Historical Transcript Benchmarking offers a strategic approach for enhancing AI model training, rooted in the analysis of historical data. By utilizing transcripts from past interactions, organizations can create a robust framework that informs AI systems about language, semantics, and user behaviors. This context-rich data enables models to learn nuanced patterns, improving their understanding and responsiveness.
Engaging with historical transcripts not only provides valuable insights but also drives innovation in AI applications. By creating benchmarks through these data sets, AI developers can evaluate the effectiveness of their models against well-defined standards. As a result, Historical Transcript Benchmarking emerges as an essential tool for ensuring accuracy, reliability, and increased user satisfaction in AI-driven solutions.
Analyze qualitative data. At Scale.
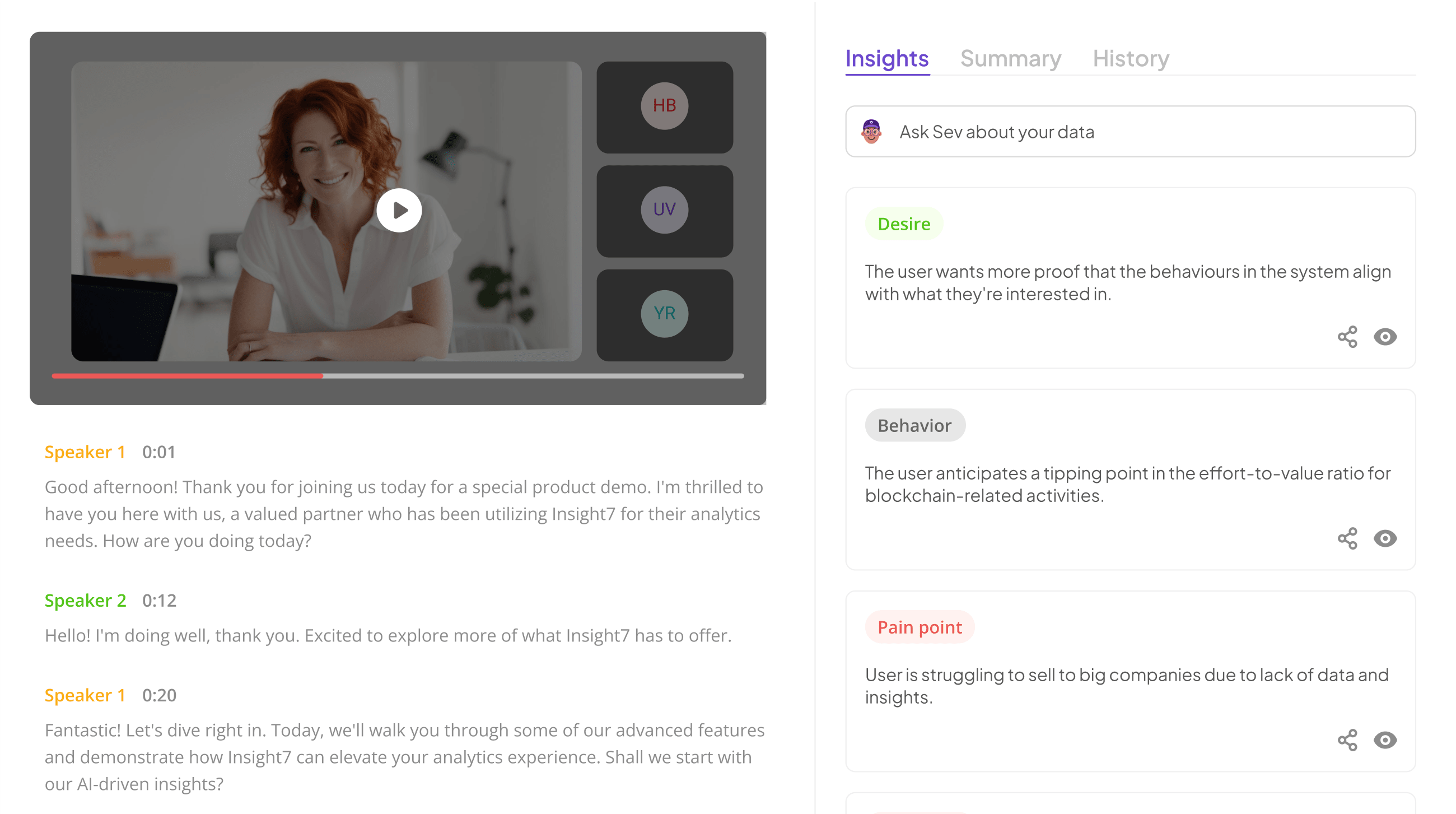
Historical Transcript Benchmarking: A Foundation for AI Model Training
Historical Transcript Benchmarking serves as a critical framework for training AI models effectively. By analyzing historical transcripts, we uncover valuable insights that contribute to the model's learning process. This benchmarking establishes a foundation that enhances model accuracy and context understanding. It enables AI systems to recognize patterns and trends within spoken data, allowing for more informed decision-making.
