How to Analyze Longitudinal Interview Data with AI
-
Bella Williams
- 10 min read
Longitudinal Interview Analysis provides a rich avenue for understanding changes and evolution in participants' perspectives over time. Traditionally, analyzing such qualitative data can be labor-intensive and subject to researcher bias, resulting in inconsistencies and delays. Many teams find themselves overwhelmed by the manual processes required to synthesize large volumes of data, often leading to missed insights or errors in reporting.
Incorporating AI technologies into Longitudinal Interview Analysis can vastly improve efficiency and accuracy. AI tools can automate coding processes, identify emerging themes, and highlight trends that may not be immediately apparent. By leveraging these technologies, researchers can enhance the depth of their analysis while saving valuable time, ultimately leading to more reliable insights to inform decisions.
Analyze & Evaluate Calls. At Scale.
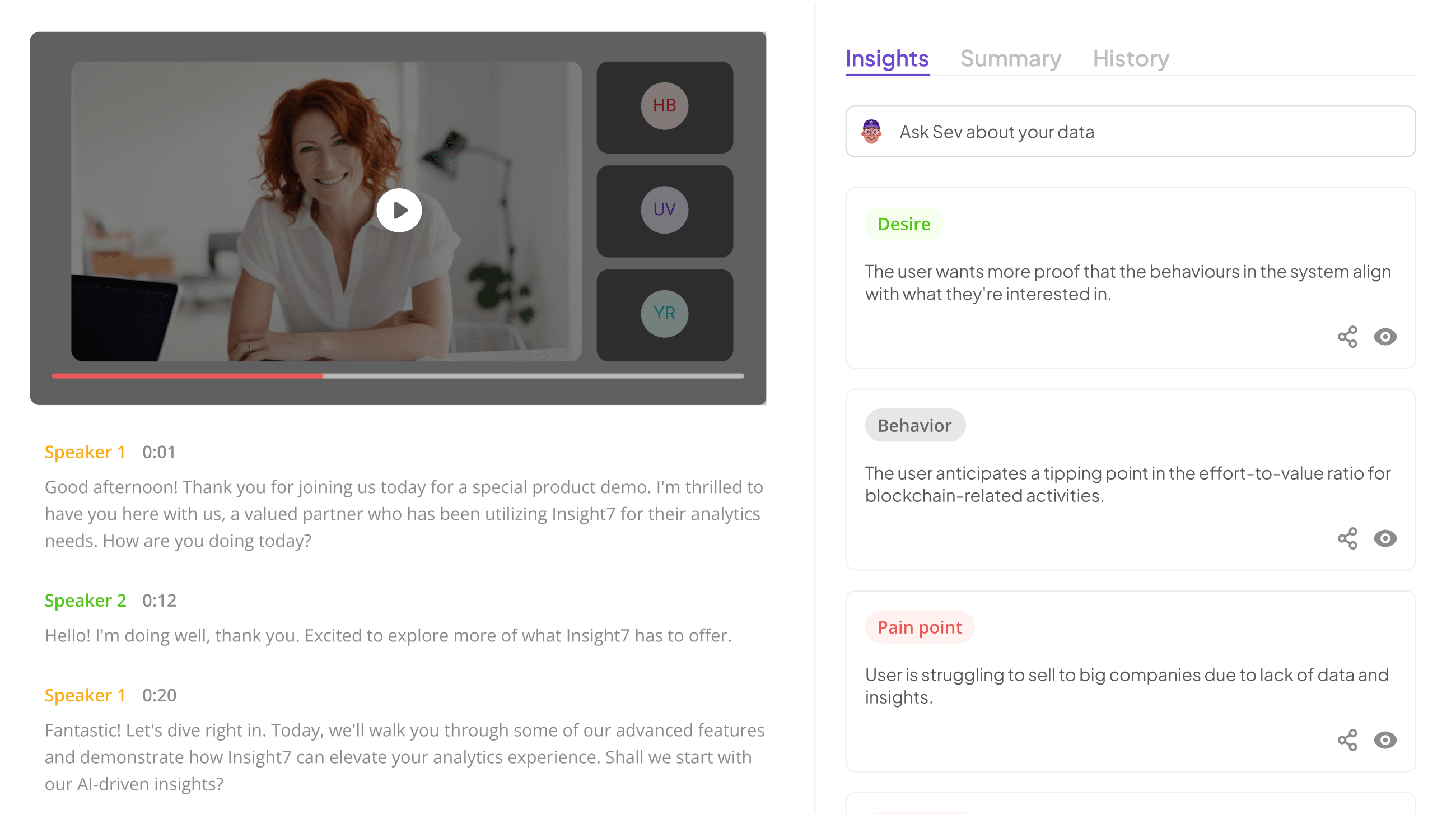
Setting the Context: Longitudinal Interview Analysis with AI
Longitudinal Interview Analysis involves the systematic study of interview data collected over time to understand trends and patterns. This technique provides deeper insights compared to traditional methods, but also poses challenges such as time consumption and potential biases. Such inconsistencies often arise due to the subjective nature of qualitative analysis, leading to varying interpretations among team members. Thus, researchers increasingly seek efficient methods to enhance the accuracy and speed of their insights.
