AI-Powered Insight Prioritization is revolutionizing exploratory research by enabling researchers to cut through vast amounts of data quickly and effectively. Traditionally, researchers struggled to discern which insights truly mattered amidst overwhelming information. Today, sophisticated algorithms analyze data patterns and user sentiments to identify priority insights that can drive innovation and strategic decisions.
This section delves into how harnessing AI is not merely about speed, but also about accuracy and relevance. By automating insight prioritization, researchers can focus their efforts on high-impact findings, aligning research processes directly with their objectives. Understanding the potential of AI in this context is crucial for researchers aiming to enhance efficiency and enhance decision-making capabilities. As the demand for actionable insights grows, embracing AI-Powered Insight Prioritization becomes essential for success in exploratory research.
Analyze qualitative data. At Scale.
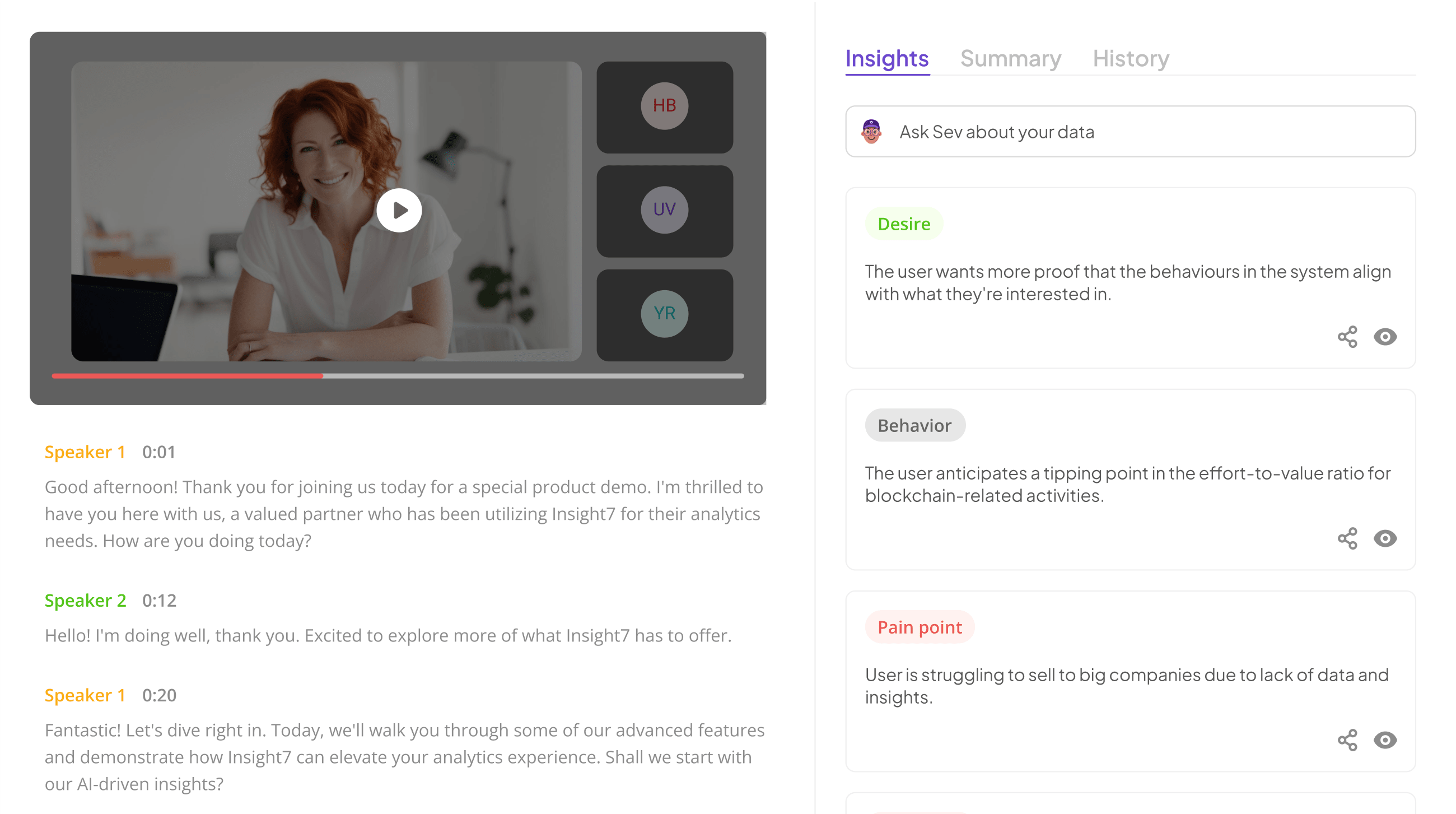
Leveraging AI for Effective AI-Powered Insight Prioritization
AI-Powered Insight Prioritization effectively transforms data into actionable findings, enabling businesses to focus their resources on the most promising insights. By employing advanced algorithms, AI can analyze large datasets quickly and accurately, identifying critical patterns and themes that may be overlooked in manual reviews. This process not only accelerates decision-making but also enhances the overall quality of insights.
