Automated Interview Insights hold immense potential for transforming the way businesses identify messaging opportunities in interview transcripts. By integrating advanced technologies like Natural Language Processing and machine learning, organizations can shift from manual tagging processes to efficient and consistent automation. This transition not only saves valuable time but also enhances the reliability of the insights drawn from interview data.
As businesses strive to understand customer sentiments, automated tagging emerges as a critical tool. It enables teams to rapidly pinpoint emerging themes and valuable messaging strategies, facilitating informed decision-making. By investing in automated interview insights, organizations position themselves to gain a competitive edge, effectively engaging their audience through data-driven approaches.
Analyze qualitative data. At Scale.
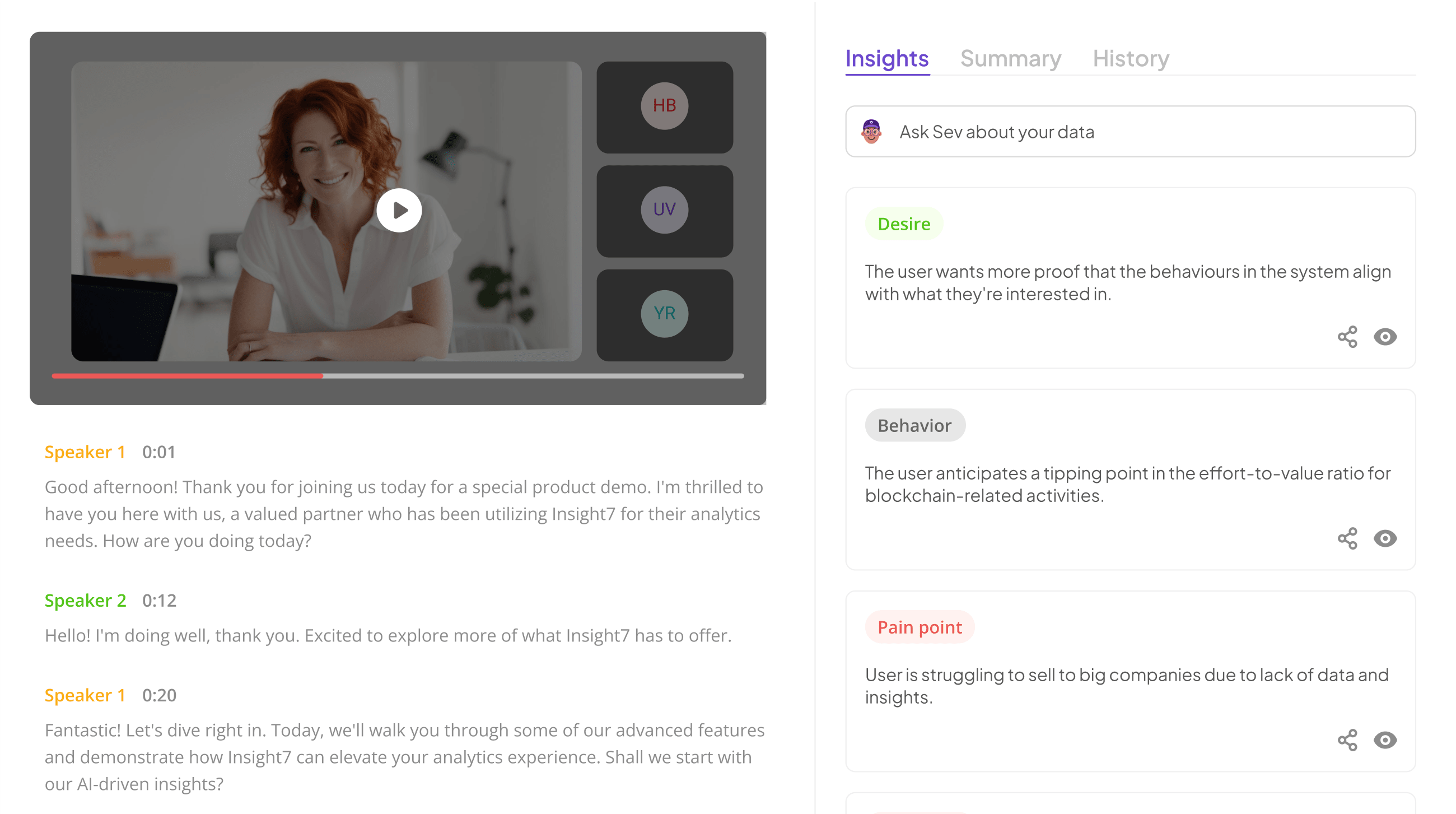
Understanding the Need for Automated Interview Insights
Automated Interview Insights are essential for streamlining the analysis of interview transcripts, significantly enhancing efficiency and accuracy. Manual tagging of transcripts can be labor-intensive and fraught with inconsistencies, leading to unreliable data analysis. By automating this process, organizations can focus on high-level insights rather than getting bogged down in time-consuming tasks. This shift not only saves valuable resources but also enables teams to dedicate more time to strategic decision-making based on accurate and consistent data.
