How to Build a Call Tagging Framework for AI Tools
-
Hello Insight
- 10 min read
AI Call Tagging is revolutionizing the way businesses analyze customer interactions. As organizations strive for continuous improvement, understanding the nuances of call conversations becomes essential. By employing AI-driven tagging frameworks, companies can extract valuable insights from customer calls, shaping their strategies and enhancing overall service quality.
This section will provide an overview of the AI Call Tagging Framework, emphasizing its importance in optimizing business communication. We will explore how these frameworks can categorize conversations into actionable data, allowing businesses to respond effectively to customer inquiries. Engaging with the framework not only streamlines operations but also fosters a culture of innovation and responsiveness.
Analyze & Evaluate Calls. At Scale.
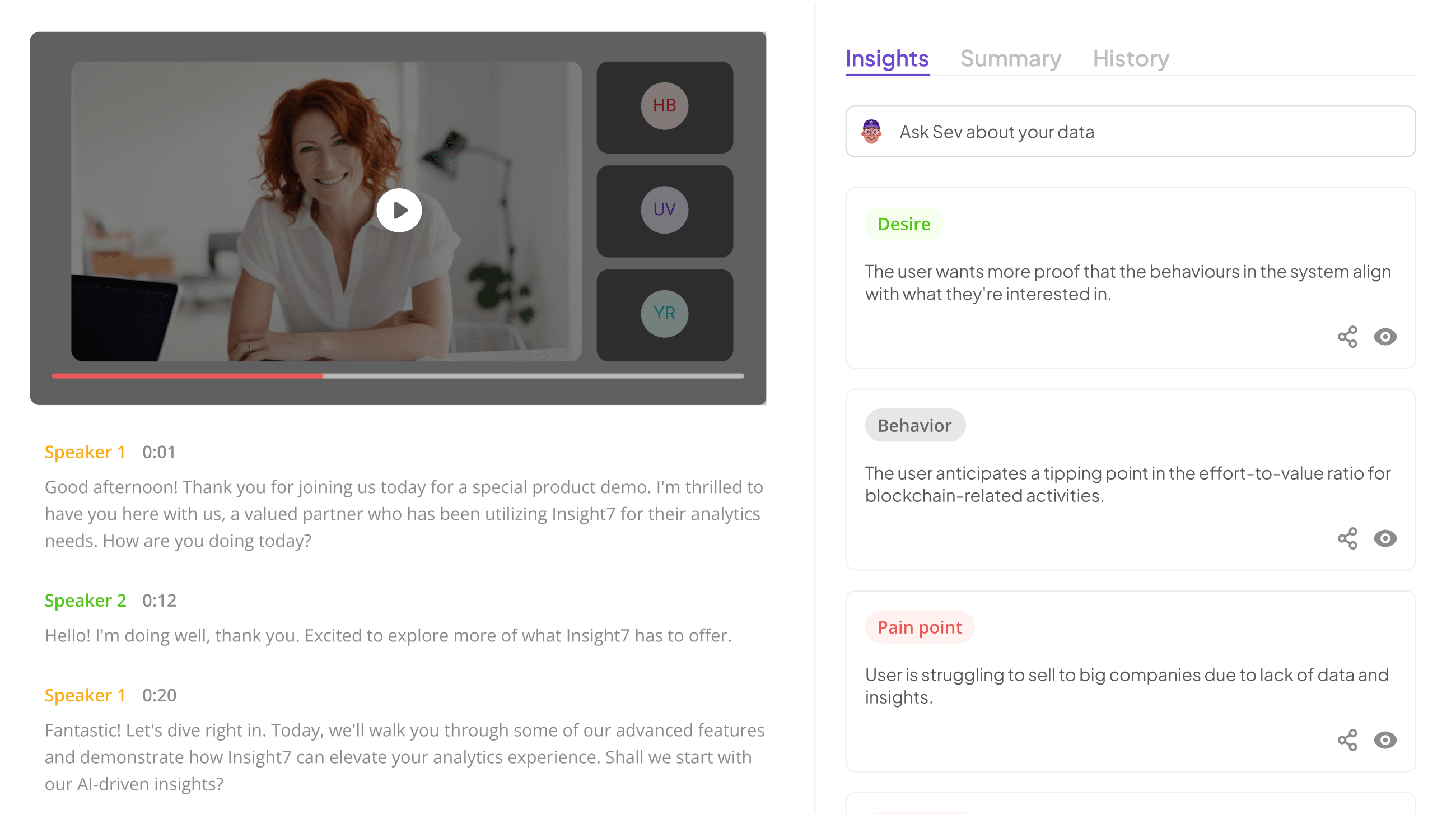
Understanding AI Call Tagging
AI Call Tagging serves as a powerful tool that enhances how organizations analyze conversations. By automating the process of categorizing and labeling calls, AI technologies help extract valuable insights from those interactions. This technique relies on sophisticated algorithms that recognize specific keywords and phrases, enabling a more streamlined analysis of customer interactions.
Understanding how AI Call Tagging works is essential for businesses aiming to improve communication. When calls are tagged accurately, organizations can identify trends and patterns in customer behavior. This information not only supports agents in refining their performance but also contributes to improved customer satisfaction. As you build your AI Call Tagging framework, consider the vital role this technology plays in gaining actionable insights and enhancing overall operational efficacy. Ultimately, harnessing AI Call Tagging can lead to more effective decision-making and stronger customer relationships.
What is AI Call Tagging?
AI Call Tagging is a process that utilizes artificial intelligence to automatically categorize and label calls based on predefined criteria. This technique allows organizations to extract insights from call data efficiently, