AI-Powered Interview Tagging revolutionizes how researchers analyze customer interviews by automating the tagging process. Traditional methods often involve painstaking manual analysis, leading to biases and inconsistencies. In contrast, AI technologies streamline this process, allowing for faster reporting and more reliable insights. This approach transforms raw data into actionable themes, enhancing the quality of your research.
Understanding the capabilities behind AI-Powered Interview Tagging is essential for effective implementation. Natural Language Processing and machine learning algorithms enable tools to sift through extensive interviews and identify key themes with remarkable accuracy. As researchers increasingly rely on data-driven decisions, leveraging AI in interview tagging becomes critical for staying competitive and delivering valuable insights to stakeholders.
Analyze qualitative data. At Scale.
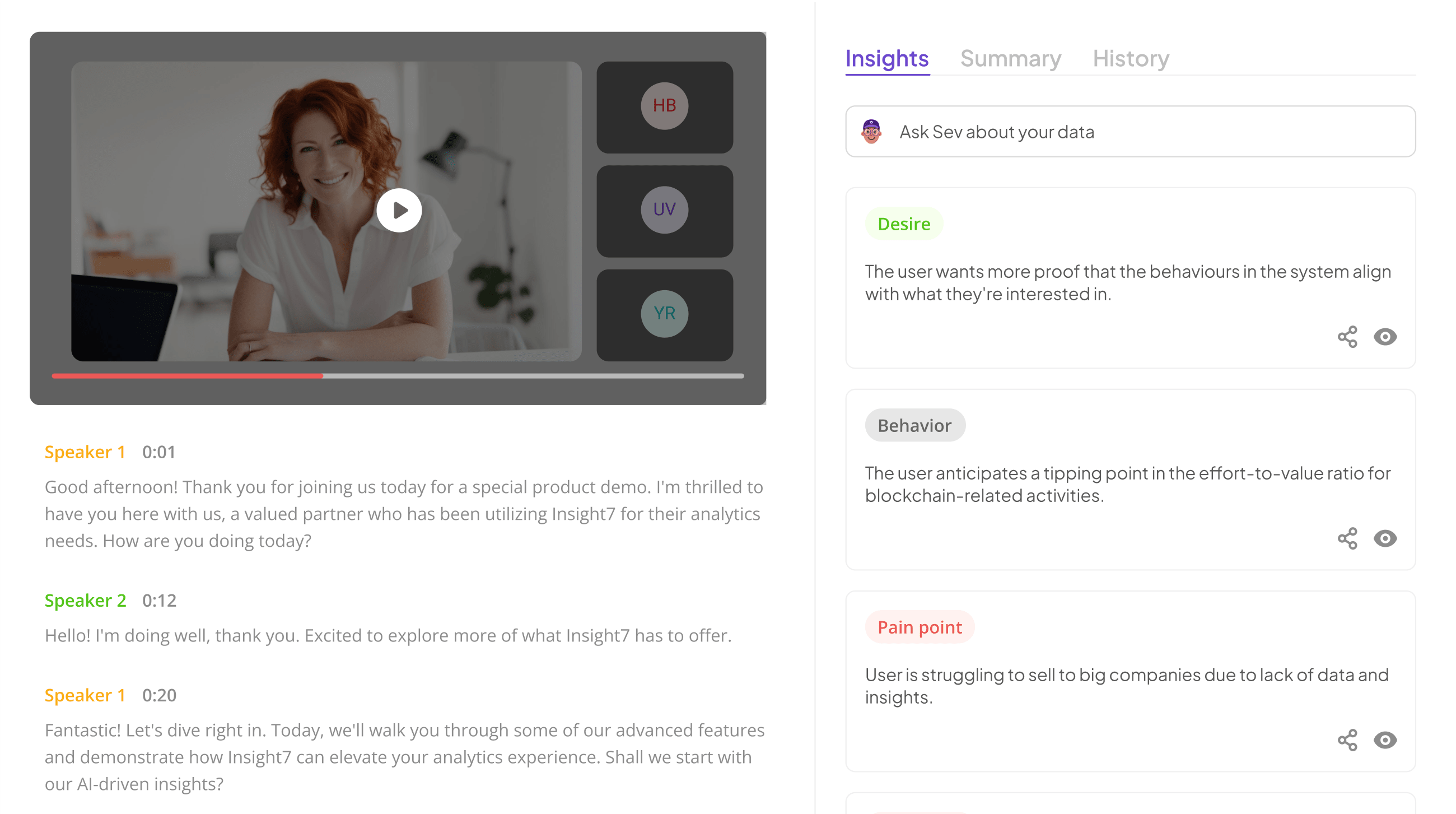
Identifying Research Themes with AI-Powered Interview Tagging
Identifying research themes with AI-Powered Interview Tagging begins by understanding how artificial intelligence can transform traditional interview analysis. The process harnesses advanced algorithms that sift through extensive interview data, pinpointing patterns and themes that may go unnoticed. This capability significantly reduces the time researchers spend manually combing through transcripts, allowing for quicker insights and delivering value to clients.
