In the rapidly evolving realm of training and development, extracting insights from real-world interactions is paramount. By employing LLM-tagged call breakdowns, organizations can effectively mine valuable training moments embedded within conversations. This approach allows teams to uncover underlying patterns, enabling data-driven decisions that enhance training effectiveness.
Training insights extraction becomes a systematic process when using LLM-tagged calls. The technology tags relevant keywords and themes from discussions, making it easier to identify areas that require attention. Furthermore, this method streamlines the evaluation process, ensuring that critical training opportunities are not overlooked. Ultimately, a thoughtful approach to insights extraction empowers organizations to foster continuous improvement and meet the dynamic needs of their workforce.
Analyze qualitative data. At Scale.
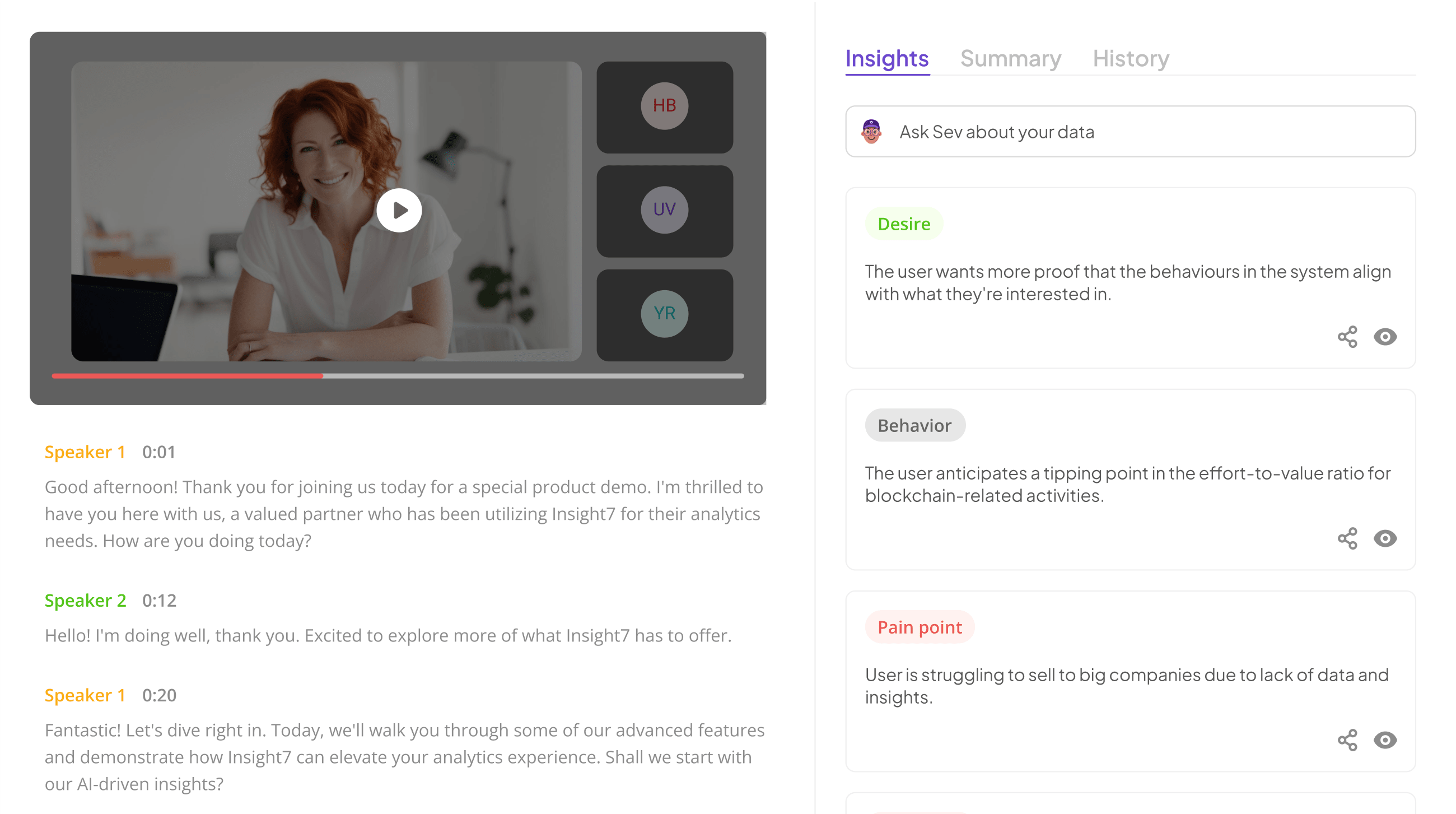
Understanding LLM-Tagged Call Breakdowns for Training Insights Extraction
Understanding LLM-tagged call breakdowns is crucial in extracting targeted training insights. By utilizing Large Language Models (LLMs), organizations can analyze call data with enhanced precision. This process allows identifying key scenarios where training moments arise, which is essential for effectively addressing workforce development needs. A well-structured breakdown highlights the nuances of conversations, enabling trainers to discern specific skills and knowledge gaps within teams.
