The Interview Data Grid serves as a vital tool for analyzing qualitative information drawn from interviews. By organizing data efficiently, it enables a comparative analysis that highlights key themes and insights. This grid structure not only enhances clarity but also simplifies the identification of patterns among various interview responses.
Creating an Interview Data Grid involves systematically placing respondents' answers against specific questions. To effectively navigate the wealth of data, analysts can tag responses with appropriate codes, allowing for a more refined visual understanding of the findings. This process ultimately transforms raw qualitative data into actionable insights that inform decision-making.
Analyze & Evaluate Calls. At Scale.
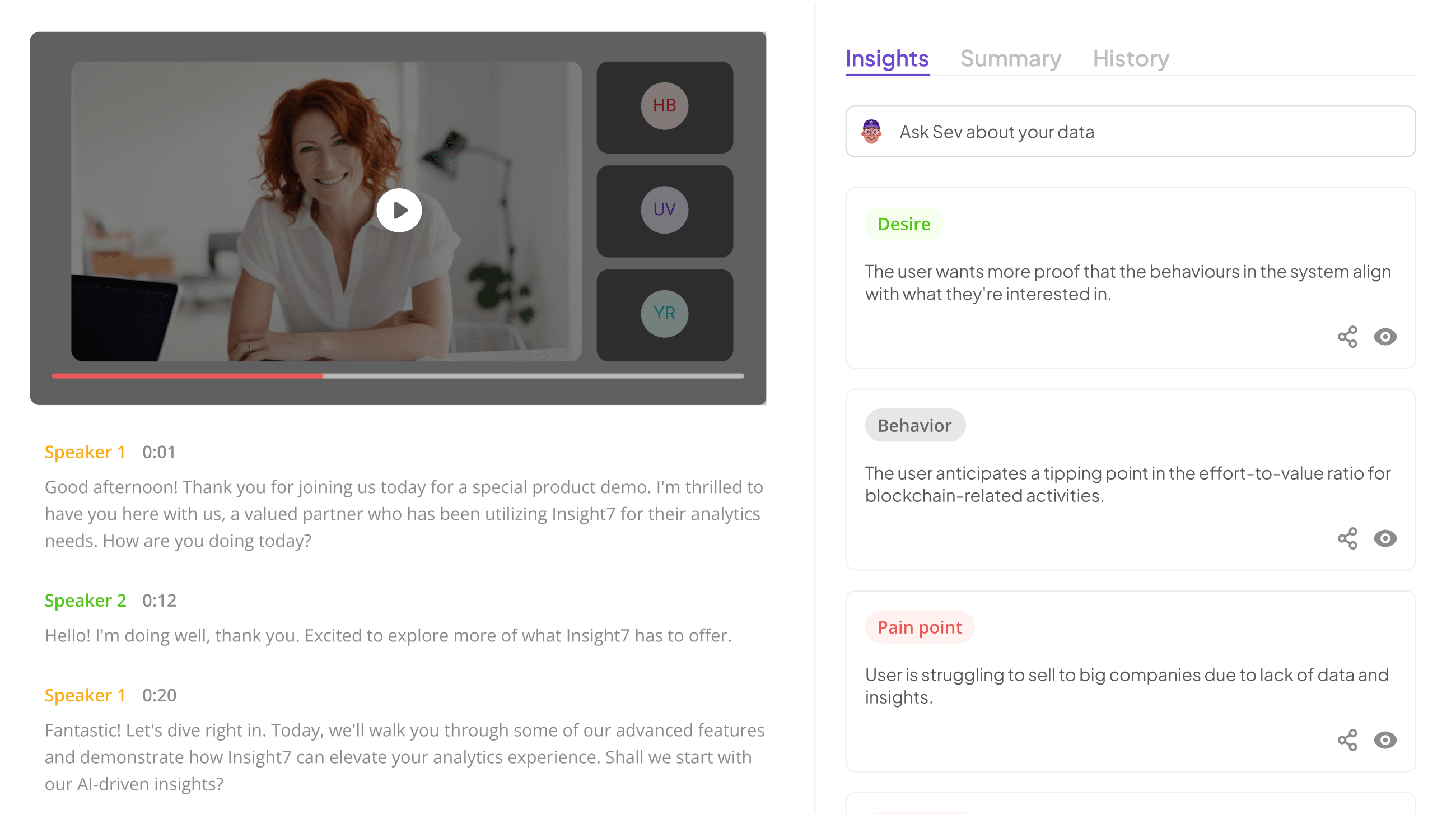
Understanding the Interview Data Grid Structure
Understanding the Interview Data Grid involves grasping its underlying framework and elements. An Interview Data Grid is designed to display responses from multiple interviews in a structured format. By arranging questions along the top and interviewee responses on the left, researchers can analyze patterns and insights efficiently. This grid allows for instantaneous synthesis of vast amounts of qualitative data, making it easier to identify key themes that may emerge across different interviews.
