AI-Driven Narrative Analysis is transforming the landscape of qualitative research, offering a fresh perspective on how we understand human stories. As researchers grapple with manual data analysis, the emergence of AI presents an opportunity to enhance efficiency and accuracy in processing complex narratives. AI-driven methods can sift through vast amounts of qualitative data, revealing meaningful patterns and insights that might otherwise go unnoticed.
This innovative approach not only accelerates the analysis process but also reduces the potential for human bias. By integrating AI into narrative analysis, researchers can achieve a more consistent and objective understanding of the data. Embracing AI-driven narrative analysis empowers researchers to focus on deeper interpretations and strategic decision-making, ultimately leading to richer qualitative insights.
Analyze & Evaluate Calls. At Scale.
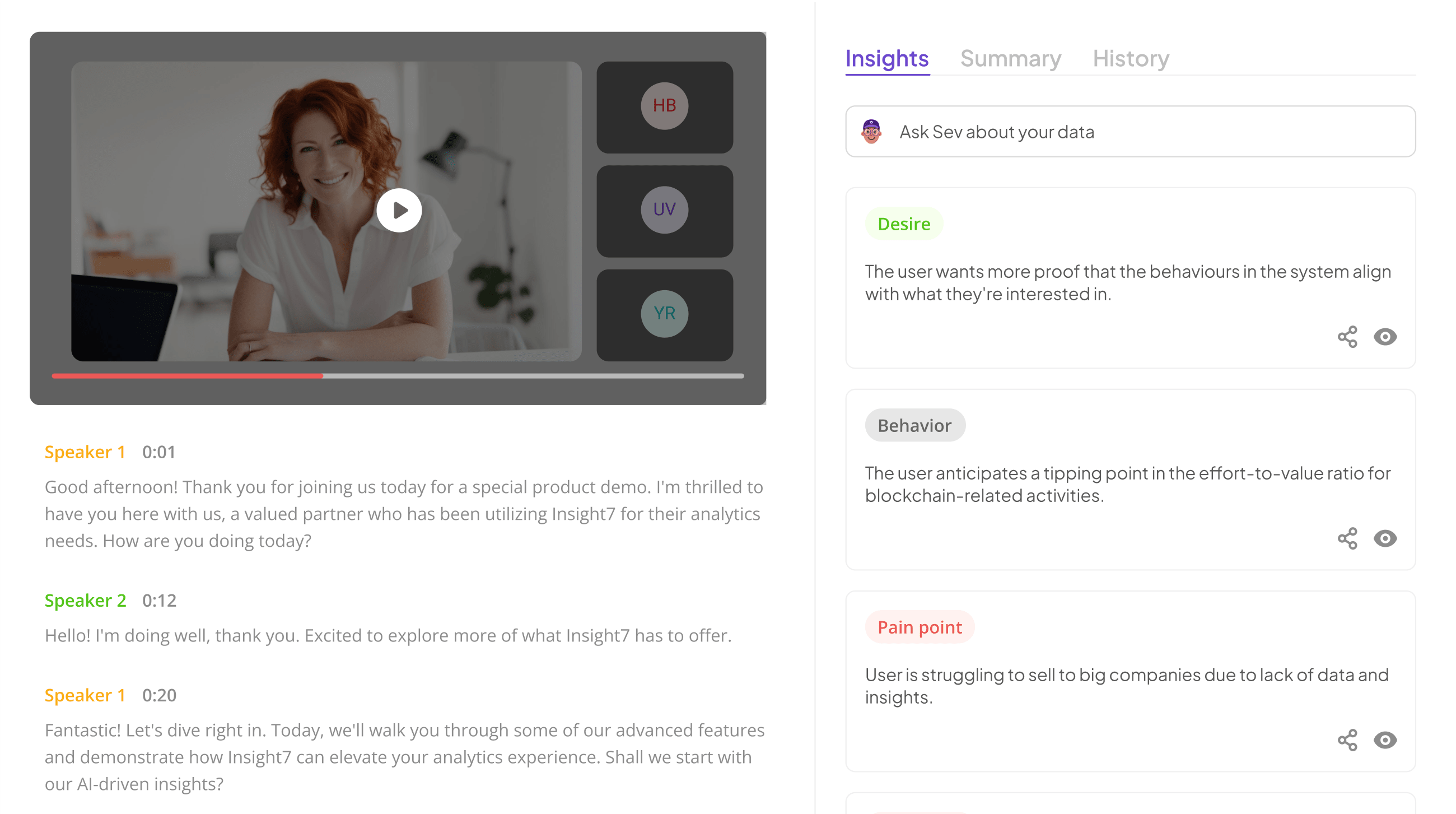
Understanding AI-Driven Narrative Analysis
AI-Driven Narrative Analysis represents a significant shift in how researchers approach qualitative data. Traditionally, narrative analysis involved labor-intensive methods that were often inconsistent and prone to bias. With the advent of AI technologies, researchers can now harness advanced algorithms to process and analyze narrative data more efficiently. These tools can quickly identify themes, trends, and anomalies within large data sets, allowing for deeper insights without the manual workload.
