How to Analyze Interview Transcripts with AI Tools
-
Gloria
- 10 min read
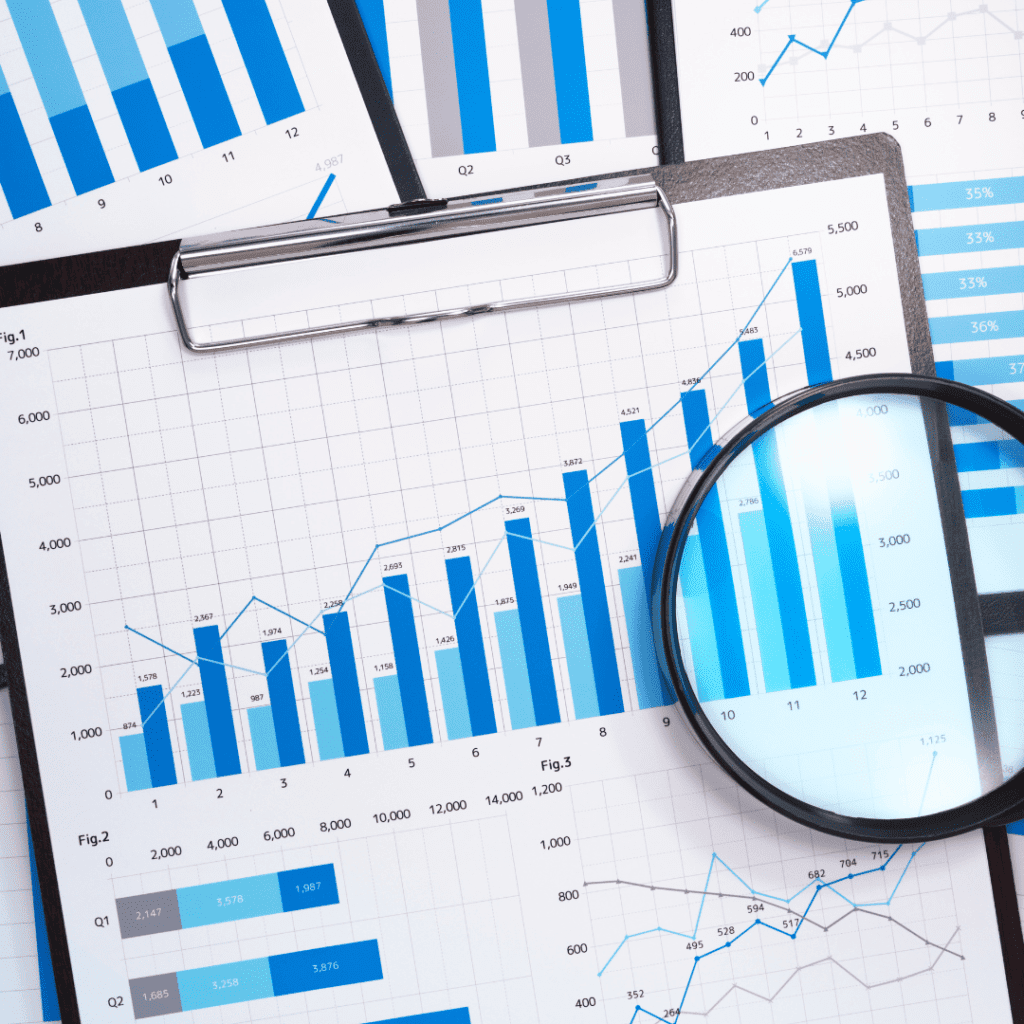
Interviews offer a goldmine of rich data – if you ask the right questions and analyze it properly. People tend to open up and reveal their authentic thoughts and emotions in those open-ended conversations. Analyzing interview transcripts manually can be an overwhelming process, especially when dealing with large amounts of data. With an AI-driven approach, organizations across industries can revamp how they gather and interpret audience feedback. For Instance
- Efficiency: AI tools can process and analyze large volumes of data quickly, significantly reducing manual effort.
- Objectivity: They minimize human biases, providing neutral, data-driven insights.
