Research interviews often unveil a wealth of information, but they can also reveal conflicting insights that complicate the analysis process. Insight Discrepancy Analysis is crucial in navigating this complexity. By systematically identifying and addressing discrepancies, researchers can uncover deeper truths that arise from diverse perspectives and experiences among interviewees.
Understanding these distinctions enhances the overall validity of research findings. When researchers recognize and analyze conflicting insights effectively, they can sift through biases and assumptions that may cloud judgment. This careful examination not only streamlines reporting but also enriches the overall understanding of the subjects being studied. Thus, Insight Discrepancy Analysis forms a foundational element of rigorous research practices.
Analyze qualitative data. At Scale.
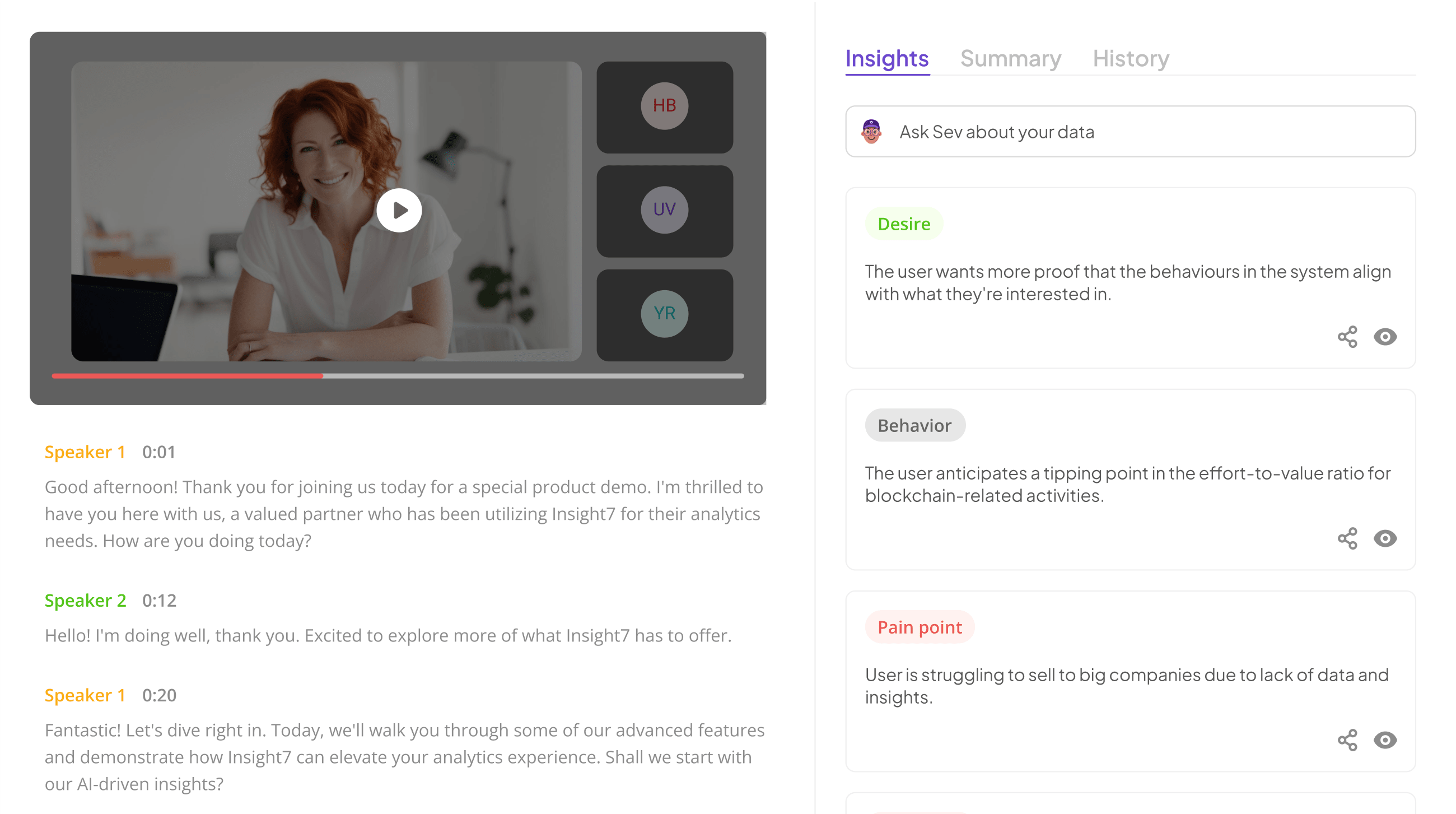
Identifying Insight Discrepancy: Key Steps in the Analysis Process
To effectively conduct Insight Discrepancy Analysis, it is crucial to adopt a systematic approach that addresses conflicting insights from research interviews. The initial step involves collecting diverse perspectives. Gathering multiple viewpoints enriches your understanding and minimizes bias. Ensure that your sample includes various demographics and experiences relevant to the research topic, thereby capturing a full spectrum of insights.