The methodology of Historical Transcript Benchmarking includes meticulous data collection and thoughtful project organization. By integrating various transcript sources, teams can synthesize comprehensive datasets, facilitating deeper analysis. This approach helps in identifying key customer sentiments and feedback, making it a vital component in refining AI capabilities. As we prioritize historical data, it becomes evident that such benchmarking not only optimizes AI training but also enhances the overall reliability of the models utilized in various applications.
Understanding the Importance of Historical Transcripts
Historical transcripts play a vital role in understanding and improving AI models. They serve as a rich repository of factual data and responses collected over time. This historical context provides invaluable insights into user behavior, language use, and trends, which are crucial for effective Historical Transcript Benchmarking. By utilizing these transcripts, researchers can better train and evaluate AI models, leading to enhanced performance and accuracy.
Moreover, historical transcripts empower AI systems to adapt to contextual changes in communication and cultural references. They ensure that AI tools do not operate in a vacuum but rather reflect real-world language patterns and sentiments. This adaptation is essential for creating user-centric AI applications that resonate with their target audiences. Thus, the importance of historical transcripts cannot be overstated, as they form the backbone of effective training and benchmarking for AI models.
Key Benefits of Using Historical Data for AI Benchmarking
Using historical data for AI benchmarking offers significant advantages that can greatly enhance the development process. Historical Transcript Benchmarking serves as a rich resource, allowing developers to build models grounded in real-world data and user interactions. One major benefit is the ability to evaluate AI performance over time, comparing current models against past benchmarks. This iterative process helps identify improvements and informs adjustments, fostering continuous learning for the AI system.
Additionally, utilizing historical transcripts enables the extraction of patterns and insights from previous data. By analyzing user sentiments and behaviors, developers can better understand their audience. This understanding not only shapes product refinement but also plays a crucial role in strategic decision-making. Consequently, the insights derived from historical data can guide future AI innovations, ensuring they meet user needs and expectations effectively. Embracing historical data thus empowers organizations to create AI models that are not only efficient but also deeply aligned with user experiences.
Tools for Historical Transcript Benchmarking and AI Training
To conduct effective Historical Transcript Benchmarking and AI Training, utilizing the right tools is essential. Various platforms provide sophisticated capabilities to analyze, process, and learn from historical transcripts. These tools can streamline the collection of data, enabling users to spot trends and patterns within substantial datasets. By simplifying the data entry process, they allow users to focus on meaningful insights rather than logistical challenges.
Among the top tools, several standout options emerge. Insight7 delivers advanced processing capabilities specifically tailored for historical transcripts. Google’s TensorFlow offers a robust framework for building, training, and fine-tuning AI models. IBM Watson provides powerful linguistic analysis tools that enhance understanding of textual data. OpenAI's GPT serves as a versatile language model, effective for diverse training applications. Finally, Amazon Comprehend excels in text analytics, further supporting AI training needs. Collectively, these tools significantly enhance the effectiveness of historical transcript benchmarking in refining AI models.
