AI-driven Longitudinal Tracking is revolutionizing the way researchers gather and analyze data over extended periods. Traditional methods often struggle with the complexities of capturing nuanced changes over time. However, AI technologies can seamlessly process vast amounts of data, extracting insights that were previously obscured in plain sight. This capability not only enables more accurate findings but also accelerates the research process, allowing researchers to focus on interpretation rather than data collection.
The integration of AI into longitudinal research enhances data reliability and depth significantly. Researchers can harness AI tools to identify trends, patterns, and anomalies that inform their studies meaningfully. Over time, such insights can lead to critical advancements in fields ranging from healthcare to social sciences. Understanding AI-driven Longitudinal Tracking is essential for researchers aiming to improve the accuracy and efficacy of their work, making it a vital area of exploration today.
Analyze qualitative data. At Scale.
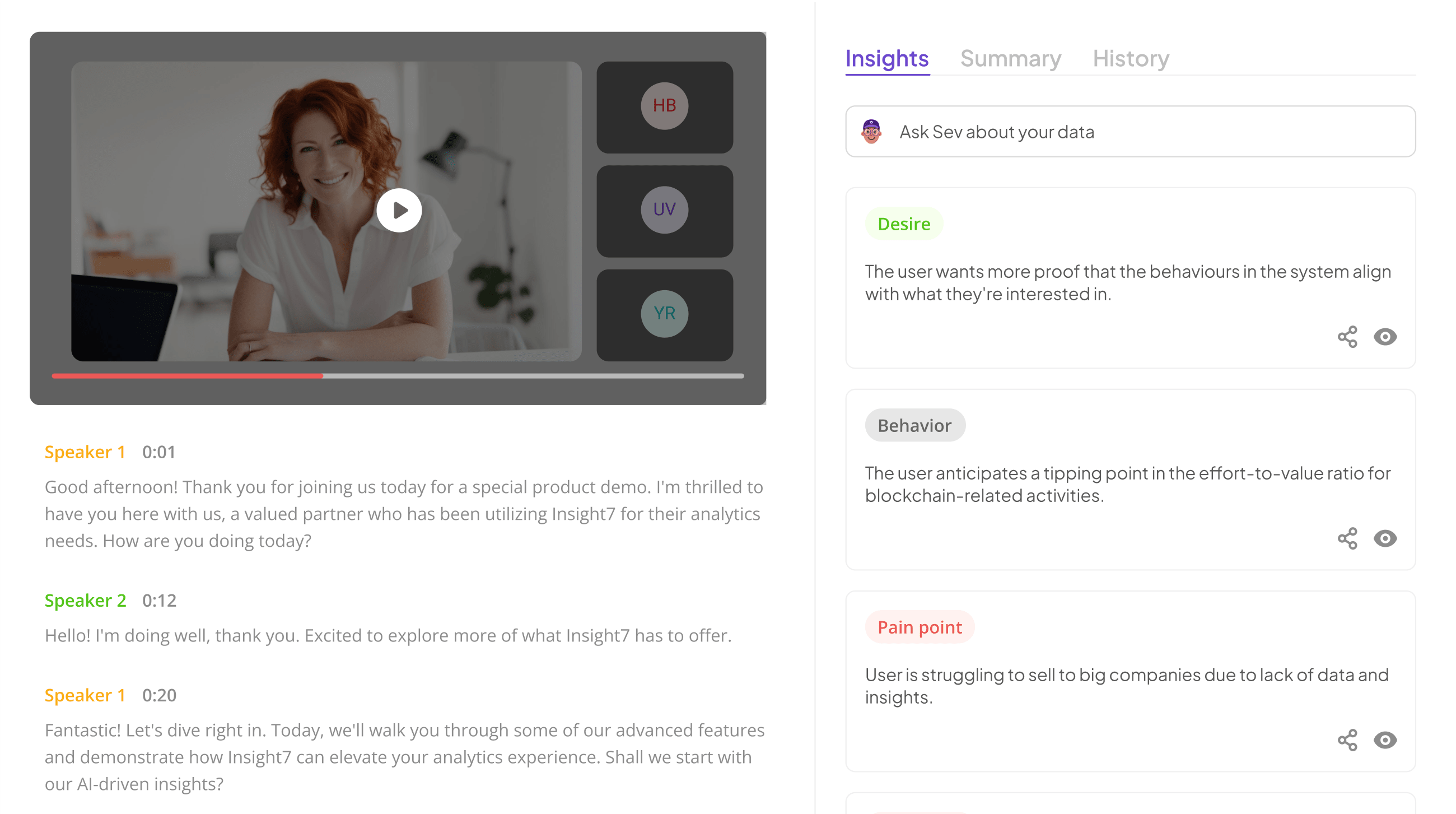
Understanding AI-driven Longitudinal Tracking
AI-driven Longitudinal Tracking revolutionizes the way researchers gather and analyze data over time. By employing artificial intelligence, researchers can effectively manage complex datasets and identify patterns that emerge across different time points. This method allows for deeper insights into trends, behaviors, and changes in various subjects, enhancing the overall research quality.
