In today's competitive landscape, understanding customer feedback is more crucial than ever for businesses aiming to thrive. Feedback clustering techniques offer a systematic approach to unraveling insights hidden within extensive interview transcripts. By grouping similar responses, organizations can discern patterns that reveal customer sentiments, preferences, and pain points.
These techniques involve both manual and automated processes, allowing for flexibility depending on the volume of data. The primary aim is to transform raw feedback into actionable insights, thereby enabling businesses to enhance their offerings and foster customer loyalty. As we delve deeper into these techniques, we'll explore their significance and how they can effectively shape strategic decision-making.
Analyze & Evaluate Calls. At Scale.
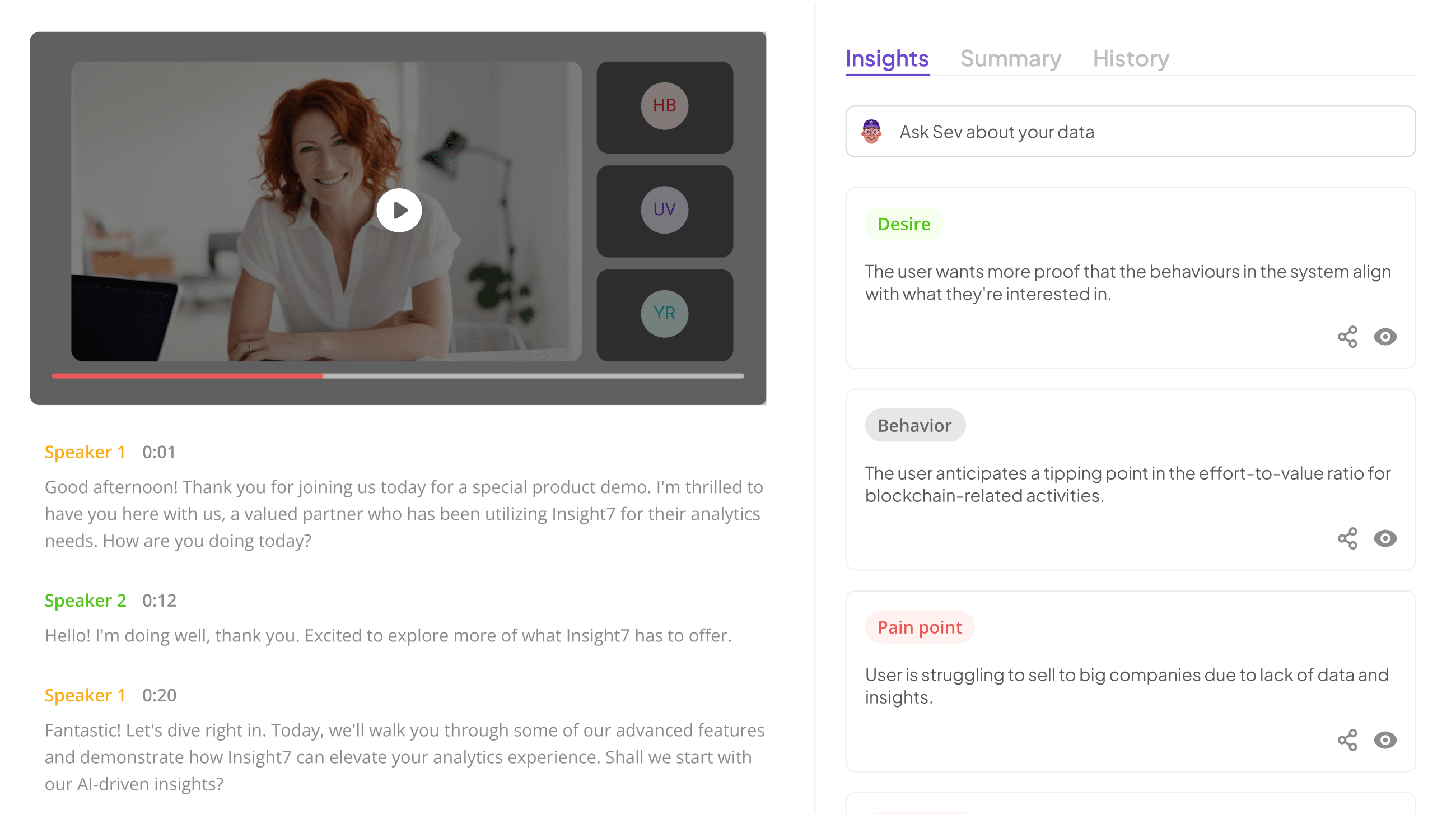
Understanding Feedback Clustering Techniques
Understanding feedback clustering techniques is crucial for transforming raw customer insights into actionable strategies. Feedback clustering techniques allow you to group similar comments, sentiments, and themes present in interview transcripts. This approach helps identify underlying patterns in customer feedback, leading to a deeper understanding of their experiences and concerns.
