How to Analyze Focus Group Discussion with AI Tools
-
Chris Nwankwo
- 10 min read
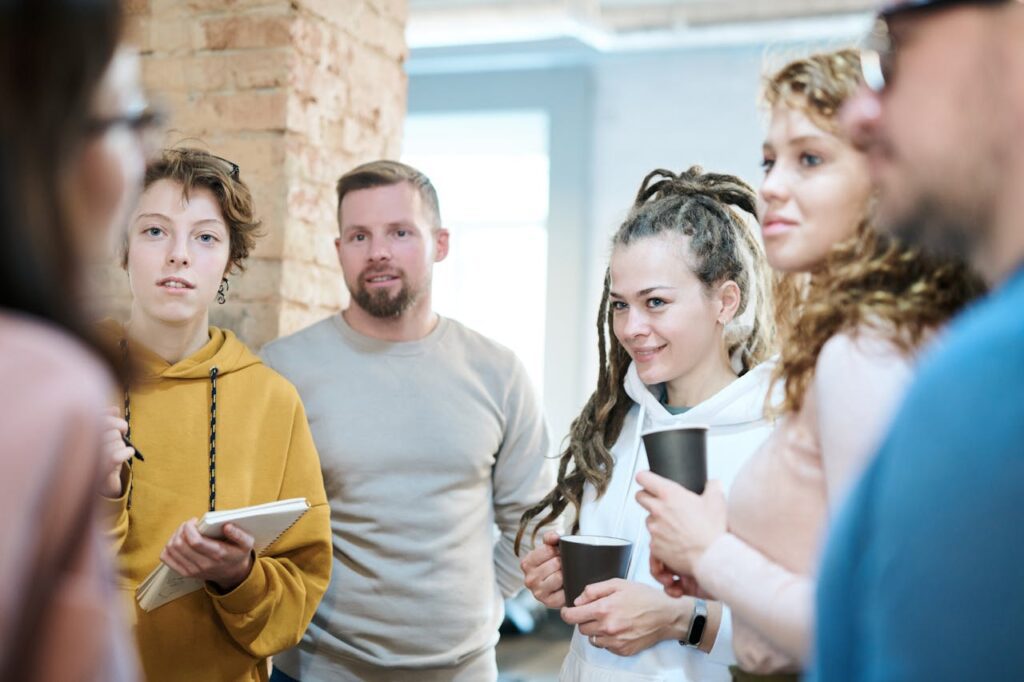
Focus group discussions (FGDs) are a powerful way to gather qualitative insights from a group of participants. However, analyzing focus group data manually can be time-consuming and prone to errors. That’s where AI-powered analysis tools come in—automating transcription, identifying key themes, and generating actionable insights in seconds.
With the rise of AI in research, analyzing focus group discussions is easier and more efficient than ever before. This article delves into the comprehensive process of FGDs and explores the potential of AI tools used to analyze focus group discussion recordings like Insight7.
By the end of this guide, you’ll be able to use AI-powered tools to streamline qualitative research and generate faster, more reliable insights.
What is Focus Group Discussion (FGD) Analysis?
FGD analysis refers to examining recorded group discussions to extract key insights, opinions, and behavioral patterns. Researchers analyze verbal responses, emotions, and interactions to identify trends and themes.
How AI Enhances Focus Group Analysis
AI tools eliminate human bias and inefficiency by:
- Automating transcription to convert audio discussions into text.
- Extracting key themes & patterns using NLP (Natural Language Processing).
- Summarizing large datasets quickly for easy reporting.
- Generating sentiment analysis to understand participant emotions.
- Visualizing insights through interactive dashboards.
These AI-powered capabilities make focus group research faster, more accurate, and scalable.
The Focus Group Discussion Process
Preparation Phase
- Defining Objectives: Clearly delineate the research goals to direct discussions effectively toward desired outcomes.
- Participant Selection: Identify and recruit participants representing the target demographic or possessing relevant expertise to ensure diverse perspectives.
- Moderator Training: Equip moderators with the necessary skills to facilitate unbiased discussions and maintain focus throughout the session.
Designing the Discussion Guide
- Structured Questioning: Craft a discussion guide comprising open-ended questions and prompts meticulously designed to explore specific aspects of the research topic.