Implementing automated solutions within the analysis of interview transcripts allows for precise tagging of messaging opportunities. This ensures that relevant insights are accurately identified and categorized. Additionally, the use of advanced technologies, such as natural language processing and machine learning, further refines the tagging process. These technologies continuously improve accuracy and adapt to new data, ultimately transforming how organizations engage with their messaging strategies. Automated Interview Insights pave the way for deeper understanding and more effective communication strategies in today’s data-driven environment.
The Problem with Manual Tagging
Manual tagging of interview transcripts presents significant challenges that can hinder effective analysis. First, the time-consuming nature of manually tagging each transcript can lead to overwhelming workloads, causing delays in extracting valuable insights. This slow process can ultimately result in missed opportunities for growth and innovation.
Second, inconsistency in tagging is another major issue. Different analysts may interpret responses in varying ways, leading to unreliable results. Such discrepancies can make it difficult to identify key themes and trends accurately. In a world where timely and precise Automated Interview Insights are essential, relying solely on manual methods can be detrimental. The adoption of automated systems can help standardize the tagging process, ensuring consistency and improving overall efficiency, ultimately unlocking the full potential of interview transcripts.
- Time-consuming process
Interview transcript analysis can be an arduous task, particularly when it comes to tagging messaging opportunities. This time-consuming process often requires meticulous attention to detail and extensive manual effort, leading to inefficiencies. Teams tasked with analyzing large volumes of interview data may find themselves overwhelmed, leading to delays in gaining actionable insights from their findings.
The challenges can be exacerbated by inconsistencies that arise from manual tagging. Different analysts may interpret the same data in varying ways, creating a lack of standardization that hinders overall outcomes. Many organizations struggle with this aspect, often resulting in missed opportunities. By automating the tagging process through advanced technologies, businesses can streamline operations and achieve a high level of accuracy. Automated Interview Insights can help eliminate these issues, allowing teams to focus on deriving meaningful conclusions instead of getting mired in repetitive tasks. This transition not only saves time but also enhances the reliability of insights generated from interview data.
- Inconsistency in the outputs
Inconsistencies in the outputs when utilizing automated methods for tagging messaging opportunities can hinder the overall efficiency of the interview analysis process. While automated interview insights aim to provide a streamlined approach, discrepancies in data interpretation often arise from varying transcription quality and algorithmic limitations. Such inconsistencies may lead to misalignment in pinpointing critical messaging opportunities, affecting decision-making and strategic planning.
To address these issues, it is crucial to recognize the factors contributing to output variability. First, the accuracy of transcription greatly influences the subsequent tagging process. If the initial data is flawed or inconsistent, the automated tagging tools will inevitably reflect those shortcomings. Second, the algorithms utilized for tagging need continuous training and improvement to adapt to diverse conversational contexts. Lastly, implementing regular validation checks can help identify and correct inconsistencies, enhancing the reliability of the insights derived from the transcripts. By prioritizing these elements, organizations can improve their automated interview insights significantly.
Benefits of Automation in Interview Analysis
Automation in interview analysis streamlines the process, offering numerous advantages that enhance the efficiency of extracting meaningful insights. By deploying automated systems, organizations can quickly analyze vast amounts of data, significantly reducing the time spent on manual tagging. This allows teams to focus on interpreting the insights rather than getting bogged down in repetitive tasks, ultimately leading to better strategic decisions.
Another critical benefit is the consistency and accuracy provided by automated solutions. Unlike manual methods, which may vary due to individual interpretation, automated interview insights ensure uniform application of tagging criteria across all transcripts. This standardization helps remove bias and improves reliability in messaging opportunities, allowing organizations to better understand customer feedback and sentiments. By transforming how interview data is processed, automation not only enhances productivity but also fosters a deeper, clearer understanding of user needs.
