AI-Powered Comment Analysis is transforming how focus group discussions are examined. Traditional methods often lead to slow and inconsistent insights, creating a challenge for researchers and organizations alike. As focus groups generate a wealth of qualitative data, the need for efficient and accurate analysis has never been greater. By utilizing AI, teams can streamline the coding and comparison of comments, leading to faster and more reliable insights.
This section will delve into the benefits of AI-powered techniques in qualitative research. From identifying patterns and trends to enhancing the accuracy of thematic coding, AI tools are essential for modern research methodologies. By understanding how these advanced technologies analyze focus group comments, you can improve the speed and quality of your research outputs.
Analyze qualitative data. At Scale.
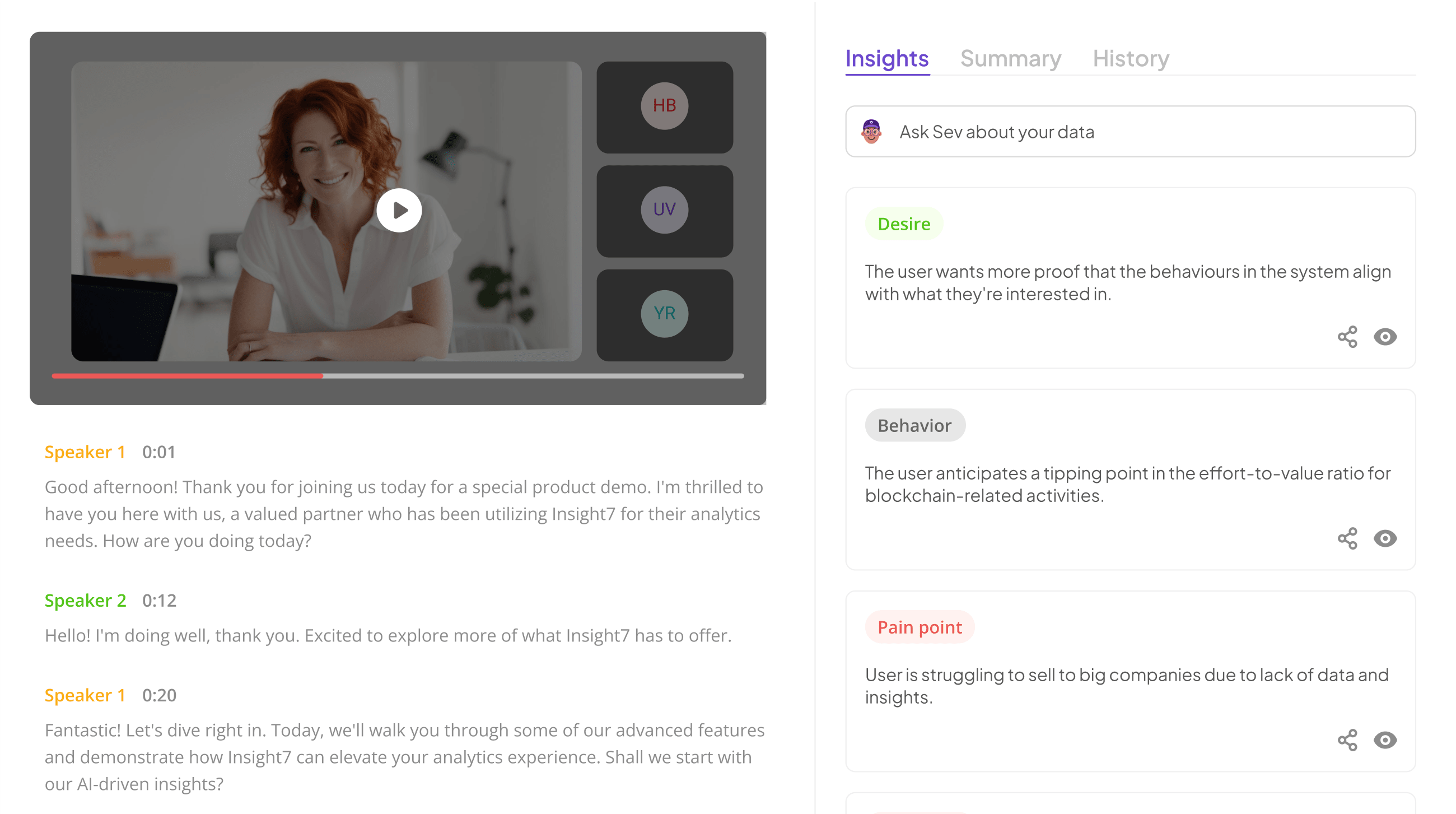
Leveraging AI-Powered Comment Analysis for Effective Data Coding
AI-Powered Comment Analysis revolutionizes the way researchers handle focus group data. By automating the coding process, AI tools can sift through vast amounts of qualitative comments, identifying prevalent themes and insights with remarkable speed. This efficiency allows qualitative researchers to focus their attention on interpreting key findings rather than navigating the tedious task of manual coding.