The core elements of the Interview Data Grid include various data points, such as interviewee demographics, responses, and coded insights. When setting up the grid, it is crucial to ensure that each cell captures specific responses related to the corresponding questions. Additionally, coding answers can help in visualizing trends and comparisons among different responses. This systematic approach not only fosters clarity but also deepens understanding, enabling a robust comparative analysis.
The Core Elements of an Interview Data Grid
An Interview Data Grid consists of essential elements that help organize and visualize qualitative data effectively. Such a grid typically features interview subjects listed vertically, alongside key questions or themes horizontally. This alignment allows for a clear comparison of responses across multiple interviews, facilitating easy identification of trends and patterns. One of the gridโs standout functionalities is its ability to summarize data, highlighting core insights derived from responses.
Another critical aspect of an Interview Data Grid is its coding layer, which enables the tagging of specific answers with relevant codes. This coding process can be performed manually or automatically, depending on the tools utilized. As a result, users can visualize breakdowns of responses while also accessing specific verbatim quotes for context. By employing these core elements within an Interview Data Grid, researchers can comprehensively analyze qualitative data, drawing meaningful conclusions from diverse perspectives.
Organizing Qualitative Data for Grid Creation
Organizing qualitative data is crucial for creating an effective Interview Data Grid. Start by gathering all transcripts and summarizing key insights. A systematic approach ensures that significant themes are highlighted, allowing easy comparison later. Use a coding system to categorize responses based on common topics or sentiments expressed during the interviews. This process can simplify your analysis.
Next, consider developing a visual structure for your grid. Clearly defined columns and rows will help in organizing data logically. Each row can represent an interviewee while columns represent different questions or themes. This structured approach aids in identifying patterns across multiple interviews and provides a streamlined way to analyze responses. As you synthesize and arrange the qualitative data into this grid, ensure that it remains accessible and easy to interpret. This will ultimately enhance the credibility and usefulness of your comparative analysis.
Steps to Build Comparative Analysis Grids from Interview Data
Building a Comparative Analysis Grid from interview data requires a structured approach for effective insights. Begin by gathering and preparing your interview data, ensuring that all responses are well-documented and easily accessible. This foundational step is crucial to form the basis of your Interview Data Grid.
Next, identify common themes and patterns within the collected responses. This involves coding the data, which can be done manually or through software. Once you have categorized the data, create a framework for the grid itself. Arrange themes across the top and responses down the side, allowing for a clear comparison between interviewees. Finally, visualize and interpret the data within this grid to unveil insights that might not be immediately evident from the raw data. This rigorous process transforms qualitative insights into structured knowledge, enabling clear, actionable conclusions.
Extract insights from interviews, calls, surveys and reviews for insights in minutes
Step 1: Data Collection and Preparation for Interview Data Grid
To initiate the process of building an Interview Data Grid, effective data collection and preparation are paramount. This step begins with gathering your interview transcripts and raw data from various sources, ensuring consistency in format. You can utilize transcription services that offer high accuracy, which streamlines the inclusion of quotes and themes into the grid. Collecting comprehensive data allows for a more nuanced analysis, highlighting varied perspectives and insights.
