In an increasingly digital world, organizations are turning to AI-Enhanced QA Scorecards to elevate their quality assurance processes. These scorecards serve as vital tools that utilize artificial intelligence to streamline evaluations, making them more precise and efficient. By automating data analysis and providing real-time feedback, stakeholders can easily track compliance and measure performance against established standards.
The adoption of AI-Enhanced QA Scorecards marks a significant shift in how businesses assess quality. Organizations can now benefit from quicker insights and improved accuracy in evaluations. This shift not only enhances the quality of service provided but also fosters a culture of continuous improvement, ultimately driving better outcomes for both employees and customers alike.
In todays digital landscape, organizations are increasingly relying on AI-Enhanced QA Scorecards to streamline their quality assurance processes. These tools integrate AI capabilities to provide more accurate and efficient evaluation metrics, ensuring that standards are consistently met.
AI-Enhanced QA Scorecards are becoming essential in modern quality assurance environments. These innovative tools leverage artificial intelligence to analyze vast datasets, enabling more precise evaluations. By automating complex data metrics, organizations can simplify their quality assurance processes, making it easier to assess performance against defined standards. This shift not only saves time but also ensures that evaluations are consistent and objective.
One crucial aspect of AI-Enhanced QA Scorecards is the ability to provide real-time feedback. Organizations can receive immediate insights about quality metrics, allowing for swift adjustments and improvements. Furthermore, these scorecards often include customizable features that align with specific criteria, tailored to an organization's unique needs. By embracing AI in quality assurance, organizations enhance their decision-making capabilities and consistently meet their defined quality standards.
Analyze qualitative data. At Scale.
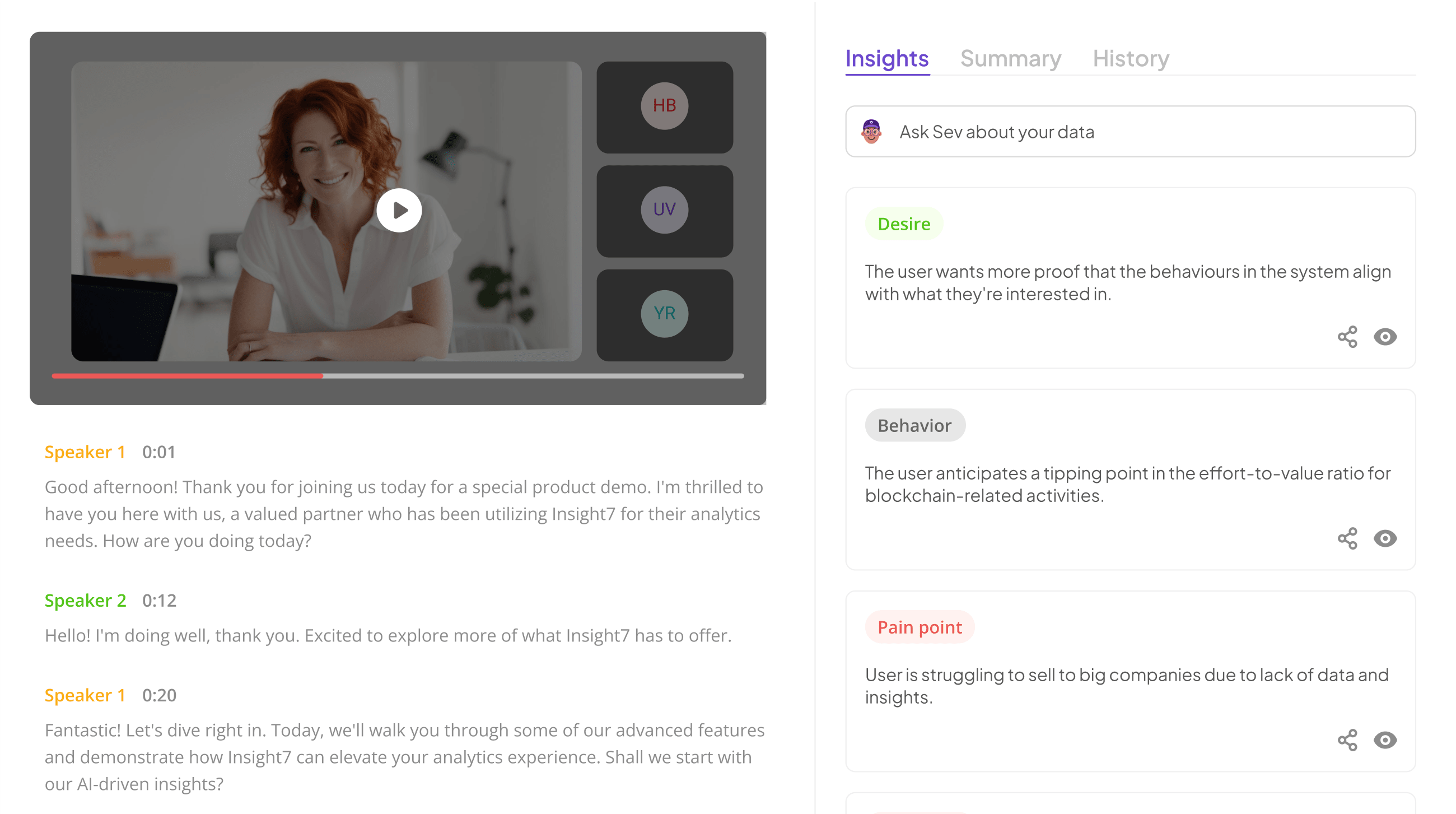
Building a Solid Foundation with AI-Enhanced QA Scorecards
Implementing AI-Enhanced QA Scorecards creates a structured foundation for quality assurance that is both efficient and effective. The initial step is to gain clarity on what these scorecards can offer, including their ability to automate complex data analysis and provide real-time feedback. Understanding the features of AI-enhanced tools helps organizations identify how these tools can integrate seamlessly into their existing processes.
