Mitigating Researcher Bias in AI-Assisted Qualitative Analysis
-
Hello Insight
- 10 min read
In the realm of AI-assisted qualitative analysis, understanding user behavior and insights is critical. This section explores various bias reduction strategies that researchers can employ to enhance their findings. Utilizing these strategies can safeguard against subjective interpretations that cloud data analysis. The goal here is to establish a solid foundation, ensuring that results are both reliable and actionable.
First, let's consider three effective strategies: data diversification, iterative feedback, and transparency in analysis. Data diversification involves gathering varied datasets from multiple sources. This practice helps reduce personal biases that may arise from limited perspectives. Next, iterative feedback encourages continual assessment of research findings through collaboration with peers. This approach allows researchers to scrutinize biases entrenched in their interpretations. Lastly, creating transparency in analysis by documenting methodologies and decisions fosters a culture of accountability. Together, these strategies significantly strengthen the integrity of qualitative research in AI contexts.
Introduction: Understanding Bias Reduction Strategies in AI-Assisted Qualitative Analysis
Bias reduction strategies play a crucial role in improving the integrity of AI-assisted qualitative analysis. As researchers increasingly rely on advanced technologies to interpret complex datasets, the potential for inadvertent biases to influence findings becomes more relevant than ever. Understanding these strategies is essential to ensure that AI tools provide objective and reliable insights.
To effectively mitigate researcher bias, it is imperative to employ a multifaceted approach. This includes rigorous training for researchers, employing diverse datasets, and utilizing algorithmic transparency to identify areas of potential bias. By prioritizing such strategies, the field can enhance the reliability of qualitative insights, leading to more informed decision-making and outcomes in research.
Analyze & Evaluate Calls. At Scale.
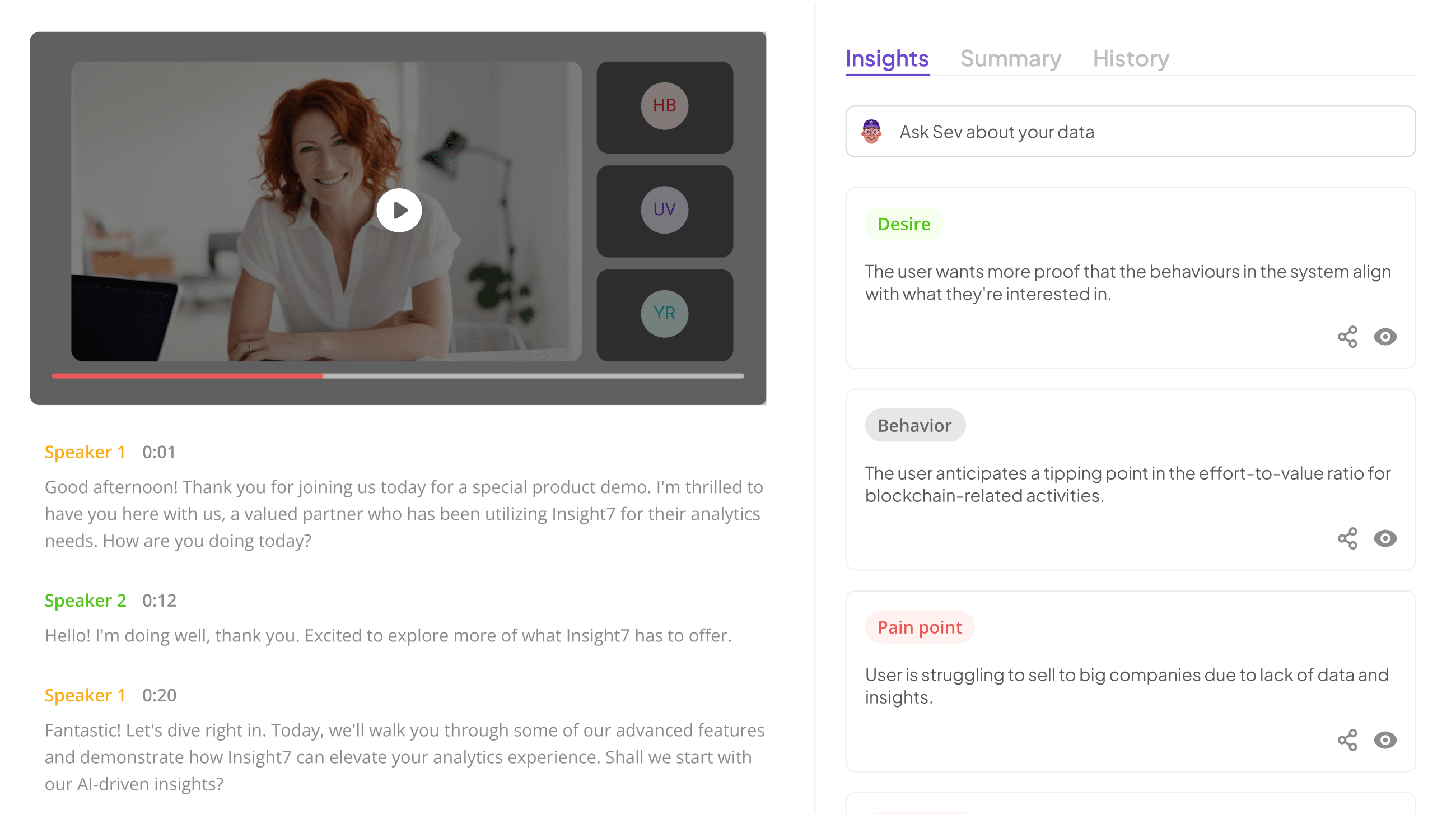
Exploring Bias Reduction Strategies in AI-Driven Research Tools
In the realm of AI-driven research tools, exploring bias reduction strategies becomes essential for maintaining the integrity of qualitative analysis. Researcher bias can seep into data interpretation, leading to skewed findings. By implementing robust tools designed to mitigate bias, researchers contribute to more reliable outcomes. It is crucial to identify and understand the types of biases that can affect AI-assisted analysis.
