Top 10 AI Agents for Predictive Customer Interaction Analysis
-
Hello Insight
- 10 min read
Predictive AI Analytics is transforming the way businesses understand and engage with their customers. As customer interaction becomes increasingly complex, the need to analyze vast amounts of conversational data efficiently has never been greater. This innovative approach allows organizations to anticipate customer needs, identify trends, and enhance overall experience, driving both satisfaction and loyalty.
Incorporating Predictive AI Analytics into customer interaction analysis offers profound insights that help businesses stay ahead of the competition. By examining past interactions, companies can uncover valuable patterns and predict future behaviors. This section will explore the key benefits and transformative potential of utilizing AI agents in customer interaction analysis, setting the stage for a deeper dive into the top options available in the market today.
Analyze & Evaluate Calls. At Scale.
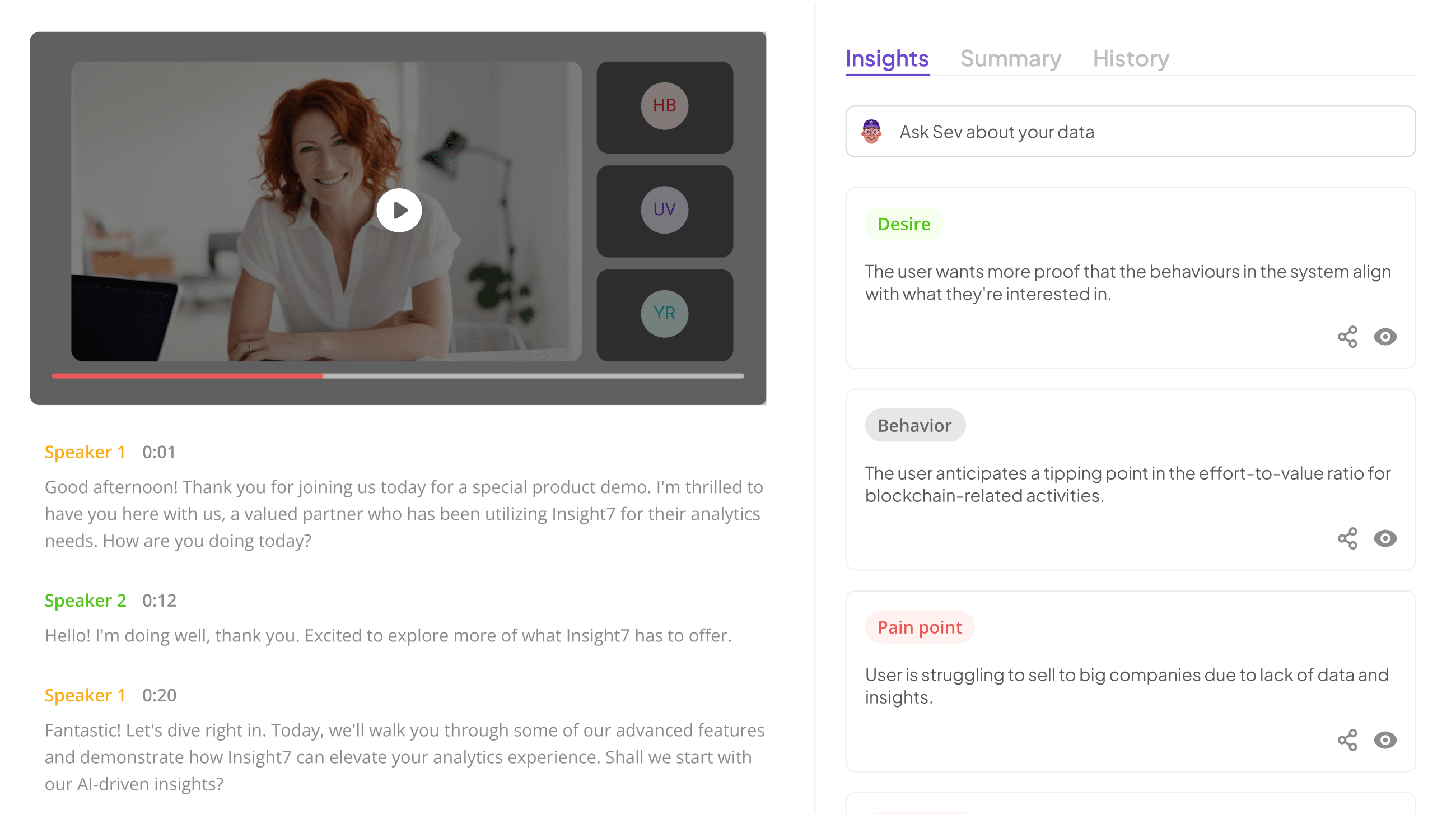
Understanding Predictive AI Analytics: Revolutionizing Customer Interactions
Predictive AI Analytics is transforming the way businesses interact with their customers, enabling a more proactive approach that enhances overall engagement. By harnessing vast amounts of data, companies can gain insights into customer behaviors and preferences, leading to improved service delivery. This analytical framework not only identifies trends but also anticipates future needs, allowing businesses to tailor their strategies accordingly.
As organizations embrace these advanced analytics, they can shift from reactive responses to proactive interactions. This change fosters a deeper connection with customers, as service representatives engage in meaningful conversations rather than merely responding to inquiries. By prioritizing customer engagement through Predictive AI Analytics, businesses can refine their offerings, improve satisfaction, and ultimately gain a competitive edge in the market.
The Role of Predictive AI Analytics in Modern Business Strategies
Predictive AI Analytics is transforming how businesses engage with their customers. By harnessing data-driven insights, organizations can anticipate customer needs and preferences, allowing for more personalized interactions. This proactive approach enables businesses to enhance customer satisfaction and loyalty, which are vital for sustained growth in today's competitive marketplace.