To effectively leverage these tag breakdowns, consider the following steps:
Identify Core Issues: Start by assessing the primary challenges that arise during calls. This can guide trainers in pinpointing training requirements.
Analyze Learning Needs: Review call transcripts to determine what skills or knowledge gaps exist, allowing for tailored training programs.
Choose Delivery Methods: Decide how best to deliver the necessary training interventions, whether through workshops, e-learning, or hands-on training.
Evaluate Impact: After training implementation, monitor performance to ensure that the intended outcomes are achieved.
By following these steps, organizations can enhance their training strategies and ultimately foster a more skilled workforce.
What Are LLM-Tagged Call Breakdowns?
LLM-tagged call breakdowns refer to the systematic process of analyzing call recordings that have been marked or annotated using large language models (LLMs). These models help to identify key themes, sentiments, and specific moments within conversations, offering valuable insights for training and development. Understanding call context is crucial, as it allows organizations to extract meaningful training insights from real-time interactions.
The importance of tagging calls with LLMs cannot be overstated. By utilizing advanced techniques in natural language processing, organizations can automatically identify training moments and performance gaps, streamlining the process of employee skill enhancement. This automated tagging improves accuracy and enhances the relevance of insights gathered, ensuring that training programs are effectively tailored to meet specific needs. In a rapidly evolving work environment, insights derived from LLM-tagged call breakdowns are essential for developing a competent workforce ready to tackle future challenges.
- Explanation of LLM and Call Breakdowns
LLM, or Large Language Model, refers to advanced AI systems designed to process and analyze vast amounts of text data. Call breakdowns involve segmenting recorded conversations into manageable components for analysis. Together, LLMs and call breakdowns create a potent method for extracting training insights by offering deep analysis of dialogue during training sessions or customer interactions.
Understanding how these technologies work is crucial for effective training insights extraction. By tagging specific segments of conversations with relevant markers, organizations can identify important training moments that may have otherwise gone unnoticed. This breakdown method enhances the training process, making it possible to pinpoint areas where improvements are needed. It not only aids in evaluating training effectiveness but also aligns future training methodologies with actual performance needs, ensuring that employees are better equipped for their roles.
- Importance of tagging calls using LLMs
Tagging calls using Large Language Models (LLMs) is essential for extracting vital training insights. This process enhances our ability to analyze conversations and identify key moments that can significantly impact training outcomes. By meticulously tagging calls, we can concentrate on specific areas that require immediate improvement, leading to more focused training strategies.
The importance of this tagging encompasses several aspects. First, it enhances the precision of the insights derived from call recordings. When calls are accurately tagged, it becomes easier to pinpoint recurring issues or themes that might otherwise go unnoticed. Secondly, the efficiency gained from this practice allows teams to shift their focus from general training to targeted interventions, ensuring that each training moment is impactful and relevant. Ultimately, the use of LLMs for tagging calls is a strategic approach to maximizing the effectiveness of training programs.
Benefits of Using LLMs for Training Insights Extraction
Utilizing LLMs for Training Insights Extraction can drastically enhance the efficiency and effectiveness of training programs. By implementing LLMs, organizations can achieve improved accuracy and relevance in identifying critical training moments. These advanced language models help sift through vast data from call breakdowns, ensuring that the extracted insights are not only pertinent but also tailored to specific audience needs.
