In today's competitive landscape, businesses face the constant threat of customer churn, which can severely impact growth and profitability. Addressing this issue effectively requires a nuanced understanding of customer emotions and experiences, making the introduction of sentiment-based churn prediction both timely and essential. By leveraging insights from sentiment analysis, companies can identify customer dissatisfaction early, allowing for timely interventions that can retain valuable clients.
Sentiment-based churn prediction translates customer feedback into actionable data that helps organizations understand their audience better. This section will delve into the significance of sentiment analysis in facilitating successful churn predictions. Not only does it uncover the underlying emotions of customers, but it also enables businesses to craft strategies that enhance customer loyalty and satisfaction. Understanding this connection between sentiment and churn is crucial for organizations aiming to thrive in an increasingly dynamic market.
Analyze & Evaluate Calls. At Scale.
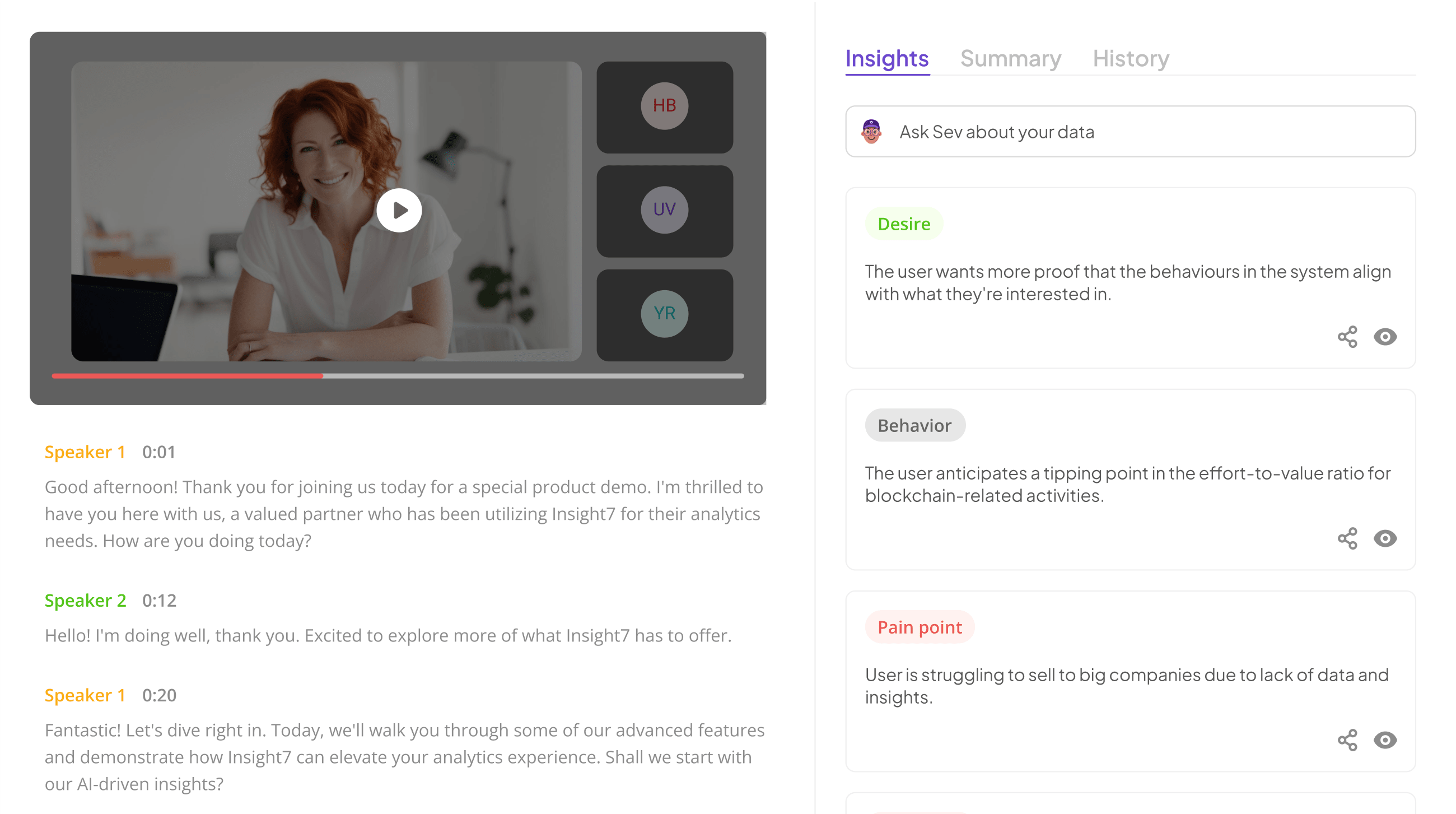
Understanding Sentiment-based Churn Prediction
Understanding Sentiment-based Churn Prediction involves grasping how customer emotions can shape business outcomes. Organizations utilize sentiment analysis to assess customer feedback, identifying feelings such as satisfaction or discontent. This analysis serves as a vital indicator of potential churn, enabling proactive interventions.
