Automated Response Clustering transforms the way we analyze qualitative data by leveraging the power of artificial intelligence. In an era where insights are paramount, businesses face the daunting task of sorting through vast amounts of qualitative responses. Manual analysis can be time-consuming, prone to biases, and inconsistent, often delaying critical decision-making processes.
By employing automated response clustering, organizations can efficiently group similar responses, revealing valuable trends and themes without the exhaustive manual effort. This approach not only enhances the accuracy of insights but also accelerates the reporting timeline, allowing teams to focus on what truly matters—transforming data into strategic action. As we delve deeper into the methodologies and tools available, understanding the fundamentals of this innovative technique becomes essential for harnessing its full potential.
In todays data-driven world, businesses and researchers are often faced with the challenge of analyzing large volumes of qualitative data. Automating the clustering of qualitative responses can provide valuable insights and save time. In this blog post, we will explore how AI can effectively automate the clustering of qualitative responses to improve decision-making processes.
In today's data-driven world, the sheer volume of qualitative data presents a significant challenge for businesses and researchers alike. Manually sorting through interviews, open-ended survey responses, and other qualitative feedback can be a labor-intensive and time-consuming process. As a result, organizations often struggle to uncover critical insights promptly, which can hinder effective decision-making.
Automated Response Clustering offers a compelling solution to this issue by using artificial intelligence to streamline the analysis of qualitative responses. By leveraging natural language processing (NLP) and machine learning algorithms, AI can efficiently group similar responses, providing a clear overview of emerging themes and trends. This automation not only saves time but also reduces the inconsistencies that often arise in manual analysis, empowering teams to focus on interpreting insights rather than getting bogged down in data sorting. As we delve further, we'll detail how AI can transform your approach to qualitative data clustering, ultimately enhancing strategic decision-making processes.
Analyze qualitative data. At Scale.
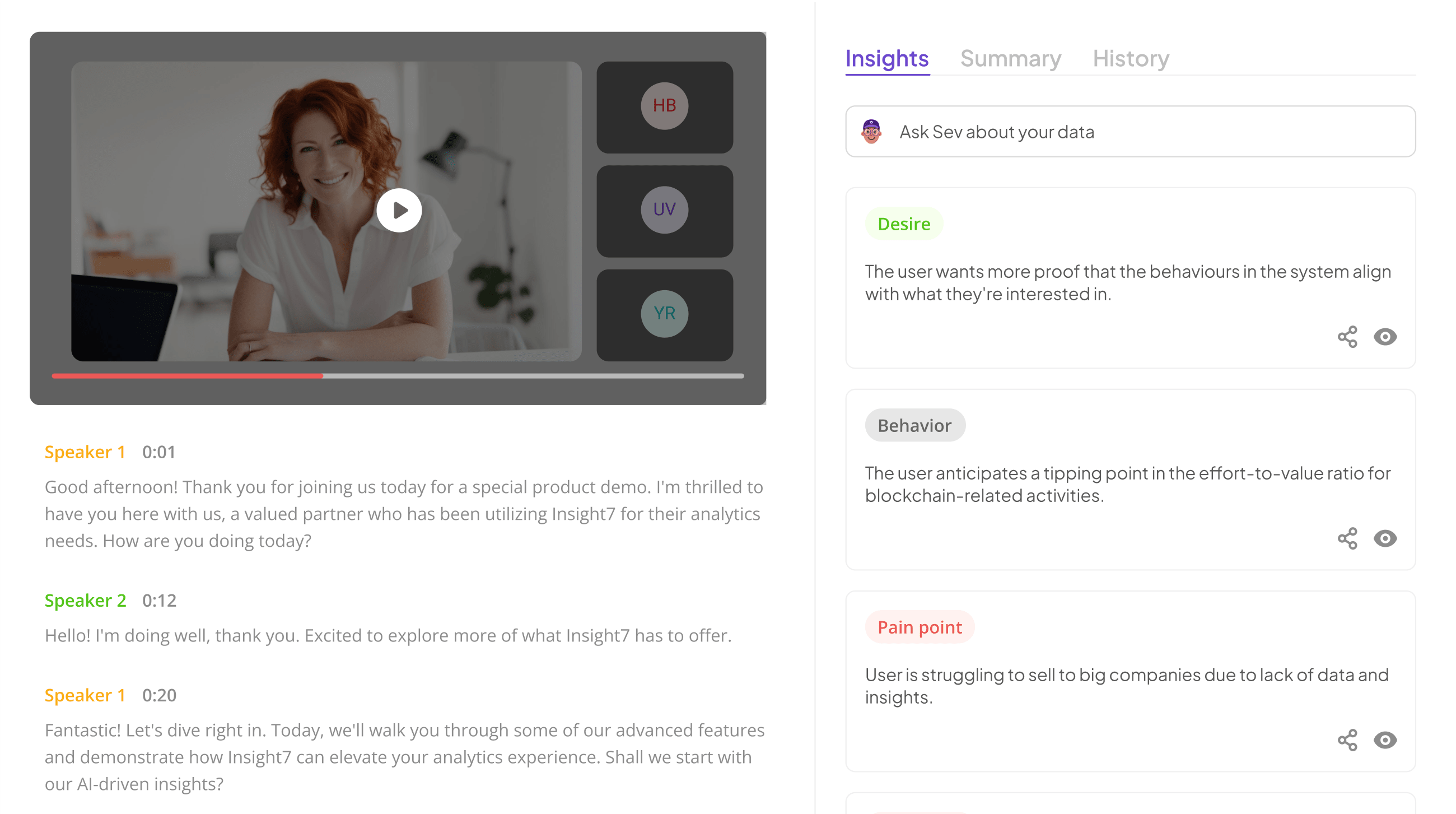
Understanding Automated Response Clustering
Understanding Automated Response Clustering is crucial for anyone looking to enhance their qualitative data analysis using AI. At its core, this technique employs advanced algorithms to sort similar responses automatically, eliminating the time-consuming and biased processes often linked with manual analysis. By automating the clustering, teams can identify patterns and trends swiftly, allowing for quicker insights and decision-making.