Understanding AI-Driven Narrative Analysis not only streamlines the research process but also enhances the quality of insights captured. By automating the analysis of interviews and open-ended survey responses, researchers can focus on interpreting the findings instead of getting bogged down in data processing. This modern approach empowers teams to generate timely reports and make well-informed decisions, ultimately elevating the research output and providing a competitive edge in various industries.
The Role of AI in Qualitative Research
AI has fundamentally changed the landscape of qualitative research by enhancing the ability to analyze narratives. Researchers often face challenges such as time constraints, bias, and inconsistency when processing qualitative data. AI-driven narrative analysis provides a transformative solution, offering tools that automate and streamline the extraction of meaningful insights from qualitative sources.
One significant advantage of AI in this context is efficiency. Researchers can process large volumes of data swiftly, allowing for quicker turnaround on insights. Additionally, AI's ability to identify patterns and themes mitigates biases that might arise in manual analysis. This ensures a more objective understanding of the narratives being studied. The combination of speed and objectivity makes AI-driven narrative analysis an invaluable asset for researchers striving for depth and precision in qualitative inquiries.
Explore how AI has revolutionized qualitative research, specifically in understanding narratives.
AI has transformed qualitative research by enhancing how we understand narratives. Traditionally, analyzing narratives was slow and subjective, often varying between researchers. AI-driven narrative analysis offers a systematic approach to processing large volumes of qualitative data efficiently. With the ability to recognize patterns, themes, and sentiments within narratives, AI provides insights that would take humans much longer to uncover.
Moreover, AI minimizes bias and improves consistency across analysis. This ensures that insights derived from qualitative research are reliable and actionable. Researchers can utilize AI tools to streamline the process, allowing them to focus on interpreting the findings rather than getting bogged down in manual data processing. As a result, AI not only accelerates the research timeline but also enhances the depth of understanding in narratives, making qualitative research more effective and impactful.
Understanding AI-Driven Narrative Analysis
AI-driven narrative analysis is altering the landscape of qualitative research. By utilizing advanced algorithms and machine learning techniques, researchers can uncover profound insights from narrative data that were previously challenging to extract manually. This method aids in automating the analysis process, which significantly enhances efficiency and accuracy in understanding complex narratives.
In qualitative research, narratives often provide rich insights into participants' experiences and emotions. AI facilitates the categorization and interpretation of these narratives, revealing patterns, themes, and sentiments within diverse datasets. By systematically analyzing qualitative data with AI, researchers can extract more nuanced insights, ultimately leading to more informed conclusions and recommendations. This approach ensures a deeper comprehension of participant perspectives, thereby enhancing the overall quality of research outcomes.
Benefits of Using AI for Narrative Analysis
AI-driven narrative analysis presents numerous benefits that significantly enhance qualitative research. One primary advantage is efficiency, where AI can process large datasets far quicker than manual analysis. This speed allows researchers to focus more on interpreting results rather than spending excessive time on data processing. Additionally, AI tools can detect patterns and trends within narratives that might be overlooked by human analysis. This capability leads to deeper insights into the data, enriching the research findings.
Another key benefit is scalability. As qualitative research often involves vast amounts of text, AI-driven methods can scale analysis and maintain quality, no matter the dataset size. Moreover, these tools offer the ability to filter insights by specific themes or keywords tailored to research goals. This flexibility makes AI-driven narrative analysis an indispensable component for researchers aiming to derive meaningful insights efficiently and effectively. By embracing these advancements, qualitative research can evolve, yielding richer, more accurate narratives.