- Depth: AI enhances the ability to extract nuanced themes, sentiments, and trends that may be missed with manual analysis.
As businesses rely more on qualitative research for customer insights and decision-making, thereโs a growing need for faster, more efficient ways to analyze transcripts. AI tools have revolutionized this space, offering the ability to streamline transcript analysis while providing deeper, more accurate insights.
If you’re in market research, customer insights, or academic research, AI tools offer significant advantages like speed, accuracy, and consistency. In this guide, you’ll learn how to use AI to analyze interview transcripts effectivelyโfrom preparation to insight applicationโand explore five of the top AI tools leading the space in 2025.
By following these steps, youโll be able to enhance your analysis process, uncover actionable insights, and make informed decisions efficiently. Whether youโre a beginner or an experienced researcher, these tips will help you understand how to leverage AI for transcript analysis.
Transcribe & extract insights from interviews. At Scale.
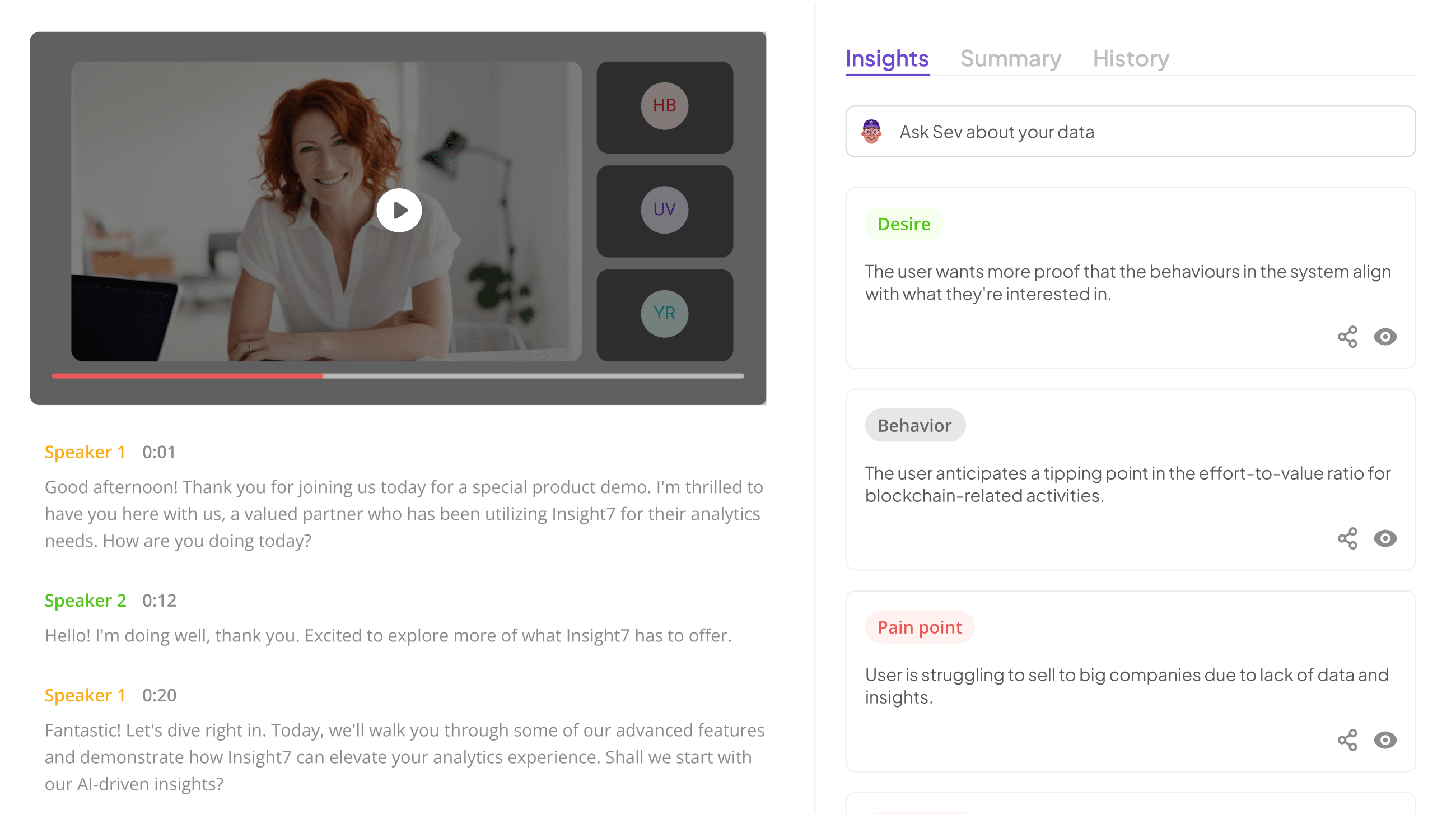
Understanding the Basics
AI-powered transcript analyzers can scan large amounts of interview data quickly, allowing you to identify themes, patterns, and emotional tones accurately. Hereโs a closer look at transcript analysis AI tools, how it works, and the primary benefits of using AI tools for interview transcript analysis.