Moreover, the role of AI in this context cannot be overstated. Utilizing natural language processing (NLP) and machine learning, automated response clustering interprets and organizes qualitative data effectively. This synergy not only enhances accuracy but also streamlines the overall workflow, making it more efficient. With a responsive AI system in place, organizations can focus on evaluating insights rather than getting bogged down by the complexities of data handling. Understanding this revolutionary approach equips you to leverage the full advantages of AI in qualitative analysis.
To harness the full potential of AI in clustering qualitative responses, its essential to grasp the fundamentals.
Understanding the fundamentals of automated response clustering is crucial for maximizing the effectiveness of AI in this field. At its core, this involves using sophisticated algorithms to group qualitative responses based on shared characteristics. By grasping these foundational concepts, researchers can streamline their analysis and generate insights with greater efficiency and consistency.
Key principles to focus on include the significance of data quality, the importance of selecting the right analytical tools, and the integration of natural language processing technologies. Ensuring clean, well-structured data serves as the bedrock for any automated clustering effort. Additionally, familiarizing yourself with various AI tools capable of handling qualitative data—like those mentioned in the previous section—will enhance your analytical capabilities. Ultimately, mastering these fundamentals not only increases the accuracy of insights but also significantly reduces the time spent on manual analysis, enabling teams to respond faster to the changing landscape of consumer feedback.
What is Automated Response Clustering?
Automated Response Clustering is a transformative technique that utilizes AI to group qualitative responses based on similarities. This process significantly reduces the need for manual categorization, enabling quicker and more efficient analysis. By employing advanced algorithms, businesses can effortlessly identify patterns and emerging trends in their qualitative data, which is particularly valuable in decision-making scenarios.
In essence, Automated Response Clustering streamlines the way we handle extensive qualitative feedback, helping organizations to extract meaningful insights from large datasets. As traditional methods often require significant time and human resources, automating this process not only enhances accuracy but also improves the overall quality of insights derived from the data. Leveraging such technology allows teams to focus more on strategic initiatives, ultimately driving better outcomes in research and business performance.
