AI Feedback Analysis transforms how researchers interpret qualitative data gathered from interviews and surveys. Traditionally, analyzing feedback can be a labor-intensive process, prone to inconsistencies and biases, particularly when handled by multiple team members. This section explores how leveraging AI can reduce such challenges by streamlining the feedback analysis process and delivering faster, more reliable insights.
In experience-based research, effective feedback analysis is crucial for understanding participant perspectives. AI tools can automate theme extraction and sentiment analysis, helping teams focus on strategic decision-making instead of manual data processing. As researchers increasingly seek efficiency without sacrificing insight quality, AI Feedback Analysis becomes indispensable in extracting actionable knowledge from experience-based studies.
Analyze qualitative data. At Scale.
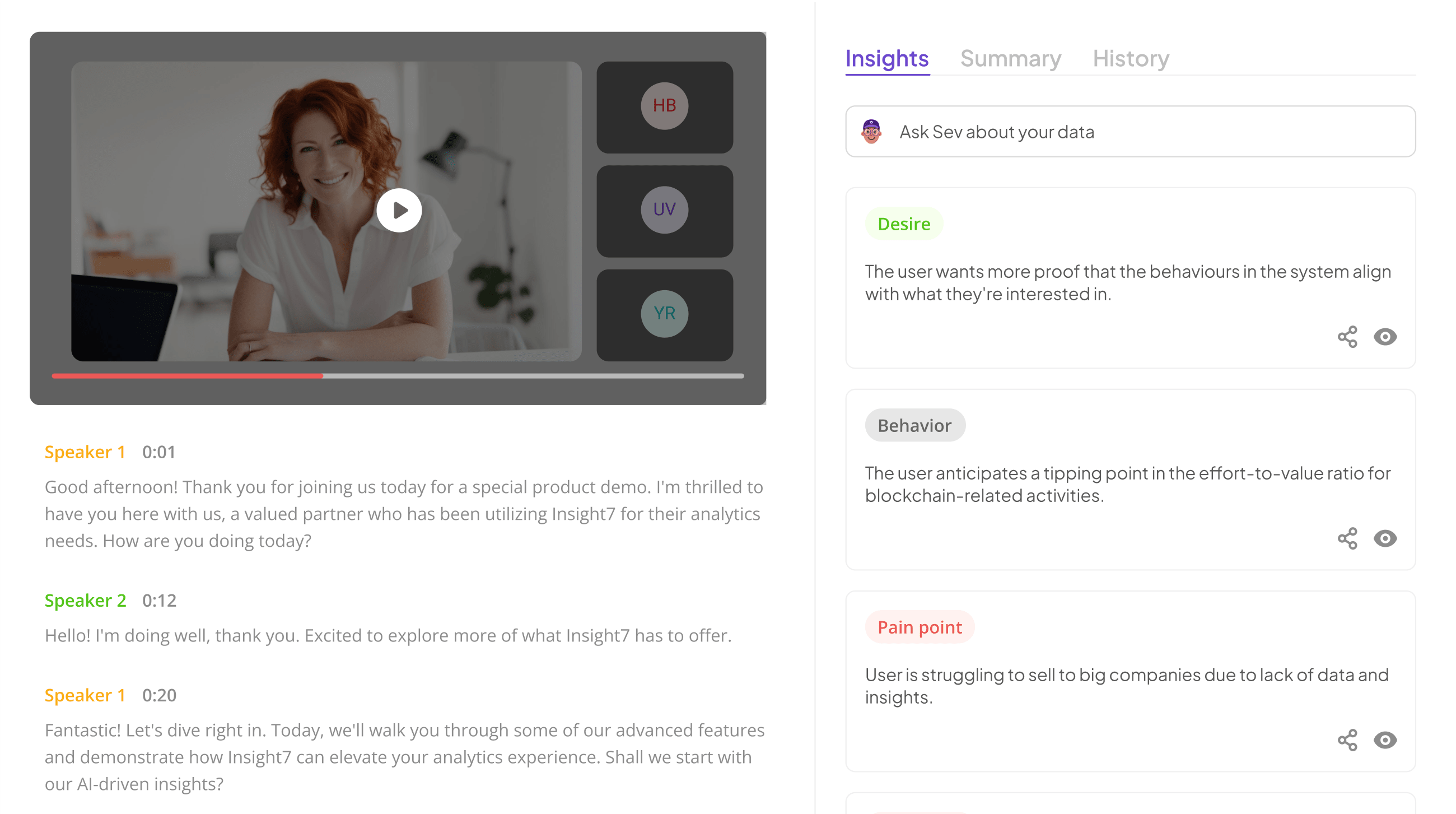
Understanding AI Feedback Analysis Tools
AI feedback analysis tools are becoming essential in navigating the complexities of experience-based research. These tools streamline the process of gathering and interpreting feedback, allowing researchers to focus on delivering quality insights. By utilizing AI, the inherent biases and inconsistencies found in manual analysis can be significantly reduced, enabling teams to produce more reliable reports and quicker turnaround times.
