How to Compare Transcription Accuracy Without Manual Review
-
Hello Insight
- 10 min read
Automated Accuracy Assessment in transcription represents a groundbreaking shift in how we ensure quality in transcription services. Manual review processes, often time-consuming and error-prone, can be supplemented or even replaced by automated methods, offering increased efficiency and accuracy. This technology enables organizations to swiftly analyze vast volumes of transcribed data while maintaining stringent standards for accuracy.
As we explore the methodologies and tools available for automated assessment, it becomes clear that incorporating these innovations can improve the overall quality of transcriptions. By utilizing advanced algorithms and machine learning, businesses can achieve a new level of precision and consistency without the burdens of traditional manual checks. Thus, the future of transcription accuracy looks not just automated but markedly improved.
Analyze & Evaluate Calls. At Scale.
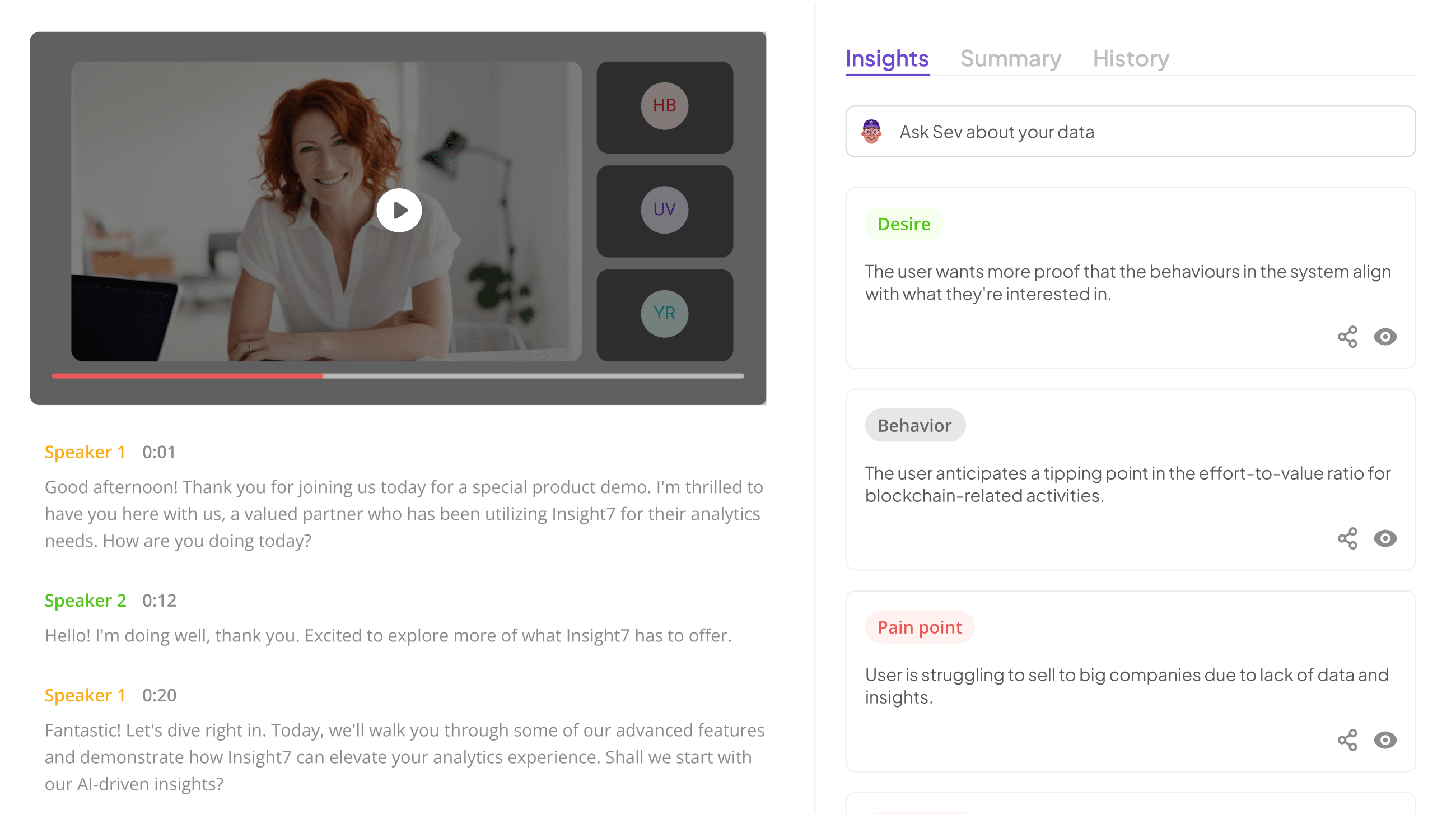
Understanding Transcription Accuracy Metrics
Understanding transcription accuracy metrics is essential for evaluating performance in automated systems. Accurate transcription is crucial for effective communication and data analysis, as it can significantly influence decision-making processes. Various metrics can be utilized to assess accuracy, including Word Error Rate (WER), Sentence Error Rate (SER), and Real Time Factor (RTF). These metrics help quantify the transcription's performance relative to the original audio content.
Automated Accuracy Assessment offers numerous advantages over traditional manual review methods. This approach streamlines the evaluation process, reducing the time and resources needed for verification. Furthermore, it enables the consistent application of criteria across vast datasets, enhancing comparability. Familiarizing yourself with these metrics ensures that you can effectively gauge transcription performance, allowing for informed decisions about which systems meet your quality standards. By prioritizing efficient evaluation methods, organizations can enhance their overall productivity and bring accuracy to the forefront of their transcription processes.
Key Performance Indicators for Transcription
To accurately assess transcription performance, it's vital to establish clear Key Performance Indicators (KPIs). These indicators should reflect essential attributes of transcription, such as accuracy, completeness, and turnaround time. Automated Accuracy Assessment can help quantify these KPIs, making it easier to compare various transcription outputs without manual review.
Assessment begins with identifying specific performance metrics. Accuracy can be measured by comparing the transcribed text against a standard or reference. Completeness evaluates whether all the spoken content has been accurately captured. Turnaround time monitors the speed of transcription completion. Each KPI should be weighted based on its relevance to your goals, allowing for a comprehensive scoring system that enhances overall understanding. Ultimately, these KPIs serve as a benchmark for transcription quality, ensuring decision-makers can trust the data they receive.
Limitations of Manual Review for Accuracy Assessment
Manual review of transcription accuracy often falls short in several significant ways. Firstly, relying on human reviewers introduces the potential for subjective bias and inconsistency. Each reviewer may interpret and score the same data differently, leading to unreliable results. This variability can undermine the integrity of the assessment, particularly in complex scenarios where context is critical. Additionally, manual review is inherently time-consuming, which can delay feedback and performance evaluations.
Another limitation concerns scalability. As the volume of transcribed data increases, the resources required for thorough manual review can become overwhelming. Automated accuracy assessment mitigates this issue by streamlining the process and allowing for instant evaluations. By embracing technology, organizations can ensure a more objective analysis of transcription quality, enabling them to focus on insights rather than inaccuracies. In the shifting landscape of transcription services, it becomes evident that automated methods can lead to more reliable and efficient accuracy assessments.
Extract insights from interviews, calls, surveys and reviews for insights in minutes
Methods of Automated Accuracy Assessment for Transcription
Automated Accuracy Assessment in transcription employs advanced technologies to ensure high-quality results efficiently. One prevalent method utilizes AI and machine learning, which streamlines the assessment process. First, pre-processing audio files is essential; this step enhances the quality of input data before it undergoes analysis. It involves noise reduction, segmentation, and normalization, making transcriptions more reliable.