Automated Response Clustering refers to using AI algorithms to group qualitative responses based on similarities without manual intervention. This technique enables efficient and consistent categorization, helping to identify emerging trends and insights.
Automated Response Clustering allows for the grouping of qualitative data using AI algorithms, which significantly streamlines the analysis process. By identifying patterns and similarities within responses, this technique eliminates the need for manual categorization, enhancing efficiency and consistency. Businesses and researchers can quickly pinpoint emerging trends, leading to actionable insights that inform decision-making.
The process begins with data preparation, ensuring that insights are based on clean and organized information. After setting up the data, choosing effective tools becomes crucial for successful clustering. Popular options include platforms that integrate natural language processing and machine learning capabilities, enabling deeper analysis and understanding. Once effectively executed, the AI algorithms cluster qualitative responses, offering fresh perspectives and highlighting key themes. In this way, Automated Response Clustering transforms the handling of qualitative data into a faster, more effective, and insightful endeavor.
How Does AI Enable Automated Response Clustering?
AI enables automated response clustering through advanced techniques such as natural language processing (NLP) and machine learning. These technologies work hand in hand to decipher the nuances of qualitative data, allowing for quick and accurate categorization. By utilizing machine learning algorithms, AI can identify patterns and similarities in responses, grouping them into meaningful clusters without the need for human intervention. This process not only enhances efficiency but also delivers consistent insights, driving better decision-making.
The methodology consists of several key components. First, NLP transforms raw text data into a format that machines can understand, extracting essential features like sentiment and context. Second, machine learning algorithms, trained on vast datasets, learn to identify similarities among responses. Together, these elements enable automated response clustering, empowering organizations to unlock valuable insights from qualitative data swiftly. This integration marks a significant advancement for businesses aiming to leverage qualitative insights effectively.
AI leverages natural language processing (NLP) and machine learning algorithms to interpret, categorize, and cluster qualitative data. Well explore how these technologies work together to enable automated clustering processes.
AI uses natural language processing (NLP) and machine learning algorithms to make sense of qualitative data. These technologies collaborate to automate the process of categorizing and clustering responses, streamlining what was once a tedious and manual task. By employing NLP, AI can understand the nuances in human language, identifying keywords, phrases, and themes that need categorization. Simultaneously, machine learning algorithms benefit from this parsed data, allowing the system to learn from previous inputs and improve its clustering accuracy over time.
Together, these technologies facilitate automated response clustering, enabling organizations to quickly identify trends and patterns in qualitative feedback. This automation not only enhances efficiency but also minimizes biases often associated with manual analysis. As we explore this integration further, we can appreciate how leveraging AI can transform qualitative data analysis into a more structured and insightful process, ultimately leading to better-informed decisions.
Extract insights from interviews, calls, surveys and reviews for insights in minutes
Steps for Implementing AI-Based Automated Response Clustering
Implementing AI-based automated response clustering requires a structured approach to ensure effective results. First, initiate the process by preparing your data thoroughly. This critical step involves cleaning and formatting your qualitative responses to eliminate any inconsistencies and ensure they are suitable for analysis. The quality of the data significantly influences the accuracy and reliability of the clustering output.
Once your data is ready, the next step is selecting the right tools for the job. Numerous options exist, each with unique features tailored for automated response clustering. For instance, tools like Insight7 and Lexalytics offer robust NLP capabilities that can enhance the clustering process. After choosing your tools, proceed to execution. Apply the AI algorithms to your prepared data and interpret the resulting clusters. The insights drawn from this analysis can help identify patterns, trends, and actionable outcomes that may have previously been overlooked. Following these steps will guide you in successfully leveraging automated response clustering for your qualitative data analysis needs.
Implementing AI-driven clustering for qualitative responses involves various steps to ensure successful outcomes.
