Interview Response Clustering allows organizations to transform raw feedback into actionable insights. As businesses increasingly rely on user interviews, the challenge lies in effectively interpreting and organizing vast amounts of qualitative data. This process not only enhances comprehension but also empowers stakeholders to make informed decisions.
By employing AI techniques, Interview Response Clustering streamlines the extraction of themes and patterns from various responses. Understanding how to segment these insights according to specific use cases ensures that organizations can address diverse objectives, ultimately driving strategic growth. Through this section, we will explore the significance of Interview Response Clustering and its role in improving analysis efficacy.
Analyze & Evaluate Calls. At Scale.
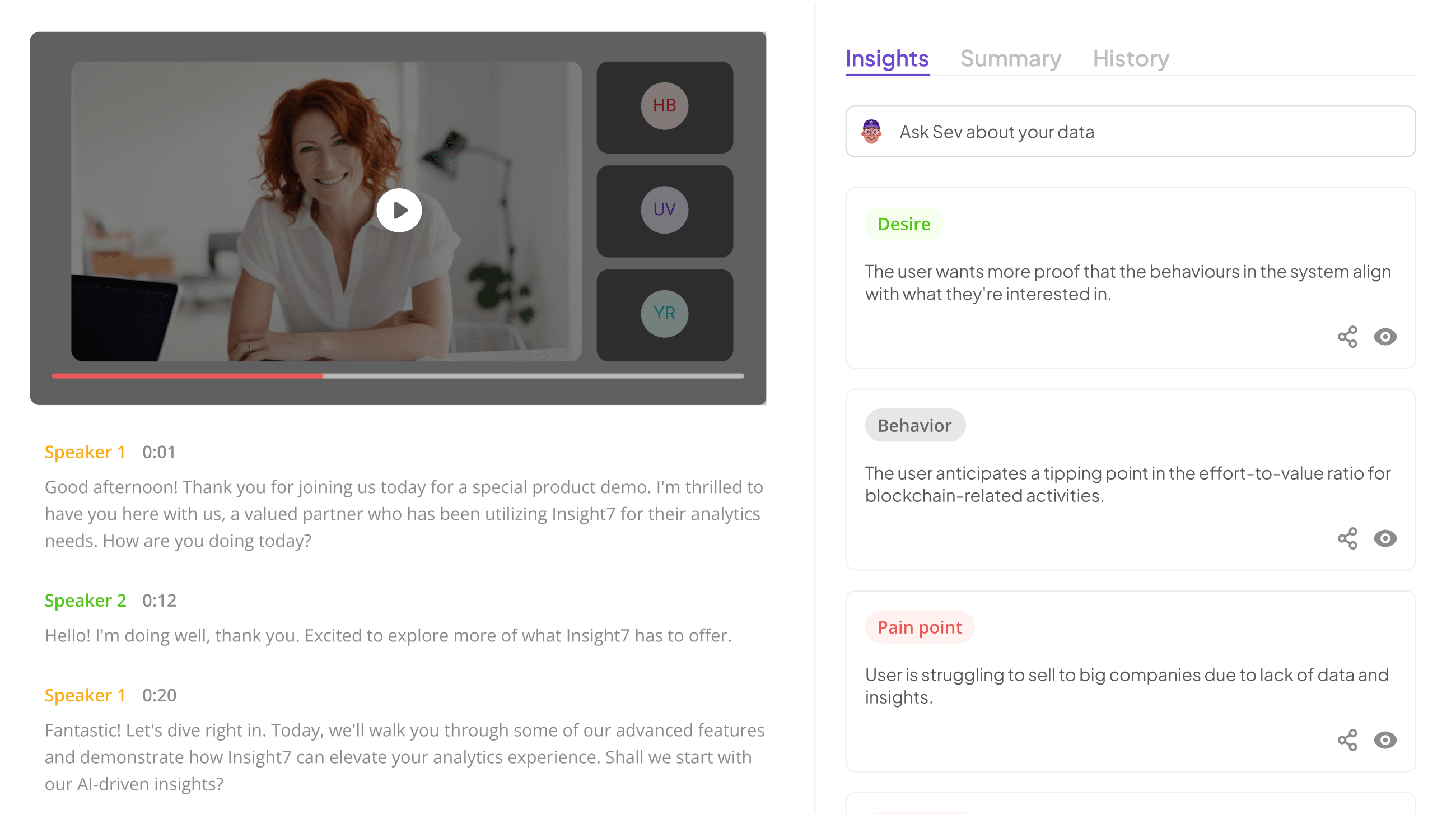
Understanding the Basics of Interview Response Clustering
Interview Response Clustering is a structured approach to organize qualitative data from interviews into meaningful groups. This process is essential for researchers seeking to simplify the analysis of verbal responses, making it easier to extract valuable insights. By clustering responses, teams can identify recurring themes, patterns, and sentiments, which contributes greatly to a more comprehensive understanding of the data.