Next, implementing speech recognition models takes center stage. These models transcribe the audio into text while measuring accuracy against established benchmarks. Additionally, employing benchmarking tools, such as Google Cloud Speech-to-Text and IBM Watson Speech to Text, allows for comprehensive comparisons of transcription results. By systematically evaluating transcriptions against these benchmarks, organizations can ascertain precision without manual review, ensuring faster turnaround times and better resource allocation.
Utilizing AI and Machine Learning
Employing AI and machine learning technologies can significantly enhance automated accuracy assessment in transcription tasks. These advanced techniques offer a systematic approach to evaluating transcription quality without cumbersome manual reviews. By utilizing AI models, companies can process and analyze large volumes of audio data efficiently, enabling quicker feedback loops for transcript accuracy.
To implement this effectively, organizations can adopt the following strategies. First, pre-processing audio files is crucial to improve speech recognition outcomes. This step may involve noise reduction and normalization, which ensure clearer audio input. Next, implementing robust speech recognition models enhances transcription accuracy by leveraging machine learning algorithms tuned to understand different accents and speech patterns. These steps collectively streamline the transcription process, delivering reliable results while minimizing human intervention.
Step 1: Pre-processing Audio Files
Pre-processing audio files is an essential initial step in achieving effective automated accuracy assessment. This process begins with organizing and formatting audio files for optimal transcription results. It is critical to ensure that the audio quality is clear and consistent, as this significantly impacts the transcription's accuracy. Consider normalizing the volume and removing background noise, which can enhance recognition accuracy in subsequent steps.
Additionally, segmenting longer files into smaller, manageable chunks can further improve the transcription process. Breaking down the audio helps with clarity and allows for easier handling during transcription. This method facilitates a more efficient analysis, enabling better extraction of insights from the conversations within those audio files. By diligently pre-processing audio files, we lay a strong foundation for the automated accuracy assessment, ensuring that the subsequent transcription efforts yield reliable and actionable results.
Step 2: Implementing Speech Recognition Models
To implement effective speech recognition models, start by selecting the appropriate technology tailored to your specific audio input types. Various models excel at different accents, languages, and recording qualities, so understanding your requirements is crucial for optimal performance. Once you have identified the suitable model, feed it your audio data to initiate the transcription process. This is where Automated Accuracy Assessment becomes vital; it enables a thorough evaluation of the transcription output without manual intervention.
Next, focus on evaluating the transcription results against the predetermined quality indicators. This step often involves comparing transcriptions with a validated dataset to gauge accuracy. Fine-tuning the models based on performance feedback helps improve future transcriptions. By continually iterating on this process, you enhance the accuracy of your speech recognition systems, establishing robust methods for automated assessments.
Implementing Benchmarking Tools
Implementing benchmarking tools for transcription accuracy involves several strategic steps. First, it’s essential to choose a reliable automated accuracy assessment framework. This framework should leverage various automated systems to compare transcription outputs against a set of predefined accuracy metrics. Utilizing established benchmarking tools like Google Cloud Speech-to-Text or IBM Watson Speech to Text can facilitate this process by providing comprehensive data and performance insights.
Next, integrating these tools into your operation is crucial for continuous improvement. Establish a routine for gathering performance data, analyzing discrepancies in transcription accuracy, and adapting your approach based on feedback. This proactive stance will not only enhance transcription reliability but will also support ongoing training for transcription models. By harnessing these tools, organizations can effectively monitor performance and gain valuable insights without the need for manual reviews, ultimately leading to improved efficiency and accuracy in transcription processes.
insight7
Automated Accuracy Assessment advances transcription analysis by utilizing advanced technologies to evaluate and compare transcription outputs without the need for manual review. This innovation streamlines the process significantly, reducing the time and resources required to achieve accuracy. By deploying AI-driven solutions, transcription services can analyze large data sets efficiently, providing rapid feedback on accuracy rates.
The process involves several key components. First, speech recognition models are employed to process audio files, rendering transcription results that are then evaluated against established benchmarks. These benchmarks ensure that outputs meet desired accuracy levels, enabling organizations to maintain high standards. Ultimately, Automated Accuracy Assessment empowers businesses to transform their data analysis processes, enhance insights, and make better-informed decisions based on accurate transcriptions.