To achieve effective AI-powered insight prioritization, organizations should consider several key factors. First, clearly define research objectives and desired outcomes. Next, select appropriate AI tools that align with those objectives, ensuring they offer robust analytic capabilities and user-friendly interfaces. Finally, continuously refine strategies based on feedback and outcomes to optimize the prioritization process. By focusing on these elements, businesses can transform exploratory research into a structured roadmap for informed decision-making, driving success in an increasingly data-driven world.
Understanding Exploratory Research and Its Challenges
Exploratory research serves as a vital tool for understanding trends, user behaviors, and market opportunities. It involves collecting qualitative data that can reveal deeper insights, but this process is often fraught with challenges. One common issue is the sheer volume of data that researchers must sift through, making it difficult to extract meaningful insights efficiently.
Another challenge lies in the subjective nature of exploratory research. Researchers may find it hard to prioritize insights due to bias or personal interpretation. This is where AI-Powered Insight Prioritization comes into play. By leveraging advanced algorithms, teams can automate the sorting and analysis of expansive datasets, making it easier to identify the most relevant findings. Furthermore, AI can help minimize biases by offering a more objective assessment of data, thus enhancing the reliability of insights drawn from exploratory research.
- Definition of exploratory research
Exploratory research is a preliminary phase aimed at gaining insights and generating hypotheses rather than testing them. It often involves qualitative methods such as interviews or focus groups to gather information on a topic where little is previously known. This type of research serves as a foundation, helping researchers identify potential patterns, themes, or questions to explore further.
In the context of AI-Powered Insight Prioritization, exploratory research plays an essential role. It allows organizations to generate raw data that AI tools can later analyze and prioritize effectively. By understanding key insights from exploratory research, researchers can focus their efforts and resources on the most promising areas. This dual process of research and prioritization ensures that insights are not only relevant but also align with strategic goals. Thus, exploratory research enables informed decision-making, enhancing the overall effectiveness of the insights generated.
- Common challenges in prioritizing insights
Prioritizing insights from exploratory research presents common challenges that can hinder effective decision-making. One significant obstacle is sifting through vast amounts of data to identify which insights hold the most strategic value. This process often results in overwhelming complexities due to varying data types and the subjective nature of the insights themselves.
Another challenge lies in establishing clear criteria for prioritization. Different stakeholders may have conflicting priorities, making it difficult to align on what constitutes valuable insights. Additionally, the inability to adopt a standardized approach can lead to inconsistencies in how insights are assessed. AI-Powered Insight Prioritization can help mitigate these issues by bringing systematic frameworks and data analysis capabilities to bear on the insight selection process. By automating theme extraction and clustering insights, AI allows teams to focus on actionable findings rather than getting lost in the data. This can ultimately streamline decision-making and foster innovation.
AI-Powered Insight Prioritization: Transforming Data into Actionable Insights
AI-Powered Insight Prioritization revolutionizes the way organizations extract value from exploratory research data. By harnessing sophisticated algorithms, AI can efficiently synthesize vast amounts of information, allowing researchers to identify key insights with remarkable speed and accuracy. This capability focuses on transforming complex data sets into concrete action points, streamlining decision-making processes.