A key aspect of AI-driven Longitudinal Tracking is its ability to automate data analysis, increasing both accuracy and efficiency. Researchers can integrate various data sources, transcribe responses, and quickly extract meaningful insights without the cumbersome processes of traditional methods. As technology evolves, the capacity for nuanced data interpretation only grows, empowering researchers to make informed decisions based on reliable longitudinal evidence. Understanding these tools is crucial for those looking to maximize their research potential and gain significant insights.
The Role of AI in Longitudinal Research
AI-driven Longitudinal Tracking plays a transformative role in longitudinal research by enhancing data collection and analysis. Traditional longitudinal studies often face challenges such as data inconsistency and slower processing times. By incorporating AI, researchers can collect real-time data from various sources, leading to more accurate insights. This technological advancement allows for a comprehensive examination of patterns and trends over extended periods, making longitudinal tracking significantly more effective.
Moreover, AI enhances the interpretative capability of researchers by identifying correlations that might go unnoticed in manual analysis. With AI's ability to automate data processing and analysis, researchers can focus on in-depth evaluations of their findings. As a result, they can uncover deeper, actionable insights, ultimately enriching the research process. The integration of AI truly revolutionizes the way longitudinal research is conducted, making it faster, more reliable, and fundamentally insightful.
- Explanation of longitudinal research and its significance.
Longitudinal research is a method of data collection that focuses on observing subjects over an extended period. This approach allows researchers to identify trends, patterns, and changes in behaviors or conditions over time. By continually tracking the same individuals or groups, longitudinal studies provide invaluable insights into developmental processes and causal relationships, significantly enhancing the understanding of complex issues.
The significance of this research method lies in its ability to provide a comprehensive view of changes that occur. Unlike snapshot studies, longitudinal research captures the evolution of data, allowing for deeper analysis. AI-driven longitudinal tracking enhances this process by automating data collection, ensuring consistency and accuracy in tracking insights. Moreover, advanced data analytics can uncover hidden patterns within the longitudinal data, helping researchers make informed decisions based on evolving trends and behaviors. This synergy between AI and longitudinal research is crucial for developing strategic interventions and understanding dynamic changes over time.
- How AI enhances the process.
AI-driven Longitudinal Tracking brings transformative enhancements to research processes by streamlining data collection and analysis. With the power of machine learning algorithms, researchers can efficiently manage vast datasets and identify trends that would otherwise remain hidden. This technology automates aspects of tracking, alleviating the manual burdens of data maintenance and enhancing overall accuracy.
Moreover, AI algorithms enable researchers to analyze changes over time with remarkable precision. They can detect subtle shifts in data patterns, providing more reliable insights. This capacity extends to improving participant engagement, as AI tools can personalize interactions based on accumulated data. By leveraging AI-driven Longitudinal Tracking, researchers not only save time but also gain more profound insights into trends across various fields, ultimately enriching the quality of research outcomes.