Extract insights from interviews, calls, surveys and reviews for insights in minutes
Top Tools for Effective Transcript Analysis
The effectiveness of historical transcript analysis hinges on the right tools. A variety of platforms can facilitate this process, making the task of processing and analyzing transcripts considerably easier. For example, advanced tools like insight7 allow users to bulk transcribe multiple audio files while extracting vital insights efficiently. This capability streamlines the entire workflow, enabling quicker access to actionable information.
Additionally, frameworks like Google's TensorFlow provide robust options for training AI models using transcribed data. Meanwhile, IBM Watson offers powerful linguistic analysis tools, enhancing understanding of the transcripts' nuanced meanings. As organizations increasingly prioritize historical transcript benchmarking, leveraging these tools can yield significant returns. High-quality analyses contribute directly to developing better AI models and refining training techniques essential for future applications.
- insight7: Advanced capabilities for historical transcript processing.
Processing historical transcripts effectively requires advanced capabilities tailored to extract meaningful insights efficiently. With the growing interest in Historical Transcript Benchmarking, the demand for sophisticated methods to analyze extensive datasets is also increasing. Technologies must not only transcribe but also understand context, nuances, and sentiments found within conversational data.
To harness the full potential of historical transcripts, key capabilities include bulk transcription, rapid analysis, and insights extraction. Additionally, the ability to filter data based on specific criteria enables users to focus on particular themes or pain points. This streamlined workflow enhances the ease of obtaining actionable insights from large volumes of transcripts, thereby improving the benchmark quality. With the right tools, businesses can maximize their understanding of customer interactions, ensuring that the knowledge gathered informs better decision-making and strategies moving forward.
- Googles TensorFlow: Robust framework for training AI models.
The framework is designed to facilitate the training of artificial intelligence models effectively and efficiently. With its user-friendly interface, a diverse range of tools, and extensive libraries, it empowers users to harness historical transcripts for benchmarking their AI projects. By analyzing conversations recorded in transcripts, this framework enables developers to extract valuable insights that can drive model improvements.
Using historical transcript benchmarking, practitioners can identify patterns, pain points, and emerging trends. This robust framework not only supports language processing tasks but also enables easy integration with other data sources. As a result, it facilitates comprehensive model training tailored to historical contexts, leading to enhanced AI performance. Ultimately, utilizing this framework can elevate the quality of AI-enabled applications, ensuring they generate more accurate and relevant outputs for various industries.
- IBM Watson: AI solutions with powerful linguistic analysis tools.
AI solutions with advanced linguistic analysis tools facilitate the effective use of historical transcripts for benchmarking AI models. These tools excel at understanding nuances in language, offering insights that go beyond basic data processing. By analyzing historical transcripts, AI systems can learn to distinguish between different speakers, contexts, and conversational structures, improving their overall accuracy over time.
Effective historical transcript benchmarking involves several key aspects. First, the extraction and categorization of relevant linguistic features are crucial. This process helps create a structured dataset, allowing AI models to recognize patterns and improve their predictive capabilities. Second, real-time analysis of transcripts provides essential feedback for ongoing model adjustments, ensuring that AI continues to evolve and adapt. Utilizing such tools ultimately enhances the accuracy of AI-generated assessments and reinforces their credibility in various applications.
- OpenAIs GPT: Versatile language model with extensive data training features.
OpenAI's GPT stands as a powerful resource in the context of Historical Transcript Benchmarking. By utilizing extensive data training features, it can analyze diverse historical transcripts efficiently. This versatility enables organizations to create robust AI models that learn from significant datasets, fostering continuous improvement in performance and accuracy.
One of the key aspects of using such a language model lies in its capability to process and interpret vast amounts of textual data, which can greatly aid in benchmarking AI systems. By converting spoken or written historical interactions into structured data, it helps meticulously track performance metrics across different agents or scenarios. Furthermore, this application not only enhances understanding but also informs future training methodologies. As a result, organizations can tailor their AI solutions effectively, ensuring they remain aligned with industry best practices and evolving user needs.