The benefits of AI-Powered Insight Prioritization extend beyond just speed. AI tools intelligently prioritize insights according to relevance and potential impact, enhancing the strategic value of each insight discovered. This prioritization helps researchers and decision-makers focus on the information that is most likely to drive impactful outcomes, ultimately leading to more effective strategies. As the landscape of data continues to grow, adopting these AI-driven approaches is essential for staying ahead in competitive environments.
- The role of AI in synthesizing large datasets
AI plays a crucial role in synthesizing large datasets, transforming seemingly insurmountable amounts of information into coherent insights. By employing advanced algorithms, AI systems can identify patterns and trends that may not be visible to the naked eye. This capability allows researchers to quickly distill complex data into actionable insights, facilitating more informed decision-making. With AI-Powered Insight Prioritization, teams can funnel their focus on high-impact findings while discarding less relevant information, thus streamlining the research process.
Moreover, AIโs ability to process data across various sources enables a comprehensive overview of insights gathered from exploratory research. Analysts can cluster data points from interviews, surveys, and previous studies, synthesizing them into concise reports or visualizations. The combination of speed and accuracy provided by AI ensures that stakeholders receive timely insights, enhancing their capacity to act swiftly in the dynamic business landscape. Ultimately, AI significantly enhances the quality and efficiency of synthesizing large datasets.
- Benefits of AI in prioritizing insights
AI-Powered Insight Prioritization offers myriad benefits, especially when it comes to transforming challenges into actionable strategies. One significant advantage is the ability to process vast amounts of data swiftly and accurately, moving beyond traditional methods of analysis. By leveraging advanced algorithms, AI identifies key themes and insights that may be overlooked, ensuring that critical information is spotlighted.
Moreover, AI enhances the trustworthiness of insights by rooting decisions in data-backed evidence, thus minimizing biases that often cloud judgment in exploratory research. This capability not only saves time but also facilitates clearer communication within teams, making it easier to produce focused reports. Furthermore, AI's ability to continually learn from new data allows organizations to adapt insights dynamically, aligning them with ever-evolving market conditions and user needs. Overall, AI-Powered Insight Prioritization streamlines the research process, resulting in high-quality insights that drive impactful business decisions.
Extract insights from interviews, calls, surveys and reviews for insights in minutes
Top Tools for AI-Powered Insight Prioritization
Effective AI-Powered Insight Prioritization relies on a variety of specialized tools that enhance how researchers analyze and manage their findings. These tools help sift through large amounts of data, allowing researchers to focus on the insights that matter most. Understanding the capabilities of these tools is essential for making informed decisions and identifying key themes within exploratory research.
Among the top tools available, Insight7 stands out for its unique features that enable users to filter insights effectively and compile comprehensive reports. Other notable tools include sentiment analysis platforms that gauge emotional responses, visualization tools that help interpret data trends, and collaborative software that enhances teamwork throughout the research process. Each of these tools offers distinct capabilities that contribute to the overall goal of AI-Powered Insight Prioritization, ultimately driving more accurate and actionable results from exploratory research.
Insight7 and Its Unique Features
Insight7 introduces innovative features designed to enhance AI-powered insight prioritization. One of its standout functionalities is the automated transcript analysis, which significantly reduces the time spent on initial data processing. This means researchers can swiftly move from data collection to actionable insights, maintaining focus on their core objectives. The platform utilizes advanced algorithms to identify themes and trends, providing clarity on critical insights.
Another unique feature is the visual data representation tools. These tools transform complex datasets into easily digestible formats, making it simpler for teams to understand and act upon their findings. Additionally, Insight7 encourages collaboration through shared workspaces that facilitate real-time discussions among team members. By combining robust analysis with effective visualization, this platform empowers researchers to prioritize insights more efficiently, ensuring their exploratory research translates into impactful decisions.
- Overview of Insight7
Insight7 offers a state-of-the-art solution designed to enhance the process of prioritizing insights derived from exploratory research. This AI-powered platform transforms vast amounts of market data and customer feedback into actionable insights, significantly reducing the time required to make informed decisions. With a seamless integration of AI technology, Insight7 effectively synthesizes qualitative and quantitative data, allowing teams to focus on strategic actions rather than getting lost in overwhelming data sets.