What is Interview Transcript Analysis?
Interview transcript analysis involves reviewing the text from interviews to extract significant themes, patterns, and insights. This process typically includes identifying recurring topics, sentiment scores, and unique keywords. Traditionally, transcript analysis was labor-intensive and required detailed coding and tagging.
However, AI tools now automate much of this process by scanning text, recognizing language patterns, and highlighting key insights. Using AI for transcript analysis means you can identify themes, sentiments, and patterns in ways that were previously challenging.
Common use cases for AI transcript analysis include customer insights, product feedback, academic research, and UX research. In all of these fields, AI can speed up the process while maintaining or even improving the quality of the analysis.
Step-by-Step Guide to Analyzing Interview Transcripts with AI Tools
- Prepare interview transcripts
- Choose the right AI tool
- Set up transcript in AI tool
- Run the analysis
- Review & apply insights
Step 1: Prepare Interview Transcripts
Before using AI tools to analyze interview transcripts, it’s essential to prepare your data for optimal results. Transcript analyzers work best with well-organized and clearly formatted files. Hereโs how to ensure your transcripts are ready:
- Format Your Transcripts Consistently: Ensure all transcripts follow the same format, with clear speaker labels and timestamps where possible.
- Choose Compatible File Types: Most AI tools support common formats like PDF, DOCX, and TXT. Confirm that your files are compatible with the selected transcript analysis tool.
- Review for Accuracy: Check transcripts for accuracy, particularly if they were automatically generated. This step ensures that AI-powered analysis yields precise insights. Reviewing transcripts manually can give you an idea of key themes or sentiments, which can then be confirmed and expanded through AI analysis.
Pro Tip: Use a consistent format for each transcript to ensure your AI tool accurately identifies different speakers and sections, which can impact the clarity of insights.
Step 2: Choose the Right AI Tool for Transcript Analysis
Each research project comes with unique objectives and needs.ย Selecting the right tool is critical for effective transcript analysis. Look for AI tools with features that align with your goals, such as sentiment analysis, theme identification, and keyword extraction.
The first step to analyzing interview transcripts with AI is selecting the right tool. Not all AI tools are created equal, and choosing the right one depends on your specific needs.
- Accuracy: Accuracy is paramount in interview analysis. Look for AI tools that have been extensively tested and have a proven track record of delivering reliable results.
- Different Levels/Types of Analysis: Whether youโre working with UX research, market insights, or customer interviews, make sure the tool can handle the specific types of analysis you need, such as sentiment analysis, thematic analysis, and keyword extraction.
- Ease of use is another key consideration. Some tools, like Insight7 or Atlasti, are designed to be user-friendly, even for non-technical users. You can easily upload transcripts, run the analysis, and review results with just a few clicks, making it ideal for both beginners and experienced researchers.
- Language Support: If the interviews are conducted in multiple languages, ensure the AI tool supports all relevant languages to ensure accurate analysis across the entire dataset.