Next, you should organize this data methodically. Create a framework that facilitates easy reference to specific themes or topics discussed in the interviews. This structure will be vital when it comes time to synthesize findings into the Interview Data Grid. Effective preparation not only enhances the clarity of your analysis but also saves time during the comparison phase. By laying a solid foundation, you will ensure that insights derived from the Interview Data Grid are actionable and relevant to your objectives.
Step 2: Identifying Themes and Patterns
Identifying themes and patterns within interview data is a crucial step in constructing an effective Interview Data Grid. This process involves carefully analyzing the data to extract recurring ideas or sentiments. By recognizing these patterns, you will create a framework that highlights the underlying narratives shared by your participants. This clustering not only aids in conceptual clarity but also allows for a more structured comparison between different interview responses.
To streamline this task, consider the following actions:
- Code the Data: Start by labeling sections of the interview data with relevant themes or tags that reflect the speakers' main ideas.
- Define Goals: Clarify what you aim to achieve with the analysis. Are you focusing on improving user experience or addressing specific challenges?
- Cluster Insights: Group the themes to visualize connections among various insights, facilitating a clearer analytical overview.
Each identified theme should help paint a comprehensive picture of the shared experiences among participants, which will ultimately enhance the quality of your comparative analysis grid.
Step 3: Developing the Comparative Analysis Grid Framework
Creating an effective Comparative Analysis Grid Framework begins with understanding how to structure the Interview Data Grid. This grid will serve as a vital tool for organizing insights and identifying patterns from multiple interviews. By placing interviewees along one axis and key questions or themes along the other, you create a clear visual representation of responses. Each cell in the grid captures specific insights, facilitating easier comparisons across different interview data.
๐ฌ Ask About This Article
Have questions? Get instant answers about this article.
To develop this framework, start by defining the categories you want to analyze. Establish key questions to guide your analysis, which will form the headers of your grid. Then, populate the grid with collected data, either manually tagging responses or using automated coding tools. This structured approach not only highlights similarities and differences but also fosters a deeper understanding of the underlying messages. Ultimately, this enables richer insights and informs decision-making based on comprehensive qualitative data.
Top Tools for Creating Interview Data Grids
Creating an effective Interview Data Grid requires the right tools to streamline the process and enhance analysis efficiency. Various software solutions can assist in organizing and synthesizing qualitative data, transforming extensive interview transcripts into easily digestible formats. Notable options include NVivo and ATLAS.ti, which excel in coding and pattern identification, making data analysis more intuitive.
Another robust tool, Dedoose, is perfect for researchers focused on mixed-methods approaches, allowing for seamless integration of both qualitative and quantitative data. MaxQDA stands out for its user-friendly interface and powerful visualization capabilities, enabling users to see trends at a glance. Each tool has unique features that address specific analytical needs, ensuring that you can create a comprehensive Interview Data Grid tailored to your research objectives. By selecting the right tool, you pave the way for insightful comparative analyses that can substantially inform decision-making processes.
insight7
Understanding the insights gained from your Interview Data Grid can transform qualitative feedback into actionable strategies. The first step lies in properly structuring a grid that reflects the themes extracted from interviews. This means not only capturing the raw data but also identifying the key insights that resonate with your target audience. As you explore this grid, itโs essential to highlight the commonalities and discrepancies among respondents, which can reveal underlying trends.
To make the most of your Interview Data Grid, consider focusing on a few foundational aspects. First, ensure that data collection is thorough and systematic, preparing responses for clarity. Second, analyze the data by grouping similar responses, which aids in recognizing patterns. Finally, visually represent this information within the Grid framework to facilitate comparison. By embracing these principles, you'll harness the true potential of your interview data, guiding strategic decisions based on solid insights.