Discuss the advantages of employing AI-driven methods, such as efficiency, scalability, and insights discovery.
Employing AI-driven methods for narrative analysis offers numerous advantages that can significantly enhance qualitative research. One of the primary benefits is efficiency. AI tools can process vast amounts of narrative data in a fraction of the time it would take a human researcher. This accelerated analysis not only saves time but allows researchers to focus on deeper insights and strategies instead of being bogged down in manual data processing.
Scalability is another key advantage. AI-driven narrative analysis can easily handle growing datasets, accommodating more interview transcripts, feedback forms, and written narratives without compromising accuracy. This ability to scale means that researchers can conduct broader studies and still manage intricate details effectively.
Finally, AI facilitates insights discovery through advanced pattern recognition and thematic analysis. It can identify hidden relationships and trends that might be overlooked during manual review. By leveraging these capabilities, researchers can derive actionable insights that drive informed decision-making, enhancing the overall impact of their qualitative studies.
Extract insights from interviews, calls, surveys and reviews for insights in minutes
Implementing AI-Driven Narrative Analysis: A Step-by-Step Guide
To implement AI-driven narrative analysis effectively, start by focusing on data collection and preparation. This crucial first step involves gathering narrative data from interviews, focus groups, or open-ended survey responses. Once collected, cleaning and organizing this data ensures that the AI can analyze it without inaccuracies. Proper formatting and structuring help improve the analysis process significantly.
Next, selecting appropriate tools is essential for conducting effective AI-driven narrative analysis. Various software options, such as insight7, NVivo, and Atlas.ti, offer powerful features for analyzing narratives. These tools allow researchers to categorically identify themes and extract valuable insights from qualitative data. Once youโve chosen your tools, it's vital to train AI models properly. This involves feeding models with adequate samples of narratives, enabling them to recognize nuances, contexts, and meanings, ensuring reliable analysis results. Following these steps will help maximize the benefits of AI-driven narrative analysis in qualitative research.
Step 1: Data Collection and Preparation
The first step in utilizing AI-driven narrative analysis is the thorough collection and preparation of data. Starting with a clear understanding of your research objectives helps determine the types of narratives you need to collect. Identifying relevant sources such as interviews, focus groups, or textual data can provide rich material for analysis. Once gathered, itโs essential to clean the dataโremoving any irrelevant or redundant information ensures that the AI tools can work effectively without unnecessary noise.
Next, organize your data into a coherent structure. This might involve categorizing responses or creating thematic clusters that align with your research questions. Properly formatted data will facilitate smoother integration with AI technologies, enhancing their analytical capabilities. Remember, the quality and clarity of the initial data significantly affect the insights yielded by AI-driven narrative analysis, making this step vital for your overall research success.
Explain the importance of pre-processing data for effective AI analysis.
Pre-processing data is a crucial step for effective AI-Driven Narrative Analysis in qualitative research. This phase involves cleaning and organizing the raw data to enhance its usability. If data is not pre-processed properly, the AI tools may misinterpret or overlook significant insights, resulting in skewed analysis. By removing irrelevant information and standardizing formats, researchers can ensure more accurate outcomes.
Additionally, pre-processing facilitates the identification of themes and patterns within narratives. This structured approach allows AI algorithms to analyze vast amounts of text efficiently. Well-prepared data improves the performance of AI-driven methods, making it easier to extract meaningful insights. Moreover, it helps mitigate biases that might arise from unfiltered information. Ultimately, investing time in pre-processing establishes a solid foundation for AI analysis, leading to richer, more trustworthy findings in qualitative research.
Step 2: Choosing the Right Tools for AI-Driven Narrative Analysis
Selecting appropriate tools is crucial to effectively conduct AI-Driven Narrative Analysis. The right software can significantly impact the overall quality and efficiency of your research. Various platforms are available, each offering unique features and functionalities tailored to different needs. Choosing the most suitable tool depends on factors such as the type of narratives you're analyzing, the complexity of your data, and your budget constraints.