Integrating Predictive AI Analytics into modern business strategies also helps streamline operations. It minimizes response times, improves decision-making, and fosters collaboration across teams. Instead of relying on outdated methods to analyze customer data, businesses that adopt these advanced techniques can turn insights into actionable strategies. The outcome is a more agile organization that not only responds to market changes but also shapes customer experiences in real time. As businesses continue to evolve, those leveraging Predictive AI Analytics are poised to lead their industries by effectively addressing customer demands and driving innovation.
Key Benefits of Implementing Predictive AI Analytics
Implementing Predictive AI Analytics offers businesses a transformative approach to customer interaction. One key benefit is enhanced customer understanding, enabling companies to tailor their strategies based on data-driven insights. This approach allows for the identification of trends and patterns, leading to more effective marketing and communication strategies.
Another significant advantage is improved operational efficiency. By automating data analysis, teams can allocate their resources effectively, reducing manual processes and focusing on critical tasks. Additionally, Predictive AI Analytics provides a competitive edge, allowing businesses to adapt quickly to market changes and customer preferences. These insights facilitate proactive decision-making, helping organizations stay ahead of their competition. Importantly, this analytical capability fosters better customer experiences, ultimately leading to increased loyalty and satisfaction.
[hre_template id=22694]Unveiling the Top 10 AI Agents for Predictive Customer Interaction Analysis
In navigating the evolving landscape of customer interactions, the importance of predictive AI analytics cannot be overstated. This section unveils ten powerful AI agents designed to enhance predictive customer interaction analysis. By harnessing these tools, businesses can glean actionable insights from vast amounts of data and significantly improve customer engagement strategies.