- Ensuring Relevance: Ensure that questions are pertinent to the research objectives, fostering meaningful dialogue and eliciting valuable insights from participants.
Conducting the Focus Group Discussion
- Establishing Environment: Create a conducive setting conducive to open discourse, free from distractions, and conducive to participant engagement.
- Introduction and Rapport Building: To cultivate a comfortable atmosphere, kickstart the session with an introduction to the topic, setting ground rules, and fostering rapport among participants.
- Facilitating Dialogue: Encourage active participation and free-flowing discussions among participants while steering the conversation towards the predefined objectives.
- Probing for Depth: Judiciously employ probing techniques to delve deeper into responses, unraveling underlying motivations and nuances within participants’ perspectives.
- Recording Insights: Record the session, with participant consent, using audio or video methods to capture insights accurately for subsequent analysis.
Analysis Phase
- Transcription: Transcribe the recorded discussions verbatim, capturing nuances, emotions, and expressions to preserve the richness of participant input.
- Thematic Coding: Analyze transcripts meticulously to identify recurring themes, patterns, and divergent viewpoints, facilitating structured data organization.
- Interpretation and Synthesis: Interpret findings in the context of research objectives, synthesizing diverse perspectives to derive meaningful conclusions and actionable insights.
Reporting Findings
- Comprehensive Documentation: Compile a detailed report encapsulating key findings, supported by illustrative quotes and participant anecdotes to add depth and credibility.
- Visual Representation: Enhance understanding and retention by supplementing the report with visual aids such as charts, graphs, and diagrams to elucidate trends and insights effectively.
Analyze & Evaluate Calls. At Scale.
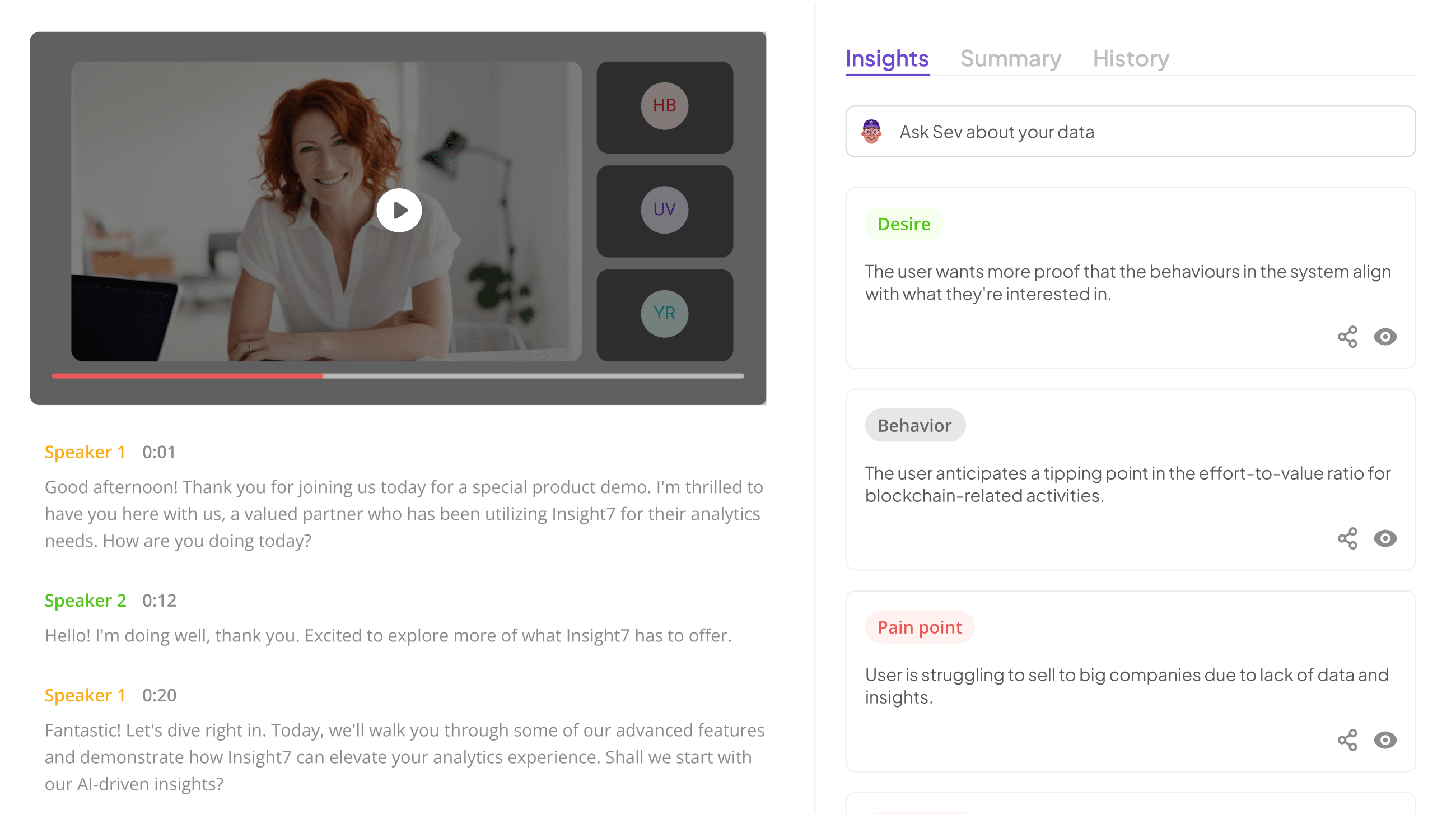
Integration of AI Tools in FGDs
Streamline Participant Recruitment
- AI-Driven Targeting: Leverage AI algorithms to sift through vast datasets and identify potential participants matching specific demographic criteria, expediting recruitment.
- Automated Screening: Implement Natural Language Processing (NLP) algorithms to screen participant responses efficiently, ensuring alignment with research prerequisites.
Optimize Discussion Guide Development
- Sentiment Analysis: Harness AI-powered sentiment analysis to parse through extensive textual data, gauging prevalent sentiments and identifying pertinent discussion topics.
