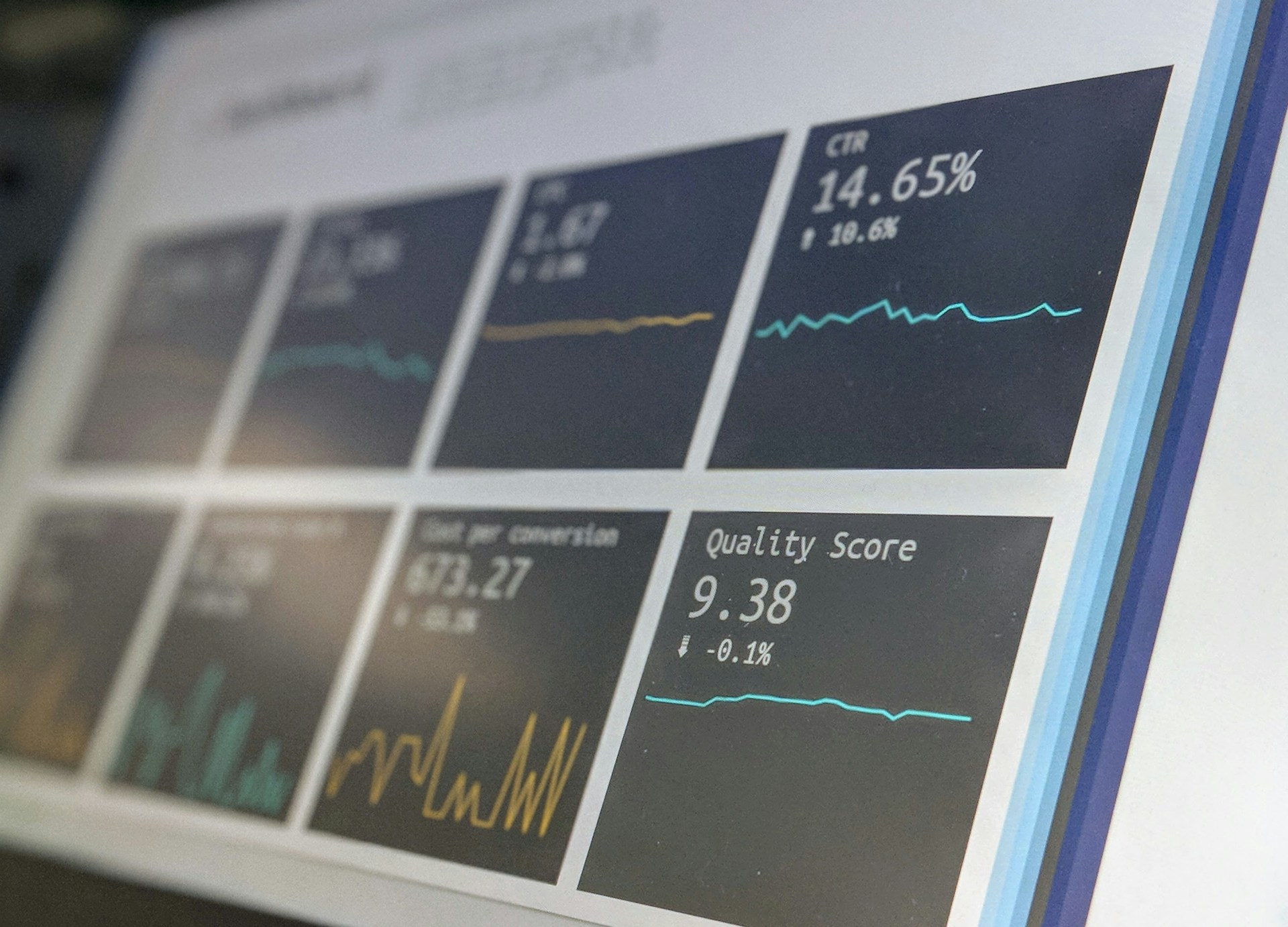
Best AI Tools For Generating and Analyzing Transcripts From Interview Data
Some of the leading automated transcription tools include:
Insight7
Insigyt7 has been described as marketers and HR managers as the best AI tool for multimodal transcription. With this popular tool, you turn your audio and video files into crisp and clear transcripts.
One of the reasons professionals like you love Insight7 is the analysis feature that helps you map sentiments and generate recurring themes from your interview transcripts. Also, you can upload and analyze up to 200 files simultaneously, and generate speaker identity, time stamps, and accurate quotes.
Get a glimpse of Insight7 in action: Watch a couple of our 1-minute demos below.