The first agent on our list is H2O.ai, an open-source platform that empowers users with robust machine learning capabilities. Following closely is RapidMiner, a data science platform that excels in streamlining predictive analytics processes. Next, we have DataRobot, offering automated machine learning solutions that simplify the deployment of AI models. Lastly, SAS Predictive Analytics stands out with its advanced analytics features tailored for comprehensive data analysis. These AI agents not only expedite data interpretation but also drive strategic decision-making in customer interactions, ensuring companies remain competitive in an ever-changing market.
insight7: Leading the Charge in Predictive AI Analytics
The realm of predictive AI analytics is transforming how businesses interact with their customers. By mining vast amounts of customer data, organizations can now anticipate needs and tailor experiences accordingly. This shift enables proactive engagement rather than merely reactive responses, ensuring that companies stay ahead of customer expectations.
Understanding the implications of predictive AI analytics is crucial for modern business success. It empowers teams to discern patterns from customer interactions and derive actionable insights. These insights help in making informed decisions that enhance customer satisfaction and foster loyalty. By adopting advanced predictive analytics tools, companies can streamline their processes and optimize outcomes, ultimately leading to a more responsive and customer-centric approach. This evolution marks a significant shift in how organizations manage their customer relationships, prioritizing data-driven strategies to stay competitive in a fast-paced market.
Additional Tools for Predictive Customer Interaction Analysis
In addition to the top AI agents, several essential tools can elevate predictive customer interaction analysis. These additional tools complement predictive AI analytics, addressing the growing need for efficient data analysis and actionable insights. H2O.ai stands out as a powerful open-source platform, enabling users to harness extensive machine learning capabilities for their unique requirements. RapidMiner offers a comprehensive data science platform, designed for predictive analytics, making it accessible to analysts at various skill levels.
Furthermore, DataRobot enhances business efficiency with its automated machine learning solutions, helping organizations glean insights faster. Lastly, SAS Predictive Analytics provides advanced analytics features that empower companies to make informed decisions driven by solid data analysis. Together, these tools illustrate how businesses can optimize their predictive analytics strategies, ensuring they stay competitive and responsive to customer needs.
- H2O.ai: Open-source machine learning platform
An open-source machine learning platform plays a crucial role in the realm of predictive AI analytics, facilitating the development of robust models that drive customer interaction insights. Its intuitive design democratizes access to advanced analytics, enabling users with varying expertise levels to effortlessly generate actionable insights. This platform allows organizations to streamline their data processing efforts, transforming raw data into dynamic reports that can be utilized for various business needs.
Moreover, the platform supports a range of functionalities, such as analyzing customer calls and identifying key pain points that can improve service delivery. Users can interact with the system to extract meaningful insights quickly, making it a powerful tool for any team looking to enhance their customer engagement strategies. By utilizing such open-source technologies, businesses can stay competitive, adapting their strategies based on real-time customer feedback and behaviors. This encourages a deeper understanding of customer preferences, ultimately enhancing the overall customer experience.
- RapidMiner: Data science platform for predictive analytics
RapidMiner stands out as a premier data science platform designed for predictive analytics. It simplifies the process of extracting insights from vast datasets, making it accessible to teams irrespective of their technical background. Users can easily perform analyses, generate reports, and identify pain points that impact customer experience without needing extensive training.
The platform’s intuitive interface allows users to upload and analyze files seamlessly. It offers features that automatically extract key insights from conversations and interactions, presenting these findings in a digestible format. By doing so, it transforms raw data into actionable intelligence that can inform business decisions. In a world where predictive AI analytics is essential for understanding customer interactions, tools like this accelerate the journey from data collection to strategic application, ultimately promoting enhanced decision-making and customer satisfaction.
- DataRobot: Automated machine learning solution
The automated machine learning solution offers a transformative approach to predictive AI analytics in customer interaction analysis. This platform enables businesses to streamline the processes of data discovery, modeling, and deployment without requiring extensive expertise in machine learning. Users benefit from intuitive interfaces that demystify complex algorithms, allowing them to derive meaningful insights into customer behavior and preferences.
Key capabilities include automatic feature selection which enhances model accuracy by identifying the most impactful variables within datasets. Additionally, this solution provides a variety of pre-built models that cater to diverse business needs, facilitating quicker time-to-market for analytical initiatives. By automating repetitive tasks, organizations can focus on interpreting results and making informed decisions, significantly improving customer engagement strategies. As enterprises navigate the complexities of customer data, integrating such automated tools can serve as a pivotal step in enhancing their predictive capabilities.