The effectiveness of Interview Response Clustering is amplified when combined with AI technologies. AI tools can streamline the clustering process, significantly reducing the time and effort required for manual analysis. Automated systems can categorize responses with great accuracy, minimizing human bias and ensuring consistency across the analysis. By employing AI in this manner, teams can not only enhance the quality of their insights but also meet the growing demand for rapid reporting in qualitative research. Ultimately, understanding and implementing Interview Response Clustering can lead to more informed decision-making and ultimately drive successful outcomes in various business contexts.
What is Interview Response Clustering?
Interview Response Clustering is a methodology that categorizes responses from interviews into meaningful groups. This process helps researchers identify common themes and insights within qualitative data. By sorting responses, organizations can streamline their analysis, reduce bias, and generate actionable findings more efficiently.
When employing Interview Response Clustering, the initial step involves collecting a diverse set of interview responses. Next, AI algorithms analyze these responses to uncover patterns, similarities, and distinct differences. Clustering enables teams to bring together related insights, which can lead to more informative and quick decision-making processes. Overall, utilizing Interview Response Clustering enhances the quality of insights derived from interviews, making it a vital tool in market research and user experience studies.
Why Use AI for Interview Response Clustering?
Organizations increasingly face the challenge of managing large volumes of interview data, making manual analysis both inefficient and error-prone. Interview response clustering addresses these challenges by grouping similar responses, thus revealing insights that may go unnoticed in the chaos of unstructured data. AI significantly enhances this process by automating clustering, ensuring consistency and speed in data analysis.
With AI, you not only save time but also reduce biases that can arise from human interpretation. This technology helps identify patterns and themes across vast datasets seamlessly, enabling more informed decisions based on comprehensive analysis. Furthermore, AI-driven clustering can adapt to various use cases, tailoring insights to specific needs. Leveraging AI in interview response clustering thus emerges as a solution that streamlines analysis while maintaining high-quality insights. In the end, adopting AI technologies enhances accuracy and empowers teams to deliver timely, relevant insights to stakeholders.
[ hfe_template id=22694]Implementing AI for Grouping Interview Responses by Use Case
Implementing AI for grouping interview responses by use case can radically transform how insights are extracted. This process begins with the preprocessing of responses, where clean and compliant data is essential. Text normalization and removal of irrelevant information set the foundation for effective analysis. Once the data is pristine, AI models can be applied to identify common themes and group similar responses, facilitating faster decision-making.
After clustering the data, the next critical step is to analyze these clusters for specific use cases. Different responses can emerge, revealing unique perspectives that can inform strategies or product developments. The insights drawn from grouping interview responses enhance not only the speed of report generation but also improve the consistency and reliability of findings. Utilizing tools designed for these tasks can help streamline the process and provide a clearer picture of user sentiment and needs, ultimately driving better business outcomes.
Step-by-Step Guide to AI-Driven Interview Response Clustering
To effectively group interview responses by use case, a systematic approach is essential. This step-by-step guide to AI-driven interview response clustering will outline the necessary process, beginning with preprocessing your data to ensure clarity and consistency. Proper data preparation helps mitigate potential biases and enhances the overall quality of insights drawn from the interviews.
Next, applying AI models plays a crucial role in identifying patterns within the responses. Advanced algorithms can automatically categorize the data, significantly speeding up the analysis process and providing deeper insights. Finally, analyzing the generated clusters will help you uncover specific use cases, allowing your team to draw actionable conclusions from the grouped information. By following this structured approach, organizations can streamline their analysis and improve the reliability of their insights, ultimately leading to more informed decision-making.
