Research Gap Analysis is essential for driving innovation and improving outcomes in various fields. As professionals sift through countless interview transcripts, the challenge of identifying gaps in knowledge and insights becomes increasingly complex. Traditional manual methods often lead to bias and inconsistencies, resulting in delayed reports and missed opportunities.
Artificial Intelligence serves as a powerful solution to streamline this process. By employing AI technologies, researchers can extract valuable themes and insights from transcripts more efficiently. This not only enhances the accuracy of analysis but also allows teams to focus on deeper strategic questions, ultimately advancing their understanding of critical issues in their respective domains.
Analyze qualitative data. At Scale.
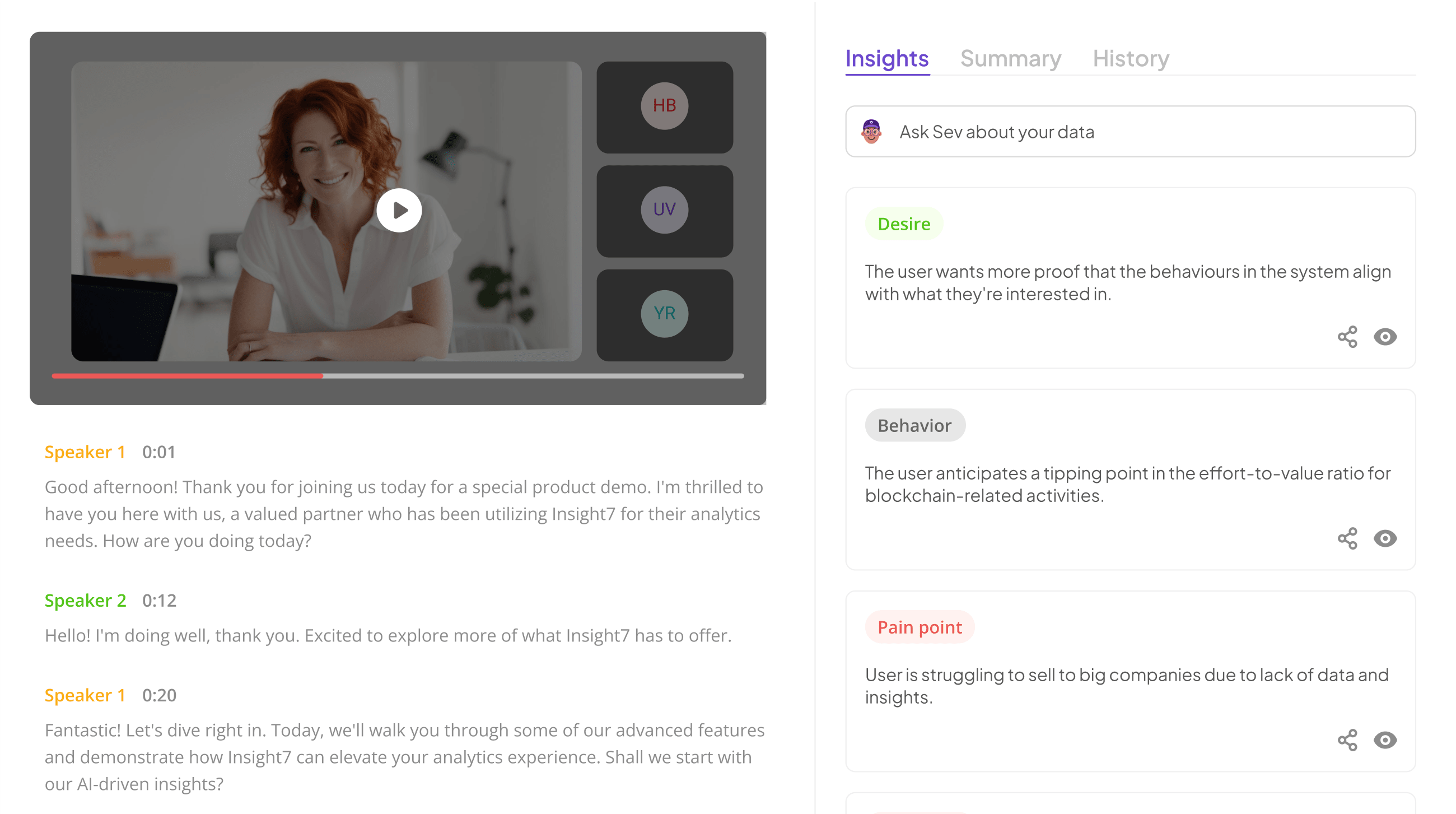
Understanding the Role of AI in Research Gap Analysis
AI plays a pivotal role in research gap analysis by facilitating the systematic evaluation of qualitative data gleaned from interview transcripts. This technology enhances the efficiency of identifying underexplored areas in various subjects. By automating the analysis, researchers can save valuable time and reduce the risk of human bias in interpreting qualitative data.
