How to Use AI to Build Research Narratives from Interviews
-
Hello Insight
- 10 min read
AI-Powered Research Narratives offer a transformative approach to extracting insights from interviews. In the realm of qualitative research, traditional methods can feel tedious and riddled with inconsistencies. However, with the integration of AI capabilities, researchers can streamline the analysis, allowing them to focus on the stories and themes that emerge from participant responses.
These narratives are not just about summarizing data; they encompass the broader implications of the findings. By leveraging AI tools, researchers can enhance the depth and clarity of their narratives, ensuring that diverse perspectives are represented. The power of AI lies in its ability to process large volumes of data quickly and efficiently, ultimately leading to richer, more actionable insights.
Analyze & Evaluate Calls. At Scale.
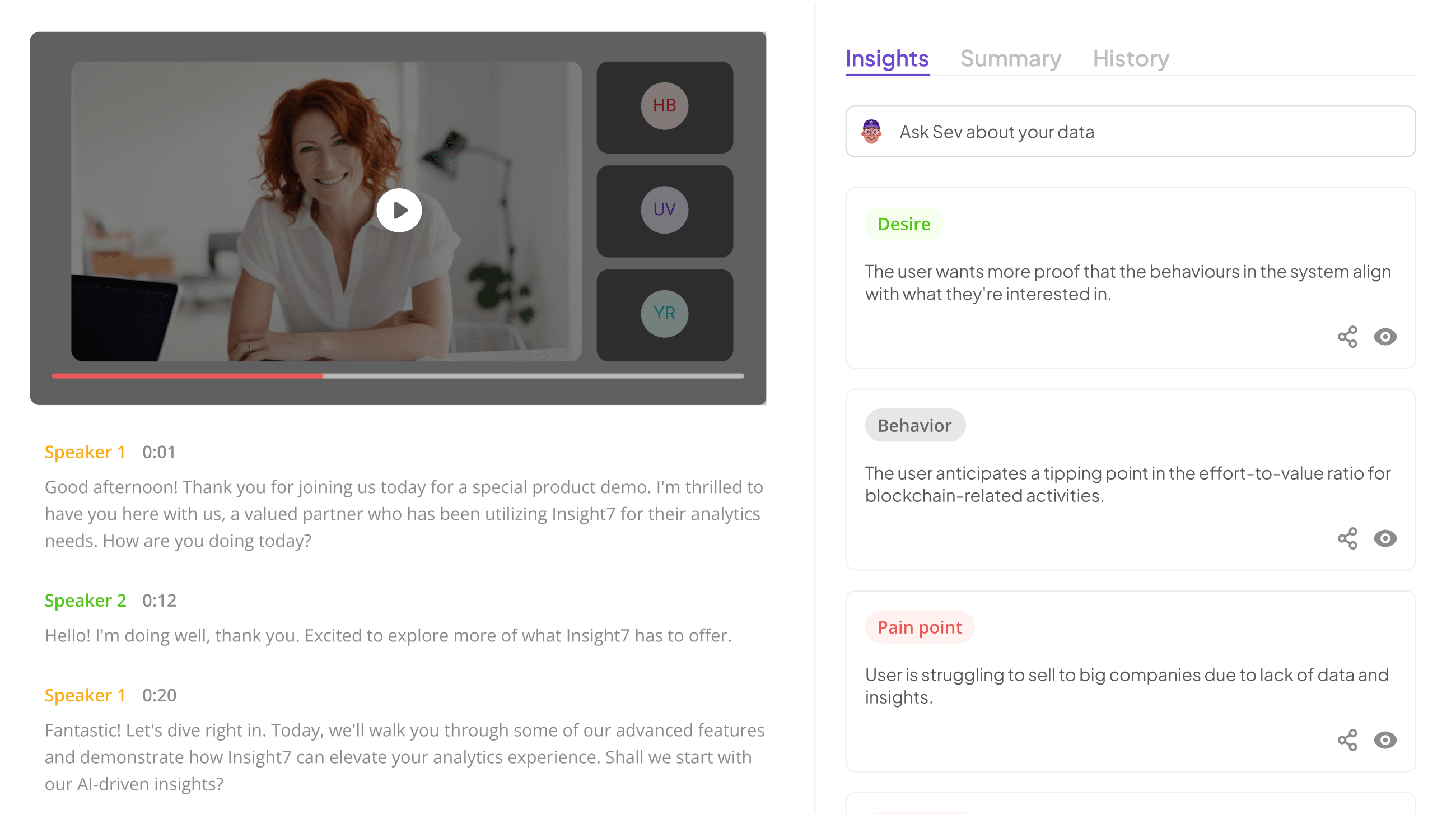
Understanding the Importance of Research Narratives
Research narratives serve as the backbone of insightful research, providing context and coherence to the data collected. When constructing these narratives, particularly through AI-powered means, one must understand their significance in conveying essential findings. A well-crafted narrative can highlight critical themes, patterns, and anomalies, translating raw data into actionable insights that stakeholders can easily comprehend.