Utilizing advanced algorithms, Insight7 identifies key patterns and trends that may otherwise remain hidden. This capability empowers businesses to understand their markets better and prioritize insights that drive growth. The platform's unique features, such as customizable dashboards and real-time analytics, facilitate a more structured approach to insight prioritization, ensuring that organizations can rapidly adapt to their customers' evolving needs. By maximizing the use of AI-powered insight prioritization, companies can stay ahead in competitive environments.
- Key functionalities for insight prioritization
Understanding the key functionalities for insight prioritization is crucial to harnessing AI's potential in research. AI-Powered Insight Prioritization provides not only speed but also enhanced accuracy in evaluating massive datasets. The technology allows researchers to synthesize information effectively, identifying patterns and trends that may be overlooked in manual analysis. This helps prioritize insights that are most relevant to the goals of the research.
Key functionalities include customized filtering options that help isolate specific themes or topics. Researchers can specify areas of interest, such as process management, ensuring that only the most pertinent insights are extracted. Additionally, the ability to generate comprehensive reports quickly allows for agile decision-making. AI can summarize findings, provide supporting quotes, and even suggest practical recommendations based on the data analyzed. By employing these functionalities, teams can deliver actionable insights promptly, ultimately leading to more effective strategies and outcomes.
Additional Tools for Enhanced Insight Prioritization
When considering additional tools for enhanced insight prioritization, you must contemplate various innovative solutions designed to streamline your research process. Effective tools can provide a structured approach to distill valuable insights from complex data sets. By integrating AI-powered capabilities, these tools enable you to categorize and prioritize findings efficiently, ensuring that you focus on the most impactful insights relevant to your goals.
For instance, thematic analysis tools can cluster insights into meaningful categories, making it easier to identify key trends and sentiments. Additionally, analysis kits tailored for specific research questions can significantly simplify data analysis routines. Furthermore, sentiment analysis can enhance your understanding of user feedback, providing actionable intelligence that drives decision-making. With these tools at your disposal, you will be better positioned to leverage AI-powered insight prioritization, ultimately leading to more informed strategic planning.
- Tool 1: Capabilities and use cases
The first tool we explore for AI-Powered Insight Prioritization is designed to streamline the research analysis process. It enables researchers to efficiently identify relevant insights tailored to specific use cases, significantly reducing the time spent on data interpretation. By leveraging advanced algorithms, it pulls data themes and codes, extracting insights that align closely with the defined research goals.
To effectively utilize this tool, follow these steps:
Define Your Goals: Clearly articulate what you want to achieve with your research. This could include improving user experience or enhancing product features.
Customize Themes: Use default themes or create your own to categorize insights effectively. For example, cluster insights related to challenges or opportunities.
Analyze Insights: The tool provides sentiment analysis and visual mapping of insights, allowing for easier interpretation. This process not only saves time but also ensures that insights are actionable and relevant to your research objectives.
Employing this tool helps in transforming extensive datasets into prioritized insights that drive strategic decision-making.
- Tool 2: Capabilities and use cases
AI-Powered Insight Prioritization is essential for transforming exploratory research into meaningful strategies. Tool 2 offers various capabilities designed to streamline the analysis process, ensuring that researchers can efficiently extract the most pertinent insights. By utilizing predefined themes and coding options, this tool allows users to categorize data effectively, focusing on critical areas such as challenges or user experiences. This categorization not only enhances the clarity of insights but also aligns them with specific research goals.
Additionally, the tool provides sentiment analysis to gauge participant responses, further enriching the understanding of collected data. This feature supports users in refining their focus, allowing for a more targeted approach in their research. With the ability to trace insights back to their original sources, researchers have a transparent view of how conclusions are formed. Ultimately, these capabilities facilitate a more structured way to leverage insights, making them actionable for decision-making processes.
- Tool 3: Capabilities and use cases
AI-Powered Insight Prioritization serves to streamline the often overwhelming process of sifting through vast amounts of exploratory research data. This tool excels in its ability to categorize insights based on pre-defined themes and goals, making it easier to focus on what truly matters. Users can create customized tags for their dataโsuch as risks or challengesโenabling a more tailored analysis that aligns with specific project objectives.
