AI-Driven Interview Analysis revolutionizes how researchers extract insights from qualitative data. Traditionally, analyzing interviews is a lengthy and subjective process that demands immense time and effort. As professionals face mounting pressure for quicker and more reliable insights, AI emerges as an indispensable tool that streamlines analysis and reduces inconsistencies across teams.
This innovative approach harnesses artificial intelligence to decode patterns and themes from interview transcripts with remarkable efficiency. By automating coding and analysis, researchers can focus on interpreting findings rather than becoming bogged down in manual tasks. Ultimately, AI-Driven Interview Analysis not only enhances the quality of insights but also empowers teams to deliver timely reports that drive informed decision-making.
Analyze qualitative data. At Scale.
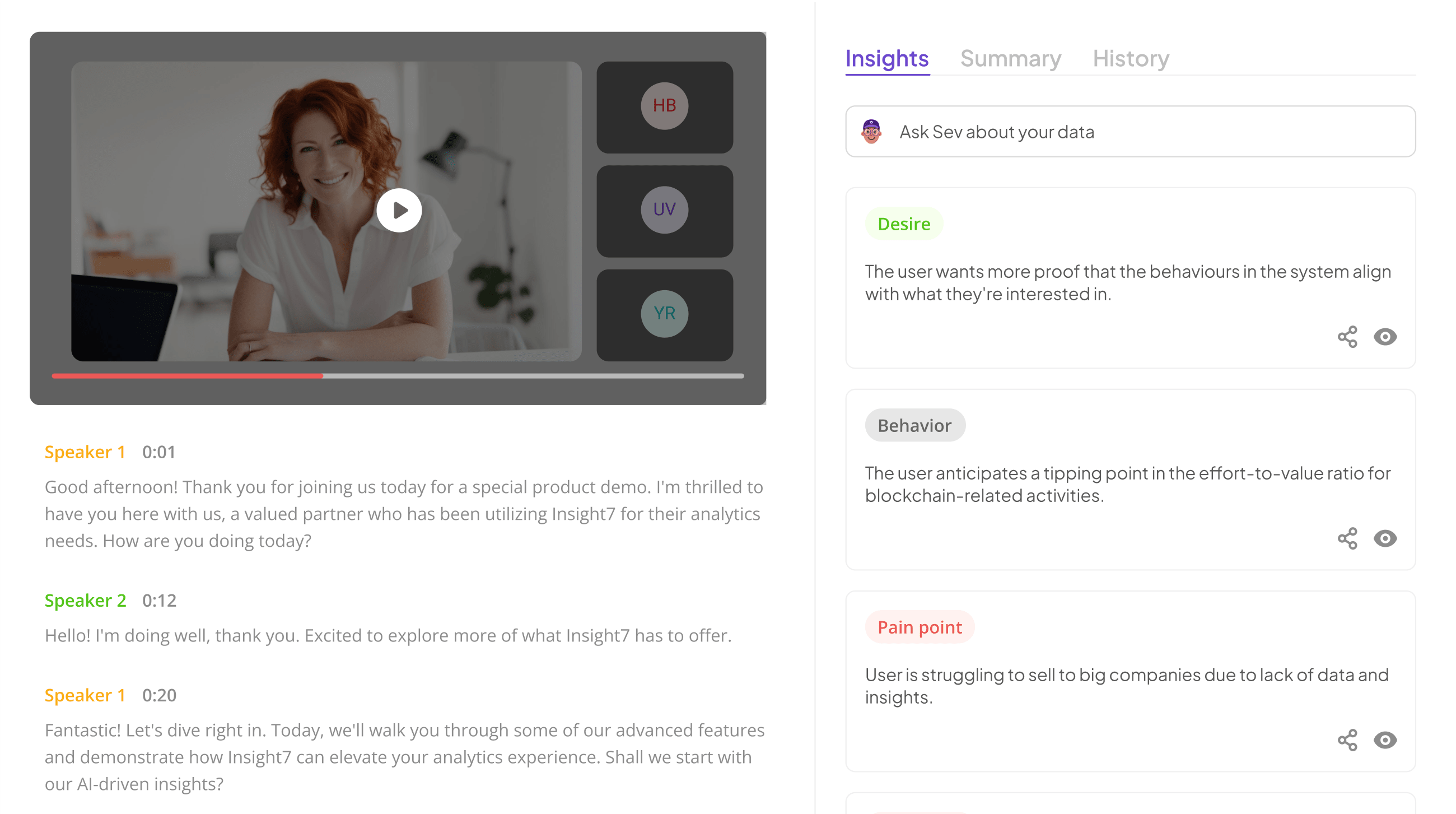
Setting the Stage: Understanding AI in Interview Analysis
The emergence of AI-driven interview analysis marks a transformative era in research methodologies. This approach blends advanced algorithms with traditional interview techniques, allowing researchers to gain deeper insights from qualitative data. As the digital landscape evolves, leveraging AI technologies in interview analysis becomes increasingly essential, enabling teams to navigate vast information more effectively.