The integration of AI within this analytical framework can significantly enhance the process. AI technologies can automate various stages of data processing, reducing manual effort and minimizing bias. By leveraging machine learning algorithms, researchers can quickly identify themes and trends within the collected data. This not only accelerates report generation but also improves the reliability of the insights derived from longitudinal interviews. Adopting an AI-driven approach empowers teams to focus on critical analysis, ensuring more valuable outcomes in their research initiatives.
Understanding the Basics of Longitudinal Interview Analysis
Longitudinal Interview Analysis involves examining interview data collected over time to uncover insights about participants' experiences and perceptions. This analysis helps researchers identify changes in attitudes, behaviors, or opinions as they evolve. Understanding the nuances of this analysis is vital, as it allows for more informed interpretations and recommendations based on the data trends observed throughout the study.
However, traditional methods of conducting Longitudinal Interview Analysis often present challenges. Manual analysis can be time-consuming and prone to bias, particularly when multiple researchers are involved. As each analyst may interpret data differently, the consistency and accuracy of findings can vary significantly. By understanding these fundamentals, researchers can better approach the potential of AI in enhancing the efficiency and reliability of their analyses, ultimately leading to richer insights.
- Definition and significance
Longitudinal Interview Analysis involves the study of data collected through interviews over extended periods. This method captures changes and trends in participant perspectives, crucial for in-depth understanding in various fields such as psychology, sociology, and market research. The significance of this analysis method lies in its ability to reveal longitudinal data patterns, providing valuable insights into how opinions and behaviors evolve over time.
Moreover, the importance of Longitudinal Interview Analysis becomes prominent when addressing complex topics that require long-term observation. By utilizing AI technology, researchers can efficiently analyze vast amounts of interview data, identify themes, and generate actionable insights quickly. This capability not only enhances the research quality but also accelerates the decision-making process in sectors reliant on evolving consumer insights. Ultimately, embracing this analytical approach empowers organizations to make informed choices based on a comprehensive understanding of trends.
- Challenges faced in traditional analysis
Traditional analysis of longitudinal interview data often presents several significant challenges that can hinder effective outcomes. Firstly, the manual process of analyzing interviews can be incredibly time-consuming. Researchers frequently find themselves sifting through hours of audio or transcript data, making it difficult to generate timely and relevant insights. This slow pace is increasingly problematic as clients demand quicker turnarounds on their reports.
Secondly, inconsistency often arises when multiple analysts are involved in interpreting the same data. Different researchers may have unique approaches to analysis, leading to varying conclusions and potential biases. This inconsistency not only complicates results interpretation but can also diminish the reliability of insights generated. Addressing these challenges is crucial for enhancing the effectiveness of longitudinal interview analysis, paving the way for AI-based solutions that streamline these tasks while minimizing human error.
AIโs Role in Streamlining Longitudinal Interview Analysis
AI significantly enhances the process of analyzing longitudinal interview data, making it faster and more efficient. Traditional analysis often involves manual review, which can be tedious and inconsistent among different researchers. Analysts frequently find themselves overwhelmed by the sheer volume of data, leading to delays in generating meaningful insights. By implementing AI tools, organizations can streamline their workflows, minimize biases, and ensure consistency in data analysis.
AI technologies, such as natural language processing, can automatically extract themes and insights from transcribed interviews. This capability not only saves time but also enhances the accuracy of findings. Additionally, AI can facilitate the identification of trends across multiple interviews conducted over time, providing deeper contextual understanding. Organizations that harness these AI-driven processes can deliver timely, reliable insights, ultimately improving their decision-making and strategic planning.
