QA-Driven Hypothesis Validation in CX marks a significant evolution in customer experience strategies. With the rapid inflow of feedback and data, organizations face challenges in understanding their customers' true needs. By effectively utilizing quality assurance review data, businesses can validate hypotheses about customer behavior and preferences. This methodology offers a structured approach to transforming qualitative insights into actionable strategies.
As organizations embark on this journey, they can align their CX initiatives more closely with actual customer experiences. By focusing on QA-driven validation, teams enable a culture of continuous improvement, ensuring that their strategies are both data-informed and customer-centric. This section sets the stage for exploring how to harness QA data to drive meaningful changes in customer experience.
Analyze qualitative data. At Scale.
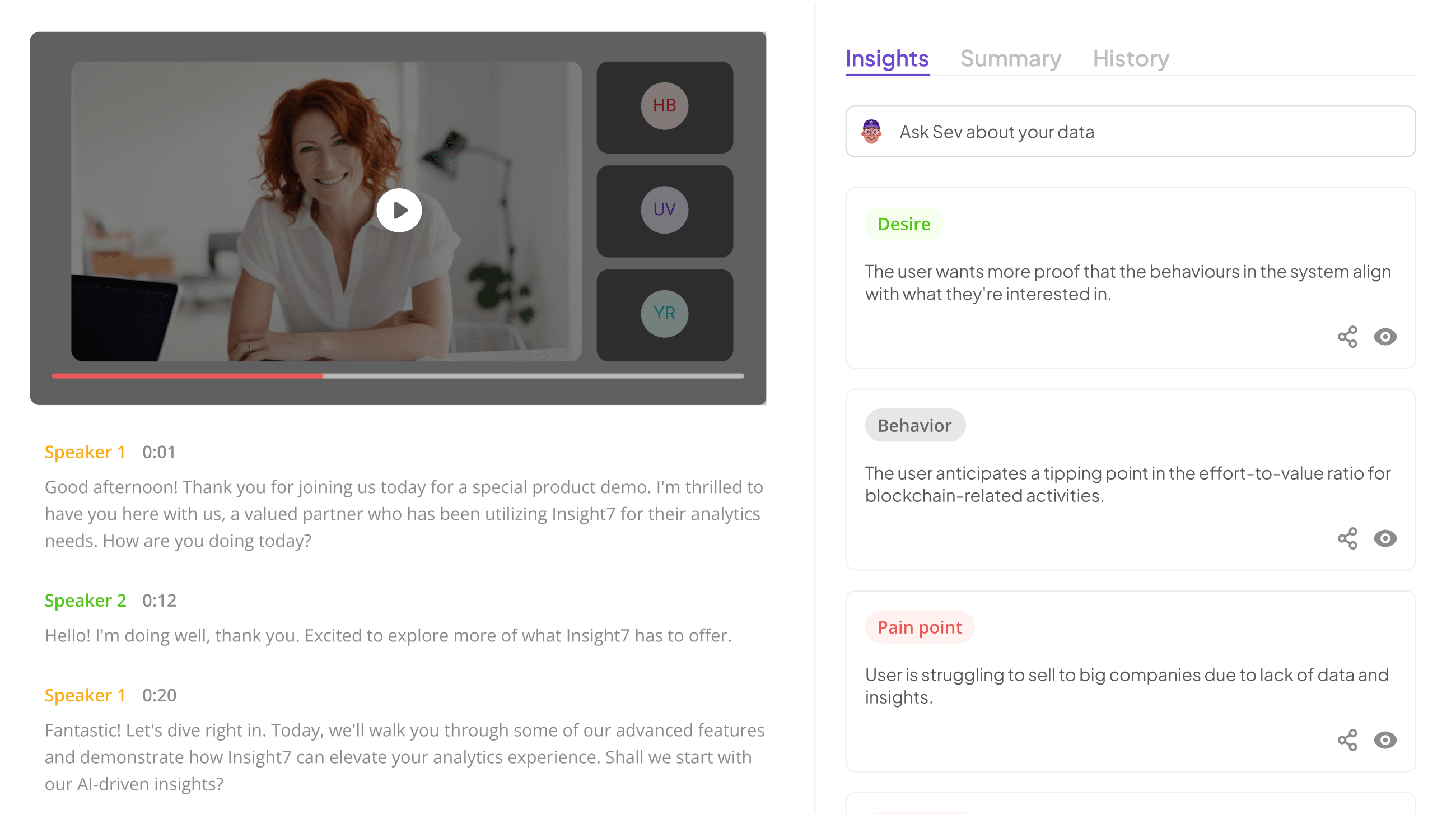
The Importance of QA-Driven Hypothesis Validation in CX
QA-Driven Hypothesis Validation plays a pivotal role in enhancing customer experience (CX) by ensuring that insights derived from customer interactions are reliable and actionable. This process begins with systematic evaluations of customer calls and interactions, where QA metrics help identify trends and areas for improvement. By analyzing data from these reviews, organizations can validate assumptions about customer behavior, preferences, and pain points. This informed approach enhances decision-making processes and aids in aligning services with customer expectations.
