Compliance Drift Detection plays a crucial role in safeguarding businesses from regulatory pitfalls. In today's rapidly evolving market, organizations must ensure that their communication adheres to compliance standards. Call transcripts provide a rich source of data that can reveal potential drift from established guidelines. By analyzing these conversations, teams can identify discrepancies and take corrective actions swiftly, protecting both their reputation and their clients.
Understanding how to detect compliance drift through call transcripts involves a systematic approach. This process includes pinpointing key indicators of compliance and leveraging technology for efficient analysis. As organizations navigate the challenges of maintaining consistency, mastering Compliance Drift Detection not only enhances operational efficiency but also fosters a culture of accountability and transparency.
Analyze & Evaluate Calls. At Scale.
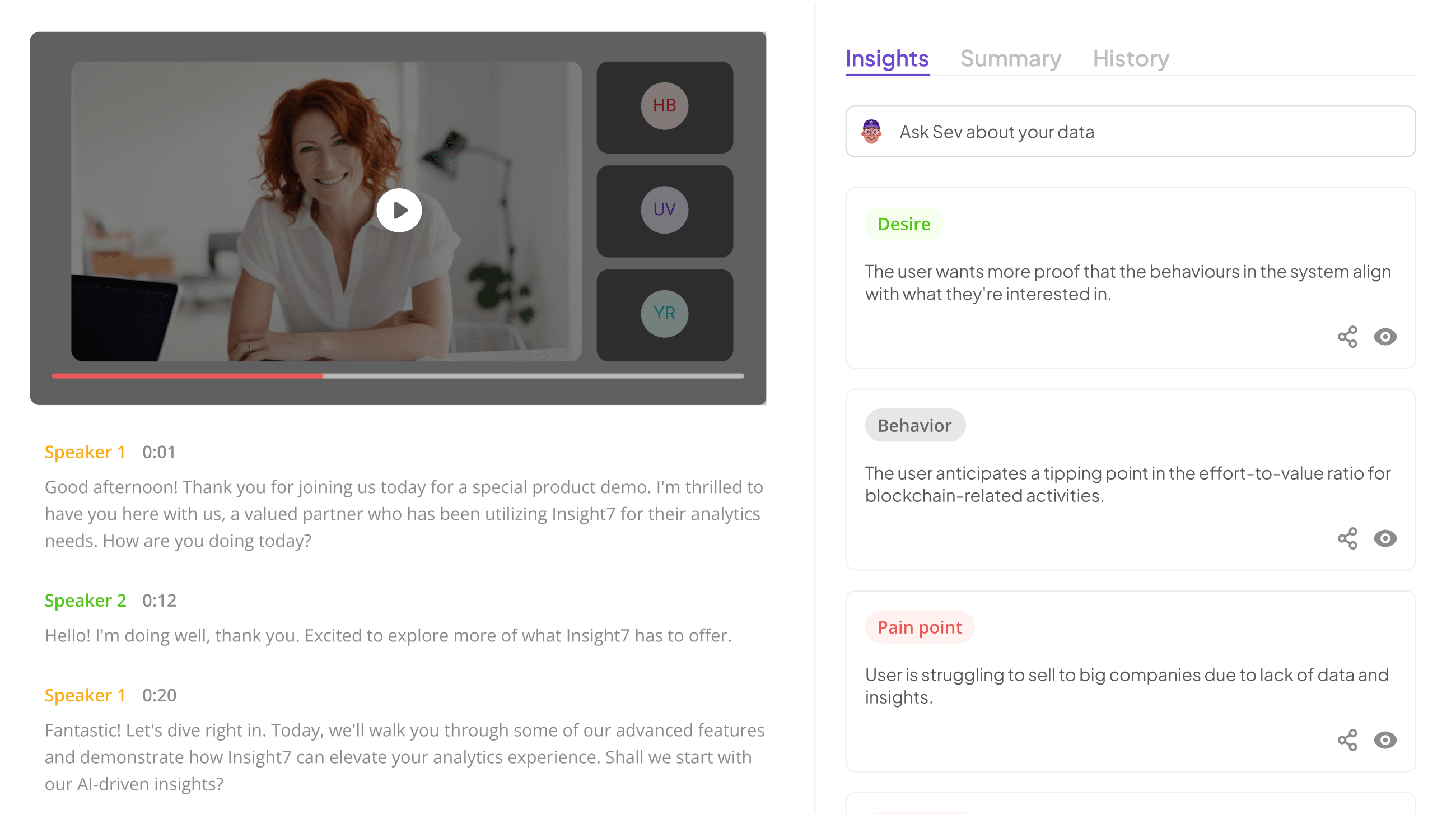
Understanding Compliance Drift Detection
Compliance Drift Detection refers to the process of identifying discrepancies between established compliance standards and actual practices evident in call transcripts. This detection process is crucial for businesses seeking to uphold regulatory requirements and deliver quality assurance. It begins with effective transcription of recorded calls, followed by a systematic analysis of the content against predefined compliance templates. By examining these transcripts, organizations can pinpoint areas of non-compliance that may lead to operational risks.