Get Started for free
Amazon Transcribe
Part of AWS’s comprehensive AI services suite, Amazon Transcribe leverages machine learning to generate text transcripts of recorded speech. It offers streaming transcriptions, multi-speaker identification, custom vocabularies, and batch transcription APIs.
Rev AI
Rev’s artificial intelligence transcription service claims up to 99% accuracy on transcripts, plus offering additional features like speaker identification and audio summarization. A self-service web interface streamlines workflows.
Otter.ai
Otter’s claim to fame is its highly interactive and shareable transcripts with AI-powered speaker labeling, seamless Zoom integration, and mobile apps. Search, player sync, and collaboration tools make exploring transcripts intuitive.
Sonix.ai
With advanced AI models tailored for accented speech, background noise filtering, and niche vocabularies, Sonix aims to provide highly accurate transcripts even for challenging audio recordings. Their pro-level tooling includes advanced time coding, multi-channel support, and translation.
These AI-driven transcription tools are affordable, scalable solutions for rapidly converting recorded interviews into text-based transcripts suitable for deeper qualitative coding and analysis. Some even provide initial categorizations or topic classifications along with just the transcript output.
An End-to-End AI Workflow for Interview Transcript Analysis
By combining all of these AI capabilities into a unified end-to-end solution, marketing teams can seamlessly operationalize advanced transcript analysis workflows to propel their research forward:
Ingestion & Coding
– Raw interview recordings from any source are securely ingested
– Custom speech recognition transcribes the audio into text data
– Trained machine learning models automatically code the transcript based on the marketer’s taxonomies for products, brands, audience segments, etc.
– Human analysts validate and tune the AI coding recommendations
– Transcript data is enriched with sentiment labels, thematic categorizations, and metadata pulled from the interview context
Insight Discovery & Synthesis
– AI models process the coded transcripts, detecting high-impact thematic clusters and underlying trends through unsupervised techniques
– Surface-level insights like sentiment breakdowns and common topics are quantified
– Granular subtopics, persona attributes, and niche customer needs are clustered and visualized
– Using large language models, the AI composes initial insight summary reports weaving together key data stories, exemplar quotes, and workflow recommendations
Exploration & Iteration
– Marketing stakeholders can then interactively explore the dataset through AI-powered search, filtering, and dynamic visualizations
– Conceptual queries enable non-linear navigation to uncover nuanced relationships
– As new questions arise, researchers can provide feedback signals to refine the AI’s understanding
– Iterated insight deliverables like presentation decks continually update to reflect the latest findings
Throughout this feedback loop between human and machine intelligence, the AI models learn to better tailor their coding precision, analysis focus areas, and insight communication structure. Performance continually increases through each research cycle for more impactful results.
Compared to legacy processes reliant on boutique service firms for manual transcript coding and analysis, this AI-driven workflow represents an exponential leap in scalability, depth, efficiency, and alignment for marketing research programs.
Get Started for free today or Book a demo
Applications Across the Marketing Lifecycle
While broad in scope, some of the most compelling AI-powered transcript analysis use cases span key marketing disciplines:
Product Innovation and UX Insights
By meticulously analyzing transcripts of product testing interviews and user experience research sessions, AI can rapidly pinpoint usability issues, feature requests, and areas of expressed friction.
AI models detect sentiment patterns that quantify resonance with each product aspect. Clustering algorithms identify distinct behavioral profiles indicative of unique requirements or underserved use cases.
The result is accelerated user research cycles, surfacing specific insights that directly impact product roadmaps and enhancement prioritization.
Brand Reputation and Perception Analysis
In this era of intensifying brand relevance battles, brand strategy teams need efficient ways to monitor brand health, resonance, and audience affinity through qualitative lenses.
AI models custom-trained to analyze transcripts from brand perception studies can isolate key drivers of brand sentiment and uncover emerging positioning opportunities. They quantify audience feelings and impressions while surfacing authentic verbatim narratives.
From competitive comparisons to messaging alignment, AI sheds light on brand reputations in highly nuanced ways for more strategic marketing activations.
Customer Experience Transformation
For marketers tasked with managing seamless customer journeys with their products or services, analyzing Voice of Customer (VoC) interviews is a constant priority.
AI can rapidly code and categorize transcripts to isolate points of friction, confusion areas, or inaccessibility from the customer’s actual words and experiences. These categorical clusters empower teams to redesign touchpoints that smooth CX friction while identifying new service models.
At scale, sentiment models and trend detection also alert teams to potential churn risks or unmet new market needs before they become critical.
Audience Segmentation and Persona Discovery
Possibly the most widely applicable AI transcript analysis use case for marketers is granular persona and micro-segment discovery.