- Preprocessing Responses
Preprocessing responses is a crucial step in the interview response clustering process. Initially, it involves sifting through raw data to ensure its usability for analysis. This preparation may include cleaning the text by removing irrelevant details, fixing typos, and converting speech-like patterns into a more structured format. Proper preprocessing establishes a solid foundation, allowing for accurate insights and thematic analysis.
Once the data is cleaned, the next phase focuses on organizing responses categorically based on similarities and contrasting themes. This stage plays a vital role in reducing bias and enhancing consistency across interpretations. By systematically grouping interview content, you set the stage for applying AI models effectively, enabling you to extract relevant insights tailored for specific use cases. This structured approach ensures that response clustering is both efficient and thorough, ultimately leading to quicker and more reliable results.
- Applying AI Models
Applying AI models is essential for effective interview response clustering. By utilizing AI, you can sift through large volumes of responses with impressive speed and accuracy. Start by preprocessing the data, which cleans and organizes it, ensuring that it is ready for analysis. This initial step is vital, as the quality of your input directly affects the output generated by the AI system. Implementing machine learning algorithms can then identify patterns and themes within the responses, allowing for efficient grouping by use cases.
Once the AI models are applied, the clustering process reveals insights that might have otherwise gone unnoticed. These insights allow stakeholders to better understand the respondentsโ perspectives and the underlying needs. By analyzing the generated clusters, businesses can tailor their strategies according to specific use cases, enhancing the overall decision-making process. Integrating AI effectively ensures that interview response clustering is not just a theoretical concept but a practical tool for actionable insights.
- Analyzing Clusters for Use Cases
Analyzing clusters for use cases involves interpreting grouped interview responses to extract meaningful insights. By arranging responses into defined themes, organizations can identify patterns that align with their specific objectives. This helps transform qualitative data into actionable strategies that can enhance user experiences and address challenges effectively.
To efficiently carry out this analysis, consider the following steps:
Define Themes: Establish specific themes or tags based on your goals, such as user satisfaction or operational challenges. This ensures that the insights are pertinent.
Utilize AI Tools: Leverage AI capabilities to automate the analysis process. Tools can identify trends within clusters, allowing you to focus on the most relevant responses.
Sentiment Analysis: Gauge the sentiment associated with each theme to understand usersโ feelings, guiding decisions about enhancements or interventions.
Trace Evidence: Maintain a connection to original responses, which allows for deeper inquiry into specific quotes or insights.
Refine Insights: Continuously iterate and refine your themes based on ongoing analysis, ensuring that your findings remain relevant and actionable.
These steps not only streamline the analysis process but also provide a framework for transforming clustered interview responses into valuable insights that support targeted decision-making.
Top AI Tools for Interview Response Clustering
The process of Interview Response Clustering can be greatly enhanced with the right AI tools. Several platforms excel in sorting and organizing qualitative data. Utilizing these tools allows researchers to quickly analyze and categorize responses, saving considerable time. Here are some top AI tools to consider for this purpose.
- Insight7: Known for its user-friendly interface, Insight7 helps streamline the clustering of responses while maintaining accuracy.
- MonkeyLearn: This tool specializes in text analysis, enabling users to automatically classify and cluster interviews effectively.
- SPSS Text Analytics: A powerful platform that offers in-depth statistical analysis and clustering capabilities for qualitative data.
- RapidMiner: With robust machine learning capabilities, this tool assists users in gaining insights through advanced interview response clustering.
- Google Cloud Natural Language: Utilizing Google's AI, this tool helps in understanding the context of responses, enhancing the clustering process.
Leveraging these tools can significantly elevate the quality and speed of your qualitative analysis, making it easier to derive actionable insights from interview responses.
- Insight7
In the realm of Interview Response Clustering, the objective is to distill diverse feedback into actionable insights that align with distinct use cases. By employing artificial intelligence, teams can efficiently categorize and analyze qualitative data, transforming it from a scattered collection of thoughts into organized clusters that reveal meaningful patterns. This approach goes beyond mere data organization; it enhances understanding and decision-making by pinpointing specific areas for improvement or potential innovation.
