AI Interview Analysis is transforming the way researchers handle qualitative data from field studies. In today’s fast-paced environment, manually analyzing interview transcripts can be daunting, often leading to inconsistent insights and extended timelines. With the growing demand for quicker insights, researchers must adapt. Here, we explore how AI can streamline the interview analysis process, making it more efficient and reliable.
By employing AI tools, researchers can expedite data processing, revealing actionable themes in mere moments. This approach not only enhances the quality of insights but also reduces biases often present in manual analysis. As we delve deeper into the methods of AI Interview Analysis, we will outline effective steps to harness technology for elevated research outcomes.
Analyze qualitative data. At Scale.
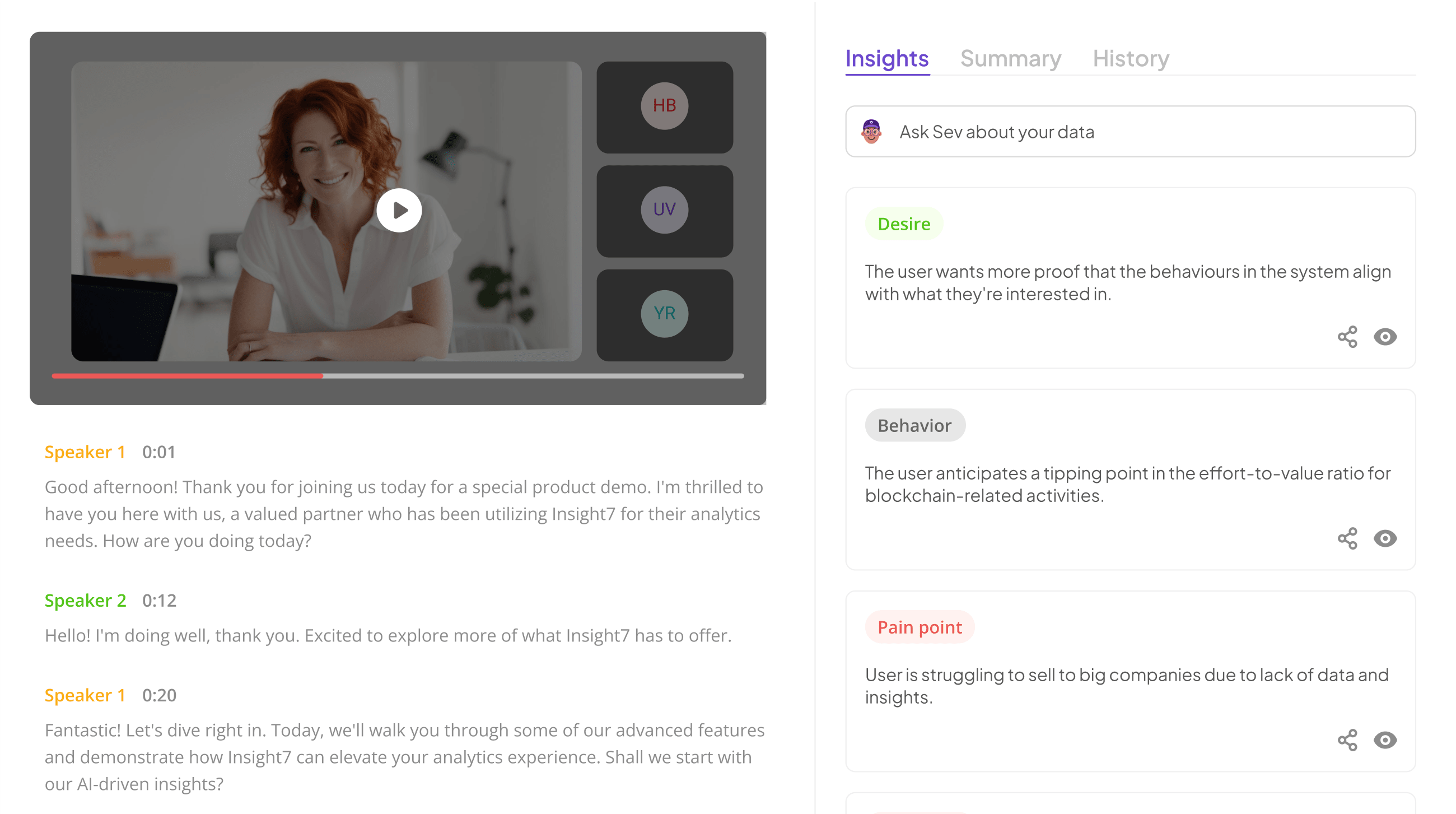
Leveraging AI Tools for Analyzing Interview Data
AI Interview Analysis offers transformative potential for researchers looking to maximize the value of their gathered data. The manual analysis of interviews can be exhaustive, often leading to delays and inconsistencies in reporting. By integrating AI tools into the process, researchers can streamline their workflows, enabling quicker insights and enhancing the overall quality of their findings.
