In a world flooded with information, discerning critical insights from research calls can feel overwhelming. Harnessing AI-Driven Research Monitoring offers a strategic advantage to researchers aiming to identify emerging trends and patterns efficiently. By automating data collection and analysis, AI technologies provide actionable insights faster than traditional methods, enabling researchers to focus on innovation and decision-making.
This advanced monitoring approach enhances the accuracy of findings while ensuring that emerging themes are recognized in real-time. As the complexity of research expands, leveraging AI becomes essential for understanding underlying trends and creating impactful strategies. Embracing these tools can significantly transform how research insights are gathered and utilized, paving the way for a more informed future.
Analyze qualitative data. At Scale.
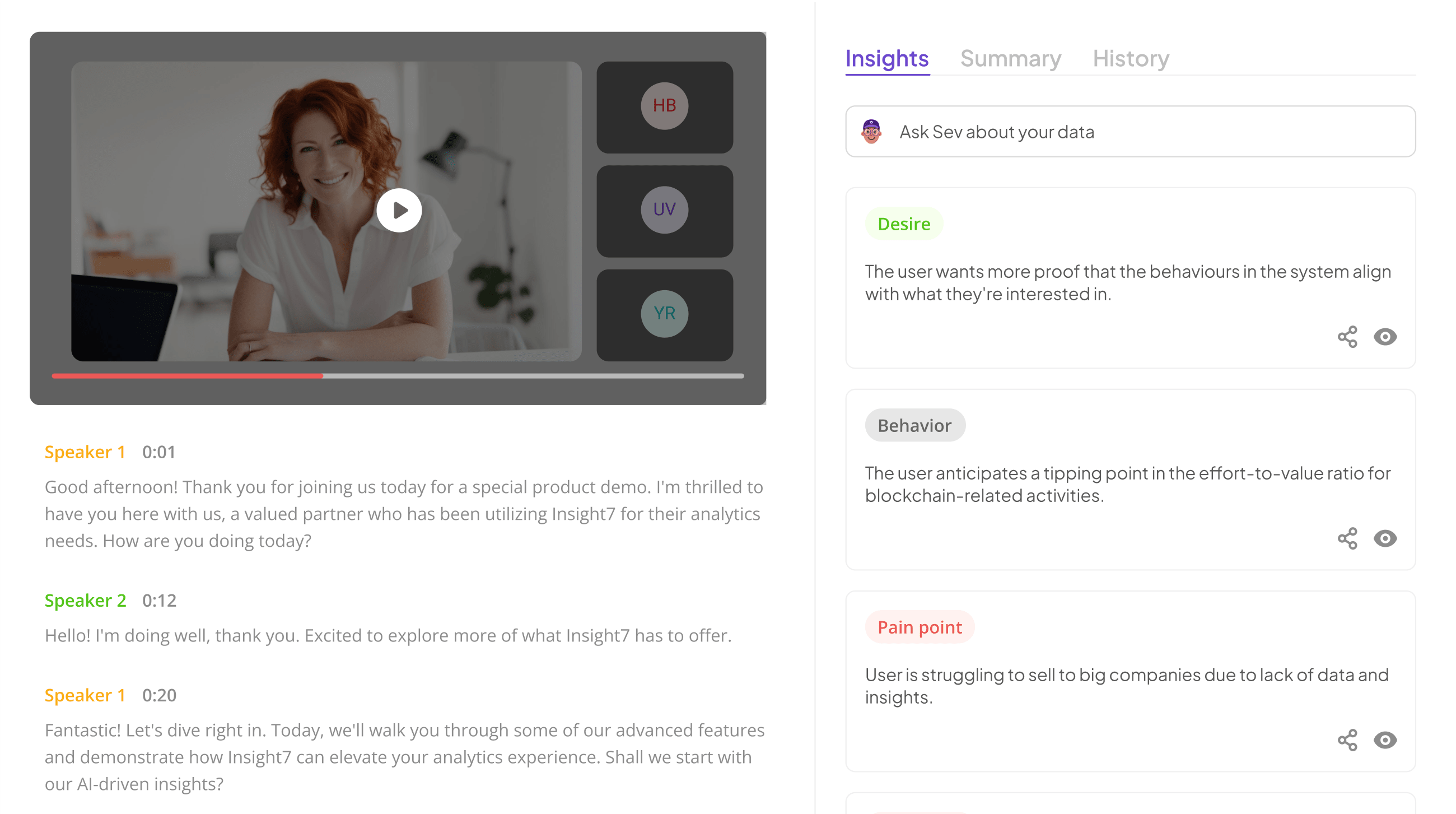
The Role of AI-Driven Research Monitoring in Identifying Trends
AI-Driven Research Monitoring plays a crucial role in recognizing and interpreting research trends. By employing advanced algorithms, it systematically collects and aggregates vast amounts of data from various sources. This technology enables the identification of emerging themes and focal points, ensuring that researchers remain aware of developing areas in their fields. Furthermore, AI refines its analysis by utilizing sophisticated pattern recognition techniques, efficiently pinpointing shifts in research focus.