Implementing AI-driven clustering for qualitative responses involves careful planning and execution across several crucial stages. The first step is data preparation, wherein researchers must clean, format, and structure their qualitative responses. Proper data preparation ensures that the AI algorithms have high-quality inputs to work with, significantly enhancing the accuracy of the clustering results.
Next, it’s essential to select the right tools that will facilitate automated response clustering. Tools such as Insight7, MonkeyLearn, and NVivo stand out for their user-friendly interfaces and powerful analytic capabilities. Once the tools are set up, the final phase is execution and analysis, where the chosen AI algorithms are run, and the resulting clusters are interpreted. This systematic approach maximizes the potential for generating valuable insights, thereby streamlining qualitative analysis and improving decision-making efficiency. Proper execution of these steps ultimately leads to successful outcomes in understanding qualitative data through automated methods.
Step 1: Data Preparation
To achieve effective Automated Response Clustering, data preparation is a critical first step. This involves organizing and cleaning your qualitative responses to ensure they are ready for analysis. Proper data preparation enhances the accuracy of the clustering process and allows the AI algorithms to function optimally. Begin by removing any irrelevant information or noise that could skew results. Quality data will lead to clearer clusters, making insights easier to derive.
Next, consider formatting your data consistently. This can include standardizing responses and categorizing different themes that emerge from your data set. By defining themes ahead of time or using default templates, you can streamline the analysis process. Once your data is clean and formatted, you create a solid foundation for the next steps. This preparation is vital, as it directly impacts the effectiveness and reliability of your Automated Response Clustering efforts.
Before initiating the clustering process, its crucial to prepare your data. This includes data cleaning, formatting, and ensuring the responses are ready for analysis.
Before diving into the clustering process, thorough preparation of your data is essential. This initial step involves careful data cleaning, formatting, and organizing responses to ensure they are suitable for analysis. Start by reviewing the data to identify and rectify inaccuracies, inconsistencies, or irrelevant information. In qualitative research, the quality of the data significantly impacts the clustering results. Ensuring clarity in how data is presented allows AI algorithms to work more effectively in the next stages.
Once cleaning is complete, focus on formatting the data uniformly. This includes standardizing text inputs, categorizing responses, and applying consistent terminologies. Readiness for analysis is paramount; if responses are scattered or improperly labeled, clustering efforts will yield unreliable insights. By investing time in these preparatory tasks, you lay a strong foundation for successful Automated Response Clustering, ultimately driving more accurate and actionable insights from your qualitative data analysis. Each step taken during this phase streamlines the integration of AI, making the overall clustering process more efficient and effective.
Step 2: Choosing the Right Tools
Choosing the right tools for Automated Response Clustering is a pivotal step in enhancing the analysis of qualitative data. The effectiveness of your clustering efforts hinges on having the best technologies at your disposal. Various tools cater to different needs, ensuring you can find a solution that aligns with your specific requirements. When evaluating these tools, consider factors such as user-friendliness, customization options, and the depth of analysis they provide.
Among the popular options, Insight7 stands out for its intuitive interface and robust features. MonkeyLearn is another excellent choice, offering customizable algorithms for precise text analysis. Meanwhile, Lexalytics excels in providing comprehensive natural language processing capabilities, making it ideal for text clustering tasks. RapidMiner and NVivo also offer strong functionalities, with RapidMiner focusing on machine learning and NVivo supporting qualitative and mixed-methods research. Selecting the right tool not only streamlines the clustering process but also maximizes the insights you can glean from your qualitative responses.
Selecting appropriate tools is vital for successful implementation. Consider the following tools:
Selecting appropriate tools is vital for successful implementation. The integration of AI in clustering qualitative responses is contingent on the tools employed. Each tool presents unique features and functionalities that cater to different analysis requirements. Therefore, choosing the right tool is crucial for achieving meaningful insights from your qualitative data.