- SAS Predictive Analytics: Advanced analytics platform
In the realm of customer interaction analysis, predictive AI analytics stands out as a powerful tool for businesses aiming to enhance their engagement strategies. This advanced analytics platform seamlessly integrates data from various sources to uncover patterns and trends in customer behavior. By leveraging predictive models, organizations can anticipate customer needs and tailor their interactions accordingly.
With user-friendly interfaces, these platforms enable individuals across all levels of a business to access insights without extensive training. Users can analyze conversations and extract key themes such as pain points and desires from customer feedback. This functionality not only democratizes data access but also fosters informed decision-making based on real-time insights. By implementing predictive AI analytics, businesses can achieve a proactive approach in their customer interactions, ultimately driving satisfaction and loyalty.
How to Choose the Best AI Agent for Your Business
When selecting the best AI agent for your business, begin by clearly identifying your specific business needs and goals. Understanding what you want to achieve is critical; whether it’s enhancing customer engagement, improving sales forecasting, or streamlining customer interactions, each AI agent offers distinct capabilities. Aligning those needs with the functionalities of the AI agent ensures a suitable match.
Next, evaluate each candidate's capabilities in predictive AI analytics. Look for features such as data processing power, ease of integration, and customization options. Assess how well the agent can analyze customer interactions and predict future behaviors. Additionally, consider their track record and user feedback, as real-world effectiveness often speaks louder than marketing claims. By methodically assessing these factors, you can confidently choose an AI agent that not only meets your current needs but will also evolve alongside your business.
Step 1: Identify Your Business Needs and Goals
To effectively harness Predictive AI Analytics for your organization, the first step is identifying your business needs and goals. Begin by assessing your current customer interaction practices, as this will guide your AI implementation strategy. Consider the specific challenges your team faces, such as understanding customer preferences or streamlining communication processes.
Next, outline clear, measurable objectives that align with your overall business strategies. This could include enhancing customer satisfaction scores, increasing engagement rates, or improving conversion rates. By establishing these goals upfront, you can ensure that any predictive analytics tools you adopt directly support your vision. Engaging stakeholders in this process helps create a shared understanding of expectations and priorities, ensuring more effective use of AI agents moving forward.
Step 2: Evaluate Each AI Agent’s Capabilities
In this critical step of evaluating AI agents, we focus on dissecting their capabilities to enhance predictive customer interaction analysis. Begin by assessing the unique features each AI agent offers, such as its data processing power, real-time analytics capabilities, and user-friendliness. Understanding these elements is essential for determining how well they align with your specific business needs.
Next, consider their adaptability in various customer engagement scenarios. Does the AI solution exhibit versatility in handling different types of customer inquiries? Additionally, evaluate its accuracy in predictive AI analytics. The precision of the insights generated can directly influence customer satisfaction and retention rates. Lastly, factor in user feedback and market performance, as this will provide a clearer picture of the agent's reliability in real-world applications. By meticulously evaluating these capabilities, you can make an informed decision on the ideal AI agent for your predictive analytics needs.
Conclusion: Harnessing Predictive AI Analytics for Enhanced Customer Interaction
In conclusion, harnessing Predictive AI Analytics is essential for improving customer interactions in today’s competitive landscape. By implementing these advanced tools, businesses can gain meaningful insights into customer behavior and preferences, allowing them to tailor their strategies effectively. This proactive approach transforms data into actionable insights, enabling companies to address customer needs more quickly and efficiently.
Moreover, leveraging Predictive AI Analytics fosters a more engaging customer experience. Organizations that utilize these analytics can anticipate customer inquiries, thus enhancing communication and satisfaction. As businesses continue to adopt these technologies, they not only improve their responsiveness but also strengthen customer relationships, setting a solid foundation for long-term success.