Moreover, AI employs advanced techniques such as natural language processing, enabling it to discern patterns and themes within complex transcripts. With the use of machine learning algorithms, AI uncovers insights that traditional methods might overlook. This capability not only accelerates the research process but also contributes to a more accurate understanding of gaps in existing literature. As researchers increasingly adopt these tools, they can expect a more streamlined process, leading to richer and more actionable insights in their field.
How AI Processes Interview Transcripts for Research Gap Analysis
AI effectively processes interview transcripts, enabling efficient Research Gap Analysis. Initially, textual data extraction occurs whereby AI sifts through large volumes of text, identifying key phrases and core themes. This automated extraction makes it feasible to manage extensive data without human error or bias. It dramatically reduces the time required for qualitative analysis, providing more reliable insights for researchers.
Subsequently, AI utilizes natural language processing (NLP) techniques to understand context and sentiment within the conversations captured in transcripts. By analyzing tone, intent, and intricacies of dialogue, AI identifies subtle patterns that may indicate research gaps. This improved analysis delivers actionable insights faster, allowing researchers to adapt and focus their studies effectively. As a result, organizations can make quicker, data-driven decisions, ultimately enhancing the quality and relevance of their research efforts.
- Textual Data Extraction
Textual data extraction is a vital step in conducting Research Gap Analysis. It involves transforming raw interview transcripts into structured information suitable for further analysis. By carefully processing the text, AI identifies key themes, sentiments, and findings that researchers can explore more deeply. This enables researchers to recognize aspects of their field that may require further exploration, revealing potential gaps in existing literature.
To effectively extract textual data, several methods can be employed. First, transcription normalization ensures consistent terminology, enhancing the quality of the analysis. Second, sentiment analysis categorizes the emotions expressed in the data, making it easier to interpret interviews' emotional tone. Lastly, topic modeling identifies prevalent themes within transcripts, providing researchers a clear vision of areas that warrant additional investigation. Through these techniques, researchers can utilize AI to pinpoint significant insights that lead to a more targeted and informed Research Gap Analysis.
- Natural Language Processing Techniques
Natural language processing (NLP) techniques are instrumental in enhancing research gap analysis. By transforming raw interview transcripts into actionable insights, NLP bridges the gap between qualitative data and analytical rigor. These techniques automate the extraction of relevant information, allowing researchers to focus on identifying uncharted areas in their field.
One essential NLP technique is text mining, which involves parsing transcripts to extract key themes and sentiments. Another is named entity recognition, where the system identifies and categorizes key entities mentioned during interviews. Additionally, sentiment analysis helps assess the emotional tone of the responses, providing deeper context for research gaps. By employing these NLP approaches, researchers can not only streamline their analysis but also gain clarity on significant trends, ultimately leading to more effective decision-making. This integration of technology into qualitative research enhances the speed and accuracy of finding research gaps within interview data, ensuring a comprehensive analysis process.
Identifying Patterns and Insights in Research Gap Analysis
Identifying patterns and insights in research gap analysis involves systematically examining interview transcripts to uncover overlooked areas of study. Utilizing AI technologies, researchers can extract essential themes and sentiments embedded within qualitative data. This process begins with coding the data, clustering insights into meaningful buckets, and evaluating sentiments to provide a comprehensive overview of participant feedback.
By tagging the data according to specific goals, such as improving member experience or enhancing collaboration, AI-driven tools reveal critical insights relevant to your research questions. The identification process helps highlight participants' concerns and suggestions, allowing researchers to address gaps effectively. These insights not only inform future research directions but also ensure that interventions are tailored to meet the actual needs of the subjects involved. Ultimately, incorporating these patterns into your research gap analysis strengthens the foundation for actionable findings and innovative advancements.
- Machine Learning Algorithms
Machine learning algorithms play a crucial role in the process of research gap analysis. By applying these algorithms to interview transcripts, one can uncover underlying patterns and insights that are often overlooked. These algorithms utilize historical data to predict trends and identify areas needing further investigation. Their sophisticated techniques help researchers quickly categorize vast amounts of qualitative data, enabling a more efficient analysis process.