Several common techniques for clustering customer feedback include manual coding, thematic analysis, and the use of automated algorithms. Manual coding involves a detailed examination of transcripts to identify recurring themes, while thematic analysis provides a structured process for categorizing feedback. Automated techniques, on the other hand, leverage technology to quickly analyze large volumes of data, increasing efficiency. By using a combination of these techniques, businesses can gain comprehensive insights into their customers' thoughts and improve service quality substantially. Adopting these methods promotes a more data-driven approach to understanding and responding to customer needs.
Importance of Clustering in Feedback Analysis
Clustering plays a crucial role in feedback analysis by organizing vast amounts of data into meaningful groups. This method allows analysts to identify common themes and sentiments that emerge from customer interviews, making it easier to understand overarching issues and opportunities. By categorizing feedback into clusters, organizations can quickly pinpoint areas that require improvement or innovation, enhancing their responsiveness to customer needs.
Employing feedback clustering techniques leads to a more efficient analysis process. It streamlines the identification of patterns in customer experiences, enabling businesses to develop targeted strategies for improvement. By focusing on particular themes, organizations can prioritize actions based on the aggregated insights. Ultimately, clustering transforms raw feedback into actionable intelligence, fostering a deeper connection with customers and guiding future decision-making processes.
- Explanation of clustering and its benefits.
Clustering customer feedback is an essential technique that enhances the analysis of interview transcripts. Feedback clustering techniques help identify common themes and sentiments within the data, allowing for a clearer understanding of customer needs. By grouping similar pieces of feedback, organizations can efficiently prioritize areas for improvement and recognize patterns that may otherwise go unnoticed. This organized approach enables decision-makers to focus their efforts where they matter most.
The benefits of clustering extend beyond mere organization. It allows for better sentiment analysis, helping to extract valuable insights that can inform strategy. For instance, identifying frequent topics or concerns can lead to more targeted solutions and improved customer experiences. Additionally, by mapping insights to specific goals, clusters can drive focused discussions and decision-making that aligns with organizational objectives. Ultimately, clustering transforms raw feedback into actionable insights, streamlining the path to enhanced customer satisfaction and loyalty.
- How clustering helps in identifying common themes and sentiments.
Clustering plays a pivotal role in distilling customer feedback into meaningful insights, making it easier to identify common themes and sentiments. Through effective Feedback Clustering Techniques, organizations can group similar responses together, allowing for a streamlined analysis. This approach enables the discovery of overarching sentiments that may not be evident when examining individual comments. By aggregating feedback, it helps highlight prevalent issues or desires, leading to more informed decision-making.
Moreover, clustering not only saves time but also enhances the accuracy of the interpretation. By coding the feedback into specific themes, businesses can pinpoint areas requiring attention, such as product improvements or service enhancements. This organized representation of data facilitates a clearer understanding of customer perspectives, ultimately shaping a more strategic response to their needs. With clustering, organizations can harness the voice of the customer, turning insights into actionable strategies.
Types of Feedback Clustering Techniques
Feedback clustering techniques play a vital role in extracting valuable insights from customer interview transcripts. Each technique serves a unique purpose, allowing analysts to better organize and understand qualitative feedback. Manual techniques, such as thematic analysis, involve human coding of data to identify patterns and sentiments. This approach ensures context is maintained and nuances in feedback are captured effectively.
On the other hand, automated techniques utilize software tools to quickly analyze large volumes of data. These tools can identify common themes, extracting insights with remarkable speed. Some popular software options include analysis kits and mind-mapping tools that support structured frameworks for categorizing feedback. By using these techniques collectively, businesses can derive comprehensive insights to enhance customer experience and operational efficiency. An understanding of these methods enables organizations to tailor their strategies based on real customer needs and sentiments.