Key indicators of compliance drift include inconsistencies in adherence to protocols and failure to follow necessary guidelines. Challenges in compliance detection often arise from the sheer volume of call data, making thorough assessment cumbersome and time-consuming. To enhance effectiveness, leveraging automated tools designed for analyzing call transcripts becomes essential. By integrating these solutions, businesses can streamline their compliance drift detection efforts, ensuring that deviations are identified promptly and rectified accordingly.
Identifying Key Indicators in Call Transcripts
To effectively identify key indicators in call transcripts, itโs essential to focus on specific elements that reveal potential compliance drift. Firstly, attention should be given to the language used by agents. Key phrases or terms that deviate from established guidelines can highlight areas of concern. For instance, if an agent inadvertently suggests a product in a misleading way, it is a clear indication of possible compliance drift.
Next, assessing customer responses is crucial. The way customers react or inquire following specific statements can provide insight into compliance adherence. Misinterpretations or unexpected questions from customers may signal that the information conveyed was unclear or incorrect. Lastly, monitoring the tone and context of discussions can also serve as a vital indicator. An unsatisfactory tone may suggest discomfort with procedures, raising red flags about compliance. By meticulously analyzing these components, organizations can proactively address compliance drift, ensuring accurate monitoring and adherence to standards.
Challenges in Detecting Compliance Drift
Detecting compliance drift presents several significant challenges that organizations must navigate. One major hurdle is the variability in language used by agents during calls. Each interaction may feature unique phrases, which can mask compliance issues and complicate analysis. This inconsistency makes it difficult to establish a uniform standard for compliance drift detection. Additionally, the sheer volume of calls creates a substantial challenge in effectively monitoring and analyzing them for regulatory adherence.
Another issue involves distinguishing between acceptable deviations and genuine compliance violations. Misinterpretations can arise if the context of the conversation is not fully understood. Furthermore, reliance on manual review processes can introduce biases and errors, slowing down the identification of compliance drift. To overcome these challenges, organizations increasingly turn to advanced analytical tools that can efficiently process large quantities of transcript data, providing more consistent insights into compliance-related concerns.
Extract insights from interviews, calls, surveys and reviews for insights in minutes
Steps for Effective Compliance Drift Detection
Effective Compliance Drift Detection involves a systematic approach to ensure adherence to established guidelines and standards. The first step is transcription and data collection, where relevant call transcripts are gathered and converted into text format. This step is crucial as it forms the foundation for any further analysis. Ensuring accurate transcriptions aids in identifying compliance issues effectively.
Next, analyzing for patterns of non-compliance is vital. This involves examining the collected transcripts for recurring themes or indicators that highlight deviations from compliance standards. By methodically reviewing the data, specific trends can be identified, enabling teams to focus their efforts where needed.
Finally, implementing machine learning for detection can greatly enhance the process. By using advanced algorithms, organizations can automate the identification of compliance drift, saving time while improving accuracy. Overall, these steps create a robust framework for continual monitoring and enforcement of compliance.