By implementing AI-Powered Interview Tagging, researchers can enhance the consistency of their findings. Human bias often creeps into manual analysis, but AI offers a more impartial lens through which to evaluate responses. This not only increases accuracy but also streamlines reporting. Ultimately, leveraging AI in this manner opens up new avenues for understanding customer sentiments and preferences, fostering a deeper connection between insights and actionable strategies for business growth.
Natural Language Processing (NLP): This technology facilitates the understanding of human language, enabling systems to analyze text effectively. NLP allows AI to recognize specific sentiments and themes within interviews.
Machine Learning Algorithms: These algorithms learn from data over time, improving the tagging process as more interviews are analyzed. By continuously refining its approach, the AI can enhance its accuracy in identifying recurring themes.
Incorporating these elements ensures a robust approach to tagging, leading to superior outcomes in research.
Understanding Core AI Capabilities
To effectively utilize AI-powered interview tagging, it is essential to understand the core capabilities of artificial intelligence. Natural Language Processing (NLP) allows systems to understand and interpret human language, making it invaluable for analyzing interview data. NLP enables the extraction of meaningful themes from customer dialogues, facilitating a clearer view of customer opinions and sentiments. This ability to comprehend language goes beyond mere keyword recognition, allowing AI tools to grasp context, intent, and nuances.
Additionally, machine learning algorithms play a significant role in identifying patterns within the data. By learning from previously tagged interviews, these algorithms continuously improve their accuracy, helping researchers recognize emerging themes over time. This adaptive flexibility is crucial for dynamic research environments where customer feedback evolves rapidly. Together, these core AI capabilities streamline the tagging process and enhance overall research efficiency. Understanding these elements empowers teams to effectively harness AI-powered interview tagging, transforming raw data into actionable insights.
- Natural Language Processing (NLP)
Natural Language Processing (NLP) plays a vital role in AI-Powered Interview Tagging, transforming raw data into actionable insights. By utilizing algorithms that understand and interpret human language, NLP enables researchers to identify critical themes from customer interviews efficiently. This technology can autonomously analyze text patterns, uncover sentiments, and classify feedback, facilitating the exploration of underlying trends in customer behavior.
The power of NLP lies in its ability to process vast amounts of conversational data quickly. It streamlines the tagging process, reducing the time required for manual analysis. Furthermore, it enhances the accuracy of identified themes, ensuring that subtle nuances in responses are captured. As a result, researchers can focus on deeper insights and strategic decisions rather than getting bogged down by repetitive tasks. Embracing NLP within the framework of AI-Powered Interview Tagging can profoundly enhance the research process and lead to more informed outcomes.
- Machine Learning Algorithms
Machine learning algorithms play a pivotal role in AI-powered interview tagging. These algorithms analyze language patterns, enabling the system to automatically categorize interviews based on identified themes. The effectiveness of this process largely stems from the algorithms’ capacity to learn from vast datasets, continuously improving their accuracy over time.