Furthermore, organizations must consider the varied offerings available in the market. Key features like automated data analysis streamline the evaluation process, allowing teams to focus on implementation rather than data collection. Real-time feedback ensures that quality standards are continuously monitored, enhancing the overall customer experience. By selecting the right AI-Enhanced QA Scorecard, organizations lay the groundwork for a dependable quality assurance system that drives continuous improvement and aligns with their objectives.
The journey to implementing AI-Enhanced QA Scorecards begins with understanding their core features and selecting the right tools to meet organizational needs.
Implementing AI-Enhanced QA Scorecards begins with a deep understanding of their essential features. First, organizations must familiarize themselves with the capabilities of these scorecards, such as automated data analysis and real-time feedback mechanisms. These features are designed to streamline the quality assurance process, enabling teams to achieve higher efficiency and accuracy in their evaluations.
Next, selecting the right tools to meet organizational needs is crucial. Evaluating various platforms in the market will help identify which one aligns best with specific quality metrics and operational requirements. Key factors to consider during this selection process include ease of customization, integration capabilities, and AI-driven analytics. By focusing on these core elements, organizations can successfully embark on the journey of implementing AI-Enhanced QA Scorecards, ultimately leading to improved quality assurance outcomes.
Key Features of AI-Enhanced QA Scorecards
AI-Enhanced QA Scorecards deliver a robust mechanism for evaluating performance and ensuring quality standards are met. One key feature is automated data analysis, where AI simplifies complex metrics, making it easier for teams to derive insights rapidly. This efficiency allows organizations to focus on improvement areas without getting bogged down in extensive data interpretation.
Another vital aspect is real-time feedback, which empowers teams to receive immediate insights during quality assessments. This capability allows for dynamic adjustments and enhances overall performance standards. With AI-Enhanced QA Scorecards, companies benefit from an integrated approach that combines accuracy and convenience, ensuring that their quality assurance processes are both reliable and efficient. By adopting these features, organizations can significantly enhance their operational workflow and achieve their quality targets more effectively.