Sentiment-based Churn Prediction leverages customer insights to highlight at-risk clients. By examining patterns in customer sentiments, businesses can anticipate dissatisfaction before it escalates. This predictive power allows them to address concerns immediately, enhancing retention efforts. For instance, negative comments on products can pinpoint areas requiring improvement, empowering teams to take corrective actions swiftly.
Understanding these dynamics fosters a more responsive customer experience, ultimately leading to better loyalty and lower attrition rates. Integrating sentiment data into broader analytical frameworks can significantly enhance the effectiveness of churn prevention strategies, making it essential for businesses aiming to thrive in competitive markets.
What is Sentiment Analysis?
Sentiment analysis is the process of assessing the emotional tone behind a series of words. It helps in understanding customer opinions as expressed through various means such as reviews, social media posts, and surveys. By using advanced algorithms, sentiment analysis can categorize expressions as positive, negative, or neutral, providing valuable insights into customer attitudes and behaviors.
Understanding sentiment analysis is crucial for businesses aiming to predict churn. Customers typically express their feelings about a product or service through their feedback. By analyzing these sentiments, companies can identify patterns indicating dissatisfaction or potential churn. Through this process, businesses can proactively address issues, improving customer retention. Ultimately, sentiment-based churn prediction offers an effective way to gauge and enhance customer experience, ensuring long-term loyalty.
- Explanation of sentiment analysis
Sentiment analysis involves the systematic examination of customer opinions expressed in various forms, such as reviews, social media posts, and survey responses. This technique employs natural language processing and machine learning algorithms to identify emotions tied to specific words or phrases. By categorizing sentiments as positive, negative, or neutral, businesses can gain valuable insights into customer feelings and preferences.
Understanding sentiment analysis is crucial for implementing sentiment-based churn prediction. It helps detect underlying issues or trends that may lead to customer dissatisfaction. By analyzing sentiments, businesses can proactively address concerns, improving the overall customer experience. This data-driven approach allows for better decision-making and targeted strategies that can mitigate potential churn. Ultimately, sentiment analysis serves as a powerful tool in identifying at-risk customers and enhancing retention efforts.
- Importance of sentiment analysis in customer experience
Sentiment-based Churn Prediction demonstrates the essential role sentiment analysis plays in enhancing customer experience. By understanding customer emotions through their feedback, businesses can identify patterns that indicate dissatisfaction or frustration. This allows companies to proactively address any emerging issues before they lead to customer churn. Recognizing and acting upon these sentiments can significantly improve customer satisfaction and loyalty.
Moreover, sentiment analysis provides insights that help personalize experiences for customers. Tailoring interactions based on their expressed feelings creates a meaningful connection, fostering trust and engagement. Businesses can refine their strategies by analyzing sentiment trends, ultimately leading to increased retention rates. By integrating sentiment analysis into customer experience initiatives, organizations not only mitigate churn but also cultivate a more loyal customer base. Thus, employing sentiment-based churn prediction is a critical strategy for enhancing overall business success.