There are several key capabilities to consider. First, AI tools can automate the clustering of insights, organizing them into coherent themes that instantly reveal patterns and trends. Second, these tools provide sentiment analysis, helping researchers gauge emotional responses associated with specific insights. Finally, users have access to analysis kits that ask targeted questions to extract relevant insights, significantly improving decision-making efficiency. By implementing these capabilities, organizations can transform raw data into actionable strategies that are vital for success.
- Tool 4: Capabilities and use cases
AI-Powered Insight Prioritization enables researchers to extract valuable insights efficiently and effectively. This tool streamlines the analysis process by categorizing data into relevant themes and identifying key patterns. Researchers can define specific goals, such as improving user experience or understanding market challenges, allowing the AI to focus on pertinent insights related to these objectives.
The capabilities of this tool include sentiment analysis, which evaluates emotional content within the data. This empowers teams to understand user sentiments better and prioritize areas for improvement. Additionally, by clustering insights into actionable themes, researchers gain clarity in their findings, making it easier to apply them in real-world scenarios. An essential use case for this tool involves enhancing decision-making processes in product development, ultimately leading to higher customer satisfaction and retention. Engaging with AI-Powered Insight Prioritization can drastically reduce time spent on manual data analysis, while simultaneously increasing the relevance and impact of research outcomes.
Best Practices for AI-Powered Insight Prioritization Implementation
Implementing AI-Powered Insight Prioritization involves a series of best practices that streamline how insights are gathered and utilized. First, defining clear research objectives is crucial. This step guides the selection of data sources and helps in identifying key insights relevant to the projectโs goals. Effective prioritization begins with knowing what you aim to achieve inherently, whether itโs understanding user behavior or assessing market trends.
Next, itโs essential to choose the right AI tools that align with these objectives. Not all tools are created equal, and selecting those with capabilities to synthesize large datasets efficiently can enhance the quality of insights. Lastly, integrating these AI solutions within existing research processes allows for smooth workflows and maximizes the utility of gathered data. By following these steps, organizations can tailor their insight prioritization strategies effectively, turning raw data into actionable insights that drive informed decision-making.
Steps to Implementing AI in Insight Prioritization
To successfully implement AI-Powered Insight Prioritization, begin by defining clear research objectives and data sources. This foundational step ensures that the AI tools you select are well-aligned with your specific goals and the nature of your exploratory research. Clearly stated goals will guide the AI algorithms in focusing on relevant insights, thus enhancing the effectiveness of your insights prioritization.
Next, select the appropriate AI tools that suit your research needs. Evaluate different platforms based on their functionalities, ease of integration, and the specific analytical capabilities required for your project. Finally, integrate these AI solutions seamlessly with your existing research processes. This integration promotes smoother data handling and insight extraction, enabling your team to prioritize insights quickly and accurately. By following these steps, organizations will be equipped to harness the power of AI in transforming exploratory research findings into actionable insights.
- Step 1: Defining research objectives and data sources
Defining research objectives is a crucial first step in AI-Powered Insight Prioritization. It sets the foundation for your exploratory research by clarifying what you aim to achieve. Clear objectives guide the direction of your research efforts, ensuring that the insights gathered align with your overarching goals. This precision is vital in filtering through data. When the objectives are established, it becomes easier to identify relevant data sources required to gather the necessary insights.
Choosing the right data sources is just as important as defining research objectives. Reliable data enhances the quality of insights generated by AI. Various data sources, such as surveys, interviews, and focus groups, can provide rich qualitative and quantitative information. Having a well-rounded mix boosts the chances of uncovering meaningful insights, thereby enriching the research process. Keep in mind that the quality of the insights will directly depend on the clarity of your objectives and the reliability of the sources.
- Step 2: Selecting the right AI tools
Selecting the right AI tools is crucial for achieving effective AI-powered insight prioritization. Begin by evaluating your specific needs and the type of exploratory research you are conducting. Tools differ greatly in capabilities, and understanding their unique features can help you streamline your process. Focus on platforms that offer robust data synthesis and analysis, making it easier to derive actionable insights from large amounts of qualitative and quantitative data.