To effectively implement Interview Response Clustering, itโs essential to follow a systematic process. Start by preprocessing responses to remove noise and ensure consistency. Next, leverage advanced AI models to automatically group the data into relevant categories. Finally, analyze the resulting clusters to draw connections to your core use cases. By embracing this structured methodology, you pave the way for a clearer path to strategic insights and a stronger alignment between customer feedback and your organizationโs goals.
- MonkeyLearn
In the realm of preparing to analyze interview responses, choosing the right AI tool can greatly enhance your approach to Interview Response Clustering. An ideal platform swiftly organizes and categorizes qualitative data, letting you identify patterns and insights across various use cases. Such capabilities allow teams to derive actionable information from raw conversational data, ultimately leading to more strategic decision-making.
A notable feature of effective tools is the ability to preprocess responses. This includes removing unnecessary information and standardizing inputs for better clarity. Additionally, applying advanced AI models strengthens the accuracy of clustering, enabling a seamless grouping of similar responses. After clustering, the real work begins as you dive into analyzing these groups, ready to uncover essential use cases that inform your strategies. By focusing on these stages, your conversations can evolve into valuable insights tailored for your goals.
- SPSS Text Analytics
SPSS Text Analytics offers robust features that enhance the process of Interview Response Clustering. By using powerful statistical methods, SPSS can transform qualitative responses into quantifiable insights. The tool allows researchers to define themes and codes, enabling a more structured analysis of interview data. Once the data is organized into clusters based on these themes, SPSS provides a sentiment analysis to gauge participant feelings and opinions effectively.
To implement SPSS Text Analytics, users begin by preprocessing their interview responses to clean and organize the data. Afterward, SPSS applies sophisticated AI models to identify patterns and unique insights within the responses. By analyzing these clusters, researchers can derive meaningful conclusions that align with specific use cases. Overall, SPSS Text Analytics serves as a critical asset for anyone looking to streamline their analysis of interview responses, driving efficiency and accuracy in data interpretation.
- RapidMiner
RapidMiner serves as a powerful tool for Interview Response Clustering, streamlining the analysis of qualitative data. When dealing with numerous interview responses, traditional methods can become overwhelming. This is where advanced algorithms and automated processes come into play, enabling users to categorize and analyze responses efficiently.
Using this platform, you can preprocess responses to enhance data quality. This involves techniques like tokenization and sentiment analysis, turning raw responses into structured insights. Once the data is ready, you can apply AI models to group similar responses into clusters based on themes or use cases, revealing underlying patterns that may not be immediately obvious. By analyzing these clusters, you gain valuable insights into user perspectives, aiding in decision-making and strategy development across various projects. Embracing such AI-driven methodologies allows for deeper understanding and insights from interview responses, ultimately enhancing the quality of outcomes.
- Google Cloud Natural Language
Google Cloud Natural Language offers powerful tools for analyzing interview responses and enabling effective response clustering. By leveraging its natural language processing capabilities, you can efficiently group interview data based on thematic similarities and use cases. This functionality is essential for gaining insights into user sentiment and experience, ultimately assisting in decision-making processes.
A critical aspect of using this platform is its ability to preprocess and analyze large volumes of text data. It categorizes responses, extracts key phrases, and identifies sentiment, allowing you to create a comprehensive overview of the feedback received. Consequently, when compiling varied interview responses, you can pinpoint major use cases and align insights for targeted improvements. Through the use of advanced AI models and deep learning, the nuances within your data can be uncovered, paving the way for more informed, data-driven strategies in your organization.
Conclusion on Interview Response Clustering
Successfully implementing Interview Response Clustering can significantly enhance the efficiency of data analysis. By utilizing AI to categorize responses, teams can quickly glean insights and streamline reports, meeting the rising demand for timely information. This not only saves time but also mitigates biases that may arise from manual interpretations.
Moreover, the insights derived from grouped responses lead to clearer use cases and informed decision-making. As organizations navigate an increasingly complex landscape, mastering Interview Response Clustering with AI will serve as a competitive advantage. Embracing this approach is essential for ensuring that qualitative data translates into actionable strategies.