Step 1: Transcription and Data Collection
To initiate the process of Compliance Drift Detection, begin by transcribing your call recordings. Transcription transforms audio files into readable text, making it easier to analyze the content for signals of compliance drift. Utilize automated tools that can efficiently handle multiple transcripts, allowing you to streamline your data collection. This foundational step ensures that all conversations are accurately documented, enabling subsequent analysis to identify any discrepancies in compliance.
Once the transcripts are generated, systematically organize the data for easy access and retrieval. Store these transcripts in a centralized library where you can reference individual conversations as needed. This organization is crucial because it allows analysts to pull insights from the transcripts effectively. By selecting specific insights to analyze, you can uncover patterns that may indicate compliance drift. This structured approach not only enhances efficiency but also supports thorough investigations into adherence to compliance standards.
Step 2: Analyzing for Patterns of Non-compliance
In this phase, the focus shifts to a pivotal aspect of Compliance Drift Detectionโanalyzing for patterns of non-compliance within call transcripts. By thoroughly assessing these conversations, organizations can uncover recurring themes or behaviors that deviated from established protocols. Start by categorizing transcripts based on specific compliance criteria, such as keyword usage, adherence to guidelines, and overall conversation quality. Noting these patterns helps to pinpoint where agents are struggling and highlights specific areas requiring additional training.
Next, it's essential to compare these findings against performance benchmarks. Identify calls that consistently fail to meet compliance standards, and evaluate the contexts in which these failures occur. You can then derive actionable insights for ongoing coaching and improvements. This analytical approach not only aids in spotting compliance drift but also empowers teams to enhance their performance continually and maintain a robust compliance framework.
Step 3: Implementing Machine Learning for Detection
To effectively implement machine learning for compliance drift detection, organizations should start by curating a quality dataset from call transcripts. This dataset serves as the foundation for training machine learning models that can identify patterns of non-compliance effectively. Feature extraction is a critical step in this process, as features may include specific phrases, tone of voice, and the context of conversations.
Next, it is essential to choose the right algorithms for model training. Supervised learning techniques, such as decision trees or support vector machines, can provide meaningful insights into compliance drift patterns. Once trained and tested, the models should be integrated into a user-friendly interface that allows compliance officers to easily access detection outcomes. Regular updates and model retraining based on new data will further enhance the systemโs accuracy in identifying compliance drift over time. By employing these steps, organizations can build a robust framework for ongoing compliance monitoring.
Top Tools for Compliance Drift Detection
For effective compliance drift detection, organizations can benefit from several top tools tailored for analyzing call transcripts. These tools help identify gaps between regulatory requirements and actual employee performance, ensuring that businesses remain compliant in their operations. By harnessing advanced technologies, companies can streamline their compliance processes and enhance the efficiency of compliance audits.
Here are some leading solutions:
Insight7: This tool offers robust analytics features, allowing users to monitor compliance through detailed insights from call data. Its user-friendly interface enables effective benchmarking against regulatory standards.
Speechmatics: Known for its accurate transcription capabilities, Speechmatics provides real-time analysis of voice conversations, thereby facilitating immediate compliance checks and adjustments.
CallMiner: This tool focuses on extracting insights from every customer interaction, helping organizations detect patterns that may indicate compliance drift effectively.
Observe.ai: By leveraging AI, Observe.ai identifies non-compliance risks in call transcripts swiftly, helping organizations take corrective action before issues escalate.
Nice inContact: This comprehensive platform integrates various compliance features, ensuring continuous monitoring and reporting capabilities across multiple channels.
Using these tools collectively will empower businesses to maintain compliance standards and foster a culture of accountability among team members.
insight7
To effectively detect compliance drift through call transcripts, organizations must first understand the importance of analyzing conversational patterns. One of the primary indicators of compliance drift is auditory inconsistencies in representative responses that deviate from established guidelines. By scrutinizing these transcripts, businesses can highlight discrepancies and identify training needs proactively.