Consider tools like Insight7, which excels in automated qualitative data clustering and boasts a user-friendly interface. Another option, MonkeyLearn, allows for customizable algorithms tailored for detailed text analysis. Lexalytics offers comprehensive NLP capabilities for effective text clustering. RapidMiner is known for its strong machine learning support, while NVivo facilitates efficient qualitative and mixed-methods research. By selecting the appropriate tool, organizations can ensure effective automated response clustering, leading to improved insights and decision-making. Each tool's strengths contribute to the overall success of your qualitative analysis efforts.
- Insight7: A leading tool in automated qualitative data clustering, known for its user-friendly interface and robust results.
Automated qualitative data clustering has transformed the way we process information, and its effectiveness is evident in the capabilities of Insight7. This tool stands out for its intuitive, user-friendly interface that simplifies complex clustering tasks. With automated response clustering, users do not need extensive training; they can focus on interpreting results rather than dealing with cumbersome processes.
The tool employs cutting-edge AI algorithms to categorize qualitative data efficiently. This robust technology enables clustering of responses based on similarities, revealing significant patterns and insights that might be overlooked. Users can easily navigate the interface to customize their settings, ensuring optimal results tailored to their specific research needs. As businesses and research entities grapple with large volumes of data, utilizing an automated response clustering tool like Insight7 allows for quicker decision-making and enhanced analytical capabilities, making the entire process seamless and efficient.
- MonkeyLearn: Offers customizable algorithms for detailed text analysis.
Automated Response Clustering can greatly enhance text analysis tasks by leveraging customizable algorithms designed for detailed analysis of qualitative data. Many AI tools enable users to tailor their algorithms to focus on specific needs, whether that’s extracting themes or categorizing responses effectively. By adjusting various parameters, users can refine the clustering process to align with their unique objectives, ensuring that the insights gathered are both relevant and actionable.
When utilizing these advanced algorithms, it's vital to understand the data preparation phase. Properly cleaning and formatting qualitative responses allows the AI systems to perform optimally. After preparing the data, users can select an appropriate tool that complements their analytical goals, enhancing the efficiency of the process. Ultimately, successfully implementing these customizable algorithms not only streamlines data analysis but also leads to more significant insights, guiding decision-making and strategic planning in various sectors.
- Lexalytics: Provides comprehensive NLP features for text clustering.
Advanced natural language processing (NLP) tools play a critical role in automated response clustering, allowing organizations to efficiently analyze qualitative feedback. These tools can process vast amounts of text data, grouping similar responses into meaningful clusters that reveal trends and insights. This capability enables more informed decision-making, making it an essential feature for businesses exploring automated analysis.
When utilizing NLP for response clustering, several features stand out. First, effective data ingestion allows for the seamless input of varied text formats, such as surveys, interviews, or social media feedback. Second, robust transcription services convert audio or video responses into text, ensuring that no valuable insights are lost. Third, advanced text analysis algorithms identify themes and patterns, making it easier to understand customer sentiments and preferences. Finally, user-friendly interfaces simplify the entire process, enabling users of all levels to harness the power of automated response clustering effectively.
- RapidMiner: A powerful platform for machine learning and data science tasks.
A powerful platform for machine learning and data science tasks stands out in the realm of Automated Response Clustering. Designed for intuitive usability, it equips users with the necessary tools to efficiently analyze large sets of qualitative data. This platform combines advanced algorithms and an accessible interface, making it an ideal choice for those looking to streamline their data analysis process.
Using this platform, researchers and businesses can automate the clustering of qualitative responses, drastically reducing the time it takes to derive meaningful insights. The system employs natural language processing to intelligently categorize responses, identifying key themes and trends without manual effort. As a result, organizations can focus on strategic decision-making instead of getting bogged down by time-consuming analysis. By integrating this powerful platform into their workflows, users can unlock significant efficiencies and enhance their understanding of qualitative research outputs.
- NVivo: Known for supporting qualitative and mixed-methods research efficiently.
Effective analysis of qualitative data is crucial in research and decision-making. One prominent tool in this domain is designed for qualitative and mixed-methods research, providing researchers with the capability to organize and analyze their data efficiently. Users appreciate its intuitive interface, facilitating seamless navigation through complex datasets.