There are several effective AI tools that can significantly improve the analysis of interview data. For instance, tools like Transcribr automate transcription tasks, allowing researchers to focus on interpreting results rather than getting bogged down in manual documentation. Similarly, Dovetail provides efficient qualitative data management, making it easier to extract and categorize themes. NVivo and ATLAS.ti offer advanced coding functions and comprehensive analytical capabilities, respectively. By harnessing these tools, researchers can achieve a more accurate and efficient analysis of interviews, ultimately enriching their insights and elevating the quality of their research.
Insight7: Leading the Way in AI Interview Analysis
AI Interview Analysis is redefining how researchers handle interview data, enhancing efficiency and accuracy. By automating time-consuming tasks, AI technologies free teams from manual processes, allowing them to focus on insights generation. This transformation is crucial for consulting firms and agencies that face tight deadlines and diverse team perspectives, leading to bias and inconsistency in analysis.
To effectively implement AI Interview Analysis, consider the following steps: first, streamline your data preparation. Ensure audio recordings and transcripts are clear and accessible. Next, utilize AI tools that suit your needs, such as transcription services and qualitative data management software. Finally, synthesize the results intelligently by examining the themes and patterns revealed by the AI, ensuring that your final insights are coherent and actionable. By embracing these innovations, organizations can expect quicker report generation and enhanced understanding of stakeholder perspectives.
Other Top AI Tools for Interview Data Analysis
When exploring other top AI tools for interview data analysis, several standout options can enhance your qualitative research efforts. Transcribr ensures efficient transcription automation, minimizing the manual work involved in capturing interviews. This tool is particularly useful for researchers who need to focus on analysis rather than transcription accuracy. Dovetail provides efficient qualitative data management, enabling users to organize their notes and findings seamlessly while collaborating with team members.
Moreover, NVivo is known for its robust coding and categorizing functions, allowing researchers to analyze data more systematically and uncover deeper insights. ATLAS.ti offers comprehensive data analysis capabilities, making it easier to visualize connections between themes and trends in the data collected. These tools collectively empower researchers, ensuring that data analysis is thorough and efficient, ultimately enhancing the depth of insights generated from interviews. Embracing these AI interview analysis tools can transform how you approach field research.
- Transcribr: Automate transcription tasks.
Accurate transcription lays the foundation for successful AI interview analysis. Transcribr automates transcription tasks seamlessly, allowing researchers to focus more on interpreting data rather than spending hours manually typing recordings. With its intuitive interface, users can easily upload audio or video files for instant transcription. This efficiency transforms how field research teams handle interview data, ensuring they can analyze responses more swiftly.
Once transcription is complete, the tool allows for organized project management. Researchers can categorize transcripts, extract prevalent themes, and generate insightful reports to draw actionable conclusions. The ability to analyze multiple transcripts collectively enhances the quality of insights derived from the interviews. As organizations increasingly rely on AI for their research, automating transcription tasks through innovative solutions like Transcribr becomes essential for maximizing outcomes in AI interview analysis. This not only streamlines workflow but also significantly increases the depth and accuracy of findings.
- Dovetail: Efficient qualitative data management.
Efficient qualitative data management is crucial for maximizing the potential of interview data from field research. Dovetail offers a streamlined platform that simplifies the complexities of analyzing qualitative data. By allowing users to upload and transcribe interviews in bulk, it significantly reduces the time spent on manual transcription. This feature not only enhances productivity but also improves the reliability of data insights, making the interview analysis process much smoother.
Through intuitive project management tools, users can extract themes, quotes, and visualizations to inform their research conclusions. The platform supports collective analysis of interview data, facilitating collaboration among team members. Additionally, AI Interview Analysis enables users to query their entire dataset, yielding quick and actionable insights. With data privacy measures in place, researchers can confidently manage sensitive information while deriving meaningful findings from their qualitative research efforts.
- NVivo: Advanced coding and categorizing functions.
Advanced coding and categorizing functions in NVivo streamline the process of analyzing qualitative interview data. With NVivo, users can apply various coding techniques that enable more nuanced data interpretation and organization. This allows researchers to better understand and visualize their findings, enhancing the overall AI Interview Analysis experience.