To make the most of AI in comment analysis, follow a few essential steps. First, gather and clean your focus group data; this ensures the AI works with high-quality input. Next, employ AI algorithms designed to code comments based on patterns and sentiments. These tools not only streamline the analysis but also enhance your ability to unveil connections across diverse perspectives. By integrating AI into your coding processes, you can achieve deeper insights and foster more effective decision-making based on user feedback.
Understanding AI in Qualitative Research
Understanding AI in qualitative research offers exciting possibilities for analyzing focus group discussions. AI-powered comment analysis can transform the way researchers handle large datasets, enhancing both efficiency and accuracy. By employing advanced algorithms, AI tools can identify patterns and themes within vast amounts of qualitative data. This not only streamlines data management but also helps in minimizing biases and inconsistencies often present when multiple researchers analyze the same dataset.
AI plays a crucial role in interpreting human sentiments and experiences captured in focus group conversations. The technology facilitates a faster coding process by automating the classification of comments, allowing researchers to focus on drawing meaningful insights. As a result, teams can generate reports quicker and make informed decisions based on rich qualitative data. Understanding and utilizing AI in qualitative research empowers teams to navigate challenges effectively, paving the way for more dynamic and impactful analyses.
- Definition of AI and its role in data analysis
Artificial Intelligence (AI) refers to the simulation of human intelligence in machines programmed to think and learn like humans. In the realm of data analysis, AI plays a crucial role by automating the processing of large volumes of data, identifying trends, and extracting meaningful insights from complex datasets. AI-powered comment analysis significantly enhances the way focus group comments are managed and interpreted. It enables researchers to synthesize information quickly and accurately, facilitating a deeper understanding of participant sentiments and perspectives.
The application of AI in data analysis also includes coding comments based on thematic relevance and sentiment. This allows for a more nuanced exploration of data, helping analysts to distinguish between different opinions and underlying motivations. AI tools can efficiently categorize comments, filtering them effectively by speaker or theme. As a result, utilizing AI-powered comment analysis transforms the often cumbersome task of data evaluation into a streamlined process where insights can be derived promptly and with greater accuracy.
- Importance of AI in managing large datasets from focus groups
The significance of AI in managing large datasets from focus groups cannot be overstated. As focus groups generate immense amounts of qualitative data, traditional methods of analysis often fall short in both speed and accuracy. AI-Powered Comment Analysis offers a solution to these challenges by automating the coding process, allowing researchers to quickly transform raw data into actionable insights. This efficiency not only saves time but also enhances the ability to discern patterns and themes that may not be immediately visible through manual methods.
Moreover, AI ensures consistency in data interpretation by minimizing human biases that can skew results. By using advanced algorithms, AI can analyze comments in various languages and formats, increasing accessibility for diverse focus groups. As the demand for timely insights grows, leveraging AI-powered tools will enable researchers to meet client needs more effectively while maintaining data integrity. Ultimately, AI serves as a valuable ally in navigating the complexities of qualitative research, fostering a deeper understanding of participant feedback.
Steps to Code Comments Using AI
To effectively harness AI-Powered Comment Analysis for coding focus group comments, begin by collecting and preparing your data. Gathering comments can involve interviews, surveys, or group discussions. Once you have your data, apply techniques such as data cleaning and organizing comments into relevant categories. This preparation is crucial for ensuring that AI algorithms can efficiently analyze the data.