- Advantages of using AI
Integrating AI into Longitudinal Interview Analysis offers substantial advantages that can enhance both the depth and accuracy of insights derived from interview data. AI can efficiently handle large datasets, providing an ability to identify patterns that may not be immediately obvious through traditional analysis methods. Moreover, automated processes can save valuable time, allowing researchers to focus on nuanced interpretations.
Utilizing AI tools facilitates the systematic coding of qualitative data, ensuring consistency and reducing potential biases during analysis. With the capability to perform sentiment analysis and detect underlying themes, AI can greatly enrich the insights gained from longitudinal interviews. Improved accuracy and efficiency not only lead to more reliable results but can also help researchers better capture the evolving narratives of participants over time. Ultimately, these advantages bolster the overall quality and applicability of the findings in decision-making processes.
- Key AI technologies at play
When discussing Key AI technologies at play in the realm of Longitudinal Interview Analysis, several innovative methodologies stand out. First, Natural Language Processing (NLP) allows researchers to analyze vast amounts of textual data efficiently. Through techniques such as sentiment analysis and topic extraction, NLP helps identify recurring themes and emotional undercurrents in interview transcripts, enhancing understanding of participant experiences.
Second, machine learning algorithms can automate pattern recognition within this data. By training models on previous interviews, these algorithms can predict trends and suggest correlations that might not be immediately evident to human analysts. Third, automated summarization tools provide concise overviews of lengthy interviews, making it easier to digest critical insights quickly. Collectively, these AI technologies revolutionize data analysis by increasing accuracy, saving time, and uncovering deeper insights from longitudinal studies.
Extract insights from interviews, calls, surveys and reviews for insights in minutes
Steps to Effectively Analyze Longitudinal Interview Data with AI
To effectively analyze longitudinal interview data with AI, start by focusing on data collection and preparation. This involves ensuring high data quality through consistent and organized transcription of interviews. Properly structuring this data is vital, as it lays the groundwork for subsequent analysis. When interviews are consistent and well-organized, AI can be employed more efficiently to uncover deeper insights.
The next step involves applying the right AI models for insight generation. Select models tailored to your specific needs, and invest time in training them with your longitudinal data. Regularly testing these models is crucial for accuracy and relevance. Finally, interpreting AI outputs in longitudinal interview analysis requires careful attention. Look for trends and patterns in the insights generated, and address any ethical considerations related to data interpretation. By following these steps, organizations can streamline their analysis processes and derive timely, useful insights from their longitudinal interview data.
Step 1: Data Collection and Preparation
Data collection and preparation are crucial first steps in Longitudinal Interview Analysis. Collect high-quality data to ensure accurate insights. This involves recruiting participants who represent your target demographics and conducting thorough interviews. As you gather this data, pay attention to factors such as the clarity of questions, interview techniques, and the participants' responses. Effective preparation also involves transcribing recorded interviews into a structured format, enabling seamless analysis later.
Structuring your dataset is equally important. Organize the data by categorizing responses according to themes or topics that emerge during interviews. This organization allows for easier identification of trends over time. Additionally, consider utilizing transcription tools to enhance accuracy and efficiency. After collecting and structuring your data, you'll be well-equipped to proceed with AI-driven analysis, unlocking meaningful insights from longitudinal interviews. The quality of your initial data will significantly influence the reliability of your results.
- Ensuring data quality
Data quality is paramount in Longitudinal Interview Analysis, as it directly impacts the validity of insights generated from such data. To ensure accuracy, researchers must prioritize meticulous data collection and maintain comprehensive documentation during each interview session. Clear transcription methods play a significant role, as any errors in this phase can lead to misinterpretations in later analysis.
Furthermore, continuous validation of the collected data is crucial. This can involve cross-verifying transcripts against audio recordings and conducting periodic checks to identify any inconsistencies. Implementing a robust coding framework also aids in systematically organizing data, ensuring that the analytical process is grounded in reliable and accurate information. By maintaining high data quality standards, researchers can fully leverage AI tools to extract meaningful insights, ultimately enhancing the relevance and applicability of their findings.
- Structuring interview data for AI analysis
Structured interview data forms the foundation of effective longitudinal interview analysis, facilitating consistent insights over time. The first step involves organizing raw interview transcripts into manageable segments. This process includes coding responses based on thematic relevance and categorizing them for easier retrieval. Clear structure enables AI tools to analyze data efficiently, extracting themes and patterns with greater precision.