Next, focus on recognizing patterns and anomalies within the collected data. Employing qualitative analysis techniques will help you identify consistent themes among responses. Utilize tools powered by AI to facilitate a deeper examination of any anomalies that arise. This dual approach not only aids in distilling the essential insights but also highlights conflicting opinions, leading to a more nuanced interpretation of the data. Ultimately, navigating potential discrepancies with clear, structured analysis enhances the overall validity of your research findings.
Step 1: Collecting Diverse Perspectives
Collecting diverse perspectives is crucial for effective Insight Discrepancy Analysis. In this initial step, it's essential to gather opinions that span a variety of backgrounds, experiences, and viewpoints. This diversity enriches the understanding of different themes and issues within the research interviews. By hearing from various stakeholders—such as customers, employees, and industry experts—you can uncover insights that might otherwise remain hidden when only a homogenous group is consulted.
To ensure a well-rounded collection of views, consider employing strategies like targeted outreach to underrepresented populations, as well as structured interviews that encourage open dialogue. Utilize techniques such as creating diverse interview panels, ensuring participants feel comfortable sharing differing opinions, and employing technology to capture a wide range of thoughts. This multifaceted approach will facilitate a deeper analysis of conflicting insights and ultimately enhance the quality of your research findings.
- Importance of gathering multiple viewpoints
Gathering multiple viewpoints is crucial for conducting thorough Insight Discrepancy Analysis in research interviews. When different perspectives are collected, they create a more comprehensive understanding of the subject matter. Diverse viewpoints allow researchers to identify contradictions and validate findings, ultimately enriching the data quality. Moreover, engaging individuals from various backgrounds can reveal hidden insights that might not emerge from a more homogenous group. This diversity fosters innovation and avoids confirmation bias, ensuring a well-rounded approach to research.
To implement this effectively, consider several strategies: first, target varied demographics, including varying ages, industries, and experiences. Second, employ different methods of data collection, such as face-to-face interviews, online surveys, and focus groups. Third, ensure that interview questions are open-ended to encourage expansive responses. By incorporating these methods, researchers can achieve a richer data set that informs decision-making and enhances the strategic relevance of the insights gathered.
- Techniques for ensuring a diverse sample of interviewees
To ensure a diverse sample of interviewees, it is crucial to adopt targeted selection strategies. Begin by identifying the relevant demographic characteristics that reflect the population under study. Consider age, gender, socioeconomic status, and geographic location to capture varied perspectives. Additionally, implement outreach methods that engage underrepresented groups, such as community organizations or social media campaigns.
Another effective technique is to use stratified sampling, which divides the population into distinct subgroups. This method helps ensure that all subcategories are represented proportionately, aiding the Insight Discrepancy Analysis. Furthermore, conducting follow-up interviews with participants from diverse backgrounds not only deepens understanding but also allows for cross-comparison of insights. By prioritizing diversity in your sample, you will uncover richer data and mitigate biases, ultimately leading to more nuanced findings in your research.
Step 2: Recognizing Patterns and Anomalies
In any research initiative, recognizing patterns and anomalies is essential for conducting a thorough insight discrepancy analysis. This process involves systematically reviewing collected data to identify recurring themes that emerge across different interviews. By clustering similar responses, researchers can glean essential insights and track shifts in perspectives. Effective pattern recognition allows for a clearer understanding of the overall sentiment and context behind participant feedback.