The Role of Sentiment Analysis in Predicting Churn
Sentiment-based Churn Prediction plays a critical role in understanding customer behavior. By analyzing customer sentiments expressed in feedback, reviews, or interactions, organizations can identify potential dissatisfaction before it escalates to churn. Negative sentiment can serve as an early warning signal, allowing organizations to take proactive measures to retain customers effectively.
Utilizing sentiment analysis provides various benefits in predicting churn. First, it offers insights into customer emotions, enabling teams to address issues promptly. Second, organizations can tailor their strategies to meet customer needs based on these emotional cues. Finally, businesses that effectively interpret sentiment signals often observe improved customer loyalty and satisfaction, ultimately reducing churn rates. By integrating sentiment analysis into churn prediction models, organizations can enhance their customer retention strategies and remain competitive in the market.
- How sentiment analysis can signal customer dissatisfaction
Sentiment analysis plays a crucial role in identifying customer dissatisfaction by analyzing their feedback and sentiments expressed across various platforms. By extracting and interpreting emotions from data, businesses can gain valuable insights into potential issues that may lead to churn. For instance, a sudden increase in negative sentiment regarding a product or service can signal underlying problems that require immediate attention.
Additionally, sentiment-based churn prediction enables organizations to proactively address customer concerns. Recognizing trends in negative feedback allows companies to implement necessary changes, thus enhancing the overall customer experience. By monitoring sentiment over time, businesses can devise tailored strategies that not only mitigate dissatisfaction but also foster loyalty. Through this proactive approach, companies stand to transform potential churn risks into opportunities for improvement and growth, solidifying customer relationships and promoting longevity in their market presence.
- Benefits of predicting churn using sentiment analysis
Predicting churn using sentiment analysis provides significant advantages for businesses seeking to enhance customer retention. Firstly, it enables organizations to proactively identify at-risk customers based on their feedback. By analyzing customer sentiments, companies can discern early signs of dissatisfaction, allowing them to address issues before they escalate. This proactive approach can lead to timely interventions that not only retain customers but also enhance overall satisfaction.
Moreover, sentiment-based churn prediction fosters a deeper understanding of customer preferences and behaviors. By examining the nuances of customer feedback, businesses can uncover trends and patterns that might otherwise go unnoticed. This insight allows for targeted strategies that cater specifically to customer needs, creating a more personalized experience. Ultimately, using sentiment analysis to predict churn empowers companies to make informed decisions, which can strengthen customer loyalty and drive long-term success.
Extract insights from interviews, calls, surveys and reviews for insights in minutes
Steps to Implement Sentiment-based Churn Prediction
Implementing a Sentiment-based Churn Prediction system involves several key steps that ensure effective analysis and accurate predictions. The first essential step is data collection and processing. This includes gathering sentiment data from diverse sources such as customer feedback, social media comments, and support interactions. Itโs crucial to clean and prepare this data meticulously to ensure reliable insights.
The second step focuses on analyzing the sentiment data. Utilizing various techniques and tools for sentiment analysis can significantly enhance the accuracy of predictions. Tools like Insight7 or Lexalytics provide automation features that streamline the analysis process, making it easier to derive actionable insights from the data.
Finally, building a churn prediction model involves integrating the analyzed sentiment data into existing churn frameworks. It is important to evaluate and refine the model regularly, ensuring that it remains accurate and relevant over time. By following these steps, organizations can leverage sentiment analysis to proactively address customer dissatisfaction and reduce churn effectively.
Step 1: Data Collection and Processing
Data collection and processing is a crucial first step in sentiment-based churn prediction. To begin, you must gather sentiment data from various sources, such as social media, customer reviews, and contact center transcripts. Each source offers unique insights into customer feelings and experiences. The next step involves cleaning and preparing this collected data for analysis. This process ensures that the data is accurate, relevant, and ready for interpretation.
Once you have cleaned the data, categorize sentiments into positive, negative, and neutral responses. This categorization helps identify trends and patterns that might indicate potential customer churn. Addressing how and where customers express their sentiments is essential, as it informs your analysis and enables you to develop effective strategies to reduce churn. Preparing a comprehensive dataset equips you to leverage these insights effectively in your churn prediction models.