- Overview of popular clustering methods.
Clustering techniques play a crucial role in analyzing customer feedback from interview transcripts. These methods categorize diverse responses into meaningful groups, enabling organizations to identify patterns and sentiments effectively. Understanding popular clustering methods is essential for interpreting feedback accurately and allows teams to focus on key insights that drive improvements.
Common techniques include hierarchical clustering, k-means clustering, and DBSCAN. Hierarchical clustering builds a tree of clusters based on similarity, while k-means organizes data into a predefined number of groups. DBSCAN, on the other hand, identifies clusters of varying shapes and sizes based on density. Each method has its advantages, depending on the nature of the feedback and the detailed analysis required. Select the right technique according to your specific needs to ensure that the insights drawn from customer feedback are both actionable and relevant.
- Discussion on manual vs. automated techniques.
In the realm of clustering customer feedback, understanding the differences between manual and automated techniques is essential. Manual analysis often entails a labor-intensive process where researchers comb through transcripts, extracting insights one detail at a time. This method may lead to biases and inconsistencies, particularly when multiple researchers interpret data differently. The time-consuming nature of manual analysis can also delay reporting, potentially impacting decision-making.
On the other hand, automated techniques, particularly those utilizing AI, can significantly expedite this process. Automated systems can swiftly analyze large volumes of transcripts and identify common themes and sentiments, reducing the risk of human error. Techniques like natural language processing enable researchers to cluster feedback efficiently, uncovering insights that would take much longer to surface manually. By embracing these feedback clustering techniques, organizations can gain timely insights while maintaining a consistent analytical approach.
Step-by-Step Process of Clustering Customer Feedback
The process of clustering customer feedback begins with a structured approach that ensures clarity and facilitates insightful analysis. First, gather and prepare the interview transcripts, making sure to clean the data by removing irrelevant information and correcting errors. This preparation is crucial, as well-organized data yields more accurate clustering results. Utilizing tools to streamline this phase can enhance efficiency and reduce manual effort.
Next, employ feedback clustering techniques effectively. Begin by segmenting the data into manageable chunks, allowing for easier analysis. Applying clustering algorithms follows, which organizes customer sentiments into distinct categories. After implementing the algorithms, review each cluster closely to extract themes and patterns that reflect common customer experiences. Lastly, document findings and draw meaningful interpretations from the clusters to inform future strategies. Following this step-by-step process will lead to enhanced understanding and actionable insights for your organization.
Preparing Interview Transcripts
Preparing interview transcripts is a crucial step in the feedback clustering process. First, ensure accurate transcription of your interviews, as this forms the foundation of your analysis. Utilizing reliable transcription tools significantly enhances accuracy, with some platforms offering 95% accuracy across multiple languages. After transcription, review the text carefully to clean up any errors or unclear segments. This is essential as even minor inaccuracies can lead to misinterpretations during analysis.
Next, organize your transcripts efficiently. By categorizing them into relevant projects, you can streamline the feedback clustering techniques. This method allows for easy identification of key themes and insights that arise from the interviews. Employing tools that can handle bulk uploads makes it quicker to prepare interviews for analysis. With all transcripts cleaned and organized, you're well-equipped to apply various clustering techniques to uncover valuable customer insights.
- Transcription process and cleaning of data for analysis.
The transcription process is a crucial step in preparing customer feedback for clustering analysis. It involves converting spoken interviews into written text, which enables easier identification of key insights. Using reliable transcription tools can enhance accuracy and efficiency. Most modern platforms offer automated transcription services that can process numerous interviews simultaneously, helping to streamline the workflow. Accurate transcripts serve as a foundation for further analysis, ensuring that all valuable feedback is captured.
After transcription, cleaning the data is essential to maintain quality and clarity. This process may include removing filler words, correcting misheard phrases, and standardizing terminology. Such cleaning not only refines the data but also aids in implementing effective Feedback Clustering Techniques. Clean data ensures that themes and sentiments can be accurately derived, informing strategic decision-making for enhancing customer experiences.
- Tools for efficient transcript preparation.