The implementation of AI-Driven Research Monitoring leads to numerous advantages. It notably enhances both accuracy and efficiency in data handling, simplifying the process of gaining insights from complex datasets. Additionally, the real-time capabilities of AI technologies allow for immediate feedback on recent patterns, facilitating timely decision-making and project adjustments. As researchers strive to keep pace with constantly evolving fields, the integration of AI-driven monitoring systems becomes increasingly essential in uncovering significant trends and guiding future investigations.
Utilizing AI Algorithms to Detect Patterns
AI-driven research monitoring utilizes sophisticated algorithms to identify emerging patterns in data, particularly in qualitative research calls. These algorithms can effectively analyze vast amounts of information gathered from interviews and surveys, revealing insights that may otherwise go unnoticed. By systematically processing data, AI enhances accuracy, minimizes human error, and allows researchers to focus on interpretation rather than mundane data analysis.
To maximize AI's benefits in monitoring research, consider these approaches:
Data Collection and Aggregation: Consolidate various data sources to provide a comprehensive overview. This ensures that no relevant information is overlooked.
Pattern Recognition Techniques: Employ machine learning methods to detect trends over time, enabling swift identification of shifts in research priorities or emerging topics.
Utilizing these techniques makes AI-driven research monitoring not just efficient, but also a crucial tool in today’s fast-paced research environment.
- Data Collection and Aggregation
Effective data collection and aggregation are foundational steps in AI-Driven Research Monitoring. This process involves gathering data from various sources, including interviews and other qualitative research, to ensure a comprehensive view of emerging patterns. By digitizing and centralizing data, researchers can facilitate deeper insights that are not easily attainable through manual methods.
Once collected, the data must be systematically organized. This might involve categorizing responses, identifying themes, and creating visualizations to highlight key findings. By employing AI, researchers can streamline this process significantly, allowing quicker access to important insights. Proper aggregation enables the identification of trends across different studies, providing a valuable resource for informed decision-making. Thus, establishing robust data collection and aggregation methods is crucial in harnessing AI's potential for monitoring research trends effectively.
- Pattern Recognition Techniques
Pattern recognition techniques are essential for effectively utilizing AI-driven research monitoring. These methods enable researchers to process vast amounts of data and extract meaningful insights. By employing advanced algorithms, AI can identify trends, correlations, and anomalies within research calls that may otherwise go unnoticed.
To harness these techniques, researchers can consider the following approaches:
Data Analysis Kits: These tools simplify the analysis process by categorizing data into relevant themes and codes. This ensures that insights align with specific research objectives.
Thematic Clustering: By grouping insights into themes like challenges, risks, or desires, researchers can target their focus strategically. This enables a more profound understanding of participant sentiment and needs.
Combining these techniques not only enhances the accuracy of findings but also accelerates the research process. With AI-driven research monitoring, organizations can stay ahead in identifying emerging research trends effectively.
Benefits of AI in Analyzing Research Calls
AI-Driven Research Monitoring brings significant advantages to the analysis of research calls, fundamentally enhancing the quality of insights gathered. First, the use of AI enhances accuracy and efficiency in data interpretation. Traditional methods can introduce bias and inconsistencies among researchers. In contrast, AI technologies standardize the analysis process, ensuring uniformity in the insights extracted from research calls. This leads to more reliable and trustworthy data representation.
