AI-Powered Response Clustering has revolutionized how researchers analyze qualitative data from focus groups. Traditional methods of manually sifting through participant responses can be time-consuming and prone to bias. In today’s fast-paced environment, stakeholders are demanding quicker insights and more precise analysis. This is where AI steps in, facilitating efficient clustering of responses into meaningful themes and patterns that would otherwise be challenging to identify.
The potential of AI in focus group research goes beyond mere data handling; it enables deeper understanding by filtering out noise and highlighting significant trends. As researchers harness AI tools for this purpose, they can not only speed up data processing but also enhance the accuracy and richness of the insights generated. This shift towards AI-Powered Response Clustering is not just a trend; it’s transforming how organizations make informed decisions based on direct consumer feedback.
Analyze & Evaluate Calls. At Scale.
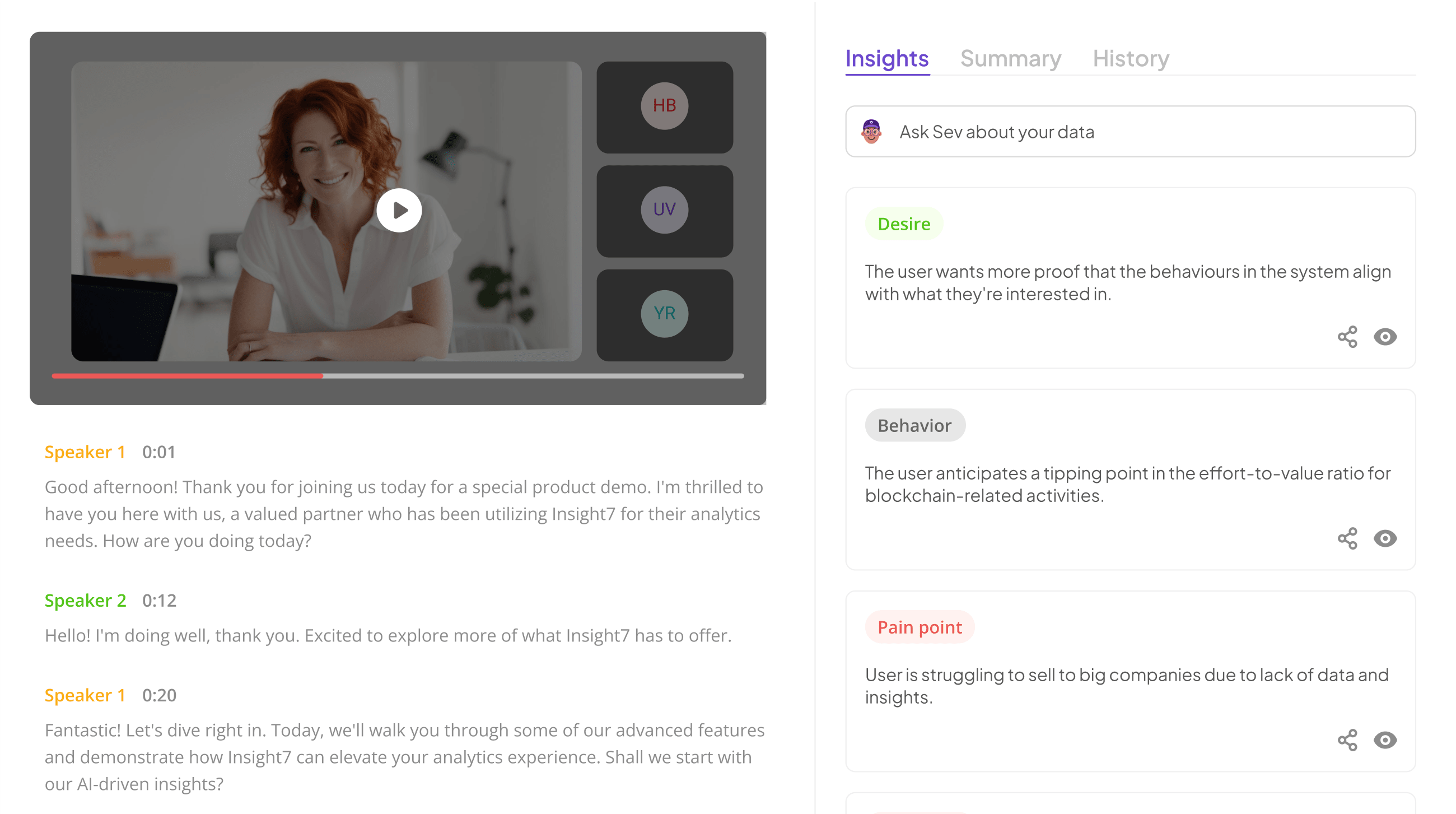
Understanding the Basics of AI-Powered Response Clustering
AI-Powered Response Clustering involves the application of artificial intelligence to organize and group qualitative responses from focus groups. This process streamlines data analysis by identifying patterns or themes within the collected responses. By utilizing AI algorithms, researchers can efficiently group similar responses, making it easier to derive meaningful insights that might otherwise go unnoticed.