Efficient transcript preparation is crucial for effectively clustering customer feedback from interview data. Utilizing appropriate tools can greatly enhance the workflow, making it easier to extract valuable insights. Begin by selecting a reliable transcription service that offers high accuracy and supports bulk uploads, which saves time and reduces manual errors. A robust transcription tool will not only transcribe audio but also clean the data, ensuring clarity and consistency in the final output.
Moreover, implementing software that facilitates collaboration can streamline the analysis process. Such tools often provide features to organize and categorize responses, making it easier to identify common themes. As you prepare your transcripts, integrate techniques that allow for easy tagging and annotation. This will help in efficiently organizing the feedback and enable quick access during the clustering phase. By using the right tools, you enhance the effectiveness of feedback clustering techniques, driving better insights and informed decisions from your customer feedback data.
Extract insights from interviews, calls, surveys and reviews for insights in minutes
Tools and Techniques for Clustering Feedback
Understanding the various tools and techniques available for clustering feedback is crucial for making sense of customer insights. Various software solutions provide users with advanced algorithms to sort and categorize written feedback effectively. These include programs like insight7, MonkeyLearn, Text2Data, Lexalytics, and Structuralize, each offering unique features to assist in the clustering process. These tools enhance the ability to analyze qualitative data by automatically identifying and grouping similar sentiments or themes.
Feedback clustering techniques can help streamline the analysis process in several ways. For instance, insight7 allows users to evaluate feedback across multiple files, summarizing responses efficiently. Similarly, MonkeyLearn's machine-learning capabilities can analyze text data and highlight trends, enhancing user comprehension. By employing these advanced tools, businesses can highlight key themes and sentiments from their customer feedback, ultimately leading to more informed decision-making. Hence, utilizing appropriate tools can significantly transform raw data into actionable insights.
- Overview of top tools for feedback clustering:
When exploring tools for feedback clustering, it's essential to understand how each can enhance the analysis of customer insights. Feedback clustering techniques can often simplify and streamline processes by categorizing large volumes of qualitative data. Utilizing the right tools can help automate tedious tasks, leaving analysts to focus on interpreting the results.
Key tools in the market, such as Insight7, MonkeyLearn, and Lexalytics, employ advanced algorithms to aggregate feedback efficiently. Insight7, for instance, excels in thematic analysis, making it easier to group insights into coherent categories. Similarly, MonkeyLearn leverages machine learning to refine sentiment analysis, thereby improving accuracy in feedback interpretation. Lexalytics offers powerful text analytics features that enhance the understanding of customer emotions and sentiments. Each of these tools not only saves time but also provides deeper insights, enabling businesses to make informed decisions based on customer feedback.
Overall, selecting the right tools for feedback clustering can significantly impact your analysis process and the value derived from customer insights.
- insight7: Comprehensive tool for analyzing and clustering feedback.
insight7 is an advanced tool designed specifically for analyzing and clustering feedback effectively. Utilizing powerful algorithms, it simplifies the complex process of gathering customer insights, allowing teams to swiftly identify key themes. This efficiency is crucial for organizations looking to improve their products or services based on user feedback. By organizing data into discernible clusters, insight7 aids in pinpointing trends and sentiments that might otherwise go unnoticed.
Through the application of various feedback clustering techniques, users can tag and group responses into categories designed for specific goals. This functionality not only enhances analysis but also saves significant time, allowing organizations to focus on actionable insights rather than manual sifting through data. Whether utilized for sentiment analysis or identifying specific user desires, insight7 provides a comprehensive solution for turning complex feedback into meaningful information, streamlining the pathway to informed decisions and improved user experiences.
- Other tools include:
Clustering customer feedback effectively requires the right tools to simplify the analysis process. Several tools can help you automate and enhance your clustering efforts, ensuring you extract the most meaningful insights from interview transcripts. Among these, MonkeyLearn, Text2Data, Lexalytics, and Structuralize stand out for their unique capabilities in processing varied feedback types.
MonkeyLearn is known for its user-friendly interface and powerful machine learning algorithms, allowing users to categorize and analyze text seamlessly. Text2Data excels in interpreting unstructured data and can assist in identifying trends from large datasets. Lexalytics provides advanced sentiment analysis and thematic extraction, making it ideal for organizations seeking deeper insights. Meanwhile, Structuralize offers templates tailored to specific analysis needs, which can save significant time during the clustering process. Leveraging these feedback clustering techniques can radically improve the efficiency and accuracy of your analysis.