Several key features within NVivo contribute to its effectiveness. First, the software supports a variety of coding methods, including manual, automatic, and thematic coding. This flexibility enables researchers to choose an approach that best fits their data. Second, NVivo offers visualization tools that help represent complex data relationships through visual maps and models. This makes it easier to identify patterns and connections, thus facilitating richer insights. Lastly, categorized data can be easily exported and shared, ensuring that findings are accessible to all stakeholders involved. Overall, NVivo's advanced functions significantly enhance the analysis of qualitative data, making it an invaluable tool for researchers.
- ATLAS.ti: Comprehensive data analysis capabilities.
ATLAS.ti offers comprehensive data analysis capabilities essential for effectively navigating interview data. This tool harnesses powerful features that allow researchers to conduct in-depth analysis using AI-driven categorization and coding of data. Users can create themes and tags, helping to structure insights relevant to specific research goals, such as improving user experience or identifying risks.
The ability to automate data analysis simplifies a traditionally complex process, making it more efficient. For example, users can quickly gather sentiments and trends from interview transcripts, which they can further refine based on predefined goals. By clustering insights into organized themes, researchers can easily trace back to original quotes, enhancing the evidence behind their findings. Overall, ATLAS.ti streamlines AI interview analysis, saving time and enhancing the accuracy of research insights.
Extract insights from interviews, calls, surveys and reviews for insights in minutes
Steps to Conduct AI Interview Analysis Effectively
To conduct AI interview analysis effectively, start by preparing your data meticulously. This involves gathering transcripts or recordings and organizing them into a structured format. Ensure that all relevant interviews are included, as the quality of input data significantly influences AI outcomes. Clean the data by removing irrelevant sections, which aids the AI in focusing on key themes.
Next, implement AI tools that can transform your interview data into actionable insights. Utilize software like Dovetail or NVivo to facilitate qualitative data management and coding. These tools can help identify recurring patterns and themes in responses, thereby streamlining your analysis process. After analyzing the data, synthesize the findings to create a coherent report. Through these steps, you will optimize your AI interview analysis, saving time and minimizing bias while generating meaningful insights.
Step 1: Preparing Your Data for AI Interview Analysis
Preparing your data for AI Interview Analysis is a crucial first step in effectively extracting insights. Begin by organizing all your interview recordings, transcriptions, and related documents in a systematic manner. This organization ensures that your data is easily accessible and avoids the frustrations of searching through scattered files. It’s beneficial to categorize your interviews based on themes or specific research questions to streamline the analysis process.
Next, consider cleaning your data. This includes removing any irrelevant information and ensuring that transcripts are accurate. High-quality data is essential for effective AI analysis, as it improves the reliability of the insights generated. By focusing on data preparation, you set the stage for a more efficient analysis, allowing the AI tools to work effectively and provide valuable, actionable insights from your collected interviews.
Taking these steps will lead to a smoother transition into the next phase of your AI interview analysis journey.
Step 2: Implementing AI Tools for Enhanced Data Insight
Implementing AI tools for enhanced data insight revolutionizes the way researchers approach the analysis of interview data. To effectively harness AI Interview Analysis, start by selecting the right tools that cater to your specific needs. A variety of platforms offer features such as automated transcription, qualitative data management, and advanced coding functionalities. These tools streamline the process, allowing you to focus on deriving meaningful insights rather than getting lost in tedious manual tasks.
Next, leverage the capabilities of AI to filter and sort insights by themes or topics. This feature enables you to isolate critical information efficiently, making your analysis targeted and relevant. For instance, if your focus is on process management, the AI can sift through the data, highlighting the most pertinent quotes and findings. Ultimately, using AI tools not only enhances the accuracy of your insights but also expedites report generation, allowing for a more polished presentation of your findings.
Conclusion: Embracing AI Interview Analysis for Future Research
Adopting AI Interview Analysis represents a transformative step in the field of research. By automating laborious processes, researchers can significantly reduce the time spent on data analysis, allowing for quicker insights and improved decision-making. This shift not only enhances efficiency but also mitigates the biases and inconsistencies that often arise from manual analysis conducted by multiple team members.
As researchers continue to explore the integration of AI in their methodologies, they will uncover new patterns and themes within their data. The embrace of AI Interview Analysis encourages a more streamlined approach to capturing qualitative insights, leading to richer narratives and more impactful findings. In doing so, researchers position themselves at the forefront of innovation, ready to tackle the challenges of a rapidly evolving landscape.