- Automated Data Analysis: How AI simplifies complex data metrics.
Automated Data Analysis within AI-Enhanced QA Scorecards revolutionizes how organizations handle complex data metrics. This technology simplifies the process by breaking down vast amounts of data into actionable insights, allowing teams to focus on critical decision-making rather than sifting through raw numbers. Traditional methods of analysis often involve time-consuming manual efforts, but AI streamlines this by offering automated summaries of key performance indicators.
Furthermore, AIโs ability to detect patterns across data sets enhances understanding of customer behavior and operational efficiency. By identifying recurring themes and pain points, businesses can make informed adjustments to their strategies. This ensures that quality assurance processes are not only efficient but also particularly responsive to real-time feedback and trends within the data.
In essence, using AI for automated analysis profoundly impacts how organizations approach quality assurance, promoting proactive improvements and enhancing overall performance.
- Real-time Feedback: Leveraging AI for instant quality insights.
Real-time feedback has become essential in the pursuit of quality assurance, where organizations strive for immediate insights. The integration of AI allows for swift analysis of performance metrics, enabling teams to identify areas of improvement almost instantaneously. This capability not only enhances the accuracy of assessments but also promotes a proactive approach to quality management.
By employing AI-Enhanced QA Scorecards, teams can gather detailed feedback on customer interactions effortlessly. The real-time capabilities mean that as data flows in, actionable insights are readily available, guiding teams towards immediate decisions. This efficiency fosters an adaptive environment where quality standards can be maintained and improved persistently. Thus, organizations can respond dynamically to consumer needs, ensuring continuous enhancement in their service delivery.
Choosing the Right AI-Enhanced QA Scorecard Builder
Choosing the right AI-Enhanced QA Scorecard Builder is a critical step for organizations aiming to elevate their quality assurance processes. The ideal tool should not only automate data analysis but also provide real-time feedback to improve evaluation metrics. When selecting a scorecard builder, consider how well it integrates with your existing systems and whether it meets your specific quality objectives. A user-friendly interface can significantly streamline the training process, enabling stakeholders to adapt to the new tool quickly.
Additionally, look for customizable features that allow you to adapt scorecards to the unique requirements of your clientele. Since each client may have distinct scripts and scoring criteria, flexibility is vital for ensuring the scorecard meets various compliance standards. Ultimately, the right AI-Enhanced QA Scorecard Builder can significantly transform your quality assurance efforts, leading to enhanced efficiency and more reliable outcomes.
Selecting the best tool for QA needs involves understanding the unique offerings of each platform. Below are some of the leading tools in the industry.
Selecting the best tool for QA needs requires a deep understanding of each platform's unique features and strengths. In the realm of AI-Enhanced QA Scorecards, various tools stand out for their innovative capabilities. For instance, some platforms excel at automated data analysis, which streamlines the evaluation process, allowing teams to focus on actionable insights rather than tedious calculations.
When exploring the leading tools, consider factors such as real-time feedback capabilities and the depth of analytical insights provided. These tools not only simplify the tracking of quality metrics but also enhance overall decision-making processes. By carefully evaluating each option, organizations can select a solution that best aligns with their specific quality assurance requirements. The right AI-Enhanced QA Scorecard can be a game changer, enabling teams to elevate their standards while efficiently managing their quality assurance workflow.
- Insight7: A leader in providing advanced AI-driven analytics for comprehensive QA scorecards.
In the realm of AI-Enhanced QA Scorecards, companies are recognizing the necessity for advanced analytics that can transform data into actionable insights. By employing sophisticated AI-driven techniques, these scorecards allow organizations to seamlessly evaluate and enhance their quality assurance processes. The ability to analyze vast amounts of data in real-time not only ensures adherence to quality standards but also empowers teams to make informed decisions swiftly.