Moreover, LLMs streamline the training evaluation process, allowing for quicker adaptations to training materials. This efficiency means trainers can respond to the unique challenges faced by new and existing employees alike. As insights are drawn directly from real-call data, training can be made more practical and contextually relevant. Overall, incorporating LLMs in training insights extraction ensures a more informed and adaptable approach to employee development, fostering a culture of continuous learning and improvement.
- Improved accuracy and relevance
Using LLM-tagged call breakdowns significantly enhances the accuracy and relevance of training insights extraction. By meticulously analyzing conversations, we can pinpoint exact moments where training interventions are necessary. This not only improves the precision of our evaluations but also ensures that the training provided aligns closely with actual user needs and performance gaps.
Identifying these critical training moments requires a structured approach to call analysis. We can establish specific criteria that highlight when discrepancies or learning opportunities arise. By leveraging machine learning algorithms, we can filter out noise and focus on the moments that will create the most impact. This strategic application of LLMs fosters a training environment that is not merely reactive but proactive and adaptive, ultimately driving better outcomes and increasing the overall effectiveness of the training provided.
- Efficiency in training evaluation
Efficiency in training evaluation revolves around the ability to quickly and accurately assess training moments that can enhance skills and knowledge. By utilizing LLM-tagged call breakdowns, organizations can optimize the evaluation process, pinpointing precise learning opportunities. This approach integrates advanced analytics and streamlines dialogue review, ensuring that training sessions yield meaningful insights effectively.
One of the key advantages of this methodology is its capability to transform vast amounts of call data into structured training insights extraction. By applying user-friendly tools like Insight7, organizations can identify trends and patterns within dialogue more efficiently, enabling targeted training interventions. This results in a more responsive learning environment that adapts to the needs of employees, ensuring that training is relevant and resonates with their duties. By focusing on consistent evaluation methods, organizations can foster a culture of continuous improvement that ultimately enhances cohesive team performance.
Extract insights from interviews, calls, surveys and reviews for insights in minutes
Steps for Identifying Training Moments through LLM Insights
Identifying training moments through LLM insights requires a structured approach. First, gather relevant call data tagged by Language Learning Models (LLMs). This tagging offers a clear pathway to analyzing interactions for patterns indicating potential training needs. Use tools like Insight7, CallRail, or Gong.io to streamline the tagging process and ensure comprehensive coverage of interactions.
Next, analyze the tagged calls for recurring themes or alarming trends. Engage in fact-finding interviews with key stakeholders across departments, allowing space for candid feedback. These conversations illuminate pain points and unexpected issues, fostering a clearer picture of necessary training. Itโs essential to document all findings meticulously, creating an audit trail that supports further training initiatives. This structured methodology not only enriches the training insights extraction process but also ensures that corrective action aligns with organizational goals. Ultimately, you can transform insights into impactful learning moments, creating a more effective workforce.
Step 1: Setting Up LLM-Tagged Call Analysis
Setting up LLM-tagged call analysis is a pivotal step toward enhancing your organization's training insights extraction. The focus lies in meticulously preparing your system to enable effective analysis of calls. Start by selecting the right tools, like Insight7 alongside other platforms, to facilitate the tagging process. These tools help streamline data organization and ensure that your calls are effectively categorized based on training needs.
Next, ensure all prerequisites are met before launching your LLMs. This typically includes defining the goals for your analysis and identifying the specific training moments you wish to target. A well-planned setup will make it easier to extract valuable insights from call breakdowns later.
By establishing a robust framework for LLM-tagged call analysis, you are laying the groundwork for identifying critical training gaps and optimizing employee performance. This foundational step will significantly enhance your ability to analyze and leverage training insights efficiently.
- Tools for tagging calls: insight7, CallRail, Gong.io
In the journey of extracting valuable training insights, employing effective tools for tagging calls becomes essential. Various tools can facilitate this process, enabling users to analyze conversations systematically. These platforms not only classify call content but also dissect it for patterns that indicate training moments. By applying natural language processing to recorded calls, organizations can identify areas of improvement and development.