Among the key features of AI feedback analysis tools is their ability to process large volumes of data quickly. They employ techniques such as natural language processing to identify key themes and sentiments within feedback. Additionally, many tools offer user-friendly dashboards that simplify the visualization of results and insights, making it easier for teams to act on their findings. By adopting AI for feedback analysis, researchers can enhance their methodologies and provide more accurate, actionable insights.
Insight7: Your Go-To Tool for Feedback
Insight7 serves as an intuitive tool that revolutionizes the way researchers approach feedback analysis. Designed for ease of use, it doesn't require users to undergo complex training or tutorials. This accessibility means anyone—from seasoned researchers to newcomers—can effortlessly engage with the platform. Essentially, Insight7 organizes your feedback data within two core areas: projects and library. The library stores your transcripts and data files, whereas projects allow users to cluster and analyze these inputs effectively.
One of Insight7's standout features is its capability to directly analyze transcripts from interviews or discussions. Users can extract insights, summarize content, or even pose questions based on specific data points. This unique functionality combined with its ready-made analysis kits enables users to kickstart their investigations with just a click, dramatically streamlining the AI feedback analysis process. From in-depth qualitative assessments to thematic clustering, Insight7 becomes an invaluable ally in transforming feedback into actionable insights.
Other Top Tools for Analyzing Feedback
When considering other top tools for analyzing feedback, it's essential to recognize their unique capabilities in enhancing AI feedback analysis. Each tool comes equipped with distinct features that cater to various data analysis needs. For instance, IBM Watson utilizes natural language processing to deeply understand sentiment and context. This AI model can analyze vast amounts of feedback and extract meaningful insights, making it a valuable choice for researchers.
Additionally, MonkeyLearn offers user-friendly capabilities for text analysis, allowing users to create custom models easily. Its drag-and-drop interface simplifies the analysis process. Lexalytics, on the other hand, provides robust sentiment and opinion analysis, making it ideal for those focused on understanding customer emotions. Lastly, Qualtrics stands out for its comprehensive data integration, allowing teams to gather and analyze feedback effectively. Together, these tools enhance the potential of AI feedback analysis, enabling more nuanced understandings of experience-based research.
- IBM Watson
IBM Watson offers a powerful platform for AI feedback analysis, allowing researchers to transform complex feedback data into actionable insights. By utilizing advanced natural language processing and machine learning, this tool helps in identifying trends and sentiments within experience-based research feedback. Organizations can process large volumes of data efficiently, revealing critical insights that might otherwise remain hidden.
To effectively leverage this tool, users should focus on three key aspects: data preparation, model training, and insight extraction. First, data preparation involves cleaning and organizing feedback to ensure accuracy. Next, model training equips the AI to understand specific terminologies and sentiments relevant to the field of research. Finally, once the analysis is complete, researchers can extract meaningful insights that inform decision-making. Ultimately, using this AI feedback analysis tool can enhance the overall understanding of user experiences, paving the way for improved research outcomes.
- MonkeyLearn
In the landscape of AI feedback analysis, one tool stands out for its user-friendly interface and powerful capabilities. This platform allows researchers to easily process and interpret qualitative data from experience-based feedback. It employs advanced machine learning techniques to categorize and analyze textual inputs effectively, making it an invaluable resource for gaining actionable insights.
Users can take advantage of features such as sentiment analysis and keyword extraction, which streamline the extraction of important themes from vast amounts of feedback. By automating these processes, researchers can focus on strategic decisions rather than be bogged down by data. Ultimately, harnessing AI feedback analysis not only enhances the depth of research but also empowers organizations to respond to user needs more effectively, turning insights into actionable strategies that drive improvements.
- Lexalytics
The use of advanced analytics tools for feedback analysis is essential in modern research. Effective sentiment analysis mechanisms can decode the emotions and opinions expressed in participant responses. By utilizing intelligent algorithms, you can gain clarity on user feedback, which typically represents valuable insights into their experiences.
These tools streamline the process of text analysis by quickly transcribing and organizing data. Once you have your transcripts, you can easily identify themes, extract meaningful quotes, and generate comprehensive reports. All of this can significantly enhance your research outcomes, making data-driven decisions simpler and more accurate. Focusing on the nuances of user feedback is critical to understanding their needs, enabling organizations to provide better services or products in response to the insights gathered. The intersection of AI and feedback analysis paves the way for a future where research enhances real-world applications effectively.
- Qualtrics
Qualtrics is an advanced platform designed to simplify the process of gathering and analyzing experience-based research feedback. At its core, it enables users to collect qualitative data through open-ended survey responses, providing a wealth of insights often overlooked in quantitative analysis. Implementing AI Feedback Analysis in Qualtrics allows researchers to transform thousands of qualitative responses into manageable, actionable insights with ease.
One standout feature is the analysis grid, which allows users to process multiple questions across various datasets simultaneously. This tool can uncover significant trends and pain points by synthesizing data from numerous transcripts, ensuring a comprehensive understanding of user feedback. By utilizing customizable templates, researchers can streamline their analysis, continuously refining their approach as new data comes in. Overall, Qualtrics acts as a powerful ally in harnessing the full potential of qualitative research, ultimately enhancing the decision-making process.
