In an era where coaching effectiveness can define organizational growth, the optimization of coaching cadence becomes paramount. Coaching Cadence Optimization aims to fine-tune how, when, and why coaches interact with their teams, ensuring impactful engagements. This section introduces the innovative role of Large Language Models (LLMs) in this optimization process, revealing how these advanced systems can identify and promote high-impact coaching techniques.
Harnessing LLMs enables organizations to analyze coaching interactions, providing deeper insights into communication patterns and effectiveness. By utilizing AI-driven data, businesses can refine their coaching strategies, ultimately fostering more meaningful connections between coaches and their teams. As we delve deeper into this topic, we'll explore the transformative potential of LLMs in shaping successful coaching practices.
Analyze qualitative data. At Scale.
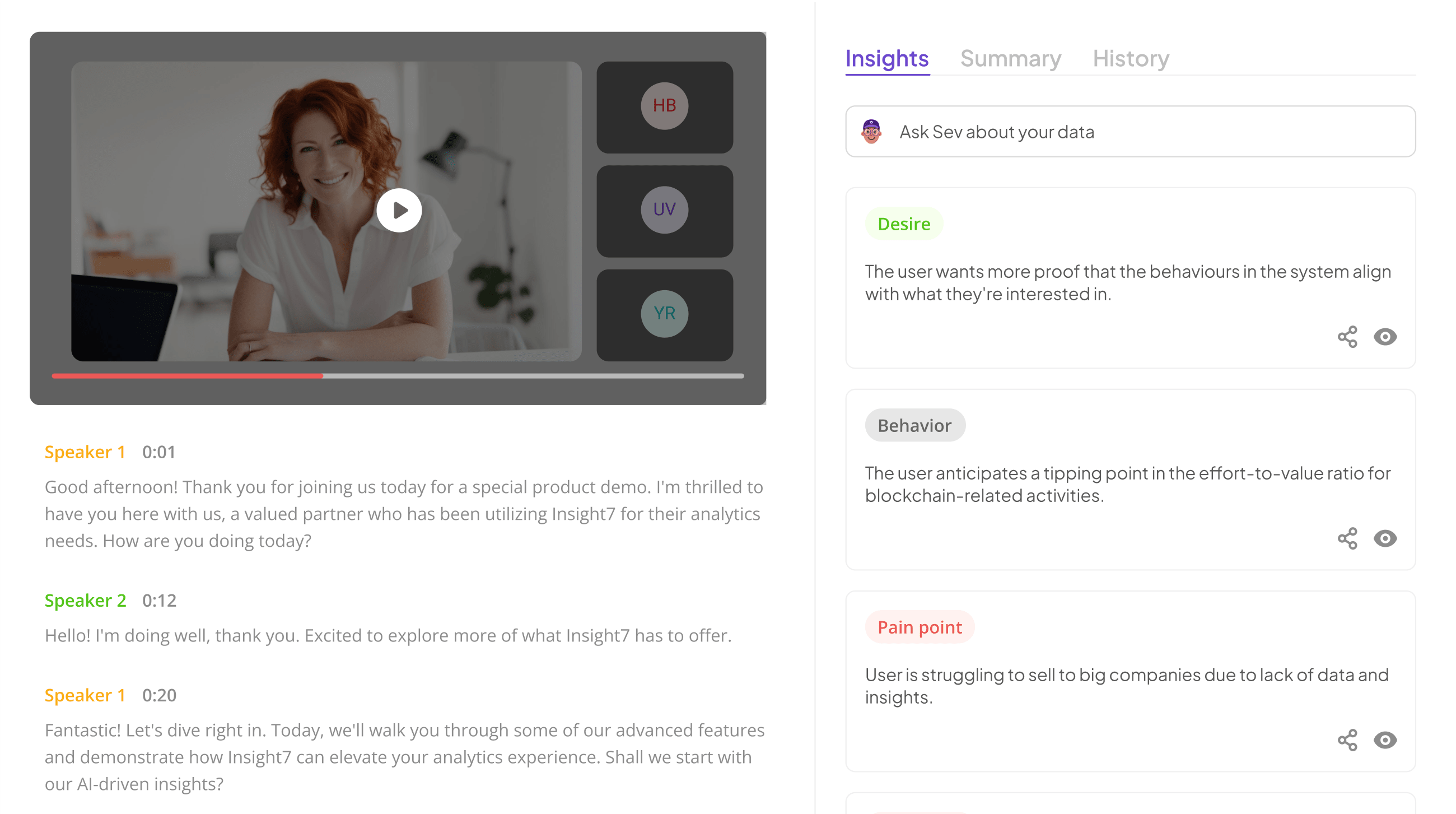
How LLMs Revolutionize Coaching Cadence Optimization
Large Language Models (LLMs) are transforming how organizations approach Coaching Cadence Optimization. By analyzing vast amounts of interaction data, LLMs can identify patterns that correlate with high-performance outcomes. This capability enables organizations to tailor their coaching strategies specifically to the needs of individual representatives. Instead of a one-size-fits-all approach, LLMs help create personalized insights that enhance coaching effectiveness.