Key Benefits of AI-driven Longitudinal Tracking
AI-driven Longitudinal Tracking brings transformative advantages to research methodologies. One key benefit is improved accuracy, allowing researchers to analyze data patterns over extended periods. This capability enhances the reliability of insights, enabling informed decision-making. Additionally, AI facilitates a faster processing of vast datasets, thus increasing efficiency. Researchers can focus on critical analysis rather than spending excessive time on data preparation.
Another significant advantage is enhanced data interpretation over time. AI technology can identify evolving trends and correlations that might be missed through traditional methods. This longitudinal approach helps in comprehensively understanding long-term effects and behavioral changes. As a result, researchers gain a richer context for their findings. Leveraging these capabilities ultimately fosters more dynamic and impactful research outcomes while meeting the growing demand for faster insights in various fields.
- Improved accuracy and efficiency.
AI-driven Longitudinal Tracking significantly enhances the accuracy and efficiency of research. By automating data collection and analysis, researchers can quickly obtain deeper insights over extended periods. Traditional methods often lead to data inconsistencies and lag in responsiveness, while AI minimizes these challenges through real-time updates and processing. This automation also reduces human error, allowing for a more precise interpretation of data trends.
Furthermore, the efficiency gained from AI tools allows researchers to focus more on strategic decision-making rather than data management. With functionalities like bulk data uploads and native transcription, teams can streamline their research workflow. Instant retrieval of insights and visualizations eliminates delays, accelerating the time to actionable conclusions. Research professionals can now harness AI-driven longitudinal tracking not just to collect data, but to drive meaningful conversations and innovations based on accurate and efficient outcomes.
- Enhanced data interpretation over time.
AI-driven Longitudinal Tracking provides a unique opportunity to enhance data interpretation over time. This approach enables researchers to analyze trends and patterns that emerge through extensive data collection. By continuously monitoring changes, AI tools facilitate a deeper understanding of variables influencing research outcomes. This ongoing analysis integrates historical data with real-time insights, helping to reveal shifts in behavior or sentiment effectively.
As data accumulates, AI-driven algorithms can adapt and refine their understanding of the underlying narratives. This evolution allows for more accurate predictions and actionable insights that are vital for research success. Not only do these tools streamline the analysis process, but they also foster a culture of continuous improvement. By examining longitudinal data, researchers can uncover subtle trends that traditional methods might overlook, ultimately leading to more informed decisions and robust conclusions.
Extract insights from interviews, calls, surveys and reviews for insights in minutes
Implementing AI-driven Longitudinal Tracking in Research
Implementing AI-driven Longitudinal Tracking in research requires a strategic approach. First, researchers must clearly define their goals and the specific data they wish to gather. This foundational step sets the direction for effectively integrating AI into longitudinal studies. Once objectives are established, selecting the right AI tools becomes essential. Various platforms offer unique features that cater to different research designs.
Next, data collection processes need to be established, ensuring consistency and reliability over time. Researchers should also consider how AI can process and analyze patterns throughout the research duration. By continually refining their methodologies, teams can leverage AI-driven longitudinal tracking to gain invaluable insights. This organized approach ensures that studies remain relevant, accurate, and aligned with ongoing research goals. By embracing these strategies, researchers can unlock the full potential of AI in capturing and interpreting longitudinal insights, ultimately enhancing the quality of their findings.
Step-by-step Guide to Setting Up AI-driven Tools
Setting up AI-driven tools for tracking longitudinal insights in research is a structured process. Begin by identifying your research goals. Understanding what you aim to achieve will guide your tool selection. Next, establish your data requirements—this includes determining the types of data you need and how frequently you intend to collect it.