- Methods to gather sentiment data from different sources
To effectively gather sentiment data for predicting churn, several methods can be employed across diverse sources. Social media platforms, for instance, play a crucial role in capturing real-time customer sentiments through comments and reactions. Monitoring reviews on websites allows businesses to analyze customer feedback and identify pain points directly influencing churn. Additionally, leveraging surveys can yield structured sentiment data, providing insights into customer satisfaction.
Other sources include customer support interactions, which often contain valuable sentiments expressed during conversations. This qualitative data can enhance understanding of customer experiences and attitudes. Moreover, utilizing email sentiment analysis helps identify positive or negative feelings in customer correspondence. By integrating these varied data sources, organizations can create a comprehensive view that informs sentiment-based churn prediction, allowing for timely interventions and improved retention strategies.
- Cleaning and preparing data for analysis
Cleaning and preparing data for analysis is a crucial step in executing sentiment-based churn prediction effectively. First, gather sentiment data from diverse sources such as customer feedback, reviews, and social media interactions. Once collected, focus on cleaning the data to eliminate inaccuracies, duplicates, and irrelevant information, which may skew your analysis.
After cleaning, organizing the data into a structured format is essential for thorough analysis. Labeling sentiment polarityโpositive, negative, or neutralโhelps in understanding customer perceptions. Additionally, using tools to automate parts of this process can enhance efficiency. This preparation allows for better insights, enabling a more accurate prediction of potential churn based on customer sentiment. By ensuring the data's integrity and clarity, businesses can significantly improve their churn prediction models and take proactive measures to retain customers.
Step 2: Analyzing Sentiment Data
Analyzing sentiment data is integral to understanding customer emotions and behaviors. Effective sentiment-based churn prediction requires a thorough examination of sentiment feedback. Begin by categorizing comments into positive and negative sentiments, pinpointing the emotional drivers behind customer sentiment. This segmentation allows for a more nuanced understanding of customer loyalty and dissatisfaction.
Once sentiments are categorized, deploy advanced analytical tools that provide deeper insights into the data. Utilize platforms that allow for querying various datasets, enabling comparisons across regions or product lines. This comparative analysis can reveal trends and patterns, enhancing the accuracy of your churn predictions. Ultimately, analyzing sentiment data transforms qualitative feedback into actionable insights, equipping businesses to proactively address issues. By mastering sentiment data analysis, organizations can significantly reduce churn rates and improve customer retention strategies.
- Techniques for sentiment analysis
To effectively implement sentiment-based churn prediction, employing various techniques for sentiment analysis is essential. First, text-based methods such as natural language processing (NLP) can be used to analyze customer feedback. This involves parsing through written data, identifying emotions, and categorizing sentiment into positive, negative, or neutral sentiments. Secondly, sentiment scoring models, which assign numerical values to ratings, allow businesses to quantify customer feelings and predict potential churn based on negative scores.
Another technique involves integrating social media sentiment analysis. By monitoring platforms where customers express feedback, companies can gauge public perception. Additionally, using machine learning algorithms to automate sentiment analysis can offer real-time insights. This process enhances the ability to swiftly identify dissatisfied customers and intervene before they choose to leave. Ultimately, these techniques form a comprehensive approach, enabling data-driven insights that significantly improve churn prediction efforts.
- Tools to automate sentiment analysis
To automate sentiment analysis effectively, several tools can help streamline the process of gathering and interpreting customer feedback. Each tool has unique features designed to facilitate sentiment-based churn prediction, making it easier to identify potential dissatisfaction among customers.
Insight7: Known for its user-friendly interface, it allows organizations to analyze customer feedback quickly. Detailed sentiment insights guide decisions to improve customer experiences and reduce churn.
MonkeyLearn: This platform provides customizable models for sentiment analysis, enabling users to tailor solutions based on specific needs. Its versatility supports various use cases, from social media monitoring to customer service optimization.
Lexalytics: This tool stands out for its robust analytical capabilities. It offers deep insights into customer sentiments and trends, simplifying the identification of churn signals.
RapidMiner: Combining machine learning and data analysis, RapidMiner helps in building predictive models. Its features support organizations in refining their sentiment analysis processes over time.