Moreover, using machine learning for research gap analysis ensures an objective evaluation of the data. It minimizes human bias by focusing on quantitative patterns rather than subjective interpretations. Techniques such as clustering and classification allow for the synthesis of complex information into actionable insights. As a result, researchers can pinpoint gaps in existing knowledge or areas where further study is needed, driving innovation and informed decision-making. Ultimately, these algorithms facilitate a more robust and systematic approach to identifying research gaps within the valuable data gathered from interview transcripts.
- Semantic Analysis
Semantic analysis plays a crucial role in research gap analysis by enabling the extraction of meaningful insights from interview transcripts. Through semantic analysis, AI systems can discern the context, sentiments, and themes within the language used by participants. This capability goes beyond mere keyword detection, allowing researchers to identify patterns that may indicate gaps in existing literature or areas needing further exploration.
To effectively harness semantic analysis, consider the following approaches:
Thematic Coding: Organize responses into themes based on common topics or sentiments. This allows for quick identification of recurring challenges or desires expressed by interviewees.
Sentiment Evaluation: Assess the emotional tone of responses to uncover underlying frustrations or areas of enthusiasm, which can highlight potential research gaps.
Contextual Relationships: Examine how specific themes relate to one another, providing insights into complex issues that may not be immediately evident.
By implementing these strategies, researchers can use semantic analysis to facilitate a comprehensive research gap analysis, ultimately guiding future investigations toward highly relevant topics.
Extract insights from interviews, calls, surveys and reviews for insights in minutes
Tools for Effective AI-Driven Research Gap Analysis
Effective AI-driven research gap analysis relies on a suite of specialized tools designed to streamline the process of identifying gaps in research. By analyzing interview transcripts through advanced machine learning algorithms and natural language processing, these tools unveil insights that would likely remain hidden in manual analysis. Utilizing AI provides a faster, more reliable way to extract key themes and trends from qualitative data.
Some noteworthy tools for research gap analysis include Atlas.ti, NVivo, MAXQDA, and Dedoose. Each of these platforms offers unique features that facilitate comprehensive analysis, such as coding capabilities, visual mapping, and collaborative features. For example, Atlas.ti helps researchers visually organize data, while NVivo excels at managing large datasets. By integrating these tools into your research workflow, you can enhance the efficiency and accuracy of identifying research gaps, ultimately driving more impactful conclusions and recommendations.
Using insight7 for Comprehensive Research Gap Analysis
Using insight7 for Comprehensive Research Gap Analysis allows researchers to thoroughly analyze interview transcripts and pinpoint areas needing further exploration. The platform's advanced features streamline the collection and synthesis of qualitative data, transforming hours of analysis into actionable insights more efficiently.
The implementation process involves uploading interview transcripts, where AI tools swiftly extract key themes. Next, natural language processing techniques review text to identify significant patterns that may highlight unaddressed research questions. By employing these tools, users can focus on developing strategies to fill these knowledge gaps, promoting a more informed and strategic approach to research. By following these steps, you can effectively utilize insight7 to enhance your research gap analysis and drive your projects forward with clarity and purpose.
- Key Features and Benefits
Utilizing AI for research gap analysis offers transformative benefits, particularly when examining interview transcripts. One of the key features is the ability to extract textual data efficiently. By processing rich qualitative information, AI identifies the primary themes and pain points expressed by interviewees, leading to deeper insights. This helps researchers pinpoint areas that lack sufficient exploration, thereby highlighting potential avenues for further investigation.
Another significant benefit is the implementation of natural language processing techniques. These algorithms analyze language use and sentiment, enabling a detailed understanding of the context surrounding each insight. This contextual awareness ensures that findings are relevant and actionable. With AI-driven tools, researchers can streamline reporting, quickly generating structured summaries and recommendations based on the data. This enhances overall productivity and facilitates more informed decision-making in future research initiatives.
- Implementation Process
The Implementation Process for utilizing AI in Research Gap Analysis begins with the careful selection of relevant interview transcripts. This involves collecting qualitative data that effectively reflects the area of interest. Subsequently, the AI system analyzes these documents through advanced algorithms, identifying patterns and themes that highlight existing research gaps.
Once the data is processed, users can select key insights to incorporate into reports. These reports not only summarize findings but also provide recommendations based on AI-generated analysis. It’s crucial to ensure that the AI system respects the context, meaning, and intent of the discussions held in the interviews. By following these steps, researchers can achieve high accuracy and reliability in their insights, streamlining their reporting processes and enhancing the overall quality of their research.