- Predictive Analytics: Employ machine learning algorithms to anticipate potential discussion avenues based on past FGDs or related research, enriching the discussion guide formulation process.
Improve Moderator Support
- AI-Powered Chatbots: Integrate AI-driven chatbots equipped with NLP capabilities to assist moderators in real-time, suggesting follow-up questions and probing techniques to foster comprehensive discussions.
- Emotional Analysis: Employ real-time sentiment analysis tools to gauge participants’ emotional states during discussions. This will enable moderators to adapt their approach accordingly and maintain engagement.
Facilitate Data Analysis
- Automated Transcription: Deploy AI-powered transcription tools to automatically convert audio recordings into text format accurately and expeditiously, saving time and resources.
- NLP-Driven Thematic Analysis: Leverage NLP algorithms to streamline thematic coding processes, identifying and categorizing recurring themes within transcripts swiftly and systematically.
Generate Actionable Insights
- Advanced Analytics: Harness AI-driven analytics platforms to aggregate data from multiple FGDs, uncovering overarching trends and extracting deeper insights beyond human capacity.
- Pattern Recognition: Employ machine learning algorithms to discern subtle correlations and patterns within data, illuminating nuanced insights and effectively informing strategic decision-making processes.
AI Tools for Focus Group Discussion Analysis
There are several AI tools available that can assist with analyzing focus group discussions. These tools can help transcribe audio recordings, identify key themes and insights, and provide valuable data analysis. Here are some popular AI-powered tools for focus group analysis:
1. Insight7
Insight7 is a powerful platform for analyzing qualitative data designed to streamline the focus group analysis process. This tool automatically transcribes audio and video recordings in bulk, allowing researchers to handle large datasets efficiently. Its intuitive coding features enable users to identify key themes and patterns within transcripts, providing a deep understanding of participant responses. The platform also generates detailed visualizations and reports, making it easy to explore insights and present findings clearly and compellingly.
- Automated Transcription: Effortlessly convert bulk audio and video recordings into accurate text transcripts, saving time and reducing manual effort.
- Thematic Coding: Use advanced coding tools to identify themes, patterns, and trends within your qualitative data, ensuring comprehensive analysis.