Google Cloud Natural Language: A popular choice for its powerful API, it can analyze and extract sentiment from text in multiple languages, enhancing global customer insights.
Utilizing these tools not only saves time but also provides valuable insights, contributing significantly to successful sentiment-based churn prediction. By automating the analysis, businesses can focus on crafting strategies to improve customer retention and satisfaction.
Tools for Sentiment Analysis
When implementing sentiment-based churn prediction, selecting the right tools is crucial. Various platforms simplify sentiment analysis, enabling businesses to analyze customer sentiments effectively. Key tools include Insight7, which excels in processing extensive datasets, thereby unearthing valuable insights from customer interactions. MonkeyLearn stands out for its intuitive interface, which allows users to customize sentiment analysis according to specific needs.
Lexalytics offers robust functionalities, catering to businesses that require in-depth textual analysis. RapidMiner provides a rich set of machine learning tools specifically designed for sentiment analysis, while Google Cloud Natural Language allows use of cloud technology to streamline sentiment extraction. By leveraging these tools, businesses can enhance their understanding of customer sentiments, leading to more precise churn predictions. Utilizing proper sentiment analysis tools can significantly transform how organizations approach customer retention strategies in today's competitive landscape.
- Insight7: Overview and features
In the realm of sentiment-based churn prediction, using advanced tools can significantly enhance your insights into customer behavior. Insight7 stands out as a user-friendly platform designed to democratize access to valuable data. It enables anyone in your organization to conduct sentiment analysis without the need for specialized training or expertise. This intuitive approach makes it easy to gather insights from customer interactions in real time.
The platform features a comprehensive library for organizing and analyzing multiple files, such as customer calls. By automatically extracting key themes like pain points and customer desires, Insight7 provides a clear overview of sentiment trends that could indicate potential churn. Additionally, users can visualize conversations, enabling a deeper understanding of customer feedback. These capabilities position Insight7 as a powerful ally in predicting churn, empowering organizations to proactively address customer concerns and enhance retention strategies.
- MonkeyLearn: Key functionalities and use cases
The platform offers a range of functionalities designed to facilitate effective sentiment-based churn prediction. Users can seamlessly navigate through its intuitive interface to conduct analyses without needing specialized training. For instance, one can easily gather customer feedback or analyze recorded conversations to uncover valuable insights. Such capabilities allow businesses to understand their customersโ sentiments on a large scale, highlighting potential areas of dissatisfaction.
Another key functionality lies in the ability to visualize and cluster insights from multiple data sources. It enables users to extract actionable information, such as pain points and customer desires, which can be critical for predicting churn. By efficiently summarizing themes from numerous calls or surveys, businesses can proactively address issues, ultimately enhancing customer retention. These use cases exemplify how companies can leverage user sentiment to develop stronger relationships and mitigate churn effectively.
- Lexalytics: Benefits and features
Incorporating effective sentiment analysis tools can drastically change how businesses perceive customer feedback. These tools can analyze vast amounts of customer data, converting unstructured text into actionable insights. A system designed for ease of use enables employees across various departments to engage with the data without needing specialized training. This democratization of insights allows organizations to identify potential churn risks by highlighting customer pain points and concerns.
Moreover, the platform automates the extraction of valuable information from customer interactions, showcasing themes like pain points and positive feedback. This ability to distill key sentiments from conversations not only supports targeted interventions but also empowers businesses to improve customer experience actively. By utilizing sentiment-based churn prediction tools, businesses can anticipate customer dissatisfaction, address issues proactively, and foster stronger, long-lasting relationships with their clients.
- RapidMiner: Features that aid in sentiment analysis
Incorporating effective tools for sentiment analysis can significantly enhance sentiment-based churn prediction. One prominent platform provides essential features designed to analyze customer feedback effectively. By enabling organizations to sift through large volumes of data, this platform helps identify key sentiment indicators related to customer satisfaction.
One of the standout features is the ability to visualize data trends. Users can quickly spot positive and negative sentiments across various customer interactions, offering invaluable insights into overall customer experiences. Additionally, the platform supports advanced querying capabilities, allowing users to compare sentiment patterns across different locations or demographics. This means businesses can tailor their strategies based on specific customer sentiments, resulting in improved retention strategies and actionable insights into potential churn. By effectively leveraging these features, organizations can better manage customer relationships and proactively address concerns, ultimately reducing churn rates.