Moreover, the importance of this validation method lies in its ability to foster trust and improve service quality. It allows organizations to quantify customer feedback effectively, ensuring that responses to changes within the business are based on solid data rather than speculation. Ultimately, QA-Driven Hypothesis Validation not only leads to better business strategies but also enhances customer satisfaction and loyalty, creating a more robust market presence.
Understanding the Role of QA Review Data
QA Review Data plays a crucial role in validating CX hypotheses, providing a foundation for understanding customer interactions. This data serves as a lens through which organizations can assess the quality of customer experiences, ensuring that they align with established standards. By meticulously evaluating calls and customer feedback, companies can identify patterns and pinpoint areas for improvement. The insights gathered through QA Review Data not only enhance compliance and performance metrics but also guide strategic decision-making processes.
Utilizing QA-Driven Hypothesis Validation enables organizations to transform raw data into actionable insights. This validation process allows teams to refine their hypotheses based on tangible evidence from customer interactions. Moreover, QA Review Data brings clarity to what influences customer satisfaction and loyalty, ensuring that businesses remain agile in their approach. As teams learn to interpret this data effectively, they can implement improvements that lead to enhanced customer experiences and sustainable growth.
Key Benefits of Leveraging QA Data
Using QA data effectively can yield substantial benefits for organizations looking to validate their customer experience (CX) hypotheses. One significant advantage is enhanced decision-making, as QA-driven insights allow businesses to identify trends and patterns in customer feedback. By analyzing this data, organizations can pinpoint strengths and weaknesses in their services, ensuring that future strategies are informed by real customer experiences.
Moreover, QA data fosters a culture of continuous improvement. When teams rely on factual insights to validate hypotheses, they become more agile in adapting their approaches. This results in a stronger alignment between products or services and customer expectations. Ultimately, leveraging QA data supports a data-informed environment where decisions are based on a solid evidence foundation, contributing to overall customer satisfaction and loyalty in the long term.
Extract insights from interviews, calls, surveys and reviews for insights in minutes
How to Conduct QA-Driven Hypothesis Validation
To conduct QA-Driven Hypothesis Validation effectively, the first step involves collecting and analyzing relevant QA review data. Begin by gathering all available QA review materials, including call transcripts and qualitative feedback. This data serves as the foundation for evaluating your customer experience (CX) hypotheses. Utilize an organized framework to evaluate call quality, ensuring that various criteria—such as compliance, understanding customer issues, and effective problem resolution—are meticulously reviewed against the transcripts.
Next, identify patterns and trends within the analyzed data. Look for recurring themes or insights that can confirm or challenge your initial hypotheses. By categorizing these insights, you can pinpoint areas that warrant further investigation or adjustment in CX strategy. Employ visual tools or charts to highlight significant findings. Through this structured approach, QA-Driven Hypothesis Validation can provide actionable insights, ultimately enhancing customer engagement and satisfaction.
Step 1: Collecting and Analyzing QA Review Data
To validate CX hypotheses effectively, the first step involves collecting and analyzing QA review data. This process begins by defining clear criteria for evaluation, focusing on key metrics such as compliance and customer sentiment. By establishing a structured framework, you can categorize and score different aspects of customer interactions. This will enable you to identify which areas align with your CX objectives while also pinpointing opportunities for improvement.
Once data collection is underway, it is crucial to analyze the findings comprehensively. Look for trends across multiple reviews to uncover recurring themes or issues. This analysis can highlight strengths and weaknesses in the customer experience. With this information, you will be equipped to formulate informed hypotheses that drive your next steps. The goal should be creating a feedback loop, where insights guide actions, ensuring continuous improvement in your CX strategy through a disciplined approach centered around QA-Driven Hypothesis Validation.
Step 2: Identifying Patterns and Trends
Identifying patterns and trends in QA review data is a crucial step in validating customer experience (CX) hypotheses. This process allows teams to pinpoint recurring themes and issues that may directly affect customer satisfaction. By aggregating relevant data, analysts can reveal insights that are often overlooked, helping to deepen their understanding of customer sentiments and preferences. It is essential to categorize the data into manageable segments, enabling a clearer interpretation of customer feedback.