Another crucial aspect is the standardization of data formats. Converting transcripts into structured formats, such as CSV or JSON, optimizes AIโs ability to process and interpret the data. Finally, incorporating metadata, like participant demographics and interview dates, enriches context for analysis. By following these steps, researchers can significantly enhance the efficacy of AI models in their longitudinal interview analysis, resulting in faster, more accurate insights that drive informed decisions.
Step 2: Applying AI Models for Insight Generation
In Step 2 of analyzing longitudinal interview data with AI, the focus is on applying AI models to generate valuable insights. Selecting the right AI model is crucial, as different models bring various capabilities to the analysis process. For instance, natural language processing (NLP) models can analyze textual data, while machine learning models can identify patterns and trends across datasets. Carefully consider your research objectives when choosing a model, ensuring its strengths align with your analysis needs.
Once the appropriate model is selected, the next stage is training and testing it on your interview data. Training typically involves feeding the model with examples to improve its predictive accuracy. Testing subsequently evaluates its performance against a separate dataset. This systematic approach enables you to refine the model, ensuring it delivers relevant findings during longitudinal interview analysis. By leveraging AI, insights can be extracted more efficiently, paving the way for informed decisions based on comprehensive data interpretations.
- Choosing the right AI model
Selecting the right AI model is crucial for effective longitudinal interview analysis. Different models possess unique strengths, making it essential to align your model choice with your specific analytical goals. Begin by identifying the key features of each model, such as natural language processing capabilities or ability to recognize patterns. Consider whether your data requires qualitative insights, quantitative analysis, or a combination of both. Understanding these distinctions will help you narrow down the options available to you.
Next, assess the modelโs performance with your particular data set. Factors like accuracy, scalability, and ease of use are paramount. A model that excels in handling large volumes of data while delivering precise insights will enhance the efficiency of your analysis. Moreover, evaluating the integration capabilities with existing tools will streamline your workflow. Ultimately, choosing the right AI model will empower you to unlock valuable insights from your longitudinal interview data, informing your research and decision-making processes.
- Training and testing the model
Training a model for Longitudinal Interview Analysis involves multiple steps designed to ensure accuracy and reliability. It begins with selecting an appropriate dataset from previously gathered interviews, which is then cleaned and formatted. The goal here is to prepare the data so that the AI can recognize patterns and themes effectively.
Once the data is ready, the model is trained using algorithms specifically suited for qualitative analysis. During this phase, various performance metrics are applied to evaluate the model's accuracy. Testing follows training, where the model is assessed using a different subset of data to measure its predictive capabilities. Metrics such as precision, recall, and F1 score help gauge how well the model captures insight from longitudinal interviews, ensuring that the final outputs are both valid and actionable.
By rigorously training and testing the model, researchers can confidently leverage AI to extract nuanced insights from their interviews over time. This structured approach lays the groundwork for a robust Longitudinal Interview Analysis process, paving the way for meaningful findings.
Step 3: Interpreting AI Outputs in Longitudinal Interview Analysis
When interpreting AI outputs in longitudinal interview analysis, understanding the generated insights is crucial. This step involves examining trends and patterns within the data, allowing researchers to identify significant themes and shifts over time. An AI system can reveal connections that may not be immediately apparent through manual analysis, offering a broader perspective on participant responses. Additionally, it helps highlight emerging trends that could influence future research directions.
Another important aspect to consider is the ethical dimensions surrounding AI outputs. Researchers must ensure that the interpretations respect participant privacy, objectivity, and contextual accuracy. They should actively engage in questioning the AI's findings, verifying patterns against qualitative insights, and addressing any biases that may arise in the analytical process. By combining AI outputs with grounded understanding, the integrity and depth of longitudinal interview analysis can be greatly enhanced.
- Analyzing trends and patterns
Analyzing trends and patterns within longitudinal interview analysis is crucial for unveiling insights over time. By examining data collected at different intervals, researchers can identify changes in participant attitudes, behaviors, and experiences. This longitudinal approach enables a deeper understanding of dynamics that might not be visible in cross-sectional studies.
Identifying recurring themes across interviews involves coding responses and categorizing them into significant buckets. For example, you might find that sentiments around collaboration evolve as project timelines progress. Applying AI tools can enhance this process by automating the identification of these themes, allowing for a more efficient analysis. These technologies can analyze sentiment, cluster insights, and even trace back to original transcripts, thus promoting transparency and reliability in your findings. Recognizing these trends not only refines the understanding of participant experiences but also aids in making informed decisions based on robust data.