While each tool offers unique capabilities, they share a common goal of enhancing call analysis. Users can leverage specialized features to categorize interactions and distill actionable insights from a repository of conversations. This allows for a targeted approach to training, ensuring that employees receive focused development in areas where they need it most. In essence, these tools serve as a bridge between raw conversation data and meaningful training insights extraction, empowering organizations to tailor their learning initiatives effectively.
- Prerequisites for launching LLMs
Launching LLMs for effective Training Insights Extraction requires careful planning and preparation. First, it is crucial to have a well-defined objective. Understanding what specific training moments need to be identified will streamline the entire process. Next, assembling the right team ensures that varied perspectives refine the insights created from LLM-Tagged Call Breakdowns. By engaging stakeholders, such as HR and managerial staff, you can deepen the accuracy of the insights being generated.
Data infrastructure is another essential prerequisite. Ensuring that the necessary technology is in place to handle large datasets and generate relevant outputs is vital. It's equally important to ensure that employees have the requisite skills for effective training. Incorporating employee feedback during the launch phase can illuminate potential hurdles, allowing for adjustments before the full implementation of LLMs. Prioritizing these prerequisites is key to leveraging effective strategies for Training Insights Extraction.
Step 2: Extracting Training Insights from Call Breakdowns
Extracting training insights from call breakdowns involves a rigorous analysis of recorded interactions to identify key moments that can enhance training frameworks. Initially, the focus is on reviewing specific call segments that demonstrate effective communication or customer engagement. By tagging these moments with relevant keywords and themes, organizations can systematically categorize instances that require attention or replication.
The next step is to extract meaningful patterns from these tagged call segments. Observing common themes across different interactions enables the identification of recurring training opportunities. It is essential to analyze the context behind each training moment to understand the underlying factors, such as employee performance, customer reactions, or regulatory compliance issues. Diligently capturing and synthesizing these insights will ultimately provide actionable recommendations, contributing to a more dynamic and responsive training strategy. Engaging with both successful and challenging interactions can offer a holistic view, allowing for continuous improvement in training effectiveness.
- Methods to identify critical training moments
To effectively identify critical training moments, organizations can employ various methods that center around comprehensive data analysis. One approach involves conducting interviews with key stakeholders, including HR representatives and operational team managers. These discussions enable the extraction of invaluable insights that reveal prevailing issues within the organization. This grassroots information forms the backbone of a robust training needs analysis, ensuring that training insights extraction remains aligned with organizational priorities.
Another vital method includes analyzing LLM-tagged call breakdowns to uncover recurring themes and trends within interactions. By documenting these findings systematically, organizations can pinpoint specific moments that necessitate training interventions. Furthermore, engaging with direct feedback from employees fosters an open dialogue that helps identify hidden pain points. This combination of qualitative and quantitative data can significantly enhance training programs and ensure they are both relevant and timely, ultimately driving continuous improvement within the organization.
- Utilizing patterns and trends for insights
Patterns and trends play a crucial role in training insights extraction, providing a framework to discern actionable information from LLM-tagged call data. By examining recurring themes and behaviors, organizations can identify specific training moments that require focus. This analytical approach enables the development of tailored training programs that enhance employee performance and engagement.
To effectively utilize patterns and trends, consider these key strategies. First, analyze the frequency of particular issues or questions that arise during calls. Understanding what challenges employees face can inform targeted training sessions. Second, observe behavioral patterns in successful interactions, allowing you to model best practices for others. Lastly, combine quantitative data with qualitative feedback to create a comprehensive overview of training needs, ensuring training initiatives resonate with team members effectively. By harnessing these insights, organizations can foster a culture of continuous improvement through well-informed training endeavors.
Conclusion: Harnessing LLM-Tagged Calls for Effective Training Insights Extraction
Harnessing LLM-tagged calls offers a powerful avenue for effective training insights extraction. By analyzing these calls, organizations can uncover essential training moments that significantly impact employee development. This approach not only identifies skill gaps but also ensures relevance, creating tailored training programs that resonate with employees.
Moreover, LLM-tagged calls streamline the evaluation and enhancement of training effectiveness. As insights are extracted, organizations can adapt their training strategies dynamically, fostering a culture of continuous learning. Ultimately, maximizing the potential of LLM-tagged calls transforms training into a more targeted and impactful experience.