Several effective strategies can enhance the objectivity of AI tools. First, employing diverse data sources helps to minimize systematic biases that arise from narrow perspectives. Additionally, utilizing calibration techniques enables researchers to refine AI outputs continually, ensuring accuracy. Regularly auditing algorithms for potential bias can offer crucial insights into their functionality and fairness. Lastly, fostering transparency in AI processes encourages collaboration among researchers and stakeholders, promoting a culture of accountability and continuous improvement. Thus, embracing these bias reduction strategies leads to stronger, more trustworthy qualitative research outcomes.
Key Elements of Bias in AI-Assisted Analysis
In AI-assisted analysis, identifying key elements of bias is essential for achieving valid results. Several types of biases can arise during qualitative analysis, including selection bias, confirmation bias, and algorithmic bias. Researchers must be aware of these biases when integrating AI tools into their workflow. Without a strong understanding of these key elements, it becomes easier to overlook how bias can shape interpretations and lead to flawed conclusions.
To mitigate these concerns, researchers should actively implement bias reduction strategies. This includes employing diverse data sources to minimize selection bias or regularly auditing algorithms to address any unintended biases. Additionally, incorporating feedback loops can help continuously refine the AI's responses, ensuring that the insights generated reflect a more comprehensive understanding of the data. By integrating these bias reduction strategies into the analysis process, researchers can enhance the objectivity and reliability of their findings.
Implementing Bias Reduction Strategies: Techniques and Best Practices
To implement effective bias reduction strategies, one must first recognize the various biases that often influence qualitative research. Training researchers to be aware of their own cognitive biases is vital to creating more objective analyses. This involves fostering an environment where critical thinking is encouraged, along with receiving constructive feedback from peers. Researchers can also incorporate strategies such as using diverse data sources and collaborating with multidisciplinary teams, which helps to minimize the risk of personal bias affecting the research outcomes.
Another effective technique is the use of systematic coding frameworks. This helps streamline data analysis, ensuring a standardized approach is applied across different datasets. Regularly revisiting and validating coding categories further aids in maintaining objectivity. Additionally, employing AI-assisted tools can facilitate consistency, as algorithms might reduce human error during analysis. By integrating these best practices, researchers can significantly enhance the credibility and reliability of their qualitative insights.
Extract insights from interviews, calls, surveys and reviews for insights in minutes
Tools and Technologies for Bias Reduction in Qualitative Research
In the sphere of qualitative research, various tools and technologies are at the forefront of bias reduction strategies. Understanding these resources is essential for researchers aiming to maintain objectivity in their analysis. Software solutions such as QDA Miner, NVivo, and ATLAS.ti provide functionalities that support systematic data coding and analysis, which can significantly diminish individual biases. These tools not only enhance the rigor of qualitative studies but also facilitate collaborative teamwork, where diverse perspectives can counteract potential biases.
To capitalize on the full potential of these tools, researchers should engage in proper training and integration. Automating certain aspects of data analysis helps to minimize subjective interpretation, promoting a more balanced representation of findings. Furthermore, using data visualization features within these tools can illuminate trends and insights that may often be overlooked, thereby fostering a holistic understanding of the research topic. Embracing these technologies ultimately paves the way for more accurate and credible qualitative research outcomes.
Insight7: Leading the Way in Bias Mitigation
Insight7 has emerged as a pioneering force in mitigating bias within qualitative research. By focusing on effective bias reduction strategies, this approach enhances the reliability of insights derived from AI-assisted analysis. The key to successful bias mitigation lies in recognizing and addressing the subtle biases that can creep into qualitative assessments, thereby influencing outcomes.
๐ฌ Questions about Mitigating Researcher Bias in AI-Assisted Qualitative Analysis?
Our team typically responds within minutes
To effectively reduce bias, it is essential to employ strategies that include training researchers on awareness of their biases, standardizing data collection methods, and using diverse data sets. For instance, equipping researchers with tools that promote reflective practices can help them identify personal biases inherent in their analysis. Additionally, collaborative practices that involve multi-disciplinary teams can foster varied perspectives, ultimately leading to richer and more balanced insights. Embracing these strategies not only elevates the quality of research but also empowers organizations to make informed, data-driven decisions.
