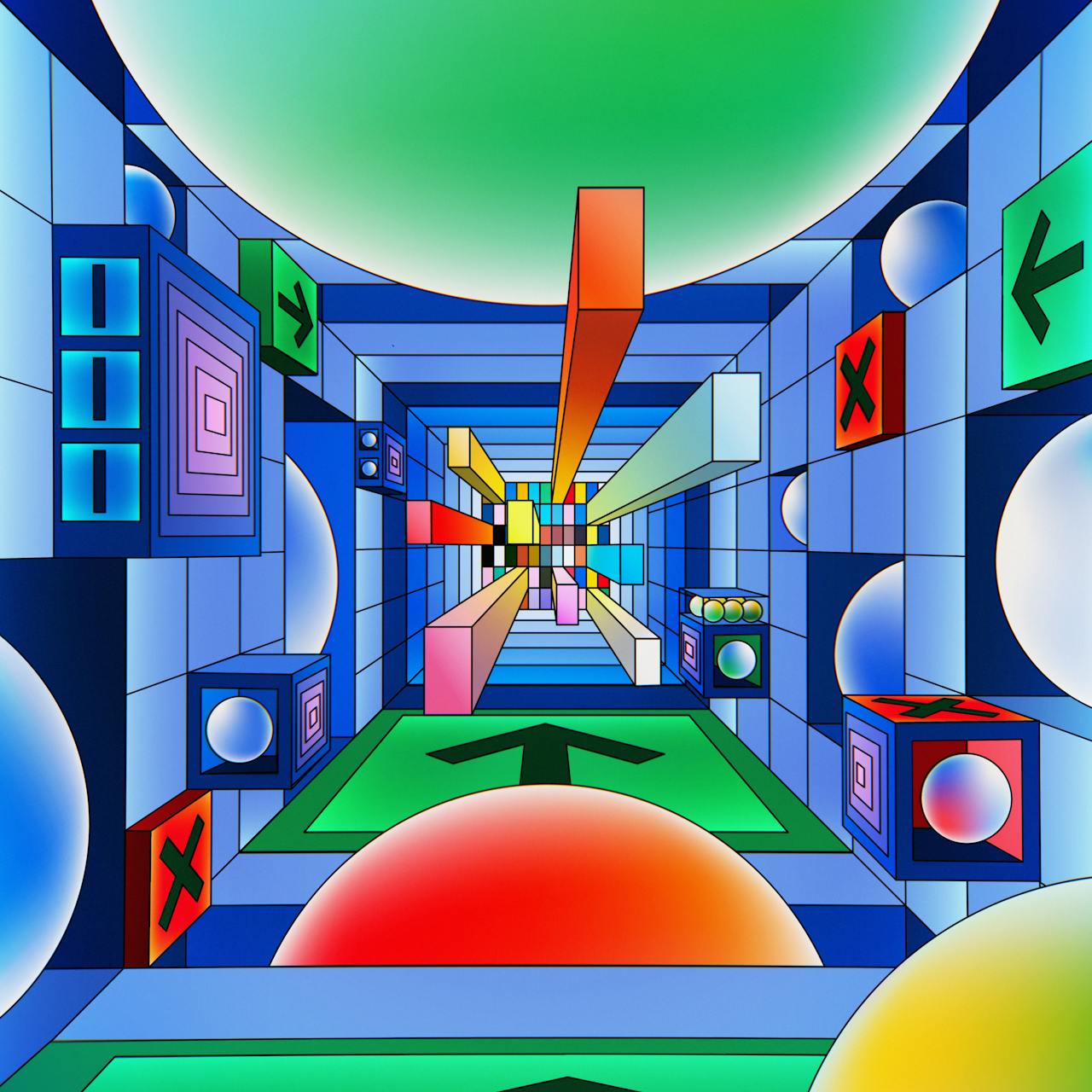
What is Customer Data Analysis
Customer data analysis is the process of examining and interpreting data related to customer interactions, behaviors, preferences, and transactions to derive actionable insights. This analysis involves collecting, organizing, and analyzing various types of customer data to better understand customer needs, improve marketing strategies, enhance product offerings, and optimize the overall customer experience.The process of customer data analysis typically involves several key steps:
- Collection: Gathering relevant customer data from various sources such as transactional records, website analytics, social media interactions, customer surveys, and CRM systems.