Moreover, the continuous learning nature of LLMs allows them to adapt over time, improving their predictive accuracy in identifying impactful coaching moments. This dynamic capability ensures that coaching techniques evolve alongside the changing needs of teams and markets. By integrating LLMs into coaching frameworks, organizations can not only enhance performance but also foster a culture of continuous improvement, driving better results through optimized interactions. This revolution in Coaching Cadence Optimization signifies a move toward data-driven, individualized coaching that maximizes employee potential.
Understanding Large Language Models (LLMs)
Large Language Models (LLMs) have emerged as transformative tools in various fields, including coaching cadence optimization. These advanced algorithms can process vast amounts of textual data, offering unique insights that can drastically improve coaching strategies. By analyzing communication patterns and interactions, LLMs can identify high-impact coaching cadences that resonate with individuals or teams.
Understanding how LLMs function is crucial for leveraging their potential. At their core, LLMs utilize vast datasets to learn language patterns, making them adept at predicting user intent and responding contextually. This capability allows coaches to tailor their strategies based on precise linguistic cues and feedback, thus optimizing coaching effectiveness. Engaging with LLMs not only enhances the coaching experience but also encourages a more interactive dialogue between coaches and clients, ultimately driving improved outcomes in performance and satisfaction.
Step 1: The Basics of LLMs and Their Capabilities
Large Language Models (LLMs) have transformed the way we approach Coaching Cadence Optimization. These powerful tools leverage vast amounts of data to understand language nuances, allowing them to analyze conversations and identify effective coaching techniques. By comprehending context, tone, and phrasing, LLMs can pinpoint coaching strategies that resonate well with individuals. This adaptability makes them invaluable in optimizing interaction cadences in a variety of coaching settings.
Understanding how LLMs operate involves recognizing their ability to learn patterns from text data. They process large datasets to refine their predictive capabilities and tailor responses effectively. This allows organizations to assess coaching interactions in-depth, extracting meaningful insights that can enhance overall performance. The ability to analyze conversations at scale opens avenues for coaches to implement high-impact strategies, ensuring they engage with their clients effectively. Ultimately, LLMs not only streamline the coaching process but also elevate the quality of support coaches can provide.
Step 2: How LLMs Learn and Predict High-Impact Cadences
Large Language Models (LLMs) learn and predict high-impact coaching cadences through sophisticated algorithms that analyze vast amounts of data. By processing qualitative and quantitative inputs from coaching interactions, LLMs can identify patterns that correlate with successful outcomes. This ability allows organizations to refine their coaching cadence optimization by pinpointing the most effective strategies that drive engagement and performance.
The predictive capabilities of LLMs also stem from their continuous learning processes. As they gather more data, these models enhance their understanding of what works best in various coaching contexts. This not only informs adjustments in training methodologies but also helps in customizing approaches based on specific team dynamics. In essence, LLMs act as analytical allies, providing insights that empower coaches to fine-tune their techniques, ultimately enhancing the effectiveness of their outreach and support efforts.
The Role of AI in Identifying Effective Coaching Patterns
AI plays a transformative role in identifying effective coaching patterns, greatly enhancing coaching cadence optimization. By analyzing vast datasets from coaching interactions, AI can discern subtle patterns and trends that are often missed by human observation. This analysis helps coaches establish what coaching methods yield the greatest impact on performance outcomes. Through continuous evaluation, AI not only identifies these successful cadences but also adapts to feedback, refining its predictive capabilities to focus on the most effective strategies.
Moreover, the ability of AI to process information swiftly means that coaches can receive real-time insights into their effectiveness. These insights allow them to tailor their approach according to the unique needs of their team or individual. With AI as a supportive tool, coaches can embrace data-driven decision-making and foster an environment conducive to growth and performance improvement. In this way, AI not only supports coaching but actively encourages a culture of continuous learning and optimization.