- Visualizations & Reporting: Generate visually engaging reports and dashboards to illustrate insights, making it easier to share results with stakeholders.
Extract insights from interviews, calls, surveys and reviews for insights in minutes
2. Dedoose
Dedoose is a web-based application specifically designed to analyze qualitative and mixed-methods data. It caters to researchers looking to manage and analyze focus group transcripts and multimedia files seamlessly. Dedoose offers automated coding suggestions by leveraging machine learning, allowing users to focus on extracting meaningful insights rather than manual coding.
- Web-Based Platform: Access Dedoose from anywhere, allowing flexible collaboration and team data management.
- Multimedia Integration: Upload and analyze transcripts, audio, and video files to capture a complete picture of your focus group data.
- Machine Learning Coding Suggestions: Enhance efficiency with AI-driven coding suggestions that streamline the analysis process.
Pros:
- User-friendly interface and easy accessibility.
- Strong support for mixed-methods research.
- Real-time collaboration capabilities.
Cons:
- Some advanced features may require additional learning.
- Subscription costs might be high for smaller research teams.
3. QDA Miner + WordStat
QDA Miner and WordStat offer a comprehensive solution for qualitative and quantitative data analysis. The tool provides robust qualitative coding and analysis tools, while WordStat integrates seamlessly to perform quantitative content analysis. Together, they identify key topics, concepts, and sentiments from focus group transcripts, offering a multidimensional view of the data.
- Qualitative Coding with QDA Miner: Utilize powerful coding tools to categorize and analyze qualitative data effectively.
- Integrated Sentiment Analysis: Automatically detect sentiments, emotions, and opinions expressed in the data.
- Quantitative Analysis with WordStat: Conduct quantitative content analysis to extract statistical insights and patterns.
Pros:
- Combines qualitative and quantitative analysis for richer insights.
- Detailed sentiment analysis capabilities.
- Suitable for large-scale data analysis.
Cons:
- It may require training for full utilization.
- Complex interface for beginners.
4. ATLAS.ti
ATLAS.ti is a leading software for qualitative data analysis, providing researchers with comprehensive tools for coding, annotating, and visualizing focus group data. It supports a wide range of media formats, including texts, videos, and audio, ensuring versatility in research. ATLAS. ti’s AI tools enhance analysis with auto-coding, sentiment analysis, and named entity recognition, making it a powerful choice for complex qualitative research projects.
- Versatile Data Support: Analyze texts, videos, and audio files for a holistic approach to data analysis.
- AI-Powered Analysis: To uncover deeper insights, use advanced AI tools for auto-coding, sentiment analysis, and entity recognition.
- Visualization & Collaboration: Create visual representations of data and collaborate with team members in real time.
Pros:
- Strong AI capabilities for in-depth analysis.
- Wide range of data format support.
- Collaborative features enhance team research.
Cons:
- Steeper learning curve for new users.
- Higher costs may be a barrier for small teams.
5. Qualtrics Text IQ
Qualtrics Text IQ is a text analytics tool integrated within the Qualtrics survey platform. It offers a sophisticated analysis of open-ended responses. It automatically identifies themes, sentiments, and emotions within focus group transcripts, allowing researchers to gain insights quickly and accurately. This integration enables seamless analysis of survey data alongside qualitative insights, providing a comprehensive view of consumer feedback.
- Integrated Text Analytics: Analyze open-ended survey responses and focus group transcripts in one platform for unified insights.
- Automatic Theme and Sentiment Detection: Leverage AI to surface key themes, sentiments, and emotions from qualitative data.
- Customizable Reports: Generate tailored reports to highlight findings and trends in your data.
Pros:
💬 Questions about How to Analyze Focus Group Discussion with AI Tools?
Our team typically responds within minutes
- Seamless integration with Qualtrics survey data.
- Advanced sentiment and emotion analysis.
- User-friendly interface with customizable reports.
Cons:
- Primarily designed for use within the Qualtrics ecosystem.
- It may require additional setup for standalone usage
The right tool depends on your specific needs in transcription, qualitative coding, quantitative analysis, and visualizations and whether you prefer an end-to-end integrated solution or best-of-breed point solutions.