Alongside human analysis, leveraging AI tools can significantly enhance anomaly detection. These advanced technologies can flag inconsistencies or unexpected insights that may require further investigation. By integrating software that analyzes qualitative data, researchers can pinpoint divergences more efficiently, ultimately resulting in a more comprehensive analysis. Thus, recognizing patterns and anomalies not only illuminates potential conflicts but also opens the door for deeper insights into participant opinions, ensuring that every voice contributes to the final narrative.
- Methods for pattern recognition in qualitative data
Recognizing patterns in qualitative data is essential for effective Insight Discrepancy Analysis. Start by immersing yourself in the collected interviews, allowing themes and codes to emerge organically. Techniques like thematic coding and sentiment analysis can help categorize insights, highlighting what participants agree on and where they diverge. This process aids in distilling complex data into clear, actionable findings.
Moreover, utilizing tools such as mind maps or analysis kits can streamline your analysis. These tools can quickly display themes and codes relevant to specific contexts, enabling researchers to spot discrepancies related to participant experiences and goals. By clustering insights into distinct categories, we can better understand patterns and deviations, ultimately clarifying the narrative that arises from the data. Such structured analysis not only saves time but enhances the overall integrity of research findings.
- Tools for analyzing anomalies: integrating AI and software tools
Analyzing anomalies in research interviews is crucial for identifying conflicting insights that can impact decision-making. The integration of AI and software tools plays a vital role in this analysis process, enhancing efficiency and accuracy. By employing AI algorithms, researchers can quickly process large volumes of qualitative data, identifying patterns and discrepancies that may otherwise go unnoticed. This capacity for rapid analysis allows teams to focus on interpreting results rather than getting bogged down in manual data processing.
AI-driven tools such as thematic analysis software and natural language processing platforms can streamline the Insight Discrepancy Analysis. These tools analyze transcripts, highlight inconsistencies, and consolidate findings, paving the way for more objective insights. Furthermore, the use of collaborative software ensures that team members can align on their observations, mitigating bias that often arises from individual interpretations. Adopting such integrated methodologies can significantly reduce the time required for reporting and enhance the overall quality of insights derived from research interviews.
Implementing Insight Discrepancy Analysis: Tools and Techniques
Implementing Insight Discrepancy Analysis involves utilizing various tools and techniques to identify conflicting insights within research interviews. The primary goal is to ensure that insights accurately reflect the perspectives gathered during interviews, helping to uncover underlying themes and patterns. A critical part of this process begins with selecting the right tools designed for qualitative analysis. Software such as Nvivo and Dedoose provides capabilities for coding and categorizing responses, making it easier to visualize discrepancies amongst interviewee insights.
In addition to software tools, employing strategies such as thematic analysis can enhance the effectiveness of Insight Discrepancy Analysis. By focusing on specific themes derived from interviews, researchers can better categorize insights, identifying where conflicts may arise. Techniques like sentiment analysis can further assist in clarifying discrepancies by revealing emotional undertones in participant responses. Ultimately, effective implementation of these techniques leads to clearer, more reliable insights that can significantly improve research outcomes.
Extract insights from interviews, calls, surveys and reviews for insights in minutes
Top Tools for Insight Discrepancy Analysis
In the realm of insight discrepancy analysis, utilizing the right tools is crucial for effectively identifying and evaluating conflicting insights from research interviews. Software like Nvivo and Dedoose can streamline the process by enabling users to organize data, recognize themes, and analyze sentiment. With these tools, researchers can group insights into specific categories, making it easier to spot discrepancies in narratives shared by interview participants.
Atlas.ti and MAXQDA are valuable additions as well, providing advanced coding options to differentiate between insights and contradictions effortlessly. Additionally, platforms like Transana allow for the transcription and analysis of interview recordings, facilitating a thorough examination of spoken content. By employing these tools, researchers can enhance their understanding of the nuances in qualitative data, leading to more reliable findings in their studies. The right combination of these software tools ultimately empowers researchers to uncover hidden insights and address conflicting narratives effectively.