- Addressing ethical considerations
When conducting Longitudinal Interview Analysis, ethical considerations must be at the forefront of the process. Participant consent is paramount; interviewees should be fully informed about how their data will be used, stored, and shared. This transparency fosters trust and empowers participants to provide honest insights. Additionally, measures should always be taken to anonymize sensitive information, ensuring that individual identities remain protected after the analysis.
Moreover, ethical considerations extend beyond participant privacy. Researchers must also be aware of biases that might arise from the AI tools employed for analysis. These tools should be designed to treat all data fairly and without discrimination. By actively monitoring for potential biases in AI models, researchers can safeguard the integrity of their Longitudinal Interview Analysis. In this way, ethical responsibility becomes integral to producing reliable and trustworthy research outcomes.
Top AI Tools for Longitudinal Interview Analysis
When considering the most effective AI tools for longitudinal interview analysis, itโs crucial to explore a variety of options that cater to different analytical needs. The right tools can significantly enhance the efficiency and accuracy of your analysis by automating labor-intensive tasks while also generating deeper insights.
First among the options is Insight7, a robust platform that simplifies data collaboration and visualization. Its features empower users to track progress and obtain real-time updates on interview findings. Additionally, NVivo offers a comprehensive solution for qualitative data analysis, providing powerful tools for coding and analyzing text data. Dedoose streamlines mixed-method research, allowing users to integrate qualitative and quantitative data seamlessly. Other notable tools include Atlas.ti, known for its intuitive interface, MAXQDA, praised for its rich feature set, and QDAMiner, which excels in organizing and summarizing findings. Each of these tools plays a vital role in enhancing longitudinal interview analysis by providing unique functionalities to meet diverse research needs.
Insight7
In Insight7, we explore how to harness the power of AI for longitudinal interview analysis to gain deeper insights. This process enables researchers to make sense of intricate data accumulated over time. Rather than relying solely on manual methods, integrating AI can enhance the analysis by identifying patterns and trends that might otherwise remain hidden.
To successfully implement AI in longitudinal interview analysis, consider a few key steps. First, you must collect and prepare your data, ensuring its quality and relevance for AI techniques. Next, choose the appropriate AI models tailored to your specific needs. Training and testing these models is crucial to ensure accuracy in interpretation. Finally, youโll analyze AI outputs, focusing on trends and ethical considerations that arise from your findings. By following these steps, you can transform raw interview data into actionable insights that inform strategic decisions.
- Features and functionalities
Effective Longitudinal Interview Analysis hinges on the features and functionalities provided by specialized AI tools. These tools are designed to process vast amounts of interview data efficiently while offering intuitive visualization options. Understanding these functionalities can greatly enhance the depth of your analysis.
One key feature is the ability to segment data based on predefined criteria. This allows users to filter insights by specific demographics or characteristics, such as industry or role. Another essential functionality is the visualization tools, like empathy maps and journey maps, that help represent data visually. These tools make it easier to identify trends, pain points, and opportunities for improvement in the user experience.
Additionally, features that allow users to formulate and save personalized queries further streamline the analysis process. By enabling repeated access to specific insights, these functionalities ensure that critical information is easily retrievable, helping researchers focus on their core objectives. Ultimately, these advanced tools empower researchers to conduct thorough longitudinal interview analyses with actionable insights.
Other AI Tools
When exploring Other AI Tools for longitudinal interview analysis, several robust options can enhance analytical capabilities. NVivo is a prominent tool designed for qualitative data analysis, allowing researchers to code text, audio, and visual data efficiently. Its sophisticated tools assist in identifying themes and patterns from interviews, making it invaluable for longitudinal studies.
Dedoose excels in managing mixed-methods data, allowing users to analyze quantitative and qualitative elements in one platform. Atlas.ti supports complex data sets, offering visualization features that simplify the analysis of longitudinal interviews. MAXQDA is another versatile tool that combines text analysis with qualitative insights, ideal for iterative data examination. Lastly, QDAMiner provides advanced text mining capabilities, enabling researchers to delve deeply into interview responses. These tools collectively facilitate a more effective approach to longitudinal interview analysis, providing comprehensive insights that inform decision-making.
- NVivo
NVivo provides a robust framework for conducting longitudinal interview analysis, enabling researchers to categorize and interpret data efficiently. This software is particularly useful for managing large volumes of qualitative data, allowing teams to focus on identifying trends, patterns, and shifts over time. By utilizing NVivo's advanced features, users can seamlessly integrate AI-driven processes, enhancing their analytical capabilities.