Second, real-time insights are another critical benefit. AI can process vast volumes of information quickly, enabling researchers to gain timely feedback. This rapid analysis helps teams respond to emerging patterns promptly, ultimately fostering better decision-making. By integrating AI-driven tools, organizations can streamline their operations and provide clients with faster, actionable insights, addressing one of the key challenges in research monitoring today. Thus, embracing AI not only mitigates common analytical issues but also positions teams to effectively navigate the evolving landscape of research.
- Enhanced Accuracy and Efficiency
AI-Driven Research Monitoring significantly enhances accuracy and efficiency in managing large datasets. A notable advantage is the elimination of manual analysis, which is often time-consuming and error-prone. By employing AI algorithms, research teams can efficiently process vast amounts of data to uncover meaningful patterns. This leads not only to speed but also to more accurate insights that inform strategic decisions.
Moreover, AI tools enable real-time data analysis, allowing researchers to respond swiftly to emerging trends. When insights are generated promptly, organizations can adapt their strategies accordingly, fostering innovation and growth. The commitment to data privacy further enhances trust in AI-driven processes. Organizations can confidently utilize these technologies, knowing that sensitive information is managed securely. Overall, implementing AI-driven methods streamlines research operations, making them more effective and reliable for future research endeavors.
- Real-time Insights
Real-time insights are pivotal for effective research monitoring. By utilizing AI-driven technologies, organizations can transform vast amounts of raw data into actionable information swiftly. These insights allow stakeholders to adapt their strategies based on current market trends and rapidly changing consumer behaviors.
To maximize the benefits of real-time insights, organizations should focus on two key strategies. First, continuously monitor data from a variety of sources—such as customer feedback, market analysis, and competitive intelligence—to ensure a comprehensive understanding of the landscape. Second, employ advanced AI tools to identify emerging patterns and provide forecasts. This proactive approach not only enhances decision-making but also positions companies to respond quickly to market fluctuations. By embracing real-time insights, businesses can stay ahead of the competition and ensure their strategies are based on the most relevant information available.
Extract insights from interviews, calls, surveys and reviews for insights in minutes
AI Tools for Research Monitoring: Top Solutions
AI-Driven Research Monitoring is reshaping how researchers navigate the complexities of emerging patterns. To enhance the efficiency of monitoring research calls, there are several top solutions available. These tools streamline the data analysis process, ensuring consistency while eliminating biases that often arise from manual efforts. They can expedite report generation, enabling researchers to deliver insights more quickly and accurately, which is essential in fast-paced academic and commercial environments.
Among the leading tools, Scite excels in contextual citation indexing, allowing users to discern how research has been built upon or contested. Iris.ai functions as a powerful research assistant, helping researchers quickly find relevant studies by understanding the context of their inquiries. Dimensions provides comprehensive mapping of research activities, while VOSviewer offers visualization tools that enhance understanding of scientific collaborations. Together, these AI tools contribute to more informed and timely research decisions, aligning with the increasing demands for rapid insights in diverse sectors.
Insight7: Leading the Charge in AI-Driven Research Monitoring
AI-driven research monitoring is transforming how we analyze and respond to emerging patterns in various fields. This innovative approach allows researchers to efficiently sift through vast data sets, pinpointing essential insights that would be challenging to identify manually. By employing sophisticated algorithms, AI enables accurate tracking of specific themes and trends across research calls, providing immediate access to pivotal information that can drive decision-making.
Integrating AI-driven research monitoring enhances not only the efficiency of data collection but also the relevance of findings. Researchers can filter data by speaker or topic, ensuring tailored reports that address unique interests or queries. This capacity for customization allows teams to generate insightful reports in minutes, streamlining the process significantly. Overall, AI-driven research monitoring is not just about extracting data; it's about unlocking the potential for deeper understanding and informed choices in research initiatives.
Other Notable Tools
In the realm of AI-driven research monitoring, several notable tools complement the primary solutions available. First, Scite offers contextual citation indexing, allowing researchers to assess the impact and relevance of studies effectively. This tool provides insights into how papers support or refute claims, enabling users to make informed decisions based on comprehensive analysis.