Understanding the basics of this technique begins with recognizing its role in enhancing data interpretation. Once interviews or surveys are collected, AI can swiftly categorize and aggregate responses. This clustering helps researchers identify key themes, allowing for in-depth analysis and more effective communication of findings. In focus group research, employing AI-powered clustering elevates the quality of insights, proving to be invaluable as researchers strive for precision and speed in their analysis.
What is AI-Powered Response Clustering?
AI-Powered Response Clustering utilizes advanced algorithms to organize and analyze qualitative data from focus group discussions. By processing participant responses, this technology identifies patterns and similarities within the information, clustering responses into meaningful categories. This clustering allows researchers to efficiently synthesize insights, revealing underlying themes that might otherwise be overlooked in traditional analysis.
The process begins with feeding raw data into AI systems, which then deploy machine learning techniques to group similar responses. This not only fosters a clearer understanding of group sentiments but also enhances decision-making. As researchers navigate the complexities of qualitative data, AI-Powered Response Clustering emerges as an invaluable tool, streamlining the analysis process while providing a comprehensive overview of participant feedback. Through efficient clustering, researchers can focus their efforts on actionable insights, ultimately improving research outcomes and driving informed strategies.
Importance of AI-Powered Response Clustering in Focus Group Research
AI-Powered Response Clustering is pivotal in focus group research, as it enables researchers to extract meaningful insights from vast amounts of data. By utilizing advanced algorithms, this approach organizes diverse participant feedback into coherent themes. This not only streamlines data analysis but also enhances the overall understanding of group sentiment and behaviors.
The importance of AI-Powered Response Clustering extends beyond mere organization. First, it allows for faster identification of trends, which is crucial in today’s competitive market landscape. Second, it mitigates bias by providing an objective framework for analysis, ensuring that all voices in a focus group are considered fairly. Lastly, as the demand for quick turnarounds in research increases, AI technologies facilitate more efficient workflow, enabling faster access to actionable insights. Embracing AI in focus group research ultimately empowers organizations to make informed decisions that drive innovation and success.
Extract insights from interviews, calls, surveys and reviews for insights in minutes
How to Implement AI-Powered Response Clustering
To implement AI-Powered Response Clustering, organizations need to follow a structured approach to ensure effectiveness and accuracy in their focus group analysis. First, data collection and preparation are crucial. Gather responses from focus group participants and clean the data to remove inconsistencies and irrelevant information. This cleaned dataset serves as the foundation for the subsequent steps in the clustering process.
Next, selecting the right AI tools is an essential decision. Tools like MonkeyLearn, IBM Watson, and Google Cloud AI offer various capabilities for text analysis and clustering. After choosing an appropriate tool, it’s time to set up AI algorithms tailored for clustering tasks. Establish parameters that align with your research objectives, such as the number of clusters or specific keywords. Finally, analyze and interpret the results. This involves reviewing the clustered responses, understanding patterns, and deriving insights that can inform future decisions and strategies. Adopting this methodical approach will elevate the quality of insights gained from focus group research through AI-Powered Response Clustering.