- Data Cleaning and Preparation: Preprocessing the collected data to ensure accuracy, consistency, and completeness. This may involve removing duplicates, correcting errors, and formatting the data for analysis.
- Exploratory Data Analysis (EDA): Exploring the dataset to identify patterns, trends, and relationships among different variables. Techniques such as data visualization, descriptive statistics, and correlation analysis are commonly used during this phase.
- Segmentation: Dividing customers into distinct groups or segments based on common characteristics or behaviors. Segmentation allows businesses to target specific customer segments with tailored marketing strategies and personalized offerings.
- Predictive Modeling: Building statistical models or machine learning algorithms to predict future customer behavior, such as purchase likelihood, churn risk, or product preferences. Predictive modeling enables proactive decision-making and targeted interventions to drive desired outcomes.
- Performance Evaluation: Assessing the effectiveness of marketing campaigns, product launches, or customer engagement initiatives based on key performance metrics and KPIs derived from the analyzed data.
- Continuous Improvement: Iteratively refining data analysis techniques, models, and strategies based on feedback and insights gained from ongoing customer interactions and market dynamics.
What Insights Can Business Generate From Customer data analysis?
– Identify high-value customers and tailor marketing efforts to retain and upsell them.
– Personalize the customer experience through targeted recommendations, promotions, and communication channels.
– Optimize VC pricing strategies, product assortments, and inventory management to meet customer demand.
– Improve customer satisfaction, loyalty, and retention by addressing pain points and enhancing service quality.
– Anticipate market trends, competitor movements, and emerging customer needs to stay ahead of the competition.
Overall, customer data analysis is a powerful tool for businesses to gain a deeper understanding of their customers, drive data-driven decision-making, and ultimately, achieve sustainable growth and profitability.
4 Types of Customer Data
Customer data can be categorized into four main components of customers: demographic data, behavioral data, psychographic data, and transactional data. Each type provides valuable insights into the characteristics, preferences, and purchasing habits of customers.
-
Demographic Data
Demographic data encompasses quantifiable characteristics of customers such as age, gender, income, education level, marital status, and geographic location. This information helps businesses segment their target audience and tailor marketing strategies accordingly.
For instance, a company selling luxury goods might target affluent individuals with higher income levels, while a budget-friendly brand might focus on younger consumers with limited disposable income.
-
Behavioral Data
Behavioral data refers to the actions and interactions of customers with a company’s products or services. This includes purchase history, browsing behavior, engagement with marketing campaigns, website visits, and product usage patterns.
Analyzing behavioral data enables businesses to understand customer preferences, identify trends, and personalize the customer experience. For example, an e-commerce platform may use purchase history to recommend products similar to those previously bought by a customer, increasing the likelihood of a repeat purchase.
-
Psychographic Data
Psychographic data delves into the psychological and lifestyle aspects of customers, including their interests, values, attitudes, beliefs, and personality traits. This information provides deeper insights into consumer motivations and helps businesses create more targeted and relevant marketing messages.
For instance, a fitness apparel brand might tailor its messaging to appeal to health-conscious individuals who value an active lifestyle, leveraging psychographic data to resonate with its target audience effectively.
-
Transactional Data
Transactional data pertains to the financial transactions between customers and a business, including purchase history, order frequency, average order value, and payment methods used. Analyzing transactional data allows businesses to identify buying patterns, forecast demand, and optimize pricing strategies.
Moreover, it enables the implementation of loyalty programs, personalized promotions, and targeted cross-selling or upselling efforts. For example, a supermarket chain may use transactional data to offer personalized discounts to frequent shoppers or suggest complementary products based on past purchases.