To effectively identify these patterns, consider the following steps:
Data Segmentation: Organize the QA review data by key themes or metrics, such as customer pain points and satisfaction levels. This segmentation facilitates a more focused analysis.
Trends Analysis: Review the segmented data over time to highlight changes in customer sentiment. Observing fluctuations can uncover seasonal trends or shifts in customer behavior.
Create Visual Summaries: Utilize visuals like charts or graphs to present your findings. These representations can quickly convey complex insights to stakeholders.
By following these practices, organizations can ensure that QA-driven hypothesis validation results in actionable insights, ultimately enhancing their CX strategy.
Tools for Effective QA-Driven Hypothesis Validation
Utilizing the right tools is crucial for effective QA-Driven Hypothesis Validation. To begin, organizations should focus on intuitive software solutions that can streamline the analysis of QA review data. For instance, Insight7 provides comprehensive capabilities for recording, transcribing, and evaluating various customer interactions. With its user-friendly interface, even those without extensive research training can extract valuable insights efficiently.
Moreover, tools like Qualaroo and Medallia enable businesses to gather real-time customer feedback that can be cross-referenced with quality assurance metrics. This interplay helps identify significant gaps in customer experience. Usabilla further enhances the process by allowing companies to gather contextual feedback directly from users, enabling a deeper understanding of their needs. By integrating these tools into their QA-Driven Hypothesis Validation strategy, organizations can create a robust framework for continuous improvement and customer satisfaction.
Insight7
QA-Driven Hypothesis Validation involves systematically evaluating customer experience hypotheses using quality assurance (QA) review data. Many organizations struggle to efficiently analyze vast amounts of customer feedback due to traditional manual methods that hinder timely insights. Instead, QA-driven approaches enable a focused understanding of customer sentiments and behaviors, turning raw data into actionable strategies.
In this section, we will explore effective steps for implementing QA-Driven Hypothesis Validation. First, gather and analyze relevant QA review data to establish a solid foundation. Next, identify patterns and trends emerging from your analysis, which can reveal critical customer insights. Utilizing these methods helps organizations distill complex data into clearer narratives, leading to improved customer experience outcomes. By embracing a QA-driven mindset, businesses can accelerate decision-making and maintain a competitive edge in their industry.
Qualaroo
Using qualitative insights is vital for enhancing customer experience (CX), and one effective way to gather such insights is through the use of specific tools. In this context, employing a platform designed for direct customer engagement can facilitate the collection of meaningful feedback, which is essential for QA-driven hypothesis validation. By targeting the right audience and asking pertinent questions, teams can unveil crucial insights about customer needs and preferences.
Through structured feedback forms and targeted surveys, teams can systematically analyze customer interactions to derive patterns and sentiments. This process not only supports improving service frameworks but also aligns employee performance with customer expectations. Ultimately, a well-executed QA-driven hypothesis validation leads to actionable insights that drive overall business growth and customer satisfaction. When organizations prioritize understanding their customers, they position themselves for lasting success.
Medallia
To effectively utilize QA review data, the role of platforms like Medallia becomes paramount. This tool enables teams to collect and analyze customer feedback, facilitating deeper insights into customer experiences. By focusing on quality assurance methodologies, organizations can validate CX hypotheses and refine their service strategies accordingly. Utilizing actionable insights from this platform allows businesses to identify areas requiring attention and enhancement.
In this process, QA-Driven Hypothesis Validation becomes essential. It guides companies in interpreting feedback from customers, ensuring that findings are aligned with the initial proposed hypotheses. By systematically applying data obtained from such tools, organizations can not only measure the efficacy of their CX strategies but can also iterate on them based on customer feedback. Ultimately, leveraging platforms like Medallia empowers businesses to cultivate a customer-centric approach that enhances overall satisfaction and drives growth.
Usabilla
Utilizing feedback tools can significantly enhance the customer experience (CX) by providing vital insights. These tools enable businesses to gather user opinions in real-time, allowing for a continuous cycle of improvement. By integrating such platforms into the QA-driven hypothesis validation process, companies can bolster their understanding of customer sentiments and needs.
Feedback tools facilitate the collection of qualitative and quantitative data, essential for identifying customer pain points. This process is crucial for validating hypotheses about customer behavior. For example, analyzing responses can reveal patterns that highlight areas needing attention or innovation. As organizations assess these insights, they can make informed decisions, ensuring that their CX strategies are not only effective but also aligned with customer expectations. Such strategic use of feedback tools fosters a culture of continuous learning and adaptation, ultimately enhancing overall customer satisfaction.