The tool empowers researchers to automate response clustering, significantly accelerating the process of identifying themes and patterns. Through the integration of advanced technologies like natural language processing, it aids in sorting vast amounts of qualitative responses into meaningful groups. This not only enhances data clarity but also saves valuable time, allowing researchers to focus on interpretation rather than manual sorting.
By using automated methods, researchers can explore connections within their data that might otherwise be overlooked. Ultimately, this tool stands out for its ability to transform qualitative analysis, making it an indispensable asset for those aiming to derive insights efficiently.
Step 3: Execution and Analysis
In Step 3: Execution and Analysis, you bring your plans into action through direct application of AI tools. This phase involves running the selected algorithms on your prepared data set to cluster qualitative responses automatically. By leveraging the capabilities of AI, you can efficiently categorize large volumes of information based on themes, sentiments, or other relevant criteria. This process enables you to pinpoint valuable patterns and insights that might not be immediately obvious.
Following the execution, analysis takes center stage. Here, scrutinizing the results becomes crucial. You'll want to delve into the clusters created by the AI, examining the insights they yield. This analysis not only informs you about emerging trends but also assists in aligning your findings with your study's objectives. By connecting these insights to specific goals, such as enhancing customer experience or identifying challenges, you effectively harness automated response clustering to drive impactful decision-making.
Executing the AI algorithms and analyzing the clusters is the final step. This includes running your chosen tool, interpreting the results, and drawing actionable insights from clustered data.
Executing the AI algorithms and analyzing the clusters is a crucial final step in the process of automated response clustering. Once you've chosen the right tool for your data, it's time to run the algorithms. This process involves feeding your prepared qualitative responses into the AI system, which will then categorize them based on their inherent similarities. The output will be clusters representing the main themes or sentiments within the data.
Interpreting these results is essential. You need to look beyond the clusters themselves and understand the narratives they tell. Identify key insights, such as recurring themes or areas of concern raised by respondents. Drawing actionable insights from the clustered data enables you to inform strategic decisions, adjust product offerings, or enhance customer experiences. Ultimately, this step transforms raw qualitative data into meaningful information, paving the way for informed decision-making.
Conclusion: The Impact of Automated Response Clustering
Automated Response Clustering fundamentally changes how qualitative data is approached in research and business. By harnessing AI's capabilities, organizations can streamline their analysis processes, leading to quicker and more reliable insights. No longer reliant solely on manual analysis, teams can minimize bias and inconsistencies, ultimately enhancing their ability to make informed decisions.
The impact of this technology extends beyond saving time; it allows researchers to focus on strategic thinking rather than mundane data processing. As businesses increasingly adopt Automated Response Clustering, they are likely to find themselves more competitive in their respective industries. Embracing this innovative approach offers valuable tools for navigating the complexities of qualitative data, driving efficiency and effectiveness in research endeavors.
Automated Response Clustering revolutionizes the way businesses and researchers analyze qualitative data. By embracing AI, you can achieve faster, more accurate insights and unlock the full potential of your qualitative responses. Implementing the steps and tools discussed can lead to more informed decision-making and a competitive edge in your field.
Automated Response Clustering fundamentally changes how businesses and researchers manage qualitative data analysis. By leveraging artificial intelligence, organizations can process vast quantities of qualitative responses in a fraction of the time it would take manually. This shift not only improves accuracy but also enhances the reliability of insights drawn from the data. The technology analyzes patterns and themes quickly, revealing valuable information that can guide strategic decision-making.
To effectively implement Automated Response Clustering, it is essential to follow specific steps. First, proper data preparation is crucial; cleaning and structuring the data ensures optimal analysis. Next, choosing suitable AI tools tailored to your needs greatly influences the outcome. Tools such as Insight7 or MonkeyLearn provide powerful capabilities for clustering qualitative insights. Once this foundation is set, executing the algorithms and interpreting the findings will lead to actionable insights. Adopting these practices can place you ahead of your competitors in gaining actionable intelligence from qualitative responses.