Benefits of AI-Driven Focus Group Discussion Integration
- Efficiency Augmentation: AI tools streamline labor-intensive tasks, such as participant recruitment, transcription, and thematic analysis, significantly reducing time and resource expenditures.
- Precision Enhancement: By minimizing human errors and biases, AI algorithms ensure consistency and accuracy in data processing, bolstering the reliability of research findings.
- Scalability Enrichment: AI-powered platforms facilitate seamless scalability, enabling researchers to conduct multiple FGDs concurrently and easily handle large volumes of data.
- Insight Expansion: AI tools unearth latent patterns and insights within data, enriching the depth and breadth of research findings beyond conventional qualitative analyses.
Challenges and Considerations
- Ethical Implications: Ethical concerns regarding data privacy and consent necessitate stringent adherence to ethical guidelines and data protection regulations throughout the research process.
- Algorithmic Bias Mitigation: Vigilant monitoring and validation are imperative to mitigate inherent biases within AI algorithms, ensuring impartiality and accuracy in research outcomes.
- Integration Complexity: The seamless integration of AI tools mandates technical expertise and resources, posing challenges for organizations with limited technological capabilities.
- Human Oversight Imperative: While AI augments efficiency, human oversight remains indispensable to uphold the validity and reliability of research findings, particularly in nuanced qualitative analyses.
Common Pitfalls to Avoid When Analyzing Focus Group Discussions with AI
While AI-powered analysis can significantly improve speed and accuracy, researchers should be aware of common pitfalls to ensure credible and unbiased results.
1: Relying on AI Transcription Without Review
Why it’s a problem:
AI transcription tools are not 100% accurate and may misinterpret technical terms, accents, or multiple speakers.
Incorrect transcriptions can lead to misinterpretation of key insights.
How to avoid it:
- Always review and correct AI-generated transcripts before analysis.
- Use human proofreaders or AI grammar checkers like Grammarly or Hemingway.
- Train AI models with custom vocabulary if working with industry-specific terms.
2: Overlooking Context in Sentiment Analysis
Why it’s a problem:
AI tools may misinterpret sarcasm, humor, or cultural nuances, leading to inaccurate sentiment analysis. Words like “great” or “good” might be positive in one context but negative in another (e.g., “That’s great… but I wouldn’t use it”).
How to avoid it:
- Always cross-check AI sentiment reports with human review.
- Use context-aware AI models like MonkeyLearn or IBM Watson NLP.
- Look for pattern-based sentiment rather than individual words
3: Ignoring Data Bias and Skewed Results
Why it’s a problem:
AI models learn from past data, which may introduce bias in analysis.
Skewed datasets may produce misleading themes and insights.
How to avoid it:
- Ensure diverse and balanced participant representation in focus groups.
- Use AI tools that allow custom rule-based learning to refine data classification.
- Cross-check AI-generated insights with traditional manual analysis to validate findings.
4: Choosing the Wrong AI Tool for Analysis
Why it’s a problem:
Not all AI tools are optimized for qualitative research.
Some platforms are better for transcription, while others specialize in data visualization or NLP.
How to avoid it:
- Choose AI tools based on your research needs (e.g., transcription, theme extraction, or report generation).
- Compare AI accuracy, scalability, and integration capabilities before committing to a tool.
- Test multiple tools such as Insight7, NVivo, and Crayon to find the best fit.
5: Failing to Store & Organize Data Properly
Why it’s a problem:
Poor data storage leads to loss of insights and difficulty in future referencing. Without proper documentation, teams working on the same project may face collaboration issues.
How to avoid it:
- Store qualitative data in secure cloud-based platforms like Google Drive, Notion, or Airtable.
- Use tagging systems to categorize key insights and themes.
- Set up data backup protocols to prevent accidental loss.
Conclusion
In conclusion, integrating AI tools holds immense promise in augmenting the efficacy and scope of Focus Group DiscussionS across diverse domains. By synergizing human expertise with AI capabilities, researchers can unlock unprecedented insights, fostering innovation and driving informed decision-making.
However, diligent attention to ethical, bias, and integration considerations is paramount to effectively harness the full potential of AI-driven FGDs.