- Customization Options: Flexibility is essential when analyzing interview data as each project may require unique parameters and criteria for analysis. Choose a tool that allows customization to adapt to specific your research needs.
- Cost: Consider the budget for the research project and compare the costs of different AI tools. Strike a balance between affordability and the tool’s capabilities.
- Integration: If you are part of a larger organization, look for tools that integrate seamlessly with your existing systems which offers robust integration with platforms like Google Drive and OneDrive.
Ultimately, the right AI tool should simplify your workflow while offering powerful features like sentiment analysis, theme extraction, and comprehensive reporting.
Step 3: Upload and Set Up the Transcripts in the AI Tool
Once youโve chosen a tool, itโs time to upload your interview transcripts and set up the analysis parameters. Follow these steps:
- Upload the Files: Most AI tools allow bulk uploads, so you can process multiple interview transcripts at once. This is particularly useful for projects with a high volume of data.
- Configure Analysis Settings: Choose specific settings like sentiment scores, speaker identification, or keyword filters, depending on your analysis goals. Tools like Insight7 doesn’t require any configuration. It lets you dive right into the analysis
- Select Parameters for Analysis: Define key parameters based on the type of insights you need, such as focusing on particular keywords or grouping responses by speakers.
By setting up the right configurations, you can fine-tune the AI-powered analysis to yield insights that align with your specific objectives.
Step 4: Run the Analysis & Extract Insights
Analyzing interview data involves looking for recurring themes, patterns, and sentiments that reveal deeper insights. AI tools simplify this by automatically categorizing responses and highlighting critical points. Once your transcripts are uploaded, itโs time to run the analysis.
- Run the Analysis: Initiate the process and allow the AI tool to scan and interpret each transcript. Results are usually generated within minutes, depending on the dataset size.
- Interpret Key Metrics: Analyze sentiment scores, theme clusters, and keyword frequency to understand the dataโs tone and focus areas.
- Identify Patterns and Trends: Use the results to spot trends, such as common topics or recurring sentiments across different interviews.
- Summarize:ย Many AI tools provide summarization features that condense lengthy transcripts into brief overviews, making it easier to grasp the main ideas.
Some AI tools offers sentiment analysis that categorizes the emotional tone of responses, helping you understand not just what was said, but how it was said. This added layer of analysis provides a deeper level of understanding that goes beyond surface-level insights. Depending on the tool, you may have the option to customize the analysis parameters, allowing for greater control over the insights you receive.
For Instance, with Insight7, the process is straightforward. Once your transcripts are ready, you can initiate the analysis with a single click. The platformโs advanced AI algorithms will scan your transcripts for recurring themes, emotional tones, and specific keywords relevant to your research.
Bulk Transcribe Interviews, calls, & conversations in minutes
Step 5: Review Results & Apply Insights
Once the analysis is complete, itโs time to dive into the results & apply these findings to your business strategies. AI tools present data in various forms, from word clouds to sentiment graphs, making it easier to identify patterns at a glance.
But this is where things can get tricky. Despite the convenience, AI might not always catch nuances the way a human could.ย A sarcastic comment, for instance, might be flagged as negative when, in fact, itโs a form of humor. These subtle misinterpretations mean you canโt fully rely on AI. There’s always a layer of human oversight required, a delicate balance between trust and doubt.
Some tools, for instance, will break down your transcripts by themes, highlighting recurring words and emotional tones. You may see words like “frustrated” appear alongside “innovative,” leaving you wondering about the complexities of your interviewees’ experiences.
Did they hate the product but love the idea behind it? Or is there something else, something the AI canโt quite grasp? These contradictions in the data are uncomfortable, but they often carry the most valuable insights.Instead of rushing to smooth over these conflicts, you might find that sitting with them, even if itโs uncomfortable, brings out deeper truths.
In the end, AI tools will help you analyze interview transcripts faster and more efficiently, but they wonโt resolve the ambiguity of human emotions and experiences for you.
AI-powered insights are invaluable for making data-driven decisions in areas such as product development, customer feedback analysis, and team alignment. These applications of AI-driven transcript analysis help organizations make strategic improvements, enhancing both customer experience and internal processes.