Next, delve into the AI-driven comment coding process. Familiarize yourself with the various AI algorithms specifically designed for text analysis. These algorithms can categorize comments into themes, identify sentiment, and highlight key insights. Implementing this AI process involves uploading your prepared data into appropriate AI tools and running the coding algorithms. By following these steps, you can effectively streamline the coding of focus group comments, allowing for deeper insights and enhanced decision-making.
- Collecting and Preparing Focus Group Data
Collecting and preparing focus group data is a crucial foundation for effective analysis. Begin by ensuring that you gather data in a structured manner, which can include both audio and text formats from participants' discussions. This initial step is essential, as it sets the stage for deeper insights utilizing AI-powered comment analysis. Transcribing audio recordings and organizing textual data helps in identifying key themes and patterns later on.
Once data is collected, preparation involves cleaning and formatting it for analysis. This process may require removing irrelevant information or ensuring consistency in terminology. Moreover, implementing techniques such as tagging and categorization can enhance data usability, making it seamless for AI tools to perform analysis. By thoughtfully collecting and preparing your data, you empower AI to generate valuable insights that inform decision-making processes effectively.
- Methods for gathering data from focus groups
Gathering data from focus groups is vital for understanding participants' insights and experiences. One effective method is through structured interviews, where participants share their thoughts on a specific topic guided by a moderator. This approach can help ensure that each discussion remains focused, allowing for rich dialogues that uncover valuable qualitative insights. Additionally, open-ended questions during surveys can elicit more detailed responses, though they often require more effort to analyze.
After collecting data, using AI-powered comment analysis can streamline the coding process. Transcripts can be uploaded to AI tools to identify common themes and sentiments. This not only saves time but also enhances accuracy by reducing human bias. As discussions can vary widely, implementing AI for comment analysis ensures that all voices are heard and understood, leading to actionable insights that can drive decision-making. AI technology effectively transforms raw data into well-organized information, paving the way for impactful conclusions.
- Data preparation techniques for effective AI analysis
Effective AI analysis begins long before the algorithms are applied; it relies heavily on thorough data preparation techniques. First, ensure that data from focus group comments is gathered systematically. This includes obtaining clear transcripts and exhaustive notes during discussions. Organizing this data into well-defined categories is crucial for successful AI-powered comment analysis.
Next, clean the data to eliminate inconsistencies and irrelevant information. This could involve removing duplicates, correcting typographical errors, and standardizing terminology used across different comments. By structuring and refining the data effectively, you prepare your AI tools to extract valuable insights accurately. Incorporating these data preparation steps will enhance the AI's performance in identifying patterns, themes, and sentiments, resulting in a more robust analysis of focus group feedback. Ultimately, proper data preparation lays the groundwork for insightful, actionable outcomes from AI-powered analyses.
- AI-Driven Comment Coding Process
The AI-driven comment coding process begins with the application of advanced algorithms designed to streamline and automate the coding of qualitative data from focus groups. This approach markedly enhances the efficiency of traditional manual coding, allowing for faster insights and reducing human error or bias. AI algorithms analyze large datasets, identifying themes and patterns that might be overlooked when reviewed manually. As a result, researchers can focus on interpreting the findings rather than getting bogged down in the repetitive task of data coding.
Key steps in this process include collecting and preparing the focus group data, followed by employing AI tools that can categorize and analyze comments effectively. By utilizing these technologies, teams not only expand their capabilities but also ensure a more consistent and objective analysis. AI-Powered Comment Analysis facilitates a thorough examination of data that produces insights to inform decision-making processes, making it an invaluable asset for researchers looking to maximize the value of qualitative research.
- Overview of AI algorithms used in comment coding
AI algorithms play a transformative role in coding comments from focus groups. These algorithms can process vast amounts of qualitative data with remarkable speed and accuracy. One common approach involves natural language processing (NLP), which enables AI to understand context, sentiment, and themes in the comments. This technology analyzes everything from individual phrases to overarching trends, providing valuable insights that inform decision-making in research.