Moreover, AI-enhanced scorecards excel in automating data analysis and providing instant feedback. This shift from traditional methods to AI-driven insights means organizations can now focus on critical areas for improvement more efficiently. For instance, companies can track performance metrics that align with their goals, paving the way for continuous enhancement in quality and customer satisfaction. With such tools, businesses are well-equipped to stay ahead of the competition, ensuring a seamless integration of insights into their operational strategies.
- ZenDesk: Efficient platform offering robust AI features.
The efficient platform in focus stands out for its robust AI features that fundamentally change how quality assurance is executed. By simplifying complex data analysis, it enables organizations to generate insights into customer interactions and operational efficiencies with remarkable ease. This platform allows users to quickly analyze recordings, pulling out critical themes such as pain points, desires, and behaviors that directly impact customer satisfaction.
Moreover, its intuitive interface promotes accessibility, meaning anyone in the organization can harness its capabilities without specialized training. This fosters a culture of insight-driven decision-making. With features such as automated reports and real-time feedback, embracing AI-enhanced QA scorecards becomes a seamless part of operational processes. Teams can not only monitor performance metrics but also act on insights to drive continuous improvement, transforming quality assurance from a reactive measure into a proactive strategy.
- MonkeyLearn: Known for its machine learning capabilities tailored for quality analysis.
Integrating machine learning into quality analysis revolutionizes how organizations approach their QA processes. AI-enhanced scorecards streamline evaluations by analyzing vast amounts of data, ensuring quality standards are consistently upheld. These tools empower teams to gain insights from various data points, making it easier for them to identify pain points and areas for improvement. The machine learning capabilities help in synthesizing complex data into understandable metrics, which facilitates informed decision-making.
Utilizing these advanced tools allows for seamless collaboration across departments. Teams can access actionable insights without extensive training, promoting a democratized approach to quality assurance. This not only fosters a culture of continuous improvement but also enhances customer satisfaction by addressing their needs more effectively. Integrating AI in QA processes marks a significant step forward, equipping organizations with the tools they need to thrive in today's competitive environment.
- Playvox: Specializes in contact center quality assurance with AI integration.
Quality assurance in contact centers often requires meticulous oversight, especially given the volume of interactions that occur daily. Within this context, AI integration plays a crucial role in refining QA processes. The use of AI-Enhanced QA Scorecards provides a powerful solution, helping organizations streamline their assessment of interactions while improving compliance and training outcomes.
AI-Enhanced QA Scorecards offer features such as automated data analysis and real-time feedback. Through advanced algorithms, they can sift through large volumes of recorded calls, extracting key insights and highlighting areas for improvement. This not only saves time but also permits managers to focus on critical coaching opportunities. By leveraging these AI-driven tools, businesses can ensure that their quality assurance efforts are not just effective but also scalable. Ultimately, this fosters an environment of continuous improvement and excellence in customer service.
- CallMiner: Offers detailed insights into call analytics and QA assessments.
In the realm of call analytics and quality assurance, detailed insights are paramount for organizational success. AI-Enhanced QA Scorecards provide the necessary framework to effectively analyze interactions, ensuring compliance with regulatory standards. By focusing on call data, these tools help organizations pinpoint areas for improvement and enhance the overall quality of customer interactions.
The capabilities of these scorecards extend beyond simple evaluations. For instance, automated data analysis turns complex call metrics into easily digestible insights, enabling teams to act swiftly on performance indicators. Moreover, real-time feedback mechanisms allow for immediate adjustments, fostering continuous improvement. Organizations benefit from prioritizing calls that matter most, selecting those with significant lengths and pertinent issues for thorough review. This strategic focus not only optimizes compliance but also enhances employee training through targeted coaching based on real performance data. Through these insights, businesses can ensure they maintain high standards while effectively managing their quality assurance processes.