Top 5 AI Tools for Analyzing Interview Transcripts in 2025
- Insight7.io
- NVivo
- ATLAS.ti
- MAXQDA
- Ravenโs Eye
1. Insight7.io
Insight7 is an AI-powered platform designed to transform interview transcription and analysis. It excels at automating transcription, identifying themes, detecting emotions, and extracting actionable insights using advanced natural language processing (NLP).
The platform handles structured and unstructured interviews, translating complex data into clear, usable intelligence with powerful keyword extraction, sentiment tracking, and theme identification.
With multi-language support and built-in data visualization tools, Insight7 simplifies collaboration and delivers comprehensive insights quickly, making it ideal for fast-paced projects.
Insight7 is perfect for market researchers, customer experience teams, and UX analysts who need to rapidly analyze interviews and uncover trends. Its automation reduces manual work, providing actionable insights for projects requiring quick decisions or deep analysis of customer feedback.
Key Features:
- Bulk Transcription & Upload: Analyze hundreds of interviews simultaneously.
- Sentiment & Emotion Detection: Automatically tags emotional tones and highlights mixed responses.
- Theme Discovery: Groups responses into themes like โpricing concernsโ or โonboarding pain points.โ
- Data Visualization: Generates journey maps, personas, and dashboards with one click.
- Secure Collaboration: SOC2 and GDPR-compliant, with real-time team sharing.
Benefits:
Insight7 is perfect for researchers who need fast, scalable, and secure analysis. You donโt need to code or train the systemโitโs plug-and-play. Itโs especially useful for market research, UX testing, and customer feedback analysis where time is limited and clarity is crucial.
2. NVivo
NVivo specializes in qualitative data analysis, including interview transcripts. It provides tools for coding, organizing, and visualizing data to uncover patterns and themes. NVivo supports complex queries, data linking, and integrates with various data sources, such as social media, surveys, and focus groups.
Key Features:
- Advanced Manual & AI Coding: Combines researcher-led tagging with auto-suggestions.
- Mixed-Methods Support: Integrates with surveys and quantitative datasets.
- Visual Queries: Explore complex relationships using charts and network maps.
- Import from Social & Survey Tools: Pull in content from SurveyMonkey, Twitter, and more.
Benefits:
NVivo is best suited for long-form studies where transparency and traceability are vital. While it has a learning curve, it offers unmatched flexibility for designing and documenting rigorous qualitative research.
3. ATLAS.ti
ATLAS.ti supports qualitative data analysis with tools for coding, annotating, and exploring themes in interview transcripts. It offers robust visualization tools and facilitates collaboration among research teams. The software also includes features for network analysis and literature review integration.
Key Features:
- Thematic Clustering: Uses AI to detect and group repeating phrases.
- Team Collaboration: Assign codes, comment on themes, and sync insights in real time.
- Flexible Import Options: Accepts everything from video to plain text.
- Literature Review Integration: Combine interview insights with external research.
Benefits:
ATLAS.ti is excellent for multidisciplinary teams or agencies handling client evaluations. It bridges the gap between human interpretation and AI-generated patterns, making it easy to balance qualitative depth with analytical speed.
4. MAXQDA
MAXQDA is a comprehensive tool for qualitative data analysis, including interview transcripts. It offers advanced text analysis features, visualization tools, and team collaboration capabilities. MAXQDA supports both qualitative and mixed-methods research.
Key Features:
- Visual Code Mapping: See where and how often key ideas show up.
- Mixed Methods Ready: Use for hybrid research projects combining qual and quant data.
- Sentiment Analysis Support: Identify tone shifts and intensity across responses.
- Dynamic Dashboards: Customize views for stakeholder reporting.
Benefits:
If youโre preparing presentations, reports, or academic papers, MAXQDA excels in helping you turn transcript data into engaging visuals and defensible claims.