- Google Cloud Natural Language: Sentiment analysis options
Understanding sentiment analysis options can significantly enhance your ability to predict churn effectively. Tools like Google Cloud Natural Language provide robust solutions for sentiment-based churn prediction. At the core, this service uses advanced machine learning techniques to evaluate customer responses, categorizing sentiments into positive, negative, or neutral. By automating this analysis, businesses can quickly assess large volumes of customer interactions, enabling proactive engagement strategies.
When utilizing these sentiment analysis options, organizations can focus on specific insights. First, they can analyze customer feedback across various platforms to identify dissent signals, actionable feedback, and potential areas of improvement. Secondly, by visualizing sentiment trends over time, businesses can correlate positive or negative shifts with customer behavior, leading to informed decisions that reduce churn rates. Ultimately, leveraging these tools for sentiment analysis allows for deeper customer understanding, ultimately driving retention strategies and supporting long-term growth.
Step 3: Building a Churn Prediction Model
Building a churn prediction model involves integrating sentiment data with existing customer insights. This is crucial for understanding customer emotions and predicting potential churn. By analyzing sentiment data, organizations can identify patterns that indicate dissatisfaction, helping to address issues before they escalate. The primary objective here is to create a robust model that effectively predicts which customers may be at risk of leaving.
The first step in building this model is to combine the sentiment analysis results with churn-related data, such as purchase history and engagement metrics. Next, apply machine learning algorithms to analyze these datasets, allowing the model to identify at-risk customers based on specific sentiment indicators. Finally, continually evaluate and refine the model to enhance its predictive accuracy and ensure it responds effectively to changing customer sentiments. This approach to sentiment-based churn prediction is essential for proactive customer retention strategies.
- Integrating sentiment data into churn models
Integrating sentiment data into churn models is a critical step toward effective sentiment-based churn prediction. This process begins with the collection of quantitative and qualitative sentiment data derived from customer interactions, like surveys and social media feedback. By analyzing this data, businesses can gain valuable insights into customer feelings and attitudes, which often foreshadow potential churn.
Next, itโs essential to incorporate this sentiment analysis within existing churn prediction models. This integration helps create a more comprehensive view of customer behavior, combining traditional metrics with emotional indicators. For instance, clients expressing dissatisfaction through negative feedback may indicate a higher likelihood of leaving. Thus, enhancing traditional churn models with sentiment data facilitates early intervention strategies to retain at-risk customers. By monitoring sentiment trends and adjusting engagements, businesses can proactively address concerns, ultimately reducing churn rates.
- Evaluating and refining the model for accuracy
Evaluating and refining the model for accuracy is a critical component of Sentiment-based Churn Prediction. First, it is essential to assess the model's performance using various metrics, including precision, recall, and F1 score. These metrics provide insights into how well the model identifies customers at risk of churn. By analyzing these figures, you can determine if the model successfully captures sentiment signals indicative of customer dissatisfaction.
Once the initial evaluation is complete, refining the model becomes crucial. This may involve adjusting parameters, incorporating additional data sources, or utilizing different algorithms to enhance predictive accuracy. Regularly retraining the model with updated sentiment analysis insights is advisable, especially as customer attitudes change over time. This iterative process ensures that your churn prediction remains relevant and effective, ultimately helping improve customer retention strategies.
Conclusion: Maximizing the Benefits of Sentiment-based Churn Prediction
To maximize the benefits of sentiment-based churn prediction, businesses must leverage the insights gained from customersโ emotions and feedback. By effectively interpreting sentiment data, organizations can anticipate potential churn points and take proactive measures. This leads to enhanced customer satisfaction and improved retention strategies, ultimately translating into higher profitability.
Implementing sentiment-based churn prediction allows companies to become more responsive to their customersโ needs. By paying attention to positive and negative sentiments expressed through various feedback channels, businesses can tailor their offerings and services. In doing so, they not only reduce churn but also foster loyalty and long-term relationships with their clientele.