- Improves efficiency
Automated Interview Insights streamline the tagging of messaging opportunities, significantly enhancing efficiency in the analysis process. Traditionally, manual tagging consumes valuable time and is prone to errors. By automating this task, teams can focus on strategic analysis instead of getting bogged down by repetitive details.
Automated systems work by leveraging advanced algorithms to analyze interview transcripts quickly and accurately. The technology not only reduces time spent on tagging but also enhances consistency across outputs. This uniformity is crucial for deriving actionable insights that drive business decisions. Moreover, automated tagging allows for a more in-depth understanding of candidate interviews, easily highlighting patterns and trends that could be missed through manual methods. The result is a more effective approach to sifting through volumes of data, enabling stakeholders to make timely, informed decisions based on clear and precise messaging opportunities.
- Ensures consistency and accuracy
Automated Interview Insights enhance both consistency and accuracy in tagging messaging opportunities within interview transcripts. This technology employs advanced algorithms to analyze vast amounts of data swiftly. As a result, the likelihood of human error diminishes significantly, leading to more reliable insights. This ability to process information uniformly ensures that similar themes and messaging are tagged consistently across different transcripts.
Ensuring this level of consistency is crucial for stakeholders who rely on accurate data for decision-making. Moreover, by automating the tagging process, organizations can focus on the analytical aspects rather than spending excessive time on manual tagging. Without the unpredictability of human intervention, the outcomes are more precise, which ultimately contributes to informed strategies. Consistency and accuracy in tagging not only foster trust but also streamline future analyses. Thus, embracing automated solutions in interview insights becomes a strategic imperative for effective communication.
[ hfe_template id=22694]Key Steps in Automating Interview Transcript Tagging for Messaging Opportunities
Automating interview transcript tagging for messaging opportunities involves several key steps that turn qualitative data into actionable insights. The first step is data preparation, which entails gathering, transcribing, and cleaning interview data. Proper formatting is crucial, as it sets the stage for a seamless analysis process. This groundwork ensures that the raw data is ready for further examination, boosting efficiency in identifying key themes.
The second step is implementing Natural Language Processing (NLP) techniques. By utilizing NLP, you can achieve a deeper semantic understanding of the interview content. This technology helps in pinpointing key messaging opportunities within the transcripts. Finally, employing machine learning algorithms allows for the continuous improvement of tagging accuracy. Training models with accumulated data helps refine the process, enabling the system to better recognize valuable insights in future interviews. By directly addressing how these steps contribute to Automated Interview Insights, organizations can enhance their communication strategies significantly.
Step 1: Data Preparation
Data preparation is the foundational step in the journey toward Automated Interview Insights. It starts by gathering and transcribing the interview data accurately. This initial collection is crucial because the quality of your transcripts will directly affect the analysis that follows. Careful transcription ensures that every nuance of conversation is captured, providing a rich resource for insight extraction.
Once the data is gathered, the next phase involves cleaning and formatting the transcripts. This process includes removing unnecessary elements, such as filler words, and standardizing text for consistency. Properly formatted data not only enhances readability but also improves the efficiency of subsequent analysis techniques. By thoroughly preparing your data, you establish a strong basis for the following steps in automation, ensuring that the insights derived from the interviews are both meaningful and actionable.
- Gathering and transcribing interview data
Gathering and transcribing interview data is a fundamental step in harnessing Automated Interview Insights. The process begins with carefully collecting information from participants, ensuring that each response is accurately captured. This step is critical for creating an effective database of insights that can later be analyzed for messaging opportunities.
Once the interviews are conducted, transcription becomes essential. Transcribing involves converting spoken language into written text, which must be done meticulously to avoid errors that could skew the analysis. Errors can lead to misinterpretation of key themes. To streamline this, various tools like Insight7 can be employed alongside other transcription software, enhancing accuracy and saving time. By utilizing automated tools, researchers can focus on analyzing the data, leading to more reliable and actionable insights in future communication strategies.
- Cleaning and formatting for analysis
Effective data preparation is essential for achieving accurate Automated Interview Insights. This involves both cleaning and formatting interview transcripts to make them suitable for analysis. Initially, raw transcripts often contain extraneous details and errors that can lead to misleading results. Therefore, the first step is to carefully review the transcripts, eliminating any irrelevant information and correcting transcription errors. This meticulous process enhances the quality of the data and ensures that the insights derived are both trustworthy and relevant.