- **MonkeyLearn**
Understanding effective feedback clustering techniques is crucial for turning customer insights into actionable strategies. One powerful tool in this space offers advanced capabilities to transform unstructured feedback into meaningful data. This tool utilizes machine learning models to analyze text, enabling users to categorize and group comments by sentiment and thematic similarities.
Users can leverage this platform to automate the tedious processes of manual analysis. By inputting interview transcripts directly, you can quickly gain insights into recurring themes, helping identify customer pain points and areas for improvement. The platform's intuitive interface allows for easy integration with existing workflows, making it accessible even for those with limited technical expertise. By automatically clustering feedback, businesses can save time and resources while obtaining valuable insights that drive decisions.
Utilizing effective feedback clustering techniques enables professionals to focus on actionable data and improve overall customer satisfaction.
- **Text2Data**
In the realm of customer feedback analysis, the transition from raw text to actionable insights is crucial. Text2Data embodies the essence of this transformation, converting unstructured data from interview transcripts into structured, insightful information. By utilizing effective feedback clustering techniques, you can reveal underlying patterns that help in understanding customer sentiments and priorities. This process not only simplifies data management but also enhances decision-making.
The backbone of Text2Data lies in its ability to identify key themes and sentiments through various clustering approaches. Whether leveraging automated algorithms or using manual coding, the goal remains the same: to draw substantial insights from customer feedback. As you dive into the clustering process, think about how themes such as employee engagement or customer satisfaction can emerge from the data. This focus allows businesses to target their strategies effectively, fostering a deeper relationship with their clientele.
- **Lexalytics**
Customer feedback is invaluable for understanding user needs, and effective feedback clustering techniques can enhance this understanding significantly. Lexalytics is a tool particularly suited for this purpose, allowing users to analyze and derive insights from feedback data. It streamlines the process of ingesting data across various channels, ensuring transcriptions are accurate and quickly accessible for analysis.
Utilizing Lexalytics, teams can categorize feedback into themes and sentiments efficiently. First, the transcription of interviews can occur directly within the platform, eliminating the need for external tools. Once transcribed, the data allows for visualization and extraction of meaningful patterns. Users can generate reports and engage with the data through various analytical lenses, making it easier to identify actionable insights. Overall, Lexalytics serves as a powerful ally in applying feedback clustering techniques, paving the way for informed decision-making and improved customer relationships.
- **Structuralize**
To effectively structuralize customer feedback through clustering, it’s essential to create a framework that organizes insights systematically. This process begins by identifying the main themes that emerge from interview transcripts. By employing feedback clustering techniques, teams can sift through large volumes of data to pinpoint patterns related to customer experiences. Each theme should represent a summarized essence of common sentiments reflected in the feedback, which aids in clarifying areas of concern or praise.
Following this initial identification, structuring the insights is vital. One could categorize findings into various domains, such as customer satisfaction, product functionality, or service quality. Once segmented, synthesizing these insights into coherent reports allows for easier interpretation and actionable recommendations. Ultimately, structuralizing feedback not only improves clarity but also empowers businesses to make informed decisions based on real customer voices, thus enhancing overall customer-centric strategies.
Executing Clustering
To execute effective clustering of customer feedback, it is essential to segment data into manageable units. This initial step facilitates a focused analysis that can bring clarity to the diverse themes emerging from interviews. By dissecting large datasets, you allow for a more nuanced understanding of customer sentiments and experiences.
Next, applying feedback clustering techniques, such as K-means or hierarchical clustering, can assist in grouping related insights. These algorithms sift through customer feedback and reveal patterns that may not be immediately obvious. Once clusters are formed, analyzing and interpreting them is vital to identify actionable insights. This involves examining the characteristics and sentiments associated with each cluster, providing a more comprehensive picture of customer experiences and expectations. By following these steps, organizations can better harness the value of customer feedback and drive informed decision-making.
- Step 1: Segmentation of data into smaller parts.
When embarking on the journey of clustering customer feedback, the first crucial step is the segmentation of data into smaller parts. This process allows researchers to break down extensive interview transcripts into manageable sections, making analysis far more effective. By identifying distinct themes or categories within the feedback, you can streamline your approach to interpretation. This segmentation helps in organizing insights around specific variables, such as the industry or demographic of interview subjects, thereby enhancing the clarity of your findings.