5. Ravenโs Eye
Ravenโs Eye is an AI-driven platform for interview analysis, emphasizing natural language processing (NLP) and sentiment analysis. It is designed to uncover nuanced insights quickly and accurately. The tool supports thematic analysis and keyword extraction. Ravenโs Eyeโs emphasis on NLP and sentiment analysis allows it to provide deep insights into the emotional tone of interview responses.
Key Features:
- Emotion & Intent Recognition: Distinguishes between sarcasm, passion, or fear.
- Keyword Clouding & Filtering: Highlights dominant phrases with filters by mood.
- Thematic Modeling: Uses AI clustering to group similar thoughts and responses.
Benefits:
Ravenโs Eye is ideal when you’re exploring emotional language in-depth or working on high-stakes communication studies. It adds layers of nuance that go beyond standard positive/negative sentiment.
Common Pitfalls to Avoid in AI Transcript Analysis
While AI can streamline the interview analysis process, there are some common mistakes that can undermine your results.
- One of the biggest issues is expecting AI to do all the work: AI is a tool that aids human judgment, not a replacement for it. Misinterpreting AI-generated data is a frequent pitfall. For example, sentiment analysis might indicate negative feelings, but without understanding the contextof those sentiments, you could make misguided decisions.
- Another mistake is using the wrong AI tool for your specific needs:ย Not all AI tools are created equal. Some are better for thematic analysis, while others excel at sentiment detection or linguistic analysis.
- Picking a tool without understanding its strengths and limitations can result in incomplete or skewed data. This is why choosing an AI tool like Insight7, which offers a balance of features such as bulk transcription, theme detection, and customizable insights, is essential.
- Additionally, some users overlook the importance of properly formatting transcripts before analysis. Poorly formatted data can lead to errors in the analysis, affecting the accuracy of the results. Before uploading transcripts, ensure they are clear, with no extraneous symbols or formatting errors that could confuse the AI.
- Finally, underestimating the need for human review can lead to flawed interpretations. While AI does the heavy lifting in identifying patterns, humans must still make sense of those patterns in a meaningful way.
Combining AI efficiency with human expertise will ensure more reliable insights.
Tips for Success in AI-Driven Interview Transcript Analysis
Leveraging AI to analyze interview transcripts effectively requires strategic planning and attention to detail. Here are some expert tips for getting the most out of your AI interview analysis:
- Choose the Right AI Tool: Before anything else, select an AI tool that meets your specific needs. For example, Insight7 is ideal for researchers and businesses looking to analyze large volumes of qualitative data quickly and accurately. Its features, like bulk transcription and customizable reports, make it versatile for different projects.
- Review the Data: After AI processes the transcripts, don’t skip the manual review. Ensure that themes and sentiments identified by AI match the overall context of the interviews. Look for any subtle details the AI may have missed.
- Combine AI with Human Insights: AI will provide you with raw data and patterns, but itโs the human interpretation that gives this data meaning. Work closely with the AIโs results to derive actionable insights, and always validate the findings with a human touch.
- Optimize the Workflow: Once you get the hang of using AI tools, find ways to streamline the process. For example, setting up templates for recurring analysis can save time and ensure consistency in future projects.
- Balance Speed with Accuracy: While AI tools dramatically increase the speed of analysis, don’t sacrifice accuracy for quick results. Take the time to verify findings and ensure they align with the objectives of your research or project.
By following these tips, you’ll not only speed up your analysis process but also achieve more accurate, insightful outcomes.
Transcribe & extract insights from interviews. At Scale.