Extract insights from interviews, calls, surveys and reviews for insights in minutes
Steps to Implement AI-Enhanced QA Scorecards
To implement AI-Enhanced QA Scorecards effectively, a structured approach is essential. First, organizations need to define clear objectives and metrics. This involves understanding the specific quality parameters that matter most to the company, enabling targeted assessments. By having well-defined goals, businesses can effectively measure progress and determine success over time.
Next, customizing scorecard features is crucial for aligning with unique organizational processes. This customization ensures that the scorecards reflect the specific quality benchmarks and standards of the company. Training stakeholders is the final step in the implementation process. Ensuring that team members understand how to utilize the AI-enhanced tools and interpret the resulting data is vital for maximizing the benefits of AI-Enhanced QA Scorecards. Emphasizing these steps will lead to more insightful evaluations and continuous improvement in quality assurance practices.
To effectively incorporate AI-Enhanced QA Scorecards, organizations should follow a structured approach.
To effectively incorporate AI-Enhanced QA Scorecards, organizations should follow a structured approach. This journey starts by defining clear objectives and metrics. Understanding the specific quality metrics that need measurement allows for tailored implementations that meet distinct evaluation needs. Next, teams must customize scorecard features to align with existing processes and quality benchmarks. This adaptability ensures the scorecard supports unique organizational goals rather than imposing generic standards.
Training stakeholders is equally crucial for success. Ensuring that all relevant team members have a solid grasp of how to utilize AI-enhanced tools empowers them to interpret data and insights effectively. This not only promotes engagement but also drives accountability. By setting these foundational steps, organizations can maximize the benefits of AI-Enhanced QA Scorecards, fostering a culture of continuous improvement and more reliable quality assessments over time.
Step 1: Define Objectives and Metrics
To create effective AI-Enhanced QA Scorecards, the first step is to define clear objectives and metrics. Organizations must identify the specific quality metrics they wish to measure, ensuring alignment with broader business goals. This process involves not only establishing quantitative metrics but also considering qualitative factors that may influence performance. For instance, regular evaluations of customer interactions can reveal training needs or knowledge gaps that numbers alone might not capture.
Additionally, it is crucial to set tangible objectives for your QA scorecard implementation. These objectives should guide the selection of metrics and the overall design of the scorecard itself. By clearly outlining what success looks like, organizations can better assess the impact of their AI-enhanced tools over time. Engaging relevant stakeholders in this process can further ensure that the scorecards address the unique challenges and benchmarks within the organization, leading to more meaningful insights and improvements.
Identify the specific quality metrics to measure and set clear objectives for the scorecard implementation.
Identifying specific quality metrics is crucial for the successful implementation of AI-Enhanced QA Scorecards. To begin, organizations must establish clear objectives that align with their overall quality assurance goals. This includes metrics such as customer satisfaction rates, compliance levels, and operational efficiency. Each metric should be quantifiable to facilitate accurate assessment and reporting.
Next, stakeholders should gather input from team members to ensure the selected metrics resonate with actual performance indicators. The implementation process can benefit from a mix of automated insights and manual evaluations. By using AI to continuously monitor these metrics, organizations can adjust objectives as needed, fostering an environment of ongoing improvement. The commitment to quality through these clearly defined metrics will enhance the effectiveness and reliability of AI-Enhanced QA Scorecards, making them invaluable for maintaining high standards.
Step 2: Customize Scorecard Features
To effectively tailor AI-Enhanced QA Scorecards, organizations should focus on customizing features that align with their specific needs. Start by evaluating the core metrics you wish to track, ensuring they reflect your quality assurance objectives. The flexibility of these scorecards allows you to add or modify evaluation criteria, enabling a more targeted assessment process.
Next, consider the user interface and functionality that best serve your team. Depending on whether you prefer a self-service model or require regular automated reports, customize the dashboard accordingly. You can include real-time feedback mechanisms, ensuring stakeholders receive immediate insights into their performance. By fine-tuning these elements, organizations can leverage AI-Enhanced QA Scorecards to drive continuous improvement and elevate quality standards.
Adapt the scorecard features to align with your organizations unique processes and quality benchmarks.