AI-Powered Research Narratives play a pivotal role in this process, enabling researchers to sift through vast volumes of interview data rapidly. By utilizing AI tools, it's possible to extract meaningful insights while organizing the narrative effectively. The capacity to automate transcription, analyze sentiments, and visualize data enhances the research quality, ensuring that key messages resonate with the audience. Ultimately, robust research narratives not only elucidate findings but also empower decision-making, making them an invaluable component of the research journey.
The Role of AI in Enhancing Research Narratives
AI-powered research narratives transform the way we analyze and present qualitative data. By automating the transcription and analysis of interview insights, researchers can focus on crafting compelling stories rather than being bogged down by manual processes. This enhancement significantly reduces biases associated with human analysis, ensuring consistent and reliable insights across different team members. With the ability to synthesize vast amounts of data quickly, AI tools allow for more profound exploration of themes and narratives.
Integrating AI into research narratives encourages a more collaborative approach among researchers. By utilizing AI-powered tools, teams can streamline their processes, enhance their efficiency, and ultimately deliver faster, more accurate reports. This evolution in research practices opens doors to deeper understanding and more impactful decisions, enriching the overall narrative experience. As the reliance on technology continues to grow, researchers can embrace these innovations to create richer, data-driven narratives that resonate with their audiences.
Building AI-Powered Research Narratives from Interviews
Building AI-Powered Research Narratives from Interviews begins with the fusion of qualitative insights gathered from conversations and the robust capabilities of artificial intelligence. This integration transforms raw interview data into structured narratives that highlight key themes and insights effectively. By employing AI tools, researchers can speed up the process, reduce biases, and ensure consistency in data interpretation across teams.
To embark on this journey, researchers must first prepare their data meticulously. This involves accurately transcribing interviews, ensuring that details are not lost in translation. Once the data is organized, AI tools such as transcription services and text analysis platforms can facilitate the extraction of actionable insights. Ultimately, this method strives to create clear, AI-Powered Research Narratives that present findings in a compelling and coherent manner, enhancing overall research quality and efficiency.
Preparing Your Data: Structuring Interviews for AI Analysis
Preparing your data for AI-powered research narratives starts with structuring your interviews effectively. Begin by transcribing your interviews accurately, capturing the nuances of participant responses. This transcription forms the foundation of your analysis and must reflect genuine dialogue. Clear, verbatim transcripts allow AI tools to identify trends, sentiments, and insights with higher precision, enhancing the overall quality of your research.
Next, focus on cleaning and organizing your data. This step is crucial for minimizing noise and ensuring clarity. Remove irrelevant information and categorize responses into themes or topics. A well-structured dataset enables AI to analyze interview content efficiently, minimizing biases and inconsistencies. By preparing your data thoughtfully, you set the stage for powerful AI analysis that transforms raw interview data into compelling narratives that communicate key insights effectively.
Step 1: Transcribe Your Interviews
Transcribing your interviews is a foundational step when building AI-Powered Research Narratives. Accurate transcription ensures that the nuances and insights shared during interviews are captured effectively. By transforming spoken content into written text, you establish a reliable dataset that serves as a canvas for further analysis. This meticulous process sets the stage for extracting deeper meanings and themes, enriching the narrative you aim to construct from your research.
To efficiently transcribe your interviews, consider utilizing advanced tools available today. Many platforms offer native transcription services that support multiple languages and ensure a high accuracy rate. By allowing bulk uploads of audio files, these tools save valuable time that would otherwise be spent on manual transcription. Once transcribed, you can easily analyze the data to draw connections, identify patterns, and ultimately create a compelling narrative that resonates with your audience.
Step 2: Clean and Organize Data
To create AI-Powered Research Narratives, organizing your data is critical. Begin by compiling all interview transcripts in a centralized format. This ensures that each conversation is accessible, facilitating easy review and analysis. As you input data, consider tagging sections based on themes or keywords to streamline retrieval later.
Next, clean your data by eliminating inconsistencies and errors. This step is essential for achieving accurate insights from your narratives. Search for common themes, patterns, or trends to determine the core messages from your interviews. Don't overlook the importance of context; ensure each data piece is relevant to your key research questions. This intentional organization paves the way for effective AI analysis, making sure that the results accurately reflect the interview insights you aim to highlight.