To achieve effective data segmentation, follow these essential practices: first, categorize responses by shared characteristics, such as sentiment or topic frequency. Second, prioritize insights based on variables that are meaningful for your objectives. Lastly, utilize tools that allow for visualization of this segmented data, such as empathy maps or journey maps. These techniques facilitate a deeper understanding of customer needs and pain points, ultimately aiding in effective feedback clustering.
- Step 2: Application of clustering algorithms.
In the application of clustering algorithms, the process begins by segmenting the data into manageable portions. This step is crucial for developing meaningful clusters that reflect distinct themes within customer feedback. Various clustering algorithms can be employed, such as k-means, hierarchical clustering, and DBSCAN. Each algorithm functions differently, making it essential to choose one based on the specific characteristics of the data.
Once the clusters form, qualitative analysis can reveal insights about customer sentiments and preferences. This stage enables teams to identify prevalent themes, allowing for tailored responses to customer feedback. Moreover, performing a sentiment analysis can complement the clustering efforts, enhancing understanding of how customers feel about their experiences. Implementing effective Feedback Clustering Techniques not only streamlines data interpretation but also empowers businesses to make informed decisions that reflect customer needs.
- Step 3: Analyzing and interpreting the clusters.
In Step 3: Analyzing and interpreting the clusters, you will dive into the heart of feedback clustering techniques. This involves reviewing the clusters created during the previous steps to extract meaningful insights. Your main objective is to identify recurring themes and sentiments encapsulated in customer feedback. By doing this, you can pinpoint specific areas for improvement or innovation based on customer input.
Firstly, take a closer look at each cluster and assess the themes that emerge. For instance, if one cluster highlights a desire for better collaboration tools, it serves as a clear insight into customer needs. Additionally, pairing your findings with sentiment analysis helps you understand the emotional context behind the feedback. This process allows you to validate your hypotheses and refine your strategies. Remember, the effectiveness of this analysis phase is crucial for translating customer feedback into actionable business strategies.
Conclusion on Feedback Clustering Techniques
Feedback Clustering Techniques play a crucial role in transforming customer interview transcripts into actionable insights. By applying these techniques, organizations can identify recurring themes and sentiments that form the backbone of customer experiences. This process not only enhances the understanding of customer needs but also informs strategic decisions that resonate with target audiences.
In conclusion, effectively clustering feedback helps streamline data analysis, making it easier to extract insights at scale. Adopting these techniques can significantly improve how companies respond to customer demands and expectations. Embracing feedback clustering ultimately leads to better service delivery and enhanced customer satisfaction.
- Summarizing the importance and effectiveness of clustering in improving customer understanding.
Understanding the importance and effectiveness of clustering is pivotal in enhancing customer insights. By organizing customer feedback into meaningful categories, businesses can better comprehend common themes and sentiments. This process allows for a clearer understanding of customer needs, preferences, and challenges. Effective clustering leads to actionable insights, empowering organizations to tailor their strategies and offerings.
When employing feedback clustering techniques, it’s essential to accurately analyze and interpret the clustered data. First, the segmentation of data helps in identifying distinct clusters that reflect specific customer experiences. Next, applying clustering algorithms allows for the automatic grouping of feedback based on similarities. Finally, detailed analysis of these clusters can reveal valuable patterns that guide decision-making. Ultimately, effective clustering not only streamlines the feedback analysis process but also enriches customer understanding, driving improved business outcomes.
- Encouragement to adopt feedback clustering for better strategic decisions.
Adopting feedback clustering techniques is essential for making informed strategic decisions. By systematically organizing and categorizing customer feedback, businesses can uncover meaningful insights which might otherwise remain hidden. This process allows for the identification of recurring themes or challenges faced by customers, enabling teams to address pressing issues more efficiently.
One effective approach is to utilize different clustering methods that fit your analysis needs. Techniques vary from manual coding, where themes are identified through personal interpretation, to automated systems that analyze large datasets swiftly. Implementing a robust feedback clustering system not only enhances understanding of customer sentiments but can also improve overall customer experience. Thus, embracing these methodologies will pave the way for informed, customer-centric strategies that align with business objectives. The future of strategic decision-making lies in the precision and clarity that feedback clustering brings to the table.