- Amazon Comprehend: Powerful tool for text analytics and model training.
Amazon Comprehend serves as an invaluable resource for extracting insights from historical transcripts, particularly in the realm of AI model training. By analyzing large volumes of text data, it reveals patterns and trends that inform effective training models. This capability enhances the benchmarking process, allowing for the evaluation of AI models against pre-existing data. Through its advanced language processing features, Amazon Comprehend can identify keywords, sentiments, and entities, providing a comprehensive understanding of the textual content.
The power of Amazon Comprehend lies in its ability to streamline the analysis of historical transcripts. It supports various industries by facilitating benchmark comparisons and improving model accuracy. As organizations continuously adapt to market demands, leveraging such a tool not only boosts the precision of AI systems but also aligns them more closely with consumer expectations. In essence, Amazon Comprehend transforms raw data into actionable insights, essential for effective historical transcript benchmarking and AI training.
Steps to Implement Historical Transcript Benchmarking
To implement Historical Transcript Benchmarking effectively, begin with data collection and preparation. This involves sourcing transcripts from various communication channels, such as calls, meetings, or discussions. Ensure these transcripts are comprehensive and relevant to your benchmarking goals. Once collected, clean and preprocess the data, removing any noise that could affect the quality of your analysis.
Next, focus on model training and evaluation. Select appropriate AI algorithms for analyzing these transcripts, using tools designed for effective data processing. Train the models with your prepared transcripts, evaluating their performance against established benchmarks. Fine-tune your models based on their performance metrics until they yield satisfactory results. By diligently following these steps, Historical Transcript Benchmarking will provide valuable insights for enhancing AI models and ensuring quality assessments within your organization.
Step 1: Data Collection and Preparation
The process of data collection and preparation is foundational for historical transcript benchmarking. Effective benchmarking depends on high-quality data, which informs AI training. Start by sourcing diverse historical transcripts from multiple domains to ensure a robust dataset. This diversity helps capture various speech patterns and terminology, enabling the AI model to better understand different contexts.
Next, focus on cleaning and formatting the collected data. Remove any irrelevant information, correct errors, and standardize terms to improve consistency. Once the data is organized, segment it into categories based on attributes such as geography, industry, or customer type. This structured approach aids in finer analysis during model training, ensuring that the AI adapts meaningfully to specific contexts. By carefully managing data preparation, you set a solid groundwork for developing effective AI models tailored to real-world applications.
Step 2: Model Training and Evaluation
Training AI models effectively requires a robust approach to model training and evaluation. In this step, we focus on utilizing historical transcript benchmarking to refine the AI's learning process. This involves processing rich conversational data from historical transcripts to create a reliable and effective training framework. By meticulously analyzing these transcripts, we can develop benchmarks that guide model performance and improvement.
The model training process centers on uploading transcribed calls alongside the established evaluation criteria. The core goal is to assess how well the AI aligns with these benchmarks as it learns from historical data. Evaluating performance at different stages allows us to adjust training parameters, optimize algorithms, and ensure that the model not only learns but excels in its tasks. In essence, model training and evaluation serve as pivotal components in the journey of transforming raw historical transcripts into actionable insights for AI development.
Conclusion on Historical Transcript Benchmarking
Historical Transcript Benchmarking serves as a critical framework for successfully training AI models. By utilizing past conversations and data, organizations can establish a clear baseline for model performance. This process allows for more accurate evaluations as AI systems learn from diverse, real-world examples.
In conclusion, embracing historical transcripts enhances the benchmarking process, leading to robust AI training methodologies. It is essential to systematically analyze and utilize these valuable historical insights for continual improvement in AI model effectiveness. Ultimately, this focus results in stronger, more reliable AI systems capable of understanding and generating human-like responses.