Extract insights from interviews, calls, surveys and reviews for insights in minutes
Implementing AI Feedback Analysis in Research
To implement AI feedback analysis in research, the first step is collecting and organizing feedback data efficiently. Gather qualitative and quantitative data from interviews, surveys, or other sources, ensuring everything is well-structured. This organization forms the foundation for any successful analysis.
Next, set up AI models to process the feedback. Depending on your research focus, choose the appropriate model that best fits your data's nature. Powerful AI tools can identify patterns, categorize responses, and extract meaningful insights, significantly reducing the time your team spends on manual analysis.
Analyzing the results is crucial. Examine the generated insights alongside traditional methods to validate findings. This comprehensive approach ensures you create actionable insights while minimizing bias and inconsistency. Informed by AI feedback analysis, your research can evolve to meet demands for quicker and more reliable results.
Step-by-Step Process for Effective AI Analysis
To conduct effective AI feedback analysis, begin by gathering and organizing your research data meticulously. This foundational step involves collecting insights from various sources, including interviews and surveys, ensuring they are easily accessible for analysis. Properly structured data enhances the AI’s ability to discern patterns and themes, leading to more accurate results.
Next, set up AI models tailored to your specific needs. This involves selecting the right algorithms and configurations suited for analyzing qualitative data. Incorporating natural language processing can significantly aid in interpreting open-ended feedback effectively. Finally, comprehensively analyze the results generated by the AI. Extract actionable insights that will contribute to resolving your research queries and informing strategic decisions.
Follow this step-by-step process consistently to ensure reliable and efficient outcomes in your experience-based research feedback analysis.
Step 1: Collecting and Organizing Feedback Data
Collecting and organizing feedback data is crucial for effective AI Feedback Analysis. Begin by gathering all relevant feedback, whether it comes from surveys, interviews, or open-ended comments. Organize this data systematically to ensure that you can easily access and analyze it later. Consider using spreadsheets or specialized software to categorize feedback by themes or sentiments, which will aid in the analysis process.
Once you have collected and organized the feedback data, the next step is to make sense of it. This involves identifying common patterns and trends in responses. You may want to create categories such as strengths, weaknesses, and suggestions for improvement. Doing so will prepare the data for AI tools, which can detect deeper insights and correlations. Effective organization sets the foundation for AI Feedback Analysis, enabling you to derive meaningful conclusions that can inform future strategies and improvements.
Step 2: Setting Up AI Models for Analysis
After collecting and organizing your feedback data, the next crucial phase involves setting up AI models for analysis. This step is vital for ensuring that the AI algorithms understand the nuances and context of your data—enabling effective analysis. You must select appropriate AI tools that offer customizable features, allowing for tailored analysis based on your research goals. These tools are equipped to process and assess various data sets, enhancing the quality of insights generated.
To set up your AI models effectively, consider the following points:
Define Objectives: Clearly establish what insights you aim to gain from your analysis. This clarity will guide your model configurations.
Choose the Right AI Tool: Select a model that aligns with your objectives and can effectively handle the kind of feedback data collected.
Input Relevant Data: Upload your organized feedback into the AI system, ensuring that the data is clean and structured for better analysis results.
Configure Analysis Parameters: Set the parameters and algorithms in the AI tool according to the insights you seek, adjusting settings for specific themes or keywords.
Run Preliminary Tests: Conduct test runs to verify that the model understands your data, iterating on the model's settings as necessary.
By following these steps, you will create a strong foundation for AI feedback analysis that not only maximizes accuracy but also enhances the relevance of the insights produced.
Step 3: Analyzing Results and Deriving Insights
In Step 3: Analyzing Results and Deriving Insights, the journey into understanding your collected feedback truly begins. AI Feedback Analysis is your gateway to transforming raw data into meaningful insights. Start by examining the identified themes and patterns that your AI tools have highlighted. This process involves diving deep into the coded data, where specific sentiments such as challenges, desires, and suggestions emerge. By utilizing analysis kits, you can easily pull relevant themes tailored to your research objectives.
Next, cluster these insights into manageable categories, or themes. This organization not only streamlines your findings but also aids in mapping them against predefined goals, such as improving user retention or enhancing overall experience. For instance, if improving collaboration is a goal, insights that reflect the need for better tools can significantly inform your strategy. Combining sentiment analysis with these clusters ensures that you have a comprehensive understanding of user needs and preferences, ultimately guiding more informed decision-making.
Conclusion: Enhancing Research with AI Feedback Analysis
Utilizing AI Feedback Analysis can significantly enhance the quality and efficiency of experience-based research. By automating the feedback processing, researchers can eliminate biases and inconsistencies that often arise from manual analyses. This technology facilitates quicker access to insights, allowing teams to deliver results faster while maintaining a high standard of quality.
Moreover, AI Feedback Analysis empowers researchers to focus on critical elements rather than getting bogged down by repetitive tasks. By integrating AI tools into the research workflow, organizations can uncover deeper insights, leading to more informed decision-making. Ultimately, embracing AI is not just about speed; it's about elevating research outcomes through innovative analysis methods.