Conclusion: Maximizing CX Success through QA-Driven Hypothesis Validation
In today’s competitive landscape, maximizing customer experience (CX) success relies heavily on QA-Driven Hypothesis Validation. By integrating quality assurance processes into hypothesis testing, organizations can derive actionable insights from customer interactions. This approach not only enhances the validity of the hypotheses but also optimizes overall service delivery. It empowers teams to refine their strategies based on concrete data rather than assumptions, leading to more effective decision-making.
Implementing QA-Driven Hypothesis Validation encourages a culture of continuous improvement. With every analysis, organizations can identify weaknesses and strengths in their CX initiatives, fostering a proactive rather than a reactive response to customer feedback. Ultimately, this method ensures that companies remain aligned with customer needs, paving the way for sustainable success in enhancing customer satisfaction and loyalty.
Analyze qualitative data. At Scale.
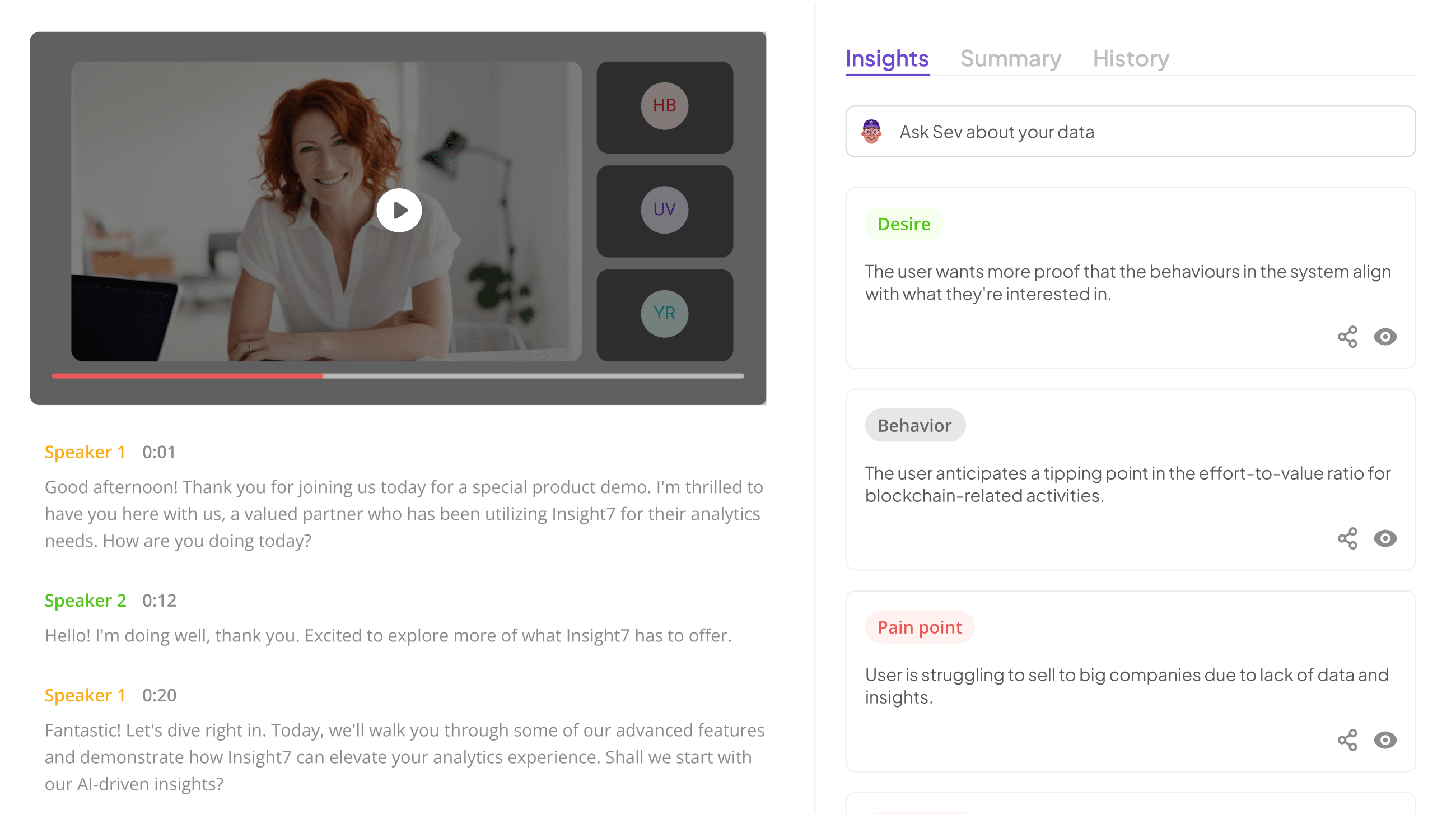