Once your goals and data criteria are set, proceed to select the appropriate AI tools for longitudinal analysis. Research the features of various tools, considering how well they integrate with your existing data systems. Ensure that the selected tools can accurately capture longitudinal data patterns, offer effective data visualization, and provide user-friendly reporting options. By carefully following these steps, you can harness the power of AI-driven longitudinal tracking to improve insights in your research efforts.
- Identifying research goals and data requirements.
Establishing clear research goals is paramount for effective AI-driven longitudinal tracking. Start by defining what specific insights you aim to gain from your research. Are you focusing on user behavior changes, product performance, or market trends? Clarifying these objectives allows you to structure your data collection and analysis effectively. Equally important is understanding your data requirements. This includes identifying what type of data will be necessary for achieving your goals, such as demographics, usage statistics, or sentiment analysis.
Once your goals and data needs are outlined, the next step is selecting the appropriate AI tools tailored to your defined objectives. Tools that offer features like advanced data clustering, tagging, and sentiment analysis can help in extracting meaningful insights from large datasets. By aligning your research goals with the capabilities of AI-driven tools, you ensure a streamlined process that enhances the quality of your longitudinal insights over time.
- Selecting appropriate AI tools for longitudinal analysis.
Selecting the right AI tools is essential for effective longitudinal analysis in research. Begin by clarifying your research objectives and the type of data you’ll be working with. Focus on tools that offer capabilities tailored to your specific needs, such as data collection, analysis, and visualization. User-friendly interfaces and robust support systems are also important considerations, as these factors can significantly streamline the research process.
Next, evaluate the features that each AI tool offers. Look for functionality that supports tracking changes over time, as effective longitudinal analysis hinges on understanding trends and shifts in data. For instance, tools that utilize natural language processing can help identify themes from qualitative data, while predictive analytics can forecast future trends based on historical data. Carefully analyzing these features will lead to a more informed selection and ultimately enhance your AI-driven longitudinal tracking efforts.
Top Tools for AI-driven Longitudinal Tracking
In the realm of AI-driven longitudinal tracking, selecting the right tools can vastly improve the efficiency and accuracy of your research. Several platforms cater specifically to the needs of longitudinal studies, providing comprehensive data analysis and insightful feedback mechanisms. Insight7, for instance, offers native transcription services that streamline the ability to ingest and analyze data. This platform allows researchers to extract themes and generate reports easily.
Another notable tool is IBM Watson. Known for its robust data processing capabilities, it aids researchers in tracking patterns over time effectively. Google AI stands out for its ability to assist in analyzing long-term data, revealing trends that might otherwise go unnoticed. SAS Visual Analytics provides powerful visualization options to interpret longitudinal insights intuitively. Lastly, RapidMiner enhances analytical depth and capability, enabling users to perform extensive data manipulation. Each of these tools plays a crucial role in the success of AI-driven longitudinal tracking, turning complex datasets into actionable insights.
- Insight7
AI-driven Longitudinal Tracking plays a pivotal role in research by transforming how data is collected and analyzed over time. It enables researchers to obtain continuous insights, rather than relying on static snapshots. This ongoing analysis allows for a deeper understanding of trends, shifts in behavior, and evolving patterns prevalent in user feedback and market dynamics.
To effectively utilize AI-driven Longitudinal Tracking, researchers should embark on several key steps. First, they must define specific research objectives to clarify what insights they seek. Next, selecting the right AI tools is essential for gathering and interpreting data efficiently. These tools can automate data collection and provide advanced analytics capabilities that enhance the accuracy of longitudinal insights. Finally, consistent monitoring is crucial as it allows researchers to adapt to the changing landscape and refine their strategies accordingly. By embracing these approaches, researchers can leverage AI technology to yield actionable insights that greatly inform decision-making processes.
- Overview and features of Insight7 for longitudinal research.
Insight7 offers a powerful platform designed for AI-driven longitudinal tracking, specifically tailored to enhance the process of conducting research over extended periods. This intuitive tool allows users to effortlessly import and organize data, making it accessible for analysis. By clustering related files within projects, researchers can streamline their workflow, facilitating deeper insights into patterns and trends.