AI enhances the traditional methods by automating transcription and categorization, significantly reducing analysis time. By converting spoken words into structured data, AI tools enable researchers to pinpoint themes and sentiment more efficiently. Consequently, this process not only streamlines the workload but also minimizes human errors that can arise from manual handling. Furthermore, AI-driven analysis can uncover patterns and correlations that may otherwise go unnoticed, significantly enriching the research findings. As we delve deeper into the practical applications of these technologies, it's crucial to understand how to implement AI-empowered tools for effective coding in interviews, setting the stage for meaningful insights.
The Role of AI-Driven Interview Analysis in Modern Research
AI-Driven Interview Analysis revolutionizes the way researchers engage with qualitative data, improving both efficiency and depth of insight. By utilizing algorithms to analyze transcripts, researchers can discover themes and patterns that may be overlooked during manual evaluations. This technology streamlines the analysis process, enabling teams to focus more on interpretation rather than data gathering, ultimately expediting report generation.
Moreover, AI-Driven Interview Analysis enhances consistency across teams that may have previously exhibited discrepancies. This improved standardization leads to more reliable insights while minimizing biases inherent in human analysis. As organizations demand quicker turnaround times and more precise findings, integrating AI into interview coding offers a solution that not only meets these needs but also enriches the overall research quality. Embracing this innovative tool ensures researchers can effectively harness the full potential of their qualitative data.
How AI Enhances Traditional Interview Methods
AI-Driven Interview Analysis transforms traditional methods by automating and refining the process of gathering insights. Interviewers often face the challenge of bias and inconsistency, which can hinder objective evaluations. By employing AI, researchers can standardize data analysis, ensuring more reliable results. Advanced algorithms can analyze speech patterns, tone, and sentiment, all while processing large volumes of data rapidly. This shifts the focus from manual efforts to strategic insight generation, enhancing overall efficiency.
In addition, AI simplifies the coding of qualitative data, a task that might otherwise consume valuable time and resources. AI-driven tools can identify themes and patterns, enabling research teams to uncover deep insights faster than with conventional methods. This not only accelerates the reporting process but also enriches the final analysis. Consequently, integrating AI into traditional interviews maximizes research productivity and enhances the quality of insights derived, moving the field into a new era of data exploration.
Benefits of Using AI for Deeper Insights
AI-Driven Interview Analysis enables researchers to delve deeper into qualitative data, uncovering insights that often remain hidden through traditional methods. By processing vast amounts of interview data, AI can identify patterns and themes effectively, allowing for comprehensive analysis. One significant advantage of employing AI for deeper insights is its ability to enhance accuracy. By minimizing human error, the technology ensures that critical nuances in conversations are preserved.
Another benefit is efficiency; AI can rapidly process large datasets, significantly reducing the time researchers spend on manual coding and analysis. Moreover, AI tools can extract quotes and themes relevant to specific topics, providing the ability to focus on particular areas of interest. This not only streamlines reporting but also enables data-driven decisions. Overall, integrating AI into interview analysis helps achieve more informed conclusions and actionable recommendations.
Extract insights from interviews, calls, surveys and reviews for insights in minutes
Implementing AI-Powered Tools for Interview Analysis
In the journey of AI-Driven Interview Analysis, implementing AI-powered tools can revolutionize the way interviews are processed and insights are gleaned. By utilizing advanced technologies, researchers can automate labor-intensive tasks such as transcription, coding, and thematic analysis. This significantly reduces time and resource constraints that often hinder deep research insights. These tools enhance accuracy and consistency, addressing common biases in manual coding, which can vary from researcher to researcher.
A few key tools can enhance this analytical approach: first, selecting the right AI tool tailored to your research objectives is crucial. Next, preparing your interview data meticulously ensures that the AI can process it effectively. Once the analysis is conducted, it’s vital to interpret the AI-generated insights meaningfully. By engaging in this systematic implementation of AI tools, researchers can unlock richer, actionable insights that propel their understanding of complex topics and drive informed decision-making.