- insight7
Analyzing conflicting insights across research interviews is essential for robust qualitative research. Insight Discrepancy Analysis helps researchers identify where opinions diverge and why. The goal is to extract valuable learnings from the differences, which can guide decision-making. Begin by synthesizing the various views you gathered, focusing on why discrepancies exist. This step not only highlights differing opinions but also may reveal deeper insights into the needs and experiences behind those views.
To effectively carry out this analysis, consider these critical approaches: First, categorize discrepancies based on themes or topics to streamline assessment. Second, employ analytical tools such as qualitative data software to visualize patterns and highlight trends in responses. Lastly, engage in reflective discussions with your team to ensure the analysis incorporates diverse perspectives. Through these methods, Insight Discrepancy Analysis can significantly enhance the validity and reliability of research findings, ultimately leading to improved strategies and decisions.
- Nvivo
Nvivo serves as a transformative tool in Insight Discrepancy Analysis, particularly when analyzing qualitative data from research interviews. This software enables researchers to systematically categorize and analyze large amounts of text, making it easier to identify conflicting insights across interviews. With features such as coding, queries, and visualizations, Nvivo allows for a deeper understanding of underlying themes and contradictions within the data.
One of its standout capabilities lies in its ability to help visualize relationships between different insights. For instance, a researcher can create models or maps that illustrate how various perspectives connect or conflict, which enhances the analysis process. By employing Nvivo in Insight Discrepancy Analysis, researchers can approach their data with greater precision and organizational clarity, ultimately fostering a more rigorous and reliable exploration of insights across interviews.
- Dedoose
Dedoose serves as a powerful analytical tool designed to streamline the qualitative research process. By enabling users to upload interview data and categorize themes efficiently, it simplifies the task of identifying insight discrepancies. This platform allows for a collaborative environment where researchers can sift through large volumes of information, highlighting patterns and contradictions across interviews. The ability to analyze data collectively within projects enhances the efficacy of Insight Discrepancy Analysis.
With features such as transcription services that support multiple languages and advanced data visualization, Dedoose facilitates deeper insights. Researchers can quickly generate reports and identify key themes essential for understanding conflicting insights. By promoting a structured approach to qualitative analysis, this tool empowers teams to focus on strategic outcomes, ultimately enriching their decision-making processes. Embracing such tools is essential for those engaged in rigorous research efforts, as they foster a more nuanced understanding of participants' perspectives.
- Atlas.ti
Atlas.ti plays a significant role in Insight Discrepancy Analysis by providing a robust framework for analyzing qualitative data. This software enables researchers to code, categorize, and interpret complex information gathered from interviews. By utilizing its features, users can efficiently navigate through diverse insights, ensuring that conflicting viewpoints are easily identifiable.
One of Atlas.ti's key functions is its ability to visualize relationships between themes and patterns within the data. This visual representation aids in pinpointing discrepancies and trends, allowing researchers to understand underlying nuances better. Furthermore, Atlas.ti supports collaborative analysis, making it easier for teams to share findings and refine their interpretations, especially when handling conflicting insights across various interviews. In this way, the application becomes a powerful tool in navigating the challenging landscape of qualitative research.
- MAXQDA
MAXQDA serves as a dynamic tool in the realm of Insight Discrepancy Analysis, particularly within qualitative research. It empowers researchers to systematically analyze interview data, helping to reveal conflicting insights between participants. By organizing and coding data effectively, users can pinpoint discrepancies, making it easier to identify patterns and themes that may not be immediately apparent. This efficiency is crucial for during the intricate process of analyzing multiple interviews, as conflicting opinions can surface and complicate overall findings.
Maximizing the benefits of MAXQDA requires a clear understanding of how to utilize its features. First, coding interviews allows for the categorization of responses, streamlining comparison between varied perspectives. Next, its visualization tools provide insightful representations of data relationships, highlighting areas of conflict. Finally, the platform's export capabilities enable comprehensive reporting, ensuring that key discrepancies can be effectively communicated to stakeholders. By leveraging MAXQDA in this way, researchers can enhance their Insight Discrepancy Analysis for more reliable and actionable conclusions.