Key features include the ‘Library’ function, where all data resides, enabling quick retrieval of past interviews or transcripts. In addition, the platform provides analytical kits that jumpstart the analysis process, allowing users to extract themes and quotes efficiently. These capabilities significantly improve the accuracy and speed of data interpretation over time, ultimately enriching the research experience. With its collaborative nature, Insight7 empowers researchers to extract actionable insights with ease, paving the way for informed decision-making in their longitudinal studies.
- IBM Watson
AI-driven Longitudinal Tracking invites researchers to tap into powerful tools that streamline the monitoring of data over extended periods. This section focuses on leveraging AI to collect, analyze, and interpret longitudinal research data seamlessly. By utilizing advanced algorithms, tools can efficiently track changes and trends, providing deeper insights into the phenomena being studied.
Effective tracking not only enhances data accuracy but also significantly reduces the time researchers spend on manual analysis. AI systems can identify patterns and correlations that might be overlooked during traditional assessments. Moreover, the adaptability of these tools allows for continuous improvement in research methodologies, ensuring that data remains relevant and actionable over time.
Incorporating AI-driven Longitudinal Tracking fosters a dynamic approach to research, empowered by technology. Researchers equipped with these tools can confidently navigate the complexities of longitudinal data, turning insights into actionable recommendations for their fields.
- Utilization in tracking research data over time.
The utilization of AI in tracking research data over time significantly transforms how researchers manage and analyze longitudinal studies. AI-driven longitudinal tracking offers powerful tools that empower researchers to continuously monitor trends and changes, enhancing the reliability of their findings. By automating data collection and analysis, researchers can focus on deeper insights rather than tedious data management tasks.
One crucial aspect is the ability to define specific themes or tags within the data, allowing researchers to classify insights according to their research objectives. For instance, by clustering insights into distinct categories, AI can generate a clear overview of participant feedback over time. This method not only streamlines data interpretation but also aids in identifying emerging patterns and sentiments. Ultimately, AI-driven longitudinal tracking enables robust analysis that adapts as new data becomes available, ensuring that researchers remain at the forefront of their studies with informed, actionable insights.
- Google AI
The integration of artificial intelligence offers transformative potential for longitudinal research. It can automate the analysis of trends over time, simplifying the identification of patterns that might otherwise go unnoticed. By processing vast amounts of data quickly, AI-driven tools allow researchers to gain insights at unprecedented speeds. This capability leads to more accurate conclusions and informed decisions, ultimately enhancing the quality of research outcomes.
To implement AI in your longitudinal studies, consider several essential steps. First, clearly define your research objectives and the type of data you need to analyze. Next, choose AI tools specialized for longitudinal tracking, which excel in organizing and interpreting data over extended periods. With these tools, you will be better equipped to uncover valuable insights and enhance the overall impact of your research efforts. This strategic approach to AI-driven longitudinal tracking can significantly elevate your research initiatives.
- How it assists in analyzing longitudinal patterns.
AI-driven longitudinal tracking plays a crucial role in analyzing patterns over time, providing researchers with a dynamic view of evolving data. By employing advanced algorithms, AI can identify trends and correlations that might otherwise remain hidden. This capability allows researchers to interpret changes effectively, enhancing their understanding of variables impacting the study.
One key aspect of this analysis involves sentiment evaluation. AI-driven tools can cluster insights into meaningful themes, such as user preferences and challenges encountered. This thematic clustering not only simplifies data interpretation but also aligns findings with specific research goals. Consequently, researchers can derive actionable insights from longitudinal patterns, ultimately improving decision-making and strategic planning. By continuously analyzing data and adjusting for emerging trends, AI ensures that insights remain relevant and useful throughout the research journey.
- SAS Visual Analytics
SAS Visual Analytics serves as a powerful tool in the realm of research, particularly when it comes to AI-driven longitudinal tracking. This platform enables researchers to visualize complex data across various time periods, making it easier to identify trends and patterns. By employing advanced analytics techniques, researchers can transform raw data into actionable insights that span multiple years, significantly enhancing the research process.