Understanding Research Matrix
A research matrix is a systematic framework used to organize, analyze, and synthesize data from multiple sources. It consists of a grid or table format where data points are categorized based on various criteria such as demographics, psychographics, behaviors, and preferences. By structuring data in this way, businesses can gain a holistic view of their customers and identify correlations, outliers, and insights that might otherwise go unnoticed.
Benefits of Using a Research Matrix for Customer Data Analysis
- Comprehensive Data Organization: A research matrix allows businesses to organize vast amounts of customer data into a structured format, making it easier to manage and analyze.
- Holistic Customer Insights: By categorizing data across different dimensions, businesses can gain a comprehensive understanding of their customers’ demographics, preferences, and behaviors.
- Identification of Patterns and Trends: The structured nature of the research matrix enables businesses to identify patterns, trends, and correlations within their customer data, leading to actionable insights.
- Data-Driven Decision Making: Armed with insights derived from the research matrix, businesses can make informed, data-driven decisions that drive growth and innovation.
- Enhanced Targeting and Personalization: By understanding their customers at a deeper level, businesses can tailor their marketing efforts and offerings to better meet individual needs and preferences.
Implementing the Research Matrix in Customer Data Analysis
- Define Objectives and Research Questions: Before constructing a research matrix, it’s essential to clearly define the objectives and research questions that will guide the analysis. What specific insights are you hoping to uncover? What aspects of customer behavior or preferences are you interested in exploring?
- Gather Relevant Data: Collect data from various sources, including customer surveys, transaction records, website analytics, social media insights, and third-party databases. Ensure that the data is relevant to your research objectives and covers a diverse range of customer attributes and behaviors.
- Design the Matrix Structure: Determine the dimensions and categories that will form the basis of your research matrix. Common dimensions include demographics (age, gender, location), psychographics (lifestyle, values, interests), behavioral attributes (purchase history, browsing behavior), and preferences (product preferences, communication channels).
- Populate the Matrix: Input the collected data into the research matrix, organizing it according to the predefined dimensions and categories. This may involve data cleaning, normalization, and aggregation to ensure consistency and accuracy.
- Analyze and Interpret Insights: Once the matrix is populated, analyze the data to uncover patterns, trends, and insights. Look for correlations between different dimensions, identify outliers, and extract actionable insights that can inform strategic decision-making.
- Iterative Refinement: Customer data analysis is an iterative process, and the research matrix should be continually refined and updated as new data becomes available or research objectives evolve. Regularly review and revisit the matrix to ensure its relevance and effectiveness in driving insights.
Case Study: Applying the Research Matrix in E-commerce
Let’s consider a hypothetical e-commerce retailer looking to enhance her customer data analysis using a research matrix. The retailer’s objectives include understanding customer demographics, identifying purchasing patterns, and improving product recommendations.
By constructing a research matrix and populating it with data from various sources, including transaction records, customer surveys, and website analytics, the retailer gains valuable insights into her customer base. For example, the matrix reveals that female customers aged 25-34 are the retailer’s most valuable segment, with a preference for eco-friendly products and a propensity for repeat purchases.
Armed with this knowledge, the retailer can tailor her marketing efforts and product offerings to better meet the needs and preferences of this key demographic, leading to increased customer satisfaction and loyalty.
Simpler Way to Perform Customer Data Analytics and Generate Research Matrix With Insight7
- Log into the Insight7 app
- Create an account
- Click on “Create” and select an option in the dropdown menu
- Upload your interview file
- Generate transcript from the video or audio file
- From there you can analyze and use the research matrix feature to extract insights.
Conclusion
It goes without saying that the ability to effectively analyze customer data is critical to success. By leveraging the power of the research matrix, businesses can gain deeper insights into their customers, identify trends and patterns, and make informed, data-driven decisions that drive growth and innovation. Through implementation of the practical strategies outlined in this guide, businesses can unlock the full potential of their hcustomer data and gain a competitive edge in the marketplace.