- Transana
Understanding the role of a powerful analysis tool can significantly enhance your approach to Insight Discrepancy Analysis. This tool allows for the seamless transcription of interviews, capturing dialogue efficiently and effectively. It enables researchers to upload audio or video data without needing external tools, streamlining the process from the very start. Once your data is transcribed, you can delve into the nuances of the information gathered, aiding in identifying conflicting insights across interviews.
After transcription, the focus shifts to analysis. You can assess individual transcripts or analyze them within a project context to recognize themes, key quotes, and relevant data. This functionality supports a more comprehensive perspective, allowing researchers to pinpoint discrepancies in insights among various interviewees. Such capabilities ultimately lead to richer analyses, revealing deeper understandings and informing decision-making processes in future research endeavors.
Qualitative vs. Quantitative Discrepancy Detection
Qualitative vs. Quantitative Discrepancy Detection highlights the two core approaches in analyzing conflicting insights from research interviews. Qualitative methods focus on subjective interpretations, such as emotions and experiences, and are vital for understanding the why behind user behaviors. On the other hand, quantitative techniques rely on numerical data and statistical analysis to uncover trends and patterns, offering a broader overview. Both approaches are crucial for comprehensive insight discrepancy analysis.
To enhance the effectiveness of your research, combining both qualitative and quantitative methods can provide a richer perspective. For instance, qualitative feedback can contextualize quantitative results, making them more meaningful. Conversely, statistical analysis can validate qualitative themes, ensuring they are not mere anomalies. By integrating these methodologies, you can create a more thorough and nuanced analysis that helps identify and resolve any discrepancies in insights from interviews.
- Differences between qualitative and quantitative analysis techniques
Qualitative and quantitative analysis techniques serve distinct purposes in research, particularly when conducting Insight Discrepancy Analysis. Qualitative techniques focus on understanding the underlying motivations and emotional responses of participants. These techniques often involve conducting detailed interviews, where researchers explore sentiments and themes that emerge from the data. This approach is particularly useful for capturing rich, nuanced insights but can be subject to bias and interpretation variability.
On the other hand, quantitative techniques are centered around numerical data and statistical analysis. They provide a broader perspective by quantifying responses and establishing measurable patterns. By harnessing surveys and standardized questions, researchers can objectively evaluate trends, leading to conclusions that might be statistically significant. Both methods, when effectively combined, enhance the robustness of Insight Discrepancy Analysis, allowing researchers to triangulate findings and minimize inconsistencies across diverse research interviews.
- How to effectively combine both methods for robust discrepancy detection
Integrating qualitative and quantitative methods can significantly enhance your capability to detect insight discrepancies, creating a more robust analysis. Begin by collecting diverse perspectives through interviews and surveys, ensuring you capture varied viewpoints. This multifaceted approach allows for a deeper understanding of the context surrounding the insights, making it easier to identify potential conflicts arising from different interpretations.
Next, use pattern recognition techniques combined with data analysis tools to pinpoint both commonalities and inconsistencies among the responses. Tools utilizing AI can assist in analyzing large datasets efficiently, revealing anomalies that may otherwise go unnoticed. By effectively combining these methods, you foster a comprehensive understanding of the insights, ensuring that conflicting viewpoints are not only acknowledged but also analyzed in a manner that leads to actionable outcomes. Applying Insight Discrepancy Analysis in this structured way can transform the research process, yielding clearer, more reliable conclusions.
Conclusion: Enhancing Research Validity Through Insight Discrepancy Analysis
In conclusion, enhancing research validity through Insight Discrepancy Analysis enables teams to uncover conflicting insights effectively. By systematically evaluating diverse perspectives, researchers can identify inconsistencies that might otherwise go unnoticed. This method not only fosters a more comprehensive understanding of the data but also enhances the reliability of the findings.
The process of Insight Discrepancy Analysis is vital for ensuring that research outcomes reflect the true sentiments of interview subjects. As teams embrace this analytical approach, they can better streamline their reporting and reduce bias. Ultimately, adopting such strategies not only improves the quality of insights but also builds greater trust in the research findings.