By detecting clusters of commonalities across coded quotes and attributes, AI can rapidly surface new cohorts of customers with distinct behaviors, demographics, psychographics, journeys and marketing implications. These may represent emergent segments, opportunities for tailored messaging, or even entirely new buyer personas to target.
Rather than relying on intuition or incomplete survey data, AI provides data-driven justification for audience definitions and detailed fodder for enriching micro-segments with authenticity.
Example Persona Cohort A:
Pull top representative quotes showcasing key attributes, brand sentiments, and use cases for this segment
Beyond Marketing: Scalable Transcript Analysis Across Industries
While the applications for AI-powered transcript analysis have clear resonance for marketing teams, the potential impact extends across virtually any industry leveraging qualitative research and open-response interviewing:
Analyzing Transcript From Interview in Human Resources
Recruiters and HR teams can use transcripts of interviews with job applicants to codify key capabilities, personality traits, and patterns that signal high potential matches for certain roles.
Employee exit interviews also become rapidly analyzable to identify drivers of turnover or cultural disconnects. AI turns these datasets into actionable coaching insights.
Market Research & Consulting
Management consulting firms can rapidly analyze transcripts from proprietary market research interviews and stakeholder engagements using AI. Findings can justify recommendations with solid qualitative grounding from the most relevant sources.
User Research & Design
Similar to marketing’s UX research, teams designing digital products and applications can use AI-analyzed usability study transcripts to understand friction points, feature needs, and usage patterns in great detail.
Code-free analysis accelerates the iterative design cycle.
Customer Support & Success
On the services side, examining client support call or customer success interview transcripts through AI allows teams to quantify drivers of escalated issues or reasons for attrition.
Clusters of common problem patterns or at-risk behavior can be automatically detected for pre-emptive action.
Higher Education
University programs can use machine learning to code and analyze transcripts of student interviews regarding curriculum feedback, advising experiences, or career planning. Qualitative data guides student success initiatives.
Compliance & Whistleblower Monitoring
AI could even be applied to transcripts of whistleblower or compliance hotline interviews for highly regulated industries like finance, healthcare or government. The ability to automatically categorize issues and analyze context is invaluable.
In each of these domains and more, AI removes the bottleneck of distilling insights from rich interview datasets. Rather than sampling small batches due to limited human bandwidth, entire transcript libraries become comprehensively analyzable.
Becoming an AI-Empowered Marketing Team
For marketing leaders, analysts, and strategists looking to transform their team’s impact through more comprehensive qualitative data activation, now is the ideal time capitalize on AI-driven transcript intelligence.
While the AI transcript technology ecosystem continues to rapidly evolve, current models and solutions are already immensely useful at streamlining go-to-market research initiatives, voice of customer programs, brand perception monitoring, and more.
By leveraging AI tools like Insight7 to quickly extract an avalanche of nuanced insights from piles of transcribed interviews, marketing teams gain advantages like:
• Accelerated speed-to-insight and compressed research timelines
• Reduction in tedious manual tasks, refocusing on strategic thinking
• Superior evidence and justification quality for marketing decisions
• Deeper authenticity and differentiation in audience/product understanding
• Cross-collaboration aligned on a centralized qualitative data repository
• Ability to fully capitalize investments in qualitative research spending
Rather than gut instinct or biased sampling, the integration of machine intelligence across the transcript analysis workflow enables data-driven marketing at its most potent and efficient.
To get started, conduct an audit of your current qualitative research pipelines and data utilization processes. Pinpoint the bottlenecks and wasted investments around transcript analysis. Then begin exploring the latest AI solutions optimized for those pain points.
Most leading platforms offer proof-of-concept trials and custom demos using your actual transcript data to evaluate opportunities for ROI.
Get Started for free today or Book a demo
FAQs
What is the purpose of an interview transcript?
The main purposes of interview transcripts are to capture the full recorded conversation in text form for documentation, enable qualitative analysis by coding the data, and provide an archivable written record that can be shared among teams.
What is the best format for interview transcripts?
The most recommended formats are plain text files (.txt), word processor documents (.docx), timed formats like .vtt or .srt that sync with recordings, or structured data files like .xml or .csv. Consistent speaker labeling and metadata is important.
How do I turn an interview into a transcript?
1. Manual transcription by typing out recordings
2. Automated speech recognition and AI software like Insight7
3. Outsourcing to human transcription services
4. Combining automated tools with human editing
The ideal approach depends on factors like audio quality, budget, deadlines, and required accuracy levels. Having humans verify automated transcripts is generally advisable.