Consider popular tools like NVivo and Atlas.ti. These platforms excel at organizing and analyzing qualitative data, enabling nuanced insights through coding and theme identification. Alternatively, Leximancer and Quirkos provide user-friendly interfaces ideal for less technical users, focusing on visual representation of data patterns. Conduct thorough research on each option, exploring their capabilities and how they align with your project goals. Ultimately, your choice of tools will shape the depth and richness of your AI-Driven Narrative Analysis, making it essential to choose wisely.
Discuss various tools that assist in AI narrative analysis:
Various tools assist in AI-driven narrative analysis, empowering researchers to streamline their qualitative projects. Among the most popular tools is NVivo, known for its robust capabilities in coding textual data and visualizing relationships. Atlas.ti stands out with its user-friendly interface, facilitating the organization of data while enabling collaboration among team members. Leximancer is another powerful option that aids in uncovering themes and patterns within narratives through text mining techniques.
Quirkos is particularly user-friendly, making it appealing for newcomers to qualitative research, providing an intuitive way to visualize data. Insight7 leverages AI to automate thematic analysis, reducing manual workload and increasing efficiency. Each of these tools enhances the research process by providing quicker insights and reliable analysis, ultimately leading to better, more informed decisions in qualitative research. Selecting the right tool can significantly improve the quality of your research outcomes while minimizing biases and inconsistencies.
- insight7
AI-Driven Narrative Analysis represents a transformative approach in qualitative research, offering innovative ways to explore complex narratives. By leveraging advanced algorithms, researchers can uncover hidden themes and insights embedded within qualitative data. This process initiates with meticulous data collection and preparation, ensuring that the material is suitable for AI interpretation.
Understanding narratives through AI allows for a level of scalability and efficiency previously unattainable. Researchers can analyze vast amounts of text, identify patterns, and generate actionable insights far quicker than traditional methods permit. AI's ability to synthesize findings enhances the research quality, enabling teams to focus on strategic decision-making. As researchers embrace this technology, they unlock the potential to derive deeper meanings from qualitative data, transforming how insights are generated and utilized in their respective fields. This demonstrates the profound impact AI can have on narrative analysis, ultimately enriching the overall research landscape.
- NVivo
The use of advanced software tools, such as NVivo, plays a crucial role in AI-driven narrative analysis. These tools empower researchers to efficiently categorize and analyze qualitative data, transforming raw narratives into structured insights. By enabling users to visualize complex relationships among themes, NVivo helps in uncovering deeper meanings and trends within the data. This capability enhances the interpretation of narratives, allowing researchers to address specific questions more effectively.
Moreover, utilizing NVivo for AI-driven narrative analysis streamlines the workflow by facilitating easier coding and annotation of diverse data sources. This enables qualitative researchers to spend less time on manual tasks and more on deriving valuable insights. The software's robust features, including data visualization and collaboration tools, further aid researchers in presenting their findings comprehensively. As researchers integrate NVivo into their methodologies, the process of narrative analysis becomes more efficient, enriching the overall research experience and outcome.
- Atlas.ti
In the landscape of AI-driven narrative analysis, selecting the right tool is essential for success. One such tool is designed to integrate seamlessly with qualitative research methodologies. This application allows researchers to organize and analyze qualitative data effectively, making it easier to extract meaningful insights from complex narratives.
The platform offers powerful features such as coding, visualization, and comprehensive reporting capabilities. These functionalities enhance the researcher's ability to identify patterns and themes within the data. Moreover, it enables collaborative work, allowing multiple researchers to contribute and access data in real-time. This collaborative aspect is crucial for enriching the narrative analysis process, as it combines diverse perspectives and expertise into a single, coherent analysis.
By utilizing AI-driven methods within this framework, researchers can significantly improve their efficiency and understanding of narrative data, ultimately leading to richer, more nuanced insights.
- Leximancer
Leximancer is a powerful AI tool designed for narrative analysis in qualitative research. It helps researchers visualize and interpret large sets of text data. By employing sophisticated algorithms, Leximancer identifies key themes and concepts, creating a cognitive map that displays the relationships between various topics. This process aids in the uncovering of underlying narratives effectively and efficiently.
Using Leximancer for AI-Driven Narrative Analysis involves several steps. First, researchers upload their data, allowing the algorithm to process it and generate a visual representation of the core themes. Next, the tool provides insights into the frequency and relational strength of different concepts, facilitating the extraction of more profound meanings. Finally, the findings can be exported into reports or presentations, streamlining the communication of insights. By integrating Leximancer into qualitative research, researchers can obtain a deeper understanding of narratives, ultimately enriching their analyses and conclusions.