Another significant method is machine learning, which allows the algorithm to learn from previous coding examples and improve over time. By training on labeled data, these algorithms can refine their predictions and adapt to different types of comments. Furthermore, sentiment analysis algorithms specifically gauge emotional tones, aiding researchers in identifying positive, negative, or neutral sentiments in responses. Through AI-powered comment analysis, researchers can obtain a clearer understanding of focus group findings, leading to more effective strategies and recommendations.
- Key steps involved in using AI tools for coding comments
To effectively harness AI-Powered Comment Analysis, it is crucial to follow a series of structured steps that streamline the coding process. Initially, begin by collecting and preparing focus group data. Gather responses through interviews or surveys, ensuring you have clear and concise data. Following data collection, invest time in preparing the dataset; this involves cleaning and structuring the data to make it easily digestible for AI tools.
Once the data is adequately prepared, proceed to the AI-driven comment coding process. Deploy AI algorithms that understand the context and nuances of the comments, facilitating efficient coding. Key elements of this process involve selecting the right AI tools suited for your specific project needs. Regularly check the outcomes generated by the AI, ensuring accuracy and relevance to maintain trust in the findings. Each step fosters a more organized approach to managing qualitative data, enhancing the speed and quality of insights gathered from focus groups.
Extract insights from interviews, calls, surveys and reviews for insights in minutes
Comparing Focus Group Comments with AI-Powered Comment Analysis
When comparing focus group comments with AI-Powered Comment Analysis, the goal is to streamline and enhance qualitative insights. AI diligently processes large volumes of data to identify patterns and trends that manual methods might overlook. By using sophisticated algorithms, AI can conduct sentiment analysis and thematic coding, providing a clearer understanding of participant feedback.
In this approach, comments are not merely coded but compared meaningfully. AI tools can reveal correlations and discrepancies across diverse focus group responses, helping researchers pinpoint significant insights. By continuously analyzing comments, AI can also adapt to changing contexts, ensuring that the analysis remains relevant. The integration of AI-Powered Comment Analysis transforms cumbersome tasks into efficient processes, enabling researchers to derive actionable insights promptly.
Techniques for AI-Powered Comment Comparison
Techniques for AI-Powered Comment Comparison involve various innovative methods that enhance the efficiency and accuracy of qualitative data analysis. AI tools can systematically evaluate focus group comments by identifying patterns and trends within the data. These technologies utilize algorithms to process vast amounts of textual data, making it easier to pinpoint recurring themes and sentiments among participants.
Another crucial technique in AI-Powered Comment Analysis is sentiment analysis, which helps researchers understand the emotional tone behind comments. This approach allows for a more nuanced interpretation of the findings, as it goes beyond mere categorization of responses. Thematic coding emerges as a powerful method as well, enabling users to classify comments into themes that align with research objectives.
By employing these methods, analysts can transform raw focus group comments into actionable insights, ultimately leading to informed decision-making and strategic improvements.
- How AI identifies patterns and trends in comments
AI-Powered Comment Analysis excels in identifying patterns and trends in comments by utilizing sophisticated algorithms to process large datasets. This technology scans through numerous comments, categorizing them into distinct themes based on their content and sentiment. By employing natural language processing, AI can detect recurring phrases or ideas, providing valuable insights into respondent sentiment and behavior.
The ability of AI to analyze trends in real-time empowers researchers to quickly adjust strategies based on findings. With its capability to discern the underlying emotions conveyed in comments, AI not only highlights significant trends but also uncovers nuanced perspectives that may go unnoticed in manual analysis. Consequently, this leads to more informed decision-making and timely interventions. As organizations leverage AI tools, they can enhance their qualitative research, benefiting from a more streamlined analysis process and yielding richer, more actionable insights from focus group comments.
- Role of AI in sentiment analysis and thematic coding
AI-Powered Comment Analysis plays a pivotal role in sentiment analysis and thematic coding, transforming how researchers interpret focus group discussions. By employing sophisticated algorithms, AI identifies emotional tones and major themes within participant comments, simplifying complex qualitative data analysis. This process enables researchers to efficiently categorize insights into relevant themes, which can enhance their understanding of participants' sentiments and perspectives.