There are several key types of machine learning algorithms utilized in this context. First, supervised learning algorithms are trained on labeled datasets, allowing them to recognize specific themes in subsequent interviews. Second, unsupervised learning algorithms can uncover hidden patterns within the data without predefined labels, offering insights that might not have been initially considered. Lastly, reinforcement learning methods adapt based on feedback, enhancing the tagging process based on user interactions.
Incorporating these algorithms into your research enhances efficiency and accuracy, making AI-powered interview tagging an indispensable tool for understanding customer insights effectively.
Benefits of AI-Powered Tagging
AI-Powered Interview Tagging offers significant advantages that enhance how researchers analyze customer interviews for actionable insights. One major benefit is the efficiency and speed it brings to data analysis. Traditional methods can be time-consuming, requiring hours of manual coding and theme identification. In contrast, AI automates these processes, allowing researchers to focus on deriving insights rather than getting bogged down in data management.
Another essential advantage is the enhanced accuracy of theme identification. AI algorithms utilize Natural Language Processing to understand context and meaning, enabling them to uncover trends that might be overlooked in manual tagging. This precision not only improves the reliability of insights but also ensures that essential themes are highlighted effectively. By harnessing AI-Powered Interview Tagging, researchers can produce high-quality reports more quickly, transforming customer feedback into strategic decisions with greater confidence.
- Efficiency and speed in data analysis
AI-Powered Interview Tagging revolutionizes how researchers analyze customer interviews, enhancing both efficiency and speed in data analysis. Traditional methods often require extensive manual coding and lengthy review processes, consuming valuable time and resources. By integrating AI technologies, researchers can quickly identify relevant themes, allowing them to focus on deriving actionable insights rather than sifting through data.
One significant advantage of using AI in this context is the ability to analyze large datasets in real-time. With sophisticated algorithms, AI can automatically categorize interviews into predefined themes or even discover new ones based on context. This capability not only fast-tracks the analysis process but also improves accuracy, ensuring that vital customer sentiments are captured. Ultimately, the implementation of AI-Powered Interview Tagging transforms the research experience and propels organizations toward more informed decision-making.
- Enhanced accuracy in identifying themes
Incorporating AI-powered interview tagging significantly enhances the accuracy in identifying research themes. Traditional methods often leave room for human interpretation errors, leading to inconsistent results. AI-powered solutions utilize advanced Natural Language Processing to analyze vast amounts of interview data rapidly, ensuring that insights are systematically categorized. This automation not only speeds up the process but also minimizes bias, providing a reliable framework for extracting actionable themes.
Moreover, these AI systems can learn from previous tagging experiences, improving their accuracy with each iteration. For example, when insights are tagged, AI can identify patterns and nuances that might be overlooked by manual processes. By continuously refining its algorithms, AI-Powered Interview Tagging ensures that researchers receive accurate and relevant themes that truly reflect customer feedback. This leads to more informed decision-making and ultimately drives better business outcomes.
Extract insights from interviews, calls, surveys and reviews for insights in minutes
Step-by-Step Guide to Implementing AI-Powered Interview Tagging
Implementing AI-Powered Interview Tagging involves a systematic approach to transform qualitative data into actionable insights. To begin, it’s essential to prepare your data by collecting all relevant interviews and ensuring they are formatted correctly. This preparation step sets the foundation for successful tagging, making subsequent analysis smoother and more efficient.
Next, selecting the right tools is crucial. There are several options available such as Insight7, MonkeyLearn, and IBM Watson. Each tool offers unique features that cater to different needs, so choose one that aligns best with your project requirements. Once your tool is selected, you can implement the AI tagging process by setting up the application and running the analysis.
Finally, the analysis and interpretation of results complete the cycle. Review the tagged themes to understand emerging patterns and draw insights that can guide decision-making. By following these steps, you will harness the power of AI to streamline your interview tagging process and enhance your research outcomes.