When adapting AI-Enhanced QA Scorecards to suit your organization's unique processes, several steps can ensure effective alignment with quality benchmarks. Start by identifying the specific criteria that resonate with your quality objectives. This process may involve defining key performance indicators that align with your operations and understanding customer feedback loops.
Next, incorporate custom features that reflect these defined metrics. For instance, you might prioritize certain aspects such as responsiveness or technical proficiency in customer interactions. By adjusting the scorecard's scoring weights and evaluating sub-criteria, you can formulate a comprehensive analysis tailored to your needs. Keeping this system adaptive supports continuous refinement as your organization grows and evolves, ensuring that the scorecards remain relevant and effective over time. By implementing these strategies, AI-Enhanced QA Scorecards can become powerful tools in driving your quality assurance efforts.
Step 3: Train Stakeholders
Training stakeholders is a crucial step in the implementation of AI-Enhanced QA Scorecards. This process ensures that all relevant team members are equipped with the knowledge and skills necessary to harness the power of these sophisticated tools. Begin by organizing training sessions focused on the scorecard's core functionalities, emphasizing its AI capabilities. Leaders and team members must understand how these features can simplify data interpretation and enhance decision-making.
Next, create an ongoing support framework that encourages questions and the sharing of best practices. This could include creating a resource hub with tutorials and FAQs, allowing stakeholders to continuously build their competency over time. Equipping your team with a strong grasp of AI-Enhanced QA Scorecards will foster a more data-driven culture and facilitate the adoption of quality assurance strategies that improve overall performance.
Ensure all relevant team members understand how to use the AI-enhanced tools and interpret the data.
Ensuring that all relevant team members understand how to use the AI-enhanced tools and interpret the data is crucial for maximizing the benefits of AI-Enhanced QA Scorecards. First, comprehensive training sessions should be organized, focusing on the functionalities and features of these tools. Each team member should have a clear grasp of how to input data, access insights, and perform queries effectively.
Next, it is vital to foster an environment where team members feel comfortable discussing their findings and asking questions. This can be achieved through regular workshops or meetings aimed at analyzing results and sharing best practices. Equipping the team with the skills to navigate AI-enhanced tools empowers them to make informed decisions, ultimately leading to improved quality assurance outcomes. Engaging with the data meaningfully will not only enhance the teamโs capabilities but also drive better results for the organization.
Conclusion
AI-Enhanced QA Scorecards are quickly becoming essential tools for organizations seeking to refine their quality assurance processes. These scorecards leverage advanced analytics to transform raw data into actionable insights, facilitating informed decision-making and ensuring consistent quality standards. As companies venture into this innovative space, they discover that the integration of AI not only streamlines workflows but also enhances the accuracy of evaluations.
Ultimately, implementing AI-Enhanced QA Scorecards can lead to significant improvements in operational efficiency and customer satisfaction. By embracing these tools, businesses position themselves to adapt to evolving market demands, maintain competitive advantage, and elevate their overall quality assurance efforts.
AI-Enhanced QA Scorecards are transforming the way organizations manage quality assurance by offering smarter, data-driven insights. By integrating these advanced tools, businesses can continuously improve their processes and achieve higher standards of quality.
AI-Enhanced QA Scorecards are revolutionizing quality assurance by providing innovative, data-driven insights that empower organizations to optimize their processes. These tools utilize advanced algorithms to analyze performance metrics, allowing businesses to quickly adapt and respond to quality challenges. As a result, companies experience more efficient evaluations that reveal areas for improvement, ultimately fostering a culture of excellence.
Moreover, AI integration facilitates real-time feedback, ensuring that organizations can make informed decisions promptly. By implementing these scorecards, companies no longer rely on outdated or manual assessment methods that consume valuable time and resources. Instead, businesses can harness continuous insights to maintain high standards of quality while streamlining operations. This transformative approach not only improves overall performance but also enhances customer satisfactionโmaking intelligent quality management a cornerstone of business success.