As technological advancements evolve, the fusion of human ingenuity with AI innovation heralds a transformative era in qualitative research methodologies, poised to revolutionize insights generation and strategic planning paradigms.
Analyze & Evaluate Calls. At Scale.
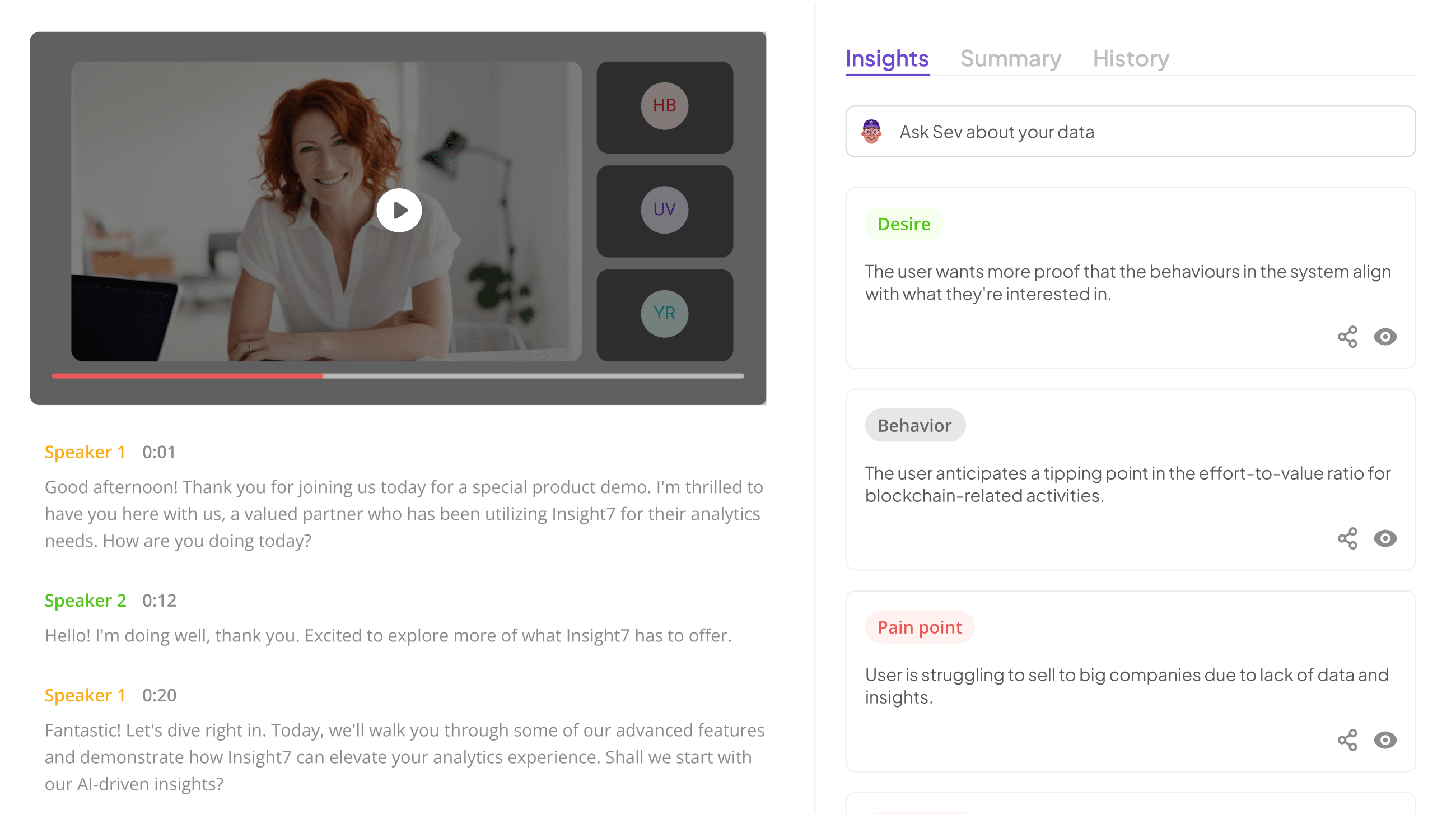
💬 Questions about How to Analyze Focus Group Discussion with AI Tools?
Our team typically responds within minutes