Key Tools for AI-Driven Interview Analysis
AI-Driven Interview Analysis empowers researchers to transform qualitative data into meaningful insights efficiently. To achieve this, several key tools play a crucial role in streamlining the analysis process. These tools not only enhance the quality of data interpretation but also reduce the time spent on manual tasks, allowing teams to focus on critical thinking and strategy.
Firstly, platforms like Transcribe convert speech to text accurately, making it easier to sift through spoken content. Meanwhile, Otter.ai fosters collaborative review processes, ensuring that multiple researchers can contribute insights seamlessly. Lastly, tools such as Sonix offer multilingual support, which is essential for global research efforts. By leveraging these technologies, teams can mitigate bias and maintain consistency in their findings, ultimately leading to a more cohesive understanding of the data. Embracing these tools in AI-Driven Interview Analysis can significantly elevate research outcomes and client satisfaction.
insight7: A Leading Force in AI Interview Analysis
AI-Driven Interview Analysis has emerged as a game-changer in the realm of qualitative research. By automating the analytical process, researchers can overcome the challenges posed by traditional manual methods. In an environment where time is of the essence, AI ensures rapid data processing, allowing teams to focus on deriving actionable insights from their findings.
Moreover, AI minimizes bias and inconsistency, which are common pitfalls in interviews conducted by multiple researchers. This technology standardizes the way insights are extracted, enabling a coherent narrative across diverse teams. As organizations increasingly strive for faster turnaround times, AI-driven solutions become indispensable in streamlining interview analysis. By harnessing this technology, researchers can transform ungainly data into clear, concise, and reliable insights, boosting overall productivity and efficiency in the research process.
Transcribe: Transforming Speech to Text with Precision
Transcribing audio and verbal content into accurate text format is a crucial step in AI-Driven Interview Analysis. This transformative process enables researchers to easily capture and organize insights extracted from interviews. With the right transcription tool, you can implement a seamless workflow that allows for immediate access to data, ready for deeper exploration.
AI-powered transcription services not only convert speech to text with high precision but also facilitate further analysis. After transcription, researchers can dive into qualitative data, identifying themes, quotes, and patterns that emerge from the conversations. Efficiently managing this information using AI enhances data accuracy and reduces human error, making your research process more effective. By harnessing technology, you pave the way for meaningful insights that drive informed decisions and foster innovation in research methodologies.
Otter.ai: Streamlining Collaborative Review Processes
The integration of AI technology significantly simplifies the collaborative review processes in interview analysis. By offering real-time transcription and summarization, AI tools enable teams to efficiently compile and share insights. This immediacy fosters an environment of continuous collaboration, allowing team members to focus on critical thinking rather than data collection.
Moreover, these AI-driven systems enhance accuracy by minimizing human error during transcription. They also provide visual presentations of data, making it easier to identify themes and patterns. As a result, researchers can swiftly pinpoint key findings and facilitate more informed decision-making. Overall, streamlining collaborative review processes not only improves productivity but also enriches the quality of insights derived from interviews, ultimately benefiting deep research efforts.
Sonix: Empowering Multi-Language Support for Global Research
In today's globalized research landscape, the ability to conduct interviews in multiple languages is essential for capturing diverse perspectives. AI-driven interview analysis plays a pivotal role in bridging language barriers, allowing researchers to collect and analyze insights from various linguistic backgrounds seamlessly. With advanced transcription services, interview data is not only converted into text but can also be processed for deeper analysis, leading to more comprehensive understandings of participants' viewpoints.
Utilizing multi-language support empowers researchers to engage a broader audience without the constraints of language limitations. This capability ensures that cultural nuances and specific terminologies are preserved, ultimately enriching the research process. By integrating AI technologies, research teams can extract themes, quotes, and actionable insights from transcriptions, facilitating well-rounded analysis and reliable results. In a world where understanding diverse narratives is crucial, these tools become invaluable assets in the pursuit of knowledge.
Step-by-Step Guide to Coding Interviews with AI
To successfully code interviews with AI, it is essential to follow a structured approach. Step one involves selecting the right AI tool tailored to your research needs. Various options exist, ranging from transcription services to comprehensive analysis platforms. Carefully assess each tool's capabilities to find the most suitable solution for your project.