- Quirkos
In the realm of qualitative research, tools that facilitate AI-driven narrative analysis have become essential. These tools provide researchers with a structured way to make sense of complex narratives. Specific software offers features designed to enhance qualitative analysis, enabling users to identify themes and patterns within textual data seamlessly. This streamlined approach saves time and enhances the reliability of insights gathered from qualitative research.
Understanding how these tools function can significantly impact research outcomes. They assist in organizing data, enabling easier retrieval and cross-referencing, which is crucial for developing a comprehensive narrative. Researchers can also visualize narratives through user-friendly interfaces, making it easier to grasp the connections between various data points. Overall, employing tools for AI-driven narrative analysis elevates the efficiency and depth of qualitative research, allowing for richer insights and more robust conclusions.
Step 3: Training AI Models to Understand Narratives
To effectively train AI models to understand narratives, it's crucial to first define the objectives of your analysis. The goal is to ensure that the AI can accurately identify themes, sentiments, and underlying messages within the narrative data. Begin by selecting a diverse set of narratives that represent various perspectives and styles. This diversity will aid in the model's ability to generalize when analyzing unseen data.
Next, implement supervised learning techniques by labeling key elements of the narratives. For example, mark sentiments, character motivations, or pivotal plot points. This labeled data serves as a foundation for training the AI. Additionally, utilize natural language processing algorithms that are adept at parsing linguistic nuances, which can enhance the modelโs interpretative abilities. Finally, iteratively test and refine the model on real-world data to improve accuracy and relevance. By following these steps, you'll foster a robust AI-driven narrative analysis, allowing for deeper insights into complex qualitative data.
Detail how to properly train AI models to comprehend and analyze narrative data.
Training AI models to understand and analyze narrative data involves several critical steps. First, it is essential to curate a diverse and relevant dataset that reflects the narratives to be analyzed. This dataset serves as the foundation upon which the AI learns. By exposing the model to various writing styles, contexts, and themes, researchers can enhance its capacity for comprehension and insight generation.
Next, pre-processing the data is crucial. This involves cleaning textual data by removing inconsistencies, errors, and irrelevant information. Tokenization and stemming also play a role in breaking down narratives into manageable segments for deeper analysis. Once the data is prepared, employing techniques like supervised learning can help train the AI to recognize patterns and sentiments within the narratives. Regular validation and refinement cycles ensure the model remains accurate and aligned with the intended analysis goals. Ultimately, effective training of AI models in this context opens new avenues for understanding complex qualitative data.
Conclusion: Harnessing the Power of AI-Driven Narrative Analysis
The integration of AI-driven narrative analysis signifies a pivotal advancement in qualitative research methodologies. By harnessing sophisticated algorithms, researchers can effectively interpret complex narratives that traditional methods may overlook. This approach enhances the depth of insights, fostering a richer understanding of human experiences captured in qualitative data.
AI-driven narrative analysis not only streamlines data processing but also uncovers patterns and trends essential for informed decision-making. Embracing this technology empowers researchers to elevate their analyses, enhancing both the accuracy and efficiency of their findings. Ultimately, the power of AI-driven narrative analysis transforms qualitative research, paving the way for impactful and actionable insights.
Summarize the transformative potential of AI-driven narrative analysis in advancing qualitative research methodologies.
AI-driven narrative analysis holds transformative potential for qualitative research methodologies by significantly enhancing the way researchers interpret narratives. By harnessing advanced algorithms, researchers can analyze large volumes of qualitative data more efficiently than traditional methods allow. This breakthrough enables the identification of patterns and themes that might have been overlooked due to human biases or time constraints.
The integration of AI tools fosters consistency and reliability in analyses, ensuring that insights are generated swiftly and accurately. This shift not only streamlines the research process but also enriches the depth of understanding drawn from narratives. As researchers adopt AI-driven approaches, they gain the ability to uncover nuanced insights, facilitating richer, more informed qualitative conclusions. Ultimately, these advancements allow for a more dynamic and insightful exploration of human experiences and perspectives within qualitative research.