NVivo
NVivo serves as an essential tool for effectively creating an Interview Data Grid, enhancing the comparative analysis of qualitative data. With its user-friendly interface and powerful features, it simplifies data organization and visualization. Users can easily input interview transcripts, categorize data, and identify key themes, significantly streamlining the grid-building process. This capability allows for a more nuanced analysis of interview data, revealing insights that may remain hidden when handled manually.
Moreover, utilizing NVivo empowers researchers to craft detailed visual representations of their findings. This ability to visualize connections among themes makes it easier to comprehend complex relationships in the data. Ultimately, incorporating NVivo into your research workflow not only facilitates efficient data management but also enriches the overall quality of your Comparative Analysis Grid. As a result, it serves as a valuable partner in your journey toward meaningful insights derived from qualitative interviews.
Dedoose
Dedoose serves as a powerful tool for analyzing qualitative research, especially when building an Interview Data Grid. By streamlining the process, Dedoose allows researchers to maximize their insights from interviews collected across various formats. Users can easily upload audio and text files for transcription, which enhances accessibility and prepares the data for analysis. This platform offers robust features such as collaborative tools and customizable grids, making it viable for teams to work collectively on complex data sets.
In constructing your Interview Data Grid, Dedoose enables efficient theming and categorization of insights. The intuitive interface allows for visual representation of data, helping users identify relationships and patterns among responses. By integrating quotes and themes into a grid format, researchers can draw clearer conclusions that influence business strategies. Consequently, Dedoose not only simplifies data management but also amplifies the value derived from interview findings.
MaxQDA
MaxQDA is a valuable tool designed to help researchers analyze qualitative data efficiently. This software aids in organizing, coding, and interpreting large volumes of interview data, facilitating the development of an Interview Data Grid. Users can easily import transcripts, allowing for a streamlined process of identifying key themes, patterns, and insights that emerge from the data.
To utilize MaxQDA effectively, start by importing your transcripts and labeling relevant segments. Next, apply coding techniques to categorize responses based on recurring themes. This structured approach not only simplifies the analysis but also highlights connections between different interviews. By transforming qualitative insights into a coherent Interview Data Grid, MaxQDA empowers researchers to make data-driven decisions that are systematic and insightful. Ultimately, it serves as an essential support tool for comparative analysis, turning raw data into actionable findings.
ATLAS.ti
ATLAS.ti is an essential tool for researchers aiming to construct effective comparative analysis grids from interview data. This software excels at organizing, managing, and analyzing qualitative research findings, making it pivotal in qualitative data analysis. By allowing researchers to tag and link codes to specific segments of text, ATLAS.ti helps in developing a well-structured Interview Data Grid. The visualization capabilities of the software enable users to easily interpret complex patterns and themes, providing a clear framework for comparison.
Using ATLAS.ti, researchers can streamline the data analysis process. The software's interactive interface facilitates collaboration, as teams can share notes, highlight insights, and draw connections between different data points. Through this collaborative effort, creating an Interview Data Grid becomes not only more efficient but also enhances the depth of analysis. Ultimately, ATLAS.ti empowers researchers to derive actionable insights from interviews, ensuring that their comparative analysis grids truly reflect the nuances of the collected data.
Conclusion: Utilizing Interview Data Grid for Effective Comparative Analysis
Utilizing an Interview Data Grid streamlines the process of comparative analysis across diverse interview data. By organizing insights systematically, analysts can identify patterns, themes, and trends effectively. This structured approach helps reveal critical comparisons that may be overlooked when data is scattered or analyzed individually.
Moreover, the Interview Data Grid serves not just as an organizational tool but also facilitates collaboration among team members. By presenting data visually and contextually, this grid enhances understanding, enabling more focused discussions and decision-making. Overall, incorporating an Interview Data Grid into your workflow maximizes the value derived from qualitative data.
๐ฌ Ask About This Article
Have questions? Get instant answers about this article.