To maximize the benefits of NVivo in longitudinal interview analysis, consider the following steps:
Data Organization: Properly coding and organizing your interview transcripts is crucial. Use NVivoโs nodes and classifications to categorize themes and participant profiles effectively.
Querying Data: Utilize NVivo's query features to explore relationships and trends within the data. This aids in pinpointing significant changes throughout the interview timeline.
Visualizations: Make use of NVivoโs visualization tools. Creating models, charts, and maps helps to present findings coherently, making it easier to convey insights to stakeholders.
Incorporating NVivo into your longitudinal interview analysis workflow not only streamlines your process but also enhances the quality of the insights you derive from your data.
- Dedoose
Dedoose serves as a powerful tool for Longitudinal Interview Analysis, offering a user-friendly interface designed to streamline the process. It allows researchers to easily upload and manage their interview transcripts, making it simpler to extract insights over time. Users can quickly organize and categorize data, enhancing the clarity and coherence of their findings.
One of the standout features of this tool is its ability to analyze data both individually and collectively. Researchers can generate visualizations and reports, making it easier to identify trends and themes within the longitudinal data. Additionally, the platform supports bulk uploading, allowing users to manage large datasets with ease. By prioritizing data privacy and compliance, it provides a secure environment for sensitive information. This integration of technology not only saves time but also facilitates more organized and impactful research outcomes.
- Atlas.ti
Atlas.ti is a powerful tool for analyzing qualitative data, particularly in the context of Longitudinal Interview Analysis. It offers a versatile platform for researchers to manage and analyze extensive interview transcripts efficiently. With its user-friendly interface, researchers can easily code responses, identify themes, and visualize data structures. This functionality is particularly critical when handling long-term data that chronicles changes over time.
Moreover, Atlas.ti supports both manual and automated coding processes. This flexibility aids researchers in sifting through large volumes of data to identify significant trends and patterns. Its advanced features, such as text retrieval and network visualization, enhance the overall analysis by enabling researchers to connect ideas and insights from various interviews. Consequently, using Atlas.ti can significantly streamline the process of conducting Longitudinal Interview Analysis, ensuring that valuable insights are not overlooked in extensive data sets.
- MAXQDA
MAXQDA is a powerful tool designed to aid researchers in analyzing qualitative data, particularly valuable for Longitudinal Interview Analysis. Its user-friendly interface facilitates the organization and coding of interview transcripts, allowing researchers to uncover deeper insights over time. By streamlining the process of categorizing data, researchers can focus their efforts on interpreting the findings rather than getting bogged down in initial data review.
This software offers several features, including robust coding options and analytical tools that enhance the understanding of trends within repeated interviews. Moreover, MAXQDA's ability to integrate multimedia elements adds richness to the data analysis process, making it easier to draw connections and understand evolving narratives. For those engaged in Longitudinal Interview Analysis, employing MAXQDA can substantially reduce the time spent on preliminary assessments and significantly improve the depth of the analysis conducted.
- QDAMiner
QDAMiner serves as a potent ally in the realm of Longitudinal Interview Analysis, providing advanced functionalities to streamline qualitative research. This tool excels in managing extensive datasets generated from interviews, making it easier for analysts to derive meaningful insights. With its intuitive interface, users can quickly navigate through numerous transcripts, allowing for efficient coding, categorization, and thematic analysis.
A few key attributes of QDAMiner enhance its effectiveness in longitudinal analysis. First, it features a robust coding system that supports both manual and automated processes, helping researchers identify emerging themes over time. Second, its data visualization tools facilitate the exploration of trends, providing a clearer picture of participant narratives across different time points. Lastly, QDAMiner supports collaboration, enabling teams to work together seamlessly in analyzing data. Overall, this tool significantly reduces the workload and enhances the quality of insights drawn from longitudinal interviews.
Conclusion: The Future of Longitudinal Interview Analysis with AI
The future of longitudinal interview analysis is being transformed by advancements in artificial intelligence. As organizations increasingly seek timely insights from qualitative data, AI can provide the efficiency and accuracy necessary for thorough analysis. This integration reduces bias, enhances consistency, and accelerates the reporting process, ultimately allowing researchers to focus on extracting meaningful narratives from their findings.
Moreover, as AI technologies evolve, they are expected to improve in addressing the nuances of human conversation, capturing subtleties that traditional methods often miss. This progress will enable deeper understanding of participant experiences over time, solidifying longitudinal interview analysis as an essential tool for sophisticated data-driven decisions.