Once the transcripts are cleaned, the next step involves formatting them for analysis. This includes structuring the data consistently, such as organizing dialogue into categorized themes or tags. By adopting a standardized approach to formatting, teams can more easily identify key messaging opportunities within the transcripts. Tools like Insight7 can assist in this process, enhancing the clarity and usability of the data for further analysis. Through effective cleaning and formatting, organizations can unlock deeper insights, ultimately leading to more informed decision-making and strategy development.
Step 2: Implementing Natural Language Processing (NLP) Techniques
Implementing Natural Language Processing (NLP) Techniques is integral to extracting Automated Interview Insights from transcripts. By applying NLP, we can effectively analyze textual data, enabling us to decode the meaning hidden within conversations. This process involves the use of various algorithms that interpret language nuances, ensuring a better understanding of the attendees' messages.
The first step in this implementation is to employ semantic analysis, which helps identify the context and sentiment of participants' responses. This involves detecting key phrases and themes prevalent in the discourse. Once these are highlighted, the next focus is on tagging messaging opportunities that arise from recurring terms or ideas highlighted during interviews. A combination of automated techniques not only minimizes manual effort but also enhances the accuracy and reliability of the tagging process, paving the way for clear and actionable insights.
- Using NLP for semantic understanding
Natural Language Processing (NLP) serves as a powerful tool for extracting meaning from unstructured data, such as interview transcripts. By using NLP for semantic understanding, researchers can automatically identify key messaging opportunities within lengthy dialogues. This ability enhances the efficiency of analyzing interviews, allowing teams to pinpoint important sentiments and themes that may otherwise go unnoticed. With automated systems handling this analysis, organizations can save substantial time while minimizing human error.
Moreover, the implementation of NLP enables the categorization of segments based on the context of discussions. It identifies not just words, but also the underlying meanings and nuances, leading to a deeper understanding of the interviewee's perspective. Automated interview insights are thus gathered quickly and effectively, paving the way for informed decision-making. As organizations explore these advancements, they can transform raw data into strategic advantages. Insights derived from this method can inform messaging strategies, leading to more focused and targeted communication efforts.
- Identifying key messaging opportunities
To effectively identify key messaging opportunities within interview transcripts, it’s essential to utilize Automated Interview Insights. This process involves a systematic approach where automated tools analyze the text to pinpoint prevalent themes, sentiments, and essential messages. By employing advanced techniques like Natural Language Processing (NLP), the system can discern the nuances of conversation, enabling companies to unearth valuable insights from interview data.
The first step is preparing the data. This includes transcribing audio interviews and cleaning the text for accurate analysis. Once the data is organized, implementing NLP techniques will facilitate the identification of critical messaging opportunities. This enables organizations to capture the most relevant insights that resonate with their target audience. Finally, it’s crucial to continuously refine the system using feedback loops, ensuring ongoing accuracy in tagging these messaging opportunities as trends evolve over time.
Step 3: Utilizing Machine Learning Algorithms
Machine learning algorithms play a crucial role in transforming interview transcripts into actionable insights. By training models specifically designed for tagging messaging opportunities, organizations can enhance the accuracy and efficiency of their analysis. Automated interview insights are achieved through these algorithms, which identify patterns and relationships within the data that might be overlooked in manual tagging.
To implement machine learning effectively, consider the following steps: First, ensure that you gather a diverse dataset of interview transcripts to train the model on varied scenarios. This diversity will help improve the robustness of the models. Next, iteratively refine the model using feedback from users, adjusting parameters based on real-world performance and the evolving understanding of messaging opportunities. Finally, incorporate user feedback continuously to enhance model accuracy over time, ensuring the insights provided remain relevant and valuable.
- Training models for accuracy in tagging
Training models for accuracy in tagging is a crucial step toward achieving Automated Interview Insights. The effectiveness of these models hinges on training them with quality data, which directly impacts their precision. By employing advanced machine learning algorithms, organizations can automate the tagging process, resulting in consistent outputs that highlight key messaging opportunities. This accuracy is important for drawing actionable insights from interview transcripts.
To enhance model performance, it’s essential to implement feedback loops that continuously refine tagging accuracy. Initially, the model should be trained on a diverse set of tagged transcripts, allowing it to learn various tagging scenarios. Furthermore, regular assessments and updates based on new interview data can improve its adaptability. Consequently, these measures guarantee that the tagging remains relevant and accurate, ultimately leading to more insightful analysis and decision-making in messaging strategies.