Google Cloud Speech-to-Text
Google Cloud Speech-to-Text provides an advanced solution for transcription needs, enabling users to convert audio files into written text efficiently. This service utilizes state-of-the-art algorithms that enhance transcription accuracy significantly, making it ideal for various applications. Through its robust automated systems, users can bypass the traditional manual review processes, thus saving time and resources.
When assessing transcription accuracy, the service offers automated accuracy assessment features that simplify the evaluation process. By leveraging speech recognition technology, it automatically transcribes audio files, allowing users to generate comprehensive transcripts without labor-intensive manual input. Additionally, it integrates well with other tools to facilitate advanced analysis, enabling businesses to derive valuable insights from their transcription data easily. Ultimately, Google Cloud Speech-to-Text revolutionizes how transcription is approached, ensuring higher accuracy rates and streamlined workflows.
IBM Watson Speech to Text
The functionality of advanced speech recognition systems can be a game changer for businesses seeking to streamline their transcription processes. One such tool makes it easy to transcribe audio files by allowing users to upload multiple recordings at once. This automated approach reduces time spent on manual tasks and increases accuracy.
Following the transcription, users can engage in a detailed analysis of the content. With features designed for easy extraction of insights, users can quickly identify key themes and pain points from calls. The system can generate summaries, highlight keywords, and even provide visual representations of the data. This way, users can not only transcribe but also assess the quality and relevance of their transcripts efficiently with minimal human intervention. By embracing automated accuracy assessment, organizations can ensure they derive maximum value from their spoken data.
Microsoft Azure Speech Service
The Microsoft Azure Speech Service provides robust capabilities for automated transcription accuracy assessment, streamlining the evaluation process. By leveraging advanced AI and machine learning technologies, users can assess transcription precision without manual oversight. This service identifies various speakers, enhances recognition with contextual information, and offers detailed scorecards to track performance metrics. Such functionality significantly increases accuracy, ensuring that users can rely on it for various applications, from compliance reviews to customer service evaluations.
Key features of this service include the ability to process audio files in real-time, which allows organizations to gain insights swiftly. Additionally, it generates comprehensive reports that can be easily edited for compliance purposes. Therefore, businesses can focus on actionable insights rather than spending resources on manual reviews. By adopting automated accuracy assessment techniques, users can enhance efficiency and reliability in their transcription tasks.
AWS Transcribe
AWS Transcribe offers an efficient solution for achieving transcription accuracy through automated processes. This service enables users to convert audio recordings into written text, significantly reducing the time and effort required for manual transcription. With its intuitive interface, users can upload multiple files simultaneously, making it ideal for bulk analysis. This feature eliminates the logistical challenges of handling diverse audio formats and allows for quick access to transcripts.
Automated accuracy assessment becomes crucial when evaluating the output of transcription services like AWS Transcribe. By utilizing built-in algorithms, users can streamline their analysis, pulling insights without the need for in-depth manual review. This service not only enhances processing speed but also ensures consistency and reliability in the transcription results. Thus, organizations can harness automated tools to facilitate a more informed decision-making process, ultimately improving overall operational efficiency.
Conclusion: Embracing Automated Accuracy Assessment for Transcription
Automated Accuracy Assessment revolutionizes how we evaluate transcription results by eliminating the need for manual review. This method not only saves significant time but also improves precision, ensuring that the transcripts align closely with the original audio. By harnessing the power of artificial intelligence, organizations can easily implement this system, gaining insights that manual assessments may overlook.
Moreover, as automated assessment tools become more sophisticated, their ability to refine accuracy through continuous learning enhances the overall transcription process. This shift towards embracing technology not only augments productivity but also empowers teams to focus on strategic tasks rather than tedious evaluations. Transitioning to Automated Accuracy Assessment means unlocking new potential in both quality and efficiency in transcription activities.