Moreover, creating a framework for this analysis is essential. Businesses should focus on the following strategies: first, establishing benchmarks for compliance; second, utilizing advanced analytics tools to track deviations; and third, fostering a culture of continuous improvement based on transcript analysis. By integrating these methods into their processes, organizations can become more adept at Compliance Drift Detection, ensuring adherence to policies while boosting overall operational efficiency.
Speechmatics
Speech analytics, particularly through advanced transcription services, plays a critical role in compliance drift detection. These tools convert spoken dialogue into text, allowing companies to sift through vast amounts of call data. By analyzing these transcripts, businesses can identify variances in compliance adherence over time. This ability to monitor dialogues can significantly enhance the overall insights into customer interactions, thereby enabling timely decision-making based on real data.
Furthermore, effective compliance drift detection hinges on robust analysis capabilities. Utilizing sophisticated technologies can uncover subtle patterns indicative of compliance risks. Identifying shifts in customer service responses can help organizations address potential issues proactively. By implementing comprehensive solutions that seamlessly integrate speech analytics, businesses can maintain compliance integrity while ensuring consistent service quality. Ultimately, leveraging such technologies empowers organizations to safeguard their operations against compliance risks effectively.
CallMiner
CallMiner serves as an effective tool for compliance drift detection by analyzing call transcripts in detail. By sifting through extensive audio data, it helps organizations pinpoint instances where compliance may have deviated from established norms. This process involves identifying key compliance indicators embedded in conversations, allowing for a more focused approach to compliance management.
Moreover, the software integrates seamless data collection methods and advanced analytics. It filters calls based on predefined criteria, helping teams promptly identify non-compliant interactions. With each analyzed transcript, organizations can enhance their training programs, ultimately refining their compliance strategies. This proactive stance ensures that companies maintain industry standards, foster accountability, and uphold regulatory requirements effectively. As a result, using CallMiner significantly contributes to mitigating risks associated with compliance drift.
Observe.ai
The journey of detecting compliance drift can significantly benefit from the capabilities of advanced AI tools. By transforming call transcripts into actionable insights, businesses can effectively monitor adherence to compliance standards. Advanced AI-driven platforms facilitate effortless data analysis without demanding extensive expertise, ensuring accessibility for all team members involved in compliance monitoring.
One key feature is the ability to organize and analyze large volumes of call data. By clustering calls into projects, users can efficiently identify patterns related to compliance drift. The AI-powered system can automatically highlight pain points, customer desires, and other key metrics, leading to informed decision-making. Ultimately, leveraging these insights promotes a proactive approach to compliance, enabling organizations to adjust strategies quickly and maintain adherence to regulations.
Nice inContact
Nice inContact plays a pivotal role in ensuring that compliance drift detection is both effective and efficient. Utilizing advanced analytics capabilities, it enables organizations to monitor call transcripts in real-time. This provides invaluable insights into how well teams adhere to established compliance frameworks during customer interactions.
By harnessing the power of automated transcriptions, Nice inContact allows for easier identification of compliance gaps. The platform's sophisticated machine learning algorithms analyze the transcripts for inconsistencies and deviations from compliance standards. As a result, businesses can swiftly address potential issues before they escalate, ensuring adherence to regulations and maintaining customer trust. Moreover, this proactive approach to monitoring ensures that organizations continue to improve their service quality, ultimately enhancing the customer experience while safeguarding compliance.
Conclusion: Ensuring Robust Compliance Drift Detection
To ensure robust compliance drift detection, organizations must adopt a strategic approach that integrates various analytical methods. Relying solely on keywords can limit the effectiveness of compliance monitoring. Developing a comprehensive understanding of the specific regulatory requirements and potential compliance risks is essential in this process.
Moreover, it is critical to establish a systematic framework for analyzing call transcripts. By flagging calls based on identified key indicators, organizations can better manage compliance drift. Ultimately, consistent monitoring and adjustment, combined with insightful analysis, will enhance compliance drift detection, allowing for timely interventions and fostering a culture of continuous compliance improvement.