- Continuous improvement through feedback loops
Feedback loops are integral to achieving continuous improvement in automated processes. In the context of automated interview insights, they foster an environment of learning and adaptation. By systematically analyzing the output of tagging systems, teams can refine algorithms, enhancing the accuracy and relevance of insights derived from interview transcripts. Each feedback iteration should focus on identifying areas for improvement while celebrating the successes achieved through automation.
Moreover, employing automated tools, such as Insight7, allows for seamless integration of feedback mechanisms that inform further advancements. Regular assessments of automated tagging results not only ensure consistency but can also reveal new messaging opportunities within the transcripts. As these feedback loops become more robust, they provide a foundation for more insightful and actionable data, ensuring that automated interview insights continually evolve to meet user needs effectively. Thus, embracing feedback loops is essential for maintaining quality and driving future developments in messaging analysis.
Top Tools for Extracting Automated Interview Insights
Automated Interview Insights play a crucial role in maximizing the value extracted from interview transcripts. Utilizing cutting-edge tools can significantly yield actionable insights that boost messaging strategies. One of the leading tools in this space is Insight7, which streamlines data analysis by providing efficient transcription and coding capabilities. This tool enables researchers to identify key messaging opportunities with ease and accuracy.
In addition to Insight7, several other tools offer valuable functionalities for automated insights. Otter.ai excels in generating comprehensive transcriptions while providing advanced analytics features. Sonix is known for its speed and accuracy in transcription, making it a reliable choice for quick turnaround projects. Trint offers automated transcription paired with editing tools, while Descript allows for seamless text-based editing of audio and video content. Lastly, Rev provides human-verified transcription services, ensuring the highest quality output. These tools collectively enhance the process of extracting valuable insights from interviews, ultimately driving effective messaging opportunities.
Insight7
Automated Interview Insights empower organizations to streamline the tagging of messaging opportunities found within interview transcripts. This process significantly reduces the burden of manual tagging, which is often time-consuming and fraught with inconsistencies. By harnessing advanced technologies, businesses can accurately identify key themes, enabling deeper analysis and clear messaging strategies.
First, the deployment of Natural Language Processing (NLP) techniques allows for semantic understanding of the conversation, facilitating the extraction of essential insights. This is further enhanced by machine learning algorithms that improve tagging accuracy over time. Regular feedback loops support continuous enhancement, ensuring the system evolves alongside your data needs. Ultimately, employing Automated Interview Insights fosters a more efficient approach, saving valuable time while ensuring that critical information remains appropriately tagged and easily accessible for future reference.
- Features and benefits
Automated Interview Insights offer significant features and benefits that streamline the analysis of interview transcripts for messaging opportunities. This approach enhances efficiency, enabling users to quickly sift through large volumes of data. By replacing manual tagging processes, organizations can ensure minimal human error and achieve greater consistency in their outputs. This improved accuracy increases the reliability of insights gathered from interviews.
One of the key features of this automation is the application of advanced Natural Language Processing (NLP) techniques. NLP allows for a more nuanced understanding of the content, effectively pinpointing potential messaging opportunities hidden within the transcripts. Additionally, the integration of machine learning algorithms continually refines the tagging process based on feedback, facilitating ongoing improvements. In summary, leveraging Automated Interview Insights not only saves time but also enhances the quality of data analysis, leading to more informed decision-making.
Additional Tools
Automated Interview Insights open a world of possibilities, especially when it comes to tagging messaging opportunities. First, consider tools like Otter.ai, which enhances transcription accuracy while offering analysis features. This tool simplifies the process, making it faster to draw insights from interview data. Additionally, services such as Sonix and Trint provide fast transcription along with essential editing capabilities, ensuring that your transcripts remain clear and relevant to your messaging goals.
Furthermore, Descript allows users to edit audio and video content through text-based methods, making it easy to create polished versions of your data. Lastly, Rev offers human-verified transcription services that can help uphold accuracy, particularly in complex interviews. By integrating these tools, organizations can optimize their approach to extracting Automated Interview Insights, resulting in more effective strategies for messaging and communication. This harmonious combination of tools empowers users to focus on what truly matters—delivering impactful messages based on reliable insights.
- Otter.ai: Enhanced transcription and analysis
Analyzing interview transcripts for messaging opportunities can be an overwhelming task without effective tools. This is where enhanced transcription and analysis solutions come into play. By utilizing advanced features, such tools streamline the transcription process, transforming raw audio into structured text efficiently. This significantly reduces manual effort while ensuring high accuracy, allowing teams to focus on deriving actionable insights from the data.