Step 1: Preparing Your Data
To begin the process of AI-Powered Interview Tagging, preparing your data is essential. Start by collecting all relevant customer interviews in one centralized location. This could be audio, video, or text formats; however, ensuring that each interview is clearly labeled can save time later when analyzing themes.
Next, format and clean the data to ensure it is suitable for AI analysis. This involves transcribing audio or video recordings into text, removing any irrelevant content, and organizing the transcripts into a consistent structure. For example, separating themes or topics discussed in each interview can significantly enhance the tagging process. Once your data is organized, you're ready to move on to selecting the right tools for AI analysis, where the real insights will emerge from the neatly prepared data.
- Collecting interviews
Collecting interviews is a fundamental step in utilizing AI-Powered Interview Tagging effectively for research themes. Begin by identifying your target participants and framing questions that will elicit valuable insights. These interviews should be structured to allow for both quantitative and qualitative analysis, ensuring rich data for the tagging process.
Once you have conducted the interviews, the next phase involves organizing the collected data. Transcribing interviews accurately is crucial, as AI systems rely on authentic text for effective tagging and theme identification. It’s beneficial to employ a transcription tool that supports bulk uploads and maintains high accuracy.
After transcription, the resulting documents can be uploaded into your chosen AI system, allowing you to streamline the process of extracting themes. This comprehensive data collection method promotes deeper analysis and ultimately leads to enhanced decision-making based on the insights garnered through AI capabilities.
- Formatting and cleaning data
Cleaning and formatting your data is essential for effective AI-Powered Interview Tagging. Start by ensuring that the interview transcripts are consistent in style and structure. This means standardizing text formatting, correcting typos, and removing non-verbal cues that may confuse the AI. Consistent formatting allows the AI to efficiently parse the text and extract meaningful themes, thus enhancing the quality of the analysis.
Next, tackle any noise in your data. This includes eliminating irrelevant information or off-topic responses. Removing extraneous details ensures that the AI focuses on key insights that drive research outcomes. Additionally, consider segmenting conversations into distinct parts, such as questions and answers. This segmentation can help the AI identify patterns and themes more effectively. By investing time in proper data formatting and cleaning, you set the stage for more accurate AI tagging and deeper insights from your customer interviews.
Step 2: Choosing the Right Tools
Selecting the right tools for AI-powered interview tagging is crucial to streamline your research process. Not all platforms are created equal, so identify those that specialize in natural language processing and data analysis. Tools like IBM Watson and MonkeyLearn offer powerful capabilities, enabling you to extract themes efficiently. Understanding the features and benefits of each tool can help tailor the tagging process to your specific requirements.
When evaluating tools, consider factors such as ease of use, customization options, and integration with your existing systems. For instance, Tagtog allows for collaborative tagging, making it ideal for teams. Lexalytics offers sentiment analysis features, which can enhance your understanding of customer feedback. Ultimately, the right choice will support your goals in synthesizing insights from customer interviews, making the thematic analysis both efficient and insightful.
- insight7
AI-Powered Interview Tagging is a transformative method to streamline the analysis of customer interviews. It employs advanced technologies like Natural Language Processing and machine learning algorithms to efficiently parse through large volumes of data. This process is not just about speed; it significantly enhances the accuracy of theme identification, leading to richer insights that can inform strategic decisions.
To effectively implement AI-Powered Interview Tagging, it’s essential to follow certain steps. First, prepare your data by collecting interviews and ensuring they are properly formatted and cleaned. Next, choose the right tools suited for your needs, like MonkeyLearn or IBM Watson, which can automate the tagging process effectively. Once the tools are set up, run the analysis and meticulously review the tagged themes. This approach will allow you to draw actionable insights and fine-tune your strategies based on customer feedback. Embracing this method can lead to more informed, impactful research outcomes.
- MonkeyLearn
AI-Powered Interview Tagging can transform the way businesses analyze customer interviews. By integrating advanced AI tools, researchers can streamline the process of identifying key themes. This approach combines various capabilities, particularly Natural Language Processing (NLP) and machine learning algorithms, to enhance the tagging accuracy.