Next, consider user-friendliness and integration capabilities. Itโs essential to choose tools that fit seamlessly within your existing research processes. Look for AI tools that allow customization, enabling you to filter insights that cater to specific themes or topics. The right selection of AI tools will enhance your capability to quickly present findings, ensuring your insights can lead to well-informed decisions.
- Step 3: Integrating AI solutions with existing research processes
Integrating AI solutions with existing research processes involves a thoughtful approach aimed at enhancing the traditional methodologies employed during exploratory research. By leveraging AI-powered tools, researchers can streamline data analysis and uncover insights more efficiently. This integration not only saves time but also improves the accuracy of the insights prioritized, allowing teams to focus on what truly matters.
To successfully merge AI into existing processes, consider the following key steps. First, assess current research methodologies to identify inefficiencies and areas where AI can add value. Then, select appropriate AI tools that align with your specific research goals and data types. Finally, ensure that the AI solutions are seamlessly woven into existing workflows, making it easier for teams to adopt and utilize these advanced capabilities for effective AI-powered insight prioritization. This holistic approach positions organizations to derive the maximum benefit from their research efforts while fostering a culture of innovation and data-driven decision-making.
Measuring the Impact and Continuous Improvement
Measuring the impact of AI-powered insight prioritization is crucial for ensuring the effectiveness of research initiatives. This process begins with evaluating the outcomes of the insights derived from explorations. By analyzing how these insights influence decision-making and customer engagement, organizations can better understand their overall impact.
Continuous improvement is essential in this context. Establishing feedback loops allows teams to gather data on the effectiveness of their strategies. As AI technologies advance, regularly refining approaches ensures that insights remain relevant and actionable. Emphasizing data-driven decision-making will not only enhance insights but also lead to more successful outcomes in the long run. Practical steps include monitoring key performance indicators and soliciting stakeholder feedback to inform ongoing adjustments. In doing so, the commitment to improvement becomes an integral part of the organizational culture.
- Evaluating outcomes and refining strategies
In your journey of AI-Powered Insight Prioritization, evaluating outcomes and refining strategies play a vital role. Start by assessing the effectiveness of your insights against established project goals. Identifying which insights drove successful outcomes provides clarity on your direction. It helps you understand which strategies resonated with your audience and which did not. Regularly reviewing these results ensures that your approach remains fresh and aligned with ongoing business objectives.
Next, implement a feedback loop to continuously enhance your strategies. This involves gathering input from stakeholders on the insights and their applicability. Consider using AI tools to analyze this feedback, enabling a data-driven approach to refining insights. Take note of emerging patterns, and adjust your priorities accordingly. As you continue this cycle, you will cultivate a more robust understanding of insights and their impact, ultimately improving your decision-making processes.
- Importance of feedback loops and ongoing learning
To maximize the benefits of AI-powered insight prioritization, organizations must prioritize feedback loops and ongoing learning. These elements are crucial for refining processes and enhancing data-driven decision-making. Feedback loops allow for the continuous evaluation of insights, enabling teams to understand what is working and what needs adjustment. This iterational process ensures that insights remain relevant and actionable.
Furthermore, ongoing learning fosters a culture of adaptation and growth. As AI tools evolve, so should the methodologies employed to harness their capabilities effectively. Engaging with user feedback and incorporating lessons learned promotes an agile environment where insights are prioritized based on real-time data and shifting trends. Ultimately, embracing these principles not only strengthens the AI-powered insight prioritization process but also ensures organizations remain competitive and agile in a fast-changing landscape.
Conclusion: Maximizing the Benefits of AI-Powered Insight Prioritization
AI-Powered Insight Prioritization revolutionizes how organizations derive value from exploratory research. By utilizing advanced algorithms, teams can quickly sift through vast amounts of data to identify key insights. This efficiency not only saves time but also enhances decision-making by spotlighting the most relevant themes and findings.
To fully maximize these benefits, it is crucial to integrate these insights into a structured reporting process. With AI, reports can be generated almost instantly, offering clear summaries and tailored recommendations. This streamlined approach empowers teams to focus their efforts on actionable insights, ultimately driving strategic initiatives forward. Embracing AI in insight prioritization enables better resource allocation and fosters innovation, paving the way for growth and success in competitive markets.