Next, you'll want to prepare your interview data for AI processing. Organizing your raw data—such as transcriptions and notes—ensures that the AI can operate effectively. Once your data is ready, conduct the AI analysis, leveraging its advanced techniques to unearth significant patterns. Finally, interpreting the results generated by the AI allows for deeper insights, shaping your research conclusions and strategies while enhancing your understanding of respondents’ perspectives. Through this detailed method, AI-driven interview analysis transforms the research landscape, boosting efficiency and accuracy.
Step 1: Selecting the Right AI Tool for Your Research Needs
When embarking on AI-driven interview analysis, selecting the right tool is crucial for maximizing research effectiveness. The landscape of AI tools offers numerous options, each designed to address different aspects of interview data processing. Start by assessing your specific needs: are you prioritizing transcription accuracy, theme generation, or insightful reporting? Choose a tool that aligns with your project goals and offers features that enhance your research workflow.
Next, consider how user-friendly the interface is and how well it integrates with your existing research methodology. An ideal tool should facilitate the coding process, allowing you to filter insights by theme and speaker effectively. Furthermore, ensure that the selected AI tool supports various output formats, making it easy to compile reports. By carefully evaluating these factors, you can streamline the AI-driven interview analysis process, ultimately leading to deeper insights and more impactful conclusions.
Step 2: Preparing Your Interview Data for AI Processing
To successfully embark on AI-Driven Interview Analysis, preparing your interview data is crucial. Begin by organizing your raw data for optimal accessibility. This means cleaning it up to eliminate any inaccuracies and inconsistencies that could skew the results. Ensure that your interviews are correctly transcribed, as AI tools rely heavily on precise text to generate meaningful insights.
Next, categorize your interviews based on themes or topics relevant to your research objectives. By doing this, you create a framework that the AI can easily navigate. This helps in identifying key patterns and insights more effectively during the analysis phase. It’s also important to anonymize any sensitive information to maintain data privacy. Following these steps will set a solid foundation for the upcoming AI analysis, positioning your research toward greater depth and clarity in insights.
Step 3: Conducting the AI Analysis
Conducting the AI analysis is a transformative step in your research process. Begin by uploading your transcribed interviews into your chosen AI tool. Here, advanced algorithms will sift through the data, identifying key themes and patterns that may not be immediately evident. This AI-driven interview analysis allows for comprehensive examination, enabling you to quickly pinpoint vital insights that would traditionally require extensive manual review.
Once the initial analysis is complete, it's crucial to validate the AI's findings. Manually review the outputs, as this ensures accuracy and highlights any missed nuances. Collaborate with your team to interpret the results collectively. This collaborative effort amplifies the AI's effectiveness, bringing diverse perspectives into the analysis. By integrating AI tools, you streamline the coding process, reduce bias, and deliver insights in a fraction of the time. Embracing this step paves the way for deeper research insights, effectively addressing client needs for faster and more reliable outcomes.
Step 4: Interpreting AI-Generated Insights
Interpreting AI-generated insights is a crucial step in the AI-driven interview analysis process. When AI analyzes interview data, it distills complex conversations into coherent insights you can use. Understanding these insights involves recognizing patterns, themes, and key quotes that resonate with your research objectives. To accurately interpret the outputs, establish a clear framework that outlines what you aim to achieve with your findings.
When diving into AI-generated insights, consider these key areas: identify thematic connections, ensure clarity in reporting, and actively compare insights against your research objectives. By emphasizing thematic connections, you can synthesize insights that directly address specific questions. Foster clarity by summarizing major findings that highlight the nuances within the data. Lastly, regular comparison with your predefined objectives ensures that your insights remain relevant and actionable. This step not only enhances your analysis but also drives impactful decision-making based on AI-derived information.
Conclusion: Unleashing the Potential of AI-Driven Interview Analysis for Comprehensive Research
AI-Driven Interview Analysis holds incredible promise for transforming research methodologies. By automating the coding and analysis of interviews, researchers can uncover patterns and insights more efficiently than ever before. This allows for a deeper understanding of qualitative data, enhancing the richness of research findings while minimizing the time spent on tedious manual tasks.
Furthermore, the integration of AI tools minimizes bias and promotes consistency across research teams. As a result, organizations can generate timely reports and actionable insights, empowering them to make informed decisions quickly. Embracing AI-Driven Interview Analysis not only optimizes workflow but also expands the potential for groundbreaking research insights that can drive success across various sectors.