Other Essential Tools for Ensuring Objectivity in Analysis
Ensuring objectivity in qualitative analysis necessitates the use of various tools that enhance the integrity of the research process. Among these, software platforms like QDA Miner, NVivo, and ATLAS.ti play vital roles. These tools not only facilitate the organization of qualitative data but also support rigorous analysis, allowing researchers to identify patterns and derive insights more systematically.
QDA Miner aids in coding text data, which helps in organizing thoughts and minimizing personal biases. NVivo enhances the capacity to analyze unstructured data by offering advanced visualization options, enabling researchers to see connections and trends clearly. ATLAS.ti complements this by fostering collaboration and allowing multiple users to interact with data, which reduces the risk of subjective interpretations. Using these essential tools and combining them with bias reduction strategies significantly enhances objectivity in the analysis and ultimately leads to more reliable conclusions.
QDA Miner
QDA Miner serves as a powerful tool for researchers aiming to navigate qualitative data efficiently and effectively. By providing a structured framework to analyze complex information, it facilitates the identification of patterns and themes within datasets. One of the standout features of QDA Miner is its ability to generate visualizations that summarize insights, directly aiding in bias reduction strategies during analysis.
Within the platform, users can create projects where they compile and analyze various qualitative sources. This organization aids in mitigating researcher bias by promoting consistent evaluation across different pieces of data. Furthermore, the software allows for the integration of transcription and direct file imports, making data management intuitive. Its analytical matrix feature empowers users to articulate specific research questions, leading to focused assessments and clearer conclusions, which further solidifies the quality of analysis undertaken.
In essence, QDA Miner is more than just a statistical tool; it is designed to enhance the rigor of qualitative research. With its robust features, it stands as an essential ally in implementing effective bias reduction strategies. This dynamic tool emphasizes the importance of systematic approaches, leading to more reliable and valid research outcomes.
NVivo
The use of advanced analytical software is paramount when addressing bias in qualitative research. NVivo, a powerful tool designed for qualitative data analysis, can facilitate collaborative projects while reducing researcher bias. By organizing and interpreting varied data sources effectively, this software aids researchers in uncovering insights that may otherwise remain hidden. Its intuitive interface allows users to import data from various platforms, such as Google Drive, simplifying the workflow.
One key approach within NVivo is its project-based framework, where researchers can upload transcribed interviews or focus group discussions. This enables users to systematically analyze responses and discern patterns. Furthermore, NVivoโs query and visualization features help surface trends across multiple data streams. These elements collectively contribute to bias reduction strategies, ensuring that findings are based on data-driven insights rather than personal interpretations. Ultimately, employing NVivo enhances the credibility of qualitative research outcomes.
ATLAS.ti
When conducting qualitative analysis, utilizing advanced tools can facilitate effective bias reduction strategies. One such powerful platform provides a structured environment for organizing and analyzing qualitative data. Users can create projects, allowing for rapid aggregation of various materials, including transcripts, quotes, and testimonials. This seamless integration helps researchers view connections and patterns, promoting objectivity throughout the analysis process.
To further enhance the reliability of insights, researchers can utilize functionalities designed specifically for identifying and addressing biases. For example, automated coding features can categorize data efficiently, reducing the chance of personal biases influencing thematic discovery. Additionally, visual analytics capabilities enable researchers to see the relationships between different data points, further supporting an unbiased interpretation of findings. By implementing such strategies, researchers can foster a more genuine understanding of their data, ensuring that insights gleaned are based on merit and not influenced by personal biases.
Conclusion: The Impact of Bias Reduction Strategies on Qualitative Research
The implementation of Bias Reduction Strategies significantly elevates the quality of qualitative research. By actively identifying and minimizing the influence of researcher biases, these strategies foster an environment ripe for objective insights. Researchers equipped with such strategies can better navigate the complexities of qualitative data, leading to more reliable and actionable findings.
Moreover, these approaches encourage a more comprehensive understanding of diverse perspectives within the data. When biases are reduced, the richness of participant experiences comes to the forefront, ultimately enhancing the credibility and applicability of the research. Embracing Bias Reduction Strategies is essential for any qualitative researcher aiming to achieve authenticity and trustworthiness in their work.
To effectively address biases in AI-assisted qualitative analysis, researchers must adopt comprehensive bias reduction strategies. These strategies aim to identify and mitigate cognitive biases that could skew the interpretation of qualitative data. Being aware of personal biases enhances the reliability of the research findings, ensuring that diverse perspectives are accurately represented.
Key strategies include triangulation, which involves cross-verifying data through multiple sources or methods. This approach supports a well-rounded analysis by capturing various viewpoints. Additionally, blind coding can reduce the influence of preconceptions; by anonymizing data during the analysis, researchers maintain objectivity. Frequent peer reviews and team discussions are also crucial to challenge potential biases and foster transparency. Implementing these strategies can significantly bolster the integrity of research outcomes, ultimately leading to more valid and actionable insights.
๐ฌ Questions about Mitigating Researcher Bias in AI-Assisted Qualitative Analysis?
Our team typically responds within minutes