The capability to define themes and categorize insights enables a deeper understanding of evolving variables over time. For instance, researchers can set specific goals—such as improving member experiences or tracking retention metrics—allowing SAS Visual Analytics to organize data accordingly. This thematic mapping streamlines the analysis process and presents findings in a user-friendly manner. Ultimately, using SAS Visual Analytics for AI-driven longitudinal tracking ensures that insights are not only accurate but also relevant, empowering researchers to make informed decisions based on comprehensive data trends.
- Tool capabilities for longitudinal data insights.
AI-driven Longitudinal Tracking offers researchers the capability to gather, analyze, and interpret data effectively over time. The tools designed for this purpose can aggregate data from various sources, enabling comprehensive insights into evolving trends and themes. One key feature of these tools is their ability to perform bulk transcriptions, converting audio or video data into text, which can then be systematically analyzed. This function not only enhances accessibility but also allows researchers to extract meaningful quotes and identify recurring patterns in responses.
Moreover, quality AI-driven tools provide advanced analytical capabilities, ensuring researchers derive actionable insights from the data gathered. Features such as project folders enable easy management of multiple datasets, facilitating collaborative analysis. By continuously monitoring changes within the data, these tools empower researchers to adjust their methodologies and hypotheses based on real-time insights. The integration of AI technologies into longitudinal studies has transformed the traditional research landscape, making it more dynamic and responsive to emerging patterns.
- RapidMiner
RapidMiner emerges as a powerful tool in the realm of AI-driven longitudinal tracking. Its capabilities enable researchers to automate the analysis of time-series data, saving invaluable time and effort. Users can easily set up their data pipelines, ensuring that ongoing research remains organized and accessible. By offering a user-friendly interface, RapidMiner allows researchers to focus on interpretation rather than getting lost in complex data manipulation.
With the integration of advanced machine learning algorithms, this tool enhances the accuracy of insights derived from longitudinal studies. Researchers can visualize trends over time, providing clarity in data interpretation. As researchers delve deeper into their findings, RapidMiner's capabilities ensure they remain equipped to identify patterns that may evolve, ultimately enriching the understanding of research subjects. By streamlining this process, RapidMiner helps in delivering timely and actionable insights, thus fostering informed decision-making based on empirical data.
- Leveraging its AI for comprehensive longitudinal analysis.
AI-driven Longitudinal Tracking serves as a transformative approach for researchers aiming to analyze data over extended periods. This technique allows for continuous monitoring and evaluation of trends, providing a more nuanced understanding of changing dynamics in research subjects. By automating data collection and analysis, AI simplifies tasks that traditionally required extensive manual effort.
To effectively harness the power of AI for comprehensive longitudinal analysis, researchers can focus on several key aspects. First, it's essential to clearly define research objectives and determine the types of data needed. This foundation ensures relevant insights emerge from the analysis. Next, selecting the right AI tools is crucial; user-friendly platforms such as those mentioned throughout the document can significantly enhance data collection and interpretation processes. Ultimately, with the right setup, AI-driven Longitudinal Tracking offers a robust framework for deriving meaningful insights that evolve over time, enriching the overall research landscape.
Conclusion on AI-driven Longitudinal Tracking in Research
AI-driven Longitudinal Tracking has the potential to transform research methodologies by providing deeper insights over time. The integration of AI allows researchers to analyze data efficiently, revealing trends and patterns that traditional methods may miss. By leveraging advanced algorithms, researchers can obtain more accurate and timely results, enhancing the quality of their findings.
Furthermore, this approach democratizes data access, enabling broader collaboration and enabling teams to synthesize insights quickly. As the landscape of research continues to evolve, embracing AI-driven Longitudinal Tracking will be crucial in staying competitive and responsive to changing research demands. Ultimately, this innovation not only streamlines processes but also enriches the overall research experience.