When you utilize these tools, you're not just increasing efficiency; you're also making data analysis more insightful. For instance, the machine learning component can learn from previous interviews and improve its tagging methods over time. As a result, teams get more precise insights from their interviews, allowing them to focus on what truly matters in customer feedback.
By adopting AI-powered interview tagging, organizations will be better equipped to derive actionable insights, ultimately leading to improved decision-making and enhanced customer understanding. Implementing such tools helps ensure that every interview contributes meaningful data to drive the research forward.
- IBM Watson
AI-powered interview tagging can significantly enhance your research process, particularly with advanced tools that harness natural language processing (NLP) and machine learning. This technology enables automated identification and categorization of themes from customer interviews, transforming raw data into actionable insights. By implementing such tools, organizations can improve the accuracy and efficiency of their data analysis efforts.
The exploration of various AI tools reveals the immense potential they hold in streamlining research initiatives. For instance, advanced machine learning algorithms can analyze large datasets and pinpoint emerging patterns, saving researchers valuable time. Furthermore, these tools help ensure consistency in tagging, minimizing human error. The integration of AI-powered interview tagging not only supports faster decision-making but also enhances the overall quality of insights derived from customer interactions. As organizations aim to improve their market understanding, these technologies can play a critical role in navigating complex data landscapes effectively.
- Lexalytics
Lexalytics offers an innovative approach to AI-powered interview tagging, simplifying the process of extracting key themes from customer interviews. By transforming spoken interviews into text through cutting-edge transcription technology, it creates a foundation for detailed analysis. Once transcribed, researchers can explore various insights using advanced features that facilitate the identification of thematic patterns and notable quotes.
The platform’s core functionality allows users to engage with data intuitively. After organizations upload their audio or video files, they can easily navigate through structured projects and libraries. This user-friendly interface ensures efficient workflow management while prioritizing data privacy and compliance. Overall, utilizing AI-powered interview tagging through this system allows businesses to derive meaningful insights from customer conversations, leading to better-informed decision-making and research initiatives.
- Tagtog
In the realm of AI-powered interview tagging, Tagtog stands out as a vital tool that streamlines the process of organizing and analyzing customer interviews. Utilizing advanced algorithms, it identifies key themes and insights within the text, allowing researchers to focus on the most pertinent information without getting lost in the details. By applying natural language processing, it categorizes responses effectively, making the analysis both efficient and insightful.
When using Tagtog, researchers begin by uploading their interview transcripts, which the platform then processes. This includes automating the tagging process based on predetermined themes or emerging patterns within the data. The result is a visual representation of insights that makes it easier to draw conclusions. As customers share their thoughts and feedback, leveraging this technology effectively can enhance research findings and drive informed decision-making. By employing such AI-powered tools, researchers can transform qualitative data into actionable insights with greater accuracy and efficiency.
Step 3: Implementing AI Tagging
In Step 3: Implementing AI Tagging, the focus shifts to operationalizing the AI-powered interview tagging process. This is a critical phase where the chosen tools come into play. Start by setting up your AI tagging tool according to your project’s specific needs. This setup involves configuring the model to recognize the unique language and themes present in your customer interviews. Ensure the system is calibrated to deliver accurate tagging by inputting sample data, allowing the AI to identify and learn from relevant patterns.
Following the setup, it’s time to run the analysis. Upload your collected interviews and allow the tool to process them. AI-powered interview tagging will analyze the content, extracting themes based on linguistic cues and sentiment analysis. This not only streamlines the identification of key insights but also enhances the overall efficiency of the research process. The insights generated from AI tagging can significantly inform your analysis and guide actionable strategies moving forward, setting the stage for deeper understanding and improved decision-making.
- Setting up the tool
To effectively initiate AI-Powered Interview Tagging, the first step involves preparing the necessary tools. Begin by selecting an AI platform that suits your specific needs. Options such as insight7 or IBM Watson can enhance the tagging process through advanced algorithms. Once you've chosen the right technology, create an account and familiarize yourself with its features. Configuring the tool properly is crucial for successful tagging; pay attention to settings related to data formats and language preferences.