Step 1: Data Collection and Preparation
In the initial phase of utilizing AI-Powered Response Clustering, data collection and preparation are crucial steps that set the foundation for effective analysis. Begin by gathering responses from focus group discussions. This data can originate from various sources such as interviews, surveys, and social media feedback. Ensure that the collected data is comprehensive, capturing diverse perspectives that provide a holistic view of the topic at hand.
After gathering the data, it's essential to organize and clean it systematically. Remove any irrelevant or redundant information to enhance clarity and accuracy. Additionally, consider categorizing responses based on specific themes or topics. This preparation will significantly improve the efficiency of the AI algorithms used for clustering. A well-prepared dataset allows AI tools to identify patterns and extract meaningful insights seamlessly, ultimately enriching the overall research process.
Step 2: Selecting the Right AI Tools
Selecting the right AI tools is crucial for effective AI-Powered Response Clustering. Various options exist, each offering unique features that can significantly impact the clustering process. First, consider the ease of data integration. You need tools that handle different data formats seamlessly, ensuring a smooth workflow. Second, prioritize user-friendliness and intuitive interfaces. This facilitates quick adoption by team members and allows for efficient analysis.
Next, evaluate the AI capabilities of each tool. The most effective options use advanced algorithms to accurately group similar responses based on themes and sentiments. It's also vital to look for tools that provide insightful reports and visualization options. These features help present findings in an understandable manner. By carefully evaluating these aspects, you can choose the right AI tools to enhance your focus group research, ultimately benefiting from a streamlined and insightful clustering process.
- insight7
AI-Powered Response Clustering enhances the efficiency of analyzing data from focus group research. By automating the clustering process, researchers can focus on strategic insights rather than being bogged down by tedious manual analysis. Implementing AI algorithms allows for the identification of patterns within responses, which can lead to more meaningful conclusions and actionable outcomes. Accurate clustering delivers a clearer understanding of participant sentiments, enabling researchers to tailor strategies that resonate with their audience.
To effectively leverage AI-Powered Response Clustering, researchers should follow these essential steps:
- Data Collection and Preparation: Gather qualitative data, such as transcripts from interviews or discussions, and prepare it for analysis.
- Selecting the Right AI Tools: Choose appropriate AI platforms that cater to your specific research needs, ensuring they can handle necessary data volume and complexity.
- Setting Up AI Algorithms for Clustering: Implement algorithms that efficiently group similar responses to reveal insights.
- Analysis and Interpretation of Results: Review the clustered data to extract actionable insights and guide decision-making processes.
Utilizing these strategies will enhance your research capabilities and transform how insights are derived from focus group data.
- MonkeyLearn
AI-Powered Response Clustering provides a powerful tool to refine focus group research. With the right methodology and technology, users can now streamline vast amounts of qualitative data into meaningful clusters. By employing AI, researchers save valuable time that can be redirected toward deeper analysis and insights.
When using the software, it is crucial to begin with clean, well-structured data inputs. The clustering tools adapt to various data formats, allowing flexibility in how responses are grouped based on various themes or sentiments. This functionality empowers researchers to identify trends and patterns that might go unnoticed. Furthermore, as researchers adjust the parameters within the AI tool, they can fine-tune clustering results, ensuring accuracy and relevance in derived insights.
AI-Powered Response Clustering not only enhances the efficiency of data analysis but also leads to more informed decision-making based on user feedback.
- IBM Watson
IBM Watson has emerged as a powerful tool for AI-powered response clustering, offering a unique approach to analyzing focus group data. By utilizing advanced natural language processing, it helps researchers identify patterns and themes within qualitative responses. This capability ensures that critical insights are drawn from the often complex and varied feedback provided by participants.
Incorporating IBM Watson into your research process allows for quick processing of large sets of data, enabling you to uncover nuanced sentiments and trends that may not be immediately apparent. The AI can cluster similar responses, making it easier to analyze diverse viewpoints and derive actionable insights. Thus, adopting AI-powered response clustering transforms how focus group research can capture depth and detail, leading to more informed decisions and strategies.