Utilizing AI Tools for Narrative Building
AI-Powered Research Narratives thrive on the effective use of AI tools, enabling researchers to transform raw interview data into compelling stories. By integrating advanced technologies such as transcription software and text analysis systems, researchers can streamline the process of extracting insights from interviews. Utilizing these tools ensures that valuable patterns and themes are not overlooked, leading to richer, data-driven narratives.
The journey begins with reliable transcription services, like Otter.ai, which convert spoken interviews into text seamlessly. Following transcription, data cleaning and organization become crucial. Once structured, AI tools such as MonkeyLearn can analyze sentiments and extract key themes, while platforms like Dovetail and Nvivo facilitate comprehensive analysis and visualization. As researchers harness these AI tools, they gain the ability to construct narratives that resonate and reflect authentic user voices. Engaging with AI-driven insights ultimately enhances the quality and depth of research narratives, making findings more impactful and actionable.
insight7: Streamlining AI Analysis
Efficiently streamlining AI analysis is essential for crafting compelling AI-powered research narratives. With traditional methods of analyzing interviews, researchers often grapple with slow turnaround times and inconsistencies in insights. By embracing AI tools, teams can automate the analysis process, resulting in quicker and more reliable research outcomes.
In this context, utilizing AI for analysis transforms how qualitative data is handled. Automation ensures uniformity across different researchers, significantly reducing the subjective bias that can influence findings. Furthermore, AI tools can swiftly identify themes and patterns within interview data, enabling teams to focus on narrative creation rather than manual data crunching. Embracing this shift facilitates timely reporting and supports the demand for faster insights, ultimately enhancing the overall quality of research narratives.
Otter.ai: Automated Transcription Services
Automated transcription services have become essential in simplifying the process of developing AI-powered research narratives from interviews. By streamlining the transcription of audio and video data, these services enable researchers to focus on their analyses rather than the tedious task of manual transcription. Once the recordings are transcribed, users can effortlessly sift through the text to identify key themes, quotes, and insights essential for constructing meaningful narratives.
Integration of these automated tools not only enhances efficiency but also improves accuracy, as they employ advanced algorithms to ensure precise data capture. As interviews are converted into written text swiftly, researchers can pivot towards deeper analysis, extracting valuable insights that guide narrative development. This symbiotic relationship between transcription services and AI technology lays the groundwork for richer, data-driven stories that bring interviews to life in ways that engage and inform audiences effectively.
MonkeyLearn: Text Analysis and Sentiment Analysis
Text analysis and sentiment analysis are pivotal in creating AI-powered research narratives from interviews. These methods separate crucial insights from an abundance of conversational data, transforming them into coherent findings. By identifying patterns in dialogue and emotional tones, they empower researchers to comprehend not only what respondents are saying but also how they feel about their experiences.
To effectively conduct text analysis, start by aggregating the interview responses. Following this, apply sentiment analysis algorithms to categorize sentiments expressed, be it positive, negative, or neutral. This approach provides deeper insights into participant emotions, allowing for nuanced storytelling. Ultimately, integrating these analytical techniques into your research narrative strategy enhances the validity of findings and illustrates the human experience more vividly, making your research compelling and impactful.
Dovetail: Qualitative Data Analysis
Qualitative data analysis is vital in converting raw interview data into insightful narratives. By applying advanced tools, researchers can sift through extensive qualitative data to identify themes, trends, and key insights. Dovetail, for instance, accelerates this process by allowing users to utilize an analysis grid which simplifies the examination of multiple interviews simultaneously. This process empowers researchers to highlight significant pain points and summarize findings with ease.
Furthermore, AI-powered research narratives benefit from ongoing iterations of coding and tagging data, which streamlines the extraction of relevant information. The ability to generate templates for recurring inquiries enhances efficiency, enabling researchers to extract consistent insights across different sets of interviews. This powerful integration of qualitative analysis and AI not only boosts accuracy but also enriches the narrative that emerges from qualitative research, ultimately leading to compelling and informative outcomes.
Nvivo: Comprehensive Data Management
Effective data management is crucial for building AI-powered research narratives. Utilizing advanced software can significantly streamline your research process. This tool supports the organization, categorization, and retrieval of qualitative data, ensuring that you can analyze vast amounts of information with ease.
One essential aspect of using this tool is its ability to help create a structured framework for your interviews. For instance, you can easily code various themes, tags, and categories, enabling systematic insights extraction from transcripts. This structured approach allows researchers to identify patterns and trends, leading to deeper insights that can shape your narrative. By combining the power of technology with human creativity, you elevate your research process and ultimately produce richer, more compelling narratives.
In conclusion, comprehensive data management not only supports efficient analysis but also enhances the overall quality of your AI-powered research narratives. It provides researchers the tools necessary to transform raw data into meaningful, actionable insights.