Next, upload your collected interview data into the tool. Ensure that the data is formatted correctly and free from errors to achieve optimal results. The AI will analyze the content and begin to identify relevant themes and patterns. After the system processes the interviews, review the tags generated by the tool. This careful examination helps validate the analysis and enables you to extract meaningful insights from your customer interviews, leading to actionable research outcomes.
- Running the analysis
Running the analysis involves the critical step of examining the data you've collected from customer interviews. Begin by implementing your AI-Powered Interview Tagging tool, which automates the process of extracting themes from the raw data. This ensures a systematic approach to identifying relevant insights while saving time and increasing reliability.
Next, consider customizing your tagging framework. Define your themes to align with your specific research goals, such as customer satisfaction or product usability. Each theme can then be coded accordingly, making it easier to organize and interpret the data. The AI will analyze the themes and provide sentiment insights, helping you understand participants' feelings toward specific topics. This structured analysis not only simplifies the process but also enhances the overall quality of insights drawn from customer interactions.
Step 4: Analyzing and Interpreting Results
In the process of AI-Powered Interview Tagging, analyzing and interpreting results is crucial for deriving meaningful insights from your interviews. Once your interviews are tagged with relevant themes, the next step is to review these tags closely. Identify patterns that emerge among the tagged data, focusing on the themes that resonate most with your research objectives. This stage is essential as it allows you to filter through the noise, ensuring that your analysis aligns with your original goals.
Next, draw insights and conclusions based on the coded themes. Utilize sentiment analysis to gauge participants' feelings and attitudes towards specific topics. By clicking into individual themes, you will discover detailed insights, including quotes supporting each theme. This method helps validate your findings, linking them back to the original interview transcripts. Ultimately, the goal is to transform raw data into actionable insights that inform strategic decision-making and improve overall outcomes.
- Reviewing tagged themes
To effectively review tagged themes, it’s important to engage with the insights generated from your AI-Powered Interview Tagging process. Each theme identified during the analysis represents a core aspect of customer feedback that can reveal significant trends and sentiments. In this stage, you can delve deeply into the nuances of each theme, extracting specific pain points and opportunities as illustrated through customer quotes and commentary.
As you evaluate the tagged themes, consider the context in which insights were gathered. This helps to ensure that interpretations are accurate and relevant. You can also customize the themes to better match your project's goals and adjust the categorization based on emerging patterns. By summarizing the findings, you create a structured overview that informs decision-making and strategic direction, ultimately leading to more customer-centric strategies. Each click can reveal essential details, making the process engaging and insightful while ensuring that the focus remains on turning data into actionable recommendations.
- Drawing insights and conclusions
In this section, we delve into the critical phase of drawing insights and conclusions from your tagged interviews. With the use of AI-powered interview tagging, collected data is organized into meaningful themes, enabling a clearer understanding of customer sentiments and feedback. By categorizing insights effectively, the analysis process transforms complex data into actionable conclusions that guide future strategies.
Once the themes are established, it becomes essential to interpret the findings accurately. Look for patterns and relationships among the insights to identify significant areas of opportunity or concern. This comprehensive assessment will provide a solid foundation for decision-making, ensuring that you are equipped to address customer needs and enhance experiences in your organization.
Conclusion: Embracing AI-Powered Interview Tagging for Better Research Outcomes
Incorporating AI-Powered Interview Tagging into your research process can significantly enhance the efficiency and accuracy of theme identification. By automating the analysis of interviews, teams can minimize inconsistencies and biases that often arise during manual evaluations. This shift not only speeds up the delivery of insights but also allows researchers to focus on interpreting data rather than just gathering it.
As the demand for quick and actionable insights continues to grow, embracing AI technology becomes imperative. This approach transforms a traditionally tedious task into a streamlined process, ensuring that research outcomes are not only timely but also reliable. Ultimately, AI-Powered Interview Tagging offers a pathway to deeper understanding and better decision-making in research endeavors.