The integration of Natural Language Processing (NLP) not only automates the transcription but also enhances the analytical capabilities of the output. Automated Interview Insights become more accessible when these tools can identify key themes and trends within conversations seamlessly. Continuous learning through machine learning algorithms further refines the system, ensuring that tagging remains precise over time. With tools capable of efficiently handling large amounts of qualitative data, businesses can unlock valuable messaging opportunities that were previously hidden in nuanced discussions.
- Sonix: Fast and accurate transcription services
Automated Interview Insights into tagging messaging opportunities rely significantly on transcription services that offer both speed and precision. With fast and accurate transcription, the time spent on manual data entry diminishes, allowing researchers to focus on analyzing and interpreting the data instead. This is particularly important for understanding the interview context, uncovering key themes, and identifying actionable messaging opportunities within transcripts.
To maximize the potential of automated insights, it is essential to utilize advanced transcription tools. By leveraging these services, the frustration of inaccurate transcriptions and prolonged turnaround times is eliminated. With a streamlined process, valuable insights can be extracted more efficiently, enabling teams to react promptly to trends and shifting audience needs. Consequently, integrating accurate transcription into the research workflow not only enhances productivity but also enriches the quality of insights derived. This ensures that organizations can adapt their messaging strategies effectively, staying ahead in an ever-evolving market.
- Trint: Automated transcription with editing capabilities
Automated transcription with editing capabilities has revolutionized the way we handle interview data, pushing boundaries in extracting value from conversations. This tool enables users to transform spoken dialogue into written transcripts swiftly, allowing for easier search and review. As a result, organizations can significantly enhance productivity and capture critical insights that might otherwise be overlooked in manual processes.
Moreover, these editing capabilities grant the ability to refine transcripts for clarity and coherence. Users can easily edit text to maintain accuracy, thereby ensuring the insights gathered reflect the true meaning of the interviews. With automated interview insights, organizations can tag relevant messaging opportunities quickly and effectively, paving the way for enhanced strategic decision-making. Ultimately, this seamless combination of automation and user control sets a new standard for managing interview data, unlocking potential that was previously hard to harness.
- Descript: Text-based editing for audio and video
Descript serves as a powerful tool for text-based editing of audio and video content. Users can streamline their editing processes by manipulating transcripts directly, enabling a more intuitive interaction with their media. This capability is especially beneficial for those generating Automated Interview Insights, as it allows quick identification and tagging of key moments.
With Descript, editing becomes an engaging experience where audio and text merge seamlessly. Users can easily cut, copy, and paste text to modify audio tracks without the traditional complexities of audio editing software. This functionality not only enhances the user experience but also ensures accuracy in capturing important messaging opportunities within interview transcripts. By enabling efficient, real-time edits, Descript helps content creators focus on generating insights that matter, making the analysis of interviews both faster and more reliable.
- Rev: Human-verified transcription services
Human-verified transcription services play a crucial role in extracting Automated Interview Insights from your data. These services ensure that your interview transcripts are not only accurate but also contextually relevant. The use of trained professionals in transcribing helps eliminate common errors associated with machine transcription, providing a robust foundation for analysis.
By utilizing human-verified transcription services, organizations can greatly enhance the quality of their interview data. This allows for effective tagging of messaging opportunities, ensuring that insights are clear and actionable. Implementing these services supports the automation process, enabling businesses to draw meaningful conclusions from their interviews. This is particularly beneficial as it enhances consistency in results and reduces time spent on manual review. Ultimately, the integration of human-verified transcriptions into automated systems significantly improves the quality of insights derived from interview data, paving the way for better strategic decisions.
Conclusion: Transforming Insights into Action for Better Communication
Automated Interview Insights provide a pathway to transforming raw data into actionable steps. By efficiently tagging messaging opportunities in interview transcripts, organizations can unlock crucial themes that might otherwise remain hidden. When insights are categorized effectively, they pave the way for clearer communication and informed decision-making, enhancing overall organizational effectiveness.
Converting insights into action requires a systematic approach that involves leveraging technology and understanding human contextual cues. Automated tagging not only saves time but also ensures consistency, allowing teams to focus on strategy and implementation. Ultimately, this transformation leads businesses toward better communication and ongoing success, making it an essential component in today's data-driven landscape.