Step 1: Analyzing Coaching Interactions
Analyzing coaching interactions is a crucial first step in optimizing coaching cadences. This analysis involves systematically reviewing past coaching sessions to identify patterns and areas for improvement. By examining interactions, coaches can better understand client behaviors, preferences, and specific challenges. This understanding allows for tailoring future coaching approaches to meet individual needs more effectively.
To effectively analyze coaching interactions, focus on three key aspects: transcript review, trend identification, and feedback synthesis. First, reviewing transcripts helps uncover common themes and recurring pain points among clients. Next, identifying trends across multiple sessions provides insights into how successful interactions can be replicated or refined. Finally, synthesizing feedback from clients ensures that the coaching approach remains responsive to evolving needs. Through diligent analysis, coaching cadences can be optimized to drive maximum impact, leading to more successful outcomes in coaching relationships.
Step 2: Extracting Patterns that Drive Success
In this step, we focus on extracting patterns that drive success in coaching cadences. Understanding how to uncover these patterns is essential for effective coaching strategies. Begin by closely examining interactions over time; this will allow you to build a comprehensive overview of recurring themes and behaviors. Identifying these commonalities can provide insights into what truly resonates with learners and which methods yield the best results.
To effectively extract these patterns, consider the following key points:
- Data Compilation: Gather data from coaching sessions and interactions to form a solid base for analysis.
- Theme Identification: Use analysis tools to spotlight significant themes and insights that emerge from the data, informing future sessions.
- Trend Analysis: Observe trends over time to determine which cadences have facilitated success and which ones may require adjustments.
- Performance Metrics: Establish clear performance metrics to evaluate the effectiveness of different coaching cadences.
By focusing on these areas, you can optimize coaching cadence and enhance overall outcomes.
Extract insights from interviews, calls, surveys and reviews for insights in minutes
Top Tools for Coaching Cadence Optimization Using LLMs
To enhance Coaching Cadence Optimization, various tools harness the power of large language models (LLMs). These tools facilitate the identification and analysis of effective coaching strategies, transforming the way organizations engage with their teams. By harnessing AI capabilities, these solutions streamline data processing, allowing users to focus on deriving actionable insights.
Key tools include Adobe Sensei, which offers advanced AI-driven insights to optimize training sessions. IBM Watson presents robust machine learning capabilities, analyzing coaching patterns for better performance outcomes. Additionally, Microsoft Azure AI provides versatile and scalable solutions, ensuring organizations can adapt their coaching methodologies swiftly. Lastly, Google Cloud AI employs a data-driven approach to identify high-impact coaching interactions. Together, these tools empower organizations to pinpoint effective cadences, ultimately driving better coaching results.
Insight7: Leading the Way in Cadence Discovery
In the realm of Coaching Cadence Optimization, Insight7 stands out as a pioneer in cadence discovery. This innovative platform is designed to harness the power of large language models (LLMs), enabling organizations to uncover high-impact coaching patterns efficiently. As businesses amass vast amounts of customer data, traditional analysis methods often fall short. Insight7 bridges this gap by providing intuitive tools for scaling data analysis, streamlining the process of translating insights into tangible coaching strategies.
The core of Insight7 lies in its ability to identify and optimize coaching cadences through in-depth analysis of customer interactions. By utilizing AI-driven insights, organizations can pinpoint successful coaching methodologies tailored to their unique needs. This leads to more effective communication strategies, improved response rates, and higher customer satisfaction. Overall, Insight7 is not just leading in cadence discovery; it is reshaping how organizations can leverage coaching for meaningful growth in dynamic environments.
Other Tools to Consider
When exploring Coaching Cadence Optimization, various tools can enhance your experience and provide deeper insights beyond just LLMs. Adobe Sensei offers enhanced AI insights that automate data analysis, allowing you to visualize coaching patterns effortlessly. With its powerful image and video analysis capabilities, it can uncover trends in training sessions, revealing what works best for your team.
IBM Watson takes a different approach with its robust machine learning capabilities, providing detailed natural language processing functions. This allows organizations to analyze coaching dialogues comprehensively and identify high-impact coaching techniques. Similarly, Microsoft Azure AI delivers versatile and scalable solutions that can adapt to your evolving needs, ensuring that your coaching strategies remain effective over time.