Extract insights from interviews, calls, surveys and reviews for insights in minutes
The Process of Developing AI-Powered Research Narratives
After gathering and organizing your interview data, the process of developing AI-Powered Research Narratives begins. The first critical step involves analyzing the collected data using AI tools. By applying natural language processing and machine learning algorithms, researchers can identify patterns and key themes within the responses. This initial analysis provides valuable insights that form the foundation of a compelling narrative.
Following the analysis, the next step is synthesizing the findings into a coherent narrative. This is where storytelling comes into play; combining raw data with human elements creates engaging narratives. It's essential to highlight not only the key insights but also the underlying quotes and themes that enrich the study. By using AI to aid this process, researchers can quickly structure their findings and ensure that the narrative resonates well with the intended audience. This approach results in well-organized AI-Powered Research Narratives that are both insightful and compelling.
Step 1: Analyzing Interview Data with AI
Analyzing interview data is the first critical step in building AI-powered research narratives. Utilizing AI in this phase streamlines the process, allowing researchers to identify themes and insights quickly. The conventional method of analyzing interviews can be labor-intensive and inconsistent, often leading to bias among team members. By employing AI tools, researchers can minimize these challenges, ensuring a more objective approach to data interpretation.
To effectively analyze interview data, follow these essential steps: First, transcribe the interviews accurately, as this provides a foundation for AI tools to work with. Next, clean and organize the data to remove any inconsistencies or irrelevant content. Finally, apply AI techniques to extract patterns and key insights from the conversation, transforming raw data into a structured narrative. This analytical approach not only enhances the quality of insights but also allows researchers to deliver findings more efficiently. Embracing AI-powered research narratives revolutionizes how we understand qualitative data.
Step 2: Synthesizing Findings into a Coherent Narrative
Synthesizing findings into a coherent narrative requires careful attention to detail and cohesive storytelling. Start by identifying the key themes that emerge from your interview data. This process will allow you to highlight trends and insights that resonate with your research objectives. With AI-powered research narratives, these themes can be mapped directly to relevant quotes and evidence, creating a seamless flow of information.
Next, arrange the findings logically, providing context for each insight gathered. This organization not only strengthens the narrative but also enhances its persuasive impact. Incorporate recommendations and potential implications based on the insights gleaned, ensuring that your narrative is actionable. By crafting a structured report that links findings to themes, you can effectively communicate your research's value. Ultimately, a coherent narrative serves not just as a summary, but as a powerful tool for driving informed decision-making.
Conclusion and Future Prospects of AI-Powered Research Narratives
AI-Powered Research Narratives have transformed how researchers process and present qualitative data. As we conclude this exploration, it's clear that integrating AI into research practices not only enhances efficiency but also enriches the narrative quality of findings. Enhanced automation allows researchers to focus on interpretation rather than data management, fostering a more insightful analysis.
Looking ahead, we envision a future where these narratives will evolve with more advanced AI tools, enabling deeper insights and broader accessibility. By continuously embracing innovations, researchers can develop richer, more nuanced narratives that resonate with diverse audiences, ultimately making research more impactful and engaging for all stakeholders involved.
The Impact of AI on Future Research Practices
The introduction of AI-Powered Research Narratives is set to redefine our approach to research practices. As researchers increasingly integrate artificial intelligence into their methodologies, the landscape of data analysis and insight generation evolves significantly. AI technologies not only enhance the efficiency of data processing but also empower researchers to uncover deeper insights from qualitative data, such as interview transcripts.
One of the most profound impacts of AI lies in its ability to synthesize vast amounts of information. Advanced tools can analyze patterns and sentiments in interview responses, allowing researchers to encapsulate complex narratives swiftly. This capability minimizes human error and biases, leading to more reliable and comprehensive findings. Furthermore, as AI continues to develop, the potential for real-time analysis becomes a reality, accelerating the research cycle and enhancing decision-making processes. By embracing these innovations, researchers can create richer, more nuanced narratives that propel their work into the future.
Embracing AI Innovations for Richer Narratives
Embracing AI innovations can significantly transform the way we build research narratives from interviews. By harnessing the capabilities of AI-powered research narratives, we can enhance our understanding of complex data. AI tools help in automating data analysis, allowing researchers to focus on interpretation and storytelling, rather than getting buried in raw data.
To effectively embrace these innovations, researchers should consider three key aspects. First, they must prepare interview data meticulously, ensuring it is transcribed and organized for optimal analysis. Second, employing various AI tools, such as automated transcription services and text analysis software, can streamline the narrative-building process. Lastly, synthesizing findings into coherent narratives will foster richer insights, making them more accessible to a wider audience. Through these steps, the integration of AI in narrative development will reveal deeper connections and patterns, ultimately enriching the research experience.