The journey of thematic coding is significantly streamlined through AI. First, AI tools analyze large datasets to uncover underlying patterns in sentiments expressed by participants. Next, these tools organize the data into coherent themes, allowing for a more structured analysis. Furthermore, researchers can customize tags based on their specific goals, such as identifying challenges or opportunities. This not only saves time but also guides decision-making based on a deeper comprehension of participant feedback. Ultimately, AI revolutionizes the way insights from focus groups are extracted, offering a comprehensive understanding that drives effective strategies.
Top Tools for AI-Powered Comment Analysis
AI-Powered Comment Analysis offers innovative tools to streamline and enhance the analysis of focus group discussions. Selecting the right tools can significantly improve efficiency, consistency, and depth in qualitative research. For those tasked with decoding and comparing comment data, leveraging specialized software can be transformative. This section highlights some of the best tools in the market, each designed to address unique analytical challenges.
Insight7
This tool excels in synthesizing complex qualitative data, making it ideal for capturing insights quickly and accurately.NVivo
NVivo provides advanced coding features, enabling researchers to categorize comments effectively and conduct detailed thematic analysis.ATLAS.ti
ATLAS.ti is notable for its user-friendly interface that supports collaborative coding, fostering greater consistency across teams.LEXIMANCER
With robust thematic analysis capabilities, LEXIMANCER helps researchers visualize patterns in qualitative data efficiently.MonkeyLearn
This tool utilizes machine learning algorithms to automate sentiment analysis, enhancing the speed and accuracy of coding processes.Quirkos
Quirkos simplifies the management of qualitative data, allowing users to interactively visualize themes and insights from focus group comments.
By applying these tools, researchers can not only save time but also enhance the reliability of their analyses, leading to more actionable insights from focus group discussions.
- Insight7
AI-Powered Comment Analysis stands at the forefront of transforming how focus group feedback is interpreted. By utilizing advanced algorithms, this technology excels in identifying patterns within qualitative data, which conventional methods may overlook. AI helps streamline the lengthy process of manually coding comments, allowing researchers to digest vast amounts of data quickly and effectively.
One key aspect of AI-powered analysis is its capability to conduct sentiment analysis. This process assesses emotional undertones in comments, revealing valuable insights that inform decision-making. Furthermore, AI algorithms can perform thematic coding, categorizing comments into relevant themes, making it easier to compare responses across different groups. Ultimately, these insights empower businesses to refine their strategies, enhance customer engagement, and foster a deeper understanding of participant perspectives. By embracing AI-powered comment analysis, organizations can position themselves to harness feedback for lasting impact.
- Unique features and benefits of using Insight7
One unique feature of using AI-Powered Comment Analysis is its exceptional capability to handle large volumes of qualitative data. This technology eliminates the tedious task of manual coding, transforming time-consuming analysis into efficient processes. With advanced algorithms, AI can quickly identify patterns and categorize comments, allowing researchers to focus on deeper insights rather than getting bogged down in routine tasks.
Another significant benefit is the ability to provide real-time sentiment analysis. This feature equips users with immediate feedback on participant reactions, which is crucial for adapting strategies and messaging effectively. Moreover, AI-Powered Comment Analysis can enhance accuracy by minimizing human errors in coding and interpreting comments. This leads to more reliable data outcomes, ultimately supporting better decision-making. By adopting this technology, organizations can significantly streamline their qualitative research processes, freeing up resources for more strategic initiatives.
- NVivo
NVivo is a powerful tool designed to enhance the process of AI-powered comment analysis, particularly in qualitative research settings like focus groups. This software offers features that enable researchers to systematically code and analyze comments, uncovering insights that may not be immediately apparent. With NVivo, users can manage large datasets, linking comments to specific themes or categories, which is crucial for effective analysis.
To effectively use NVivo, researchers can follow a few key steps. First, collecting and preparing focus group data is essential; this includes ensuring that the data is organized and formatted correctly for analysis. Next, the AI-driven coding process begins, where algorithms help in categorizing comments based on keywords and themes. By utilizing NVivo, researchers can leverage these capabilities to visualize data and facilitate better comparisons between focus group responses, ultimately paving the way for informed decision-making.