Google Cloud AI stands out with its data-driven features, assisting in making informed decisions based on robust data analysis. Each of these tools can play a critical role in developing a powerful coaching cadence optimization strategy, empowering organizations to get the most out of their coaching efforts.
Adobe Sensei: Enhanced AI Insights
In the realm of Coaching Cadence Optimization, Adobe Sensei introduces a transformative approach to leveraging AI insights effectively. This advanced AI technology processes vast amounts of data, identifying unique patterns and trends that can drive coaching success. By using sophisticated algorithms, it enables organizations to tailor their coaching strategies based on insightful analyses of previous interactions. The result is a more personalized coaching experience that aligns with individual needs and maximizes impact.
The insights gleaned from Adobe Sensei empower teams to refine their coaching cadences systematically. Coaches can identify what works best, balancing interaction frequency with content relevance. The enhanced data-driven decisions lead to optimized coaching practices. Whether through direct feedback analysis or behavior prediction, these insights can significantly elevate an organizationโs coaching framework, ultimately fostering a culture of continuous improvement and effectiveness. Embracing these advanced capabilities helps organizations harness the power of data for better coaching methodologies.
IBM Watson: Robust Machine Learning Capabilities
IBM Watson offers powerful machine learning capabilities that can significantly enhance coaching cadence optimization. With its advanced algorithms, it analyzes coaching interactions to uncover patterns and insights that might otherwise go unnoticed. By processing large volumes of data, the system can identify which coaching strategies lead to the most impactful outcomes, thereby streamlining decision-making for leaders and coaches.
Additionally, Watson's user-friendly interface ensures that it can be accessed by anyone within an organization, regardless of technical expertise. This democratization of data allows teams to collaboratively harness insights from coaching sessions. Importantly, the machine learning model continuously learns from new data, improving its accuracy over time. Consequently, organizations can fine-tune their coaching methods based on real-time feedback, leading to a more effective and customized approach to coaching cadence optimization.
Microsoft Azure AI: Versatile and Scalable Solutions
Microsoft Azure AI provides a versatile and scalable solution for optimizing coaching cadences in various environments. With its advanced algorithms and machine learning capabilities, users can harness the power of AI to identify successful coaching patterns. By integrating this technology, organizations can significantly enhance their coaching methodologies, ensuring that they are not only effective but also efficient.
The tool offers robust features that analyze vast amounts of data to extract meaningful insights about coaching interactions. This analysis allows for the development of tailored coaching strategies that align with specific organizational goals. By focusing on Coaching Cadence Optimization, users can leverage these insights to implement consistent coaching methods that lead to measurable improvements in performance and engagement. This combination of versatility and scalability empowers businesses to adapt their coaching practices to ever-changing needs while maintaining high standards of effectiveness.
Google Cloud AI: Data-Driven Approach to Coaching
A data-driven approach to coaching focuses on utilizing advanced analytics to enhance coaching effectiveness. With tools powered by AI and machine learning, organizations can digest and analyze substantial amounts of data from coaching interactions. This approach identifies high-impact coaching cadences, allowing for targeted strategies that elevate performance. Effective coaching relies on insights gleaned from various data sources, including call transcripts and customer feedback, ensuring that coaches are equipped to foster development.
To optimize coaching cadence, organizations should follow several key steps. First, accurately analyze coaching interactions to determine what works and what doesnโt. Next, extract actionable insights that highlight patterns driving success across various teams. This data-centric framework empowers coaches to adopt more consultative techniques, moving beyond mere order-taking to meaningful engagement with clients. Utilizing such methodologies ultimately results in effective coaching that aligns closely with evolving market demands and customer needs.
Conclusion: Embracing LLMs for Optimal Coaching Cadence Optimization
The integration of Large Language Models (LLMs) into coaching practices represents a transformative leap for optimizing coaching cadence. By embracing LLMs, organizations can gain deeper insights into coaching interactions, uncovering patterns that lead to improved performance. This innovative approach enables teams to tailor their training schedules based on data-driven insights, ultimately enhancing their effectiveness.
As we move forward, it is crucial to adapt to these technological advancements. By identifying high-impact coaching cadences through LLMs, organizations can foster a culture of continuous improvement. Embracing these tools not only optimizes training processes but also drives significant results in achieving coaching goals. The future of coaching cadence optimization lies in this strategic embrace of LLMs.