Other Noteworthy Tools for Research Gap Analysis
When conducting research gap analysis, several noteworthy tools can enhance your understanding of data from interview transcripts. Each of these tools offers unique features designed to streamline the process of identifying critical insights. For instance, Atlas.ti excels in visually mapping data, enabling researchers to see relationships and themes in a structured way. NVivo, on the other hand, provides sophisticated coding capabilities, allowing you to tag transcript segments to specific research questions.
MAXQDA supports mixed methods research, making it ideal for those combining qualitative and quantitative data. Additionally, Dedoose is particularly user-friendly for collaborative projects, facilitating teamwork in coding and analysis. Each of these tools plays a crucial role in research gap analysis by helping researchers categorize data, evaluate themes, and trace quotes back to original sources, ultimately producing comprehensive insights.
- Atlas.ti
Atlas.ti serves as a powerful tool for facilitating research gap analysis by transforming qualitative data, such as interview transcripts, into manageable insights. Its graphical user interface allows researchers to code and categorize qualitative data seamlessly, aiding in the identification of themes and patterns. This process enhances the ability to pinpoint areas where existing research may be lacking or where further inquiry is necessary.
Moreover, Atlas.ti supports advanced text analysis capabilities, including word frequency and co-occurrence analysis. By utilizing these features, researchers can uncover hidden trends and shed light on significant gaps in their current understanding. This approach ensures a comprehensive evaluation of qualitative data, ultimately bolstering the research process and leading to more impactful conclusions. Engaging with this tool assists researchers in garnering well-informed insights that drive the success of their research initiatives.
- NVivo
NVivo serves as an essential tool in the process of research gap analysis, particularly when examining interview transcripts. It facilitates a structured and systematic approach for researchers, allowing them to organize qualitative data efficiently. By utilizing this software, researchers can easily code, categorize, and visualize themes within their transcripts, ultimately leading to clearer identification of gaps in the current body of knowledge.
Moreover, NVivo's powerful analytics capabilities enable researchers to conduct a more in-depth exploration of their data. The tool employs features like word frequency queries and sentiment analysis, which can unveil hidden insights and trends. As a result, researchers can make informed decisions about what areas require further investigation. This blending of advanced technology with qualitative analysis transforms the way research gaps are identified and addressed, enriching the overall research landscape.
- MAXQDA
MAXQDA is a powerful tool for conducting research gap analysis, especially when working with interview transcripts. Researchers can utilize MAXQDA’s capabilities to organize and analyze vast amounts of qualitative data effortlessly. The software facilitates coding of transcripts, enabling users to highlight themes, patterns, and sentiments within the data set, which can reveal critical insights often overlooked.
By employing MAXQDA, researchers can systematically compare different interviews and identify recurring gaps in knowledge and understanding. This structured approach allows for a more in-depth analysis of the interview data, leading to well-informed conclusions. Furthermore, the platform supports various export options, which can streamline the presentation of findings. Ultimately, leveraging MAXQDA for research gap analysis not only enhances the clarity of insights but also paves the way for actionable strategies in addressing those gaps effectively.
- Dedoose
Dedoose is a powerful tool that facilitates the analysis of qualitative data, especially when it comes to research gap analysis. By streamlining the process of organizing and interpreting interview transcripts, researchers can uncover critical insights more efficiently. This platform supports users in uploading their data from multiple sources, allowing easy access to essential themes and patterns within the narratives.
One significant advantage of Dedoose is its ability to provide native transcription services, ensuring high accuracy and support for numerous languages. This means that researchers can focus on extracting potential research gaps rather than getting bogged down by data organization. Additionally, the tool allows for collaborative workspaces where teams can analyze data collectively, thus enhancing the overall research process. By harnessing Dedoose, researchers can not only save time but also significantly improve the clarity and relevance of their findings in the context of research gap analysis.
Conclusion: Transforming Research Gap Analysis with AI
AI is revolutionizing Research Gap Analysis by streamlining how insights are derived from interview transcripts. This transformation enhances accuracy and reduces biases that often emerge in manual analysis. As researchers integrate AI tools, they can quickly identify trends and patterns that may otherwise go unnoticed, ensuring a more comprehensive understanding of their subject matter.
Adopting AI not only accelerates the research process but also improves the overall quality of insights. Researchers can now generate faster reports and maintain consistency across teams, ultimately leading to more actionable insights. Embracing these advancements will illuminate research gaps and promote more effective decision-making in the future.