- RapidMiner
RapidMiner is a powerful tool designed to facilitate AI-powered response clustering, making it an excellent choice for focus group research. It offers an intuitive interface that allows researchers to manage large datasets efficiently. As the need for understanding nuanced consumer insights increases, RapidMiner stands out as a resource for processing qualitative data, such as transcripts from focus group discussions.
This platform equips users with sophisticated algorithms that automate the clustering process, significantly reducing time spent on data analysis. By employing various clustering techniques, RapidMiner enables the identification of patterns and themes in participants' responses. These insights can consequently inform strategic decision-making. Researchers can utilize RapidMiner to enhance their analysis capabilities, ultimately leading to richer, evidence-based recommendations grounded in consumer feedback. This innovative approach to data interpretation ensures that actionable insights drive future research and business outcomes.
- Google Cloud AI
Google Cloud AI offers powerful solutions for clustering diverse responses in focus group research. By harnessing AI-powered response clustering, researchers can systematically organize qualitative data, making it easier to derive meaningful insights. This tool utilizes advanced machine learning algorithms to identify patterns and relationships among participant responses in an efficient manner.
To implement AI-powered response clustering effectively, researchers should first focus on their data collection and preparation stages. Once the data is organized, researchers can utilize the capabilities of Cloud AI for clustering analysis. This process enhances the clarity of findings, allowing stakeholders to visualize trends and sentiments across participant feedback. Embracing this technology furthers the goal of delivering deeper insights from focus groups while significantly improving the speed of analysis and interpretation. Through this integration, businesses can transform raw data into actionable strategies that align closely with consumer needs and expectations.
Step 3: Setting Up AI Algorithms for Clustering
In Step 3: Setting Up AI Algorithms for Clustering, the focus shifts to utilizing AI-powered algorithms to group the responses effectively. This stage involves selecting appropriate clustering techniques, such as K-means, hierarchical clustering, or DBSCAN, depending on your dataset's unique characteristics. It's essential to understand the nature of your responses and the specific insights you seek before choosing an algorithm. Each technique has its strengths and ideal use cases, affecting the clustering's accuracy and relevance.
Once the algorithm is selected, configuring it for optimal performance is crucial. This may involve setting parameters such as the number of clusters or the distance metric to be used. Training the model on a pre-processed dataset helps in segmenting the responses meaningfully. After running the clustering process, evaluate the results by interpreting the data patterns and themes that emerge. Doing so allows for a comprehensive analysis of the focus group insights, enabling you to leverage the full potential of AI-powered response clustering.
Step 4: Analysis and Interpretation of Results
In the analysis and interpretation of results, AI-Powered Response Clustering plays a crucial role in transforming raw data into actionable insights. Upon gathering focus group responses, the next step is to systematically analyze these inputs. Begin by organizing the data into themes that reflect common responses. This thematic coding helps streamline the process of identifying key trends and insights drawn from participant feedback. By using AI algorithms, you can efficiently group similar responses, making it easier to extract significant observations.
Once you have clustered the responses, the interpretation phase begins. This involves examining each theme's significance and understanding how they relate to your research goals. For instance, if a cluster reveals insights regarding collaboration tools, it’s essential to consider how these findings can inform strategy or improve user experience. Ultimately, the goal is to transform the clustered data into strategic recommendations that enhance research outcomes and inform decision-making.
Conclusion: Benefits of AI-Powered Response Clustering in Enhancing Research Outcomes
AI-Powered Response Clustering offers transformative advantages that significantly enhance research outcomes. By effectively organizing qualitative data from focus group discussions, this approach allows researchers to identify key themes and insights quickly. This streamlined process leads to faster decision-making, enabling researchers to respond to client needs more efficiently.
Moreover, the ability to aggregate information from multiple sources enhances the depth of understanding. Researchers can visualize patterns in responses, revealing nuanced perspectives that might otherwise go unnoticed. Ultimately, adopting AI-Powered Response Clustering not only improves research efficiency but also enriches the quality of insights derived, leading to more informed strategies and actions.