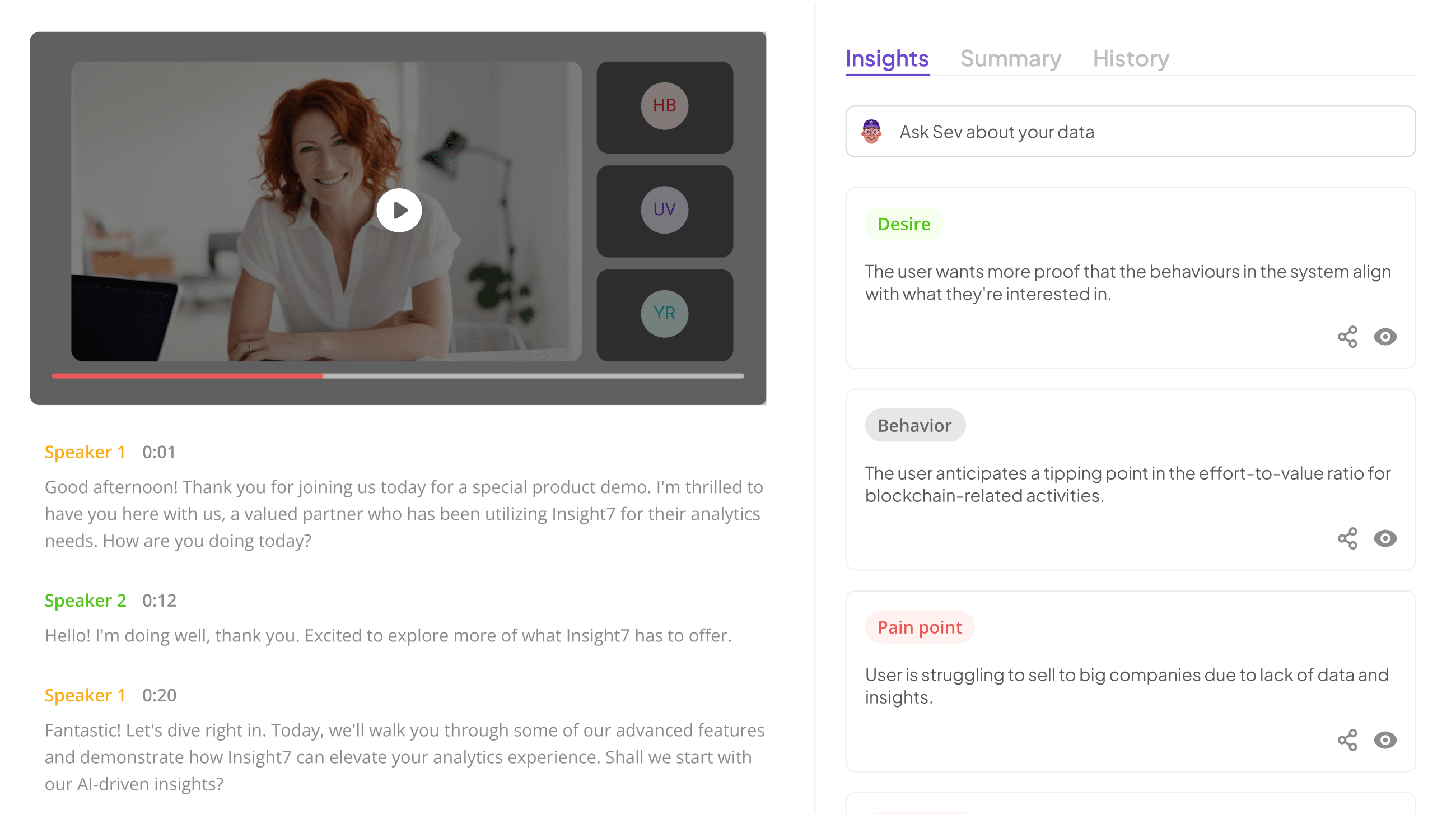
Conclusion: Why AI Tools are Essential for Interview Transcript Analysis
Analyzing interview transcripts with AI offers a faster, more efficient way to uncover meaningful insights. By following this guide, you can streamline the process, avoiding common pitfalls and applying tips for success to maximize the quality of your results.
However, itโs crucial to remember that AI is a toolโone that requires human oversight, interpretation, and judgment. By choosing the right tool, understanding its capabilities, and blending AI efficiency with human intuition, you can unlock richer insights from your qualitative data.
With Insight7, you get an AI-powered transcript analysis tool tailored to deliver actionable insights from your data, helping your organization make data-driven decisions that fuel growth and innovation.
Ready to streamline your interview analysis process? Sign up for Insight7 today and see the power of AI in action.
๐ฌ Need Help? Chat with Support
Our team typically responds within minutes