Next, Iris.ai acts as an AI-powered research assistant, streamlining the literature review process. By intelligently analyzing user inputs, this tool surfaces relevant research papers and abstracts, saving valuable time while enhancing the depth of knowledge available to researchers. Dimensions serves a similar purpose by mapping research activity across various fields, identifying key trends and patterns in real-time. Finally, VOSviewer provides essential visualization capabilities, helping users understand complex scientific relationships and making data interpretation more intuitive. Together, these tools enhance the ability to monitor and analyze emerging patterns effectively in research calls.
- Scite: Contextual Citation Indexing
Contextual citation indexing provides a novel approach to understanding scholarly work. This methodology emphasizes the importance of not just where citations exist but also how they contribute to the research landscape. With AI-driven research monitoring, contextual citation indexing becomes an invaluable tool for detecting emerging patterns in scholarly communication.
By aggregating data from various sources, contextual indexing illuminates the nuanced relationships between different studies. This clarity allows researchers to focus on specific themes or subjects while tracking the lineage of ideas and insights. For instance, when analyzing research calls, scholars can easily isolate references to pressing issues, such as process management or innovative methodologies. This process ensures that users efficiently gather high-quality insights while minimizing bias, ultimately enhancing the value of their research endeavors. The integration of AI in this dimension elevates not just the information accuracy but also the speed at which researchers can adapt to evolving academic discussions.
- Iris.ai: AI-Powered Research Assistant
Utilizing an AI-powered research assistant represents a breakthrough in the realm of AI-driven research monitoring. This tool aids researchers by streamlining the process of analyzing extensive datasets, extracting relevant insights, and identifying emerging patterns in research calls. With its ability to process information rapidly, researchers can save significant time and focus on innovation rather than data management.
This assistant employs advanced algorithms to sift through vast amounts of literature, aggregating and interpreting findings with remarkable accuracy. It also offers valuable contextual understanding, enabling users to navigate complex topics and discern trends that may not be immediately visible. By adopting such AI-driven solutions, researchers gain a strategic advantage, ensuring they remain at the forefront of their respective fields while enhancing the quality and impact of their research outcomes.
- Dimensions: Research Activity Mapping
Understanding the dimensions of Research Activity Mapping is crucial in the landscape of AI-Driven Research Monitoring. This process involves systematically organizing research data to reveal insights that may otherwise remain hidden. By categorizing and analyzing data based on themes, researchers can identify trends and emerging patterns effectively.
In Research Activity Mapping, AI algorithms play a pivotal role. They automatically cluster insights into relevant categories, enabling researchers to focus on specific areas of interest. This method not only simplifies the data analysis but also enhances the accuracy of results. Techniques such as sentiment analysis further provide valuable feedback from participants, capturing their desires and challenges. Consequently, mapping research activities helps researchers adapt and refine their strategies, ensuring alignment with their goals. By harnessing AI in this manner, organizations can make informed decisions that lead to improved outcomes.
- VOSviewer: Visualizing Scientific Landscapes
VOSviewer offers powerful visualization capabilities for mapping scientific research, making it a valuable tool for understanding academic trends. By creating graphical representations of research outputs, such as publications and citations, it enables researchers to see patterns and connections that may not be immediately apparent. This visualization can help users identify emerging focuses within their fields, allowing for informed decisions regarding future research priorities.
In the context of AI-driven research monitoring, VOSviewer simplifies the process of discerning significant trends. Its ability to cluster related publications provides a clearer view of research dynamics over time. Additionally, users can analyze how specific topics evolve and how different research areas interact, thus maximizing insights from various research calls. This comprehensive overview is instrumental for researchers aiming to stay ahead of developments in their domains and effectively respond to changing scientific demands.
Conclusion: The Future of AI-Driven Research Monitoring
As we look to the future of AI-Driven Research Monitoring, the potential for transformative change is significant. Advancements in AI technology promise to enhance our ability to identify emerging research patterns with unprecedented speed and accuracy. By continually leveraging sophisticated algorithms, researchers can access real-time insights that drive informed decision-making and strategic planning.
Moreover, ongoing developments in AI tools will further refine the monitoring process, making it more user-friendly and efficient. As organizations embrace these innovative solutions, AI-Driven Research Monitoring will become indispensable, enabling teams to stay ahead of trends and adapt proactively to evolving research needs. This shift holds the promise of a future where knowledge is not only gathered but actively shaped by intelligent systems.