- Capabilities of NVivo in AI-assisted comment analysis
NVivo offers significant capabilities for AI-powered comment analysis, transforming how researchers approach qualitative data. Its advanced functionalities enable users to automate the coding of focus group comments efficiently. By leveraging AI technology, NVivo effectively identifies themes, patterns, and sentiments within extensive datasets, which is crucial for thorough analysis.
One of NVivo's standout features is its ability to facilitate data visualization. Users can create various visual representations, such as models and maps, to help interpret complex data. This aids in revealing the interconnectedness of ideas expressed in focus groups. Furthermore, NVivo supports collaboration, allowing teams to work together seamlessly, share insights, and refine coding strategies. Such capabilities ultimately enhance the richness of qualitative analysis and provide deeper insights into participant feedback, making it an invaluable tool for researchers in various fields.
- ATLAS.ti
In qualitative research, ATLAS.ti serves as a powerful tool for AI-Powered Comment Analysis, facilitating the coding and comparison of focus group comments. This software provides intuitive features that streamline the process of organizing and analyzing qualitative data. By incorporating AI technologies, ATLAS.ti enhances the ability to derive meaningful insights from large volumes of text, allowing researchers to code comments effectively.
The key functions of ATLAS.ti include its innovative coding interface, which enables researchers to easily tag and segment comments by themes or sentiments. Furthermore, its visualization tools, such as network views, help in comparing comments across different focus groups. This capability not only aids in identifying patterns but also enriches the overall analysis. Ultimately, leveraging ATLAS.ti can significantly improve the efficiency and depth of AI-powered comment analysis, transforming raw data into actionable insights.
- How ATLAS.ti aids in coding and comparing comments
ATLAS.ti plays a pivotal role in coding and comparing focus group comments by harnessing powerful AI-driven techniques. With streamlined data analysis capabilities, it enables researchers to extract meaningful insights swiftly. Users can easily define their coding themes or opt for predefined ones, making the task both flexible and efficient. Once the themes are set, sentiments are analyzed, clustering insights to reveal patterns related to specific topics. This process not only saves valuable time but also ensures that the analysis remains relevant to the objectives outlined for the study.
Moreover, the platform allows for comprehensive comparison across various comments, supporting the identification of trends and inconsistencies. Detailed visualization features enhance data interpretation, allowing researchers to correlate insights directly with participant quotes. By utilizing such sophisticated tools, ATLAS.ti empowers users to conduct rich, AI-Powered Comment Analysis, driving informed decisions based on robust qualitative data. Researchers, therefore, enjoy a structured and insightful experience, streamlining the often complex workflow of focus group analysis.
- LEXIMANCER
LEXIMANCER is an innovative tool designed for thematic analysis, particularly beneficial in the context of AI-Powered Comment Analysis. With its focus on identifying themes and patterns within qualitative data, LEXIMANCER efficiently processes vast amounts of focus group comments. This tool uses an intuitive interface that simplifies the extraction and visualization of significant themes, thus facilitating deeper insights for researchers and analysts.
A key aspect of LEXIMANCER is its ability to automatically code and categorize comments, which enhances the speed and accuracy of analysis. Users can input qualitative data, and the software will generate a comprehensive overview of themes, supported by graphical representations. This visual approach not only assists in understanding complex data but also aids in communicating findings effectively to stakeholders. By harnessing LEXIMANCER's capabilities, researchers can transform raw comments into actionable insights, driving informed decision-making in various projects.
- Special features of LEXIMANCER for thematic analysis
LEXIMANCER offers powerful tools for thematic analysis that significantly enhance the AI-powered comment analysis process. One of its standout features is the ability to create thematic maps, which visually represent the relationships between various topics within focus group comments. This feature not only simplifies the identification of key themes but also highlights emerging patterns that may otherwise go unnoticed.
Additionally, LEXIMANCER allows users to customize their coding process. You can define your own themes, helping to tailor the analysis to specific project goals, such as understanding participant sentiments on collaboration tools. The software also supports sentiment analysis, enabling a nuanced interpretation of how participants feel about different aspects of the discussion. By mapping quotes directly to themes, you gain concrete evidence of insights, streamlining the process of developing actionable recommendations from focus group data.
- MonkeyLearn
AI-Powered Comment Analysis can be significantly enhanced using advanced tools designed for qualitative data. One such tool allows users to effortlessly analyze focus group transcripts by providing an intuitive interface. Users can easily import various data formats, whether from raw transcripts or video transcriptions, centralizing their analysis in a collaborative workspace.
Within projects, valuable insights emerge through techniques that cluster related comments, leading to deeper thematic understanding. Users can pull specific themes, quotes, and insights easily. Additionally, the platform features pre-made kits that streamline the analysis process, offering recommendations tailored to different research needs. This proactive approach allows researchers to extract meaningful patterns and trends quickly, significantly improving data-driven decision-making. Whether you’re looking into marketing, product development, or customer feedback, this tool serves as a powerful ally in transforming comments into actionable insights.
- Utilization of MonkeyLearn for machine learning comment analysis
Utilizing MonkeyLearn for machine learning comment analysis greatly enhances the evaluation of focus group feedback. This AI-powered comment analysis tool employs sophisticated algorithms to automate the coding of qualitative data. By efficiently processing large volumes of text, it identifies key themes and sentiments, providing insights that are crucial for decision-making.
One of the main advantages of using MonkeyLearn is its ability to streamline the comment coding process. Users can customize models to suit specific analysis needs, making it easier to extract actionable insights. Additionally, the platform supports sentiment analysis, allowing researchers to gauge the emotional tone of comments. This functionality not only saves time but also helps teams focus on strategic improvements based on data-driven findings. As focus groups generate a wealth of information, leveraging such tools becomes essential for organizations aiming to utilize insights effectively.
- Quirkos
In the realm of AI-Powered Comment Analysis, Quirkos stands out as a user-friendly tool designed to streamline qualitative data management. This software simplifies the process of coding and organizing focus group comments. Unlike more complex programs, it prioritizes ease of use, ensuring that even those without technical expertise can effectively analyze their data. With Quirkos, users can visualize responses, spot trends, and create insightful reports, all while maintaining an intuitive interface.
The strength of Quirkos lies in its ability to handle large datasets effortlessly. Users can easily group comments by themes, enabling a better understanding of participant perspectives. The program also facilitates collaboration, allowing teams to share insights and work collectively towards conclusions. Ultimately, Quirkos empowers researchers to extract actionable insights from their focus group data, enhancing the overall effectiveness of qualitative research.
- Advantages of Quirkos in qualitative data management
In the realm of qualitative data management, the advantages of Quirkos are particularly notable. This platform enables flexible and intuitive coding of qualitative data, making it easier to manage extensive comment datasets efficiently. The drag-and-drop interface allows researchers to categorize comments seamlessly, enhancing the organization of insights gathered from focus groups. This simplicity allows for quick adjustments, accommodating evolving themes and emerging patterns within the data.
Moreover, Quirkos features an analysis grid that empowers users to ask multiple questions across various data files simultaneously. This capability not only streamlines the data analysis process but also enhances the depth of AI-Powered Comment Analysis. By summarizing data and generating insights in real-time, Quirkos transforms large amounts of qualitative data into actionable information, significantly reducing the time and effort required for thorough analysis. Overall, Quirkos stands out as an invaluable tool for researchers looking to harness the full potential of qualitative insights from focus group comments.
Conclusion on AI-Powered Comment Analysis for Qualitative Insights
AI-Powered Comment Analysis has revolutionized how qualitative insights are derived from focus group feedback. By automating the coding and comparison of comments, organizations can gain meaningful insights more efficiently than traditional methods allow. This technology reduces manual workloads, minimizes bias, and ensures consistency across analyses, leading to quicker, more reliable results.
As businesses strive for swift and actionable insights, adopting AI-powered tools is an invaluable solution. These tools not only enhance the analysis process but also empower teams to focus on strategic decision-making rather than data processing. Ultimately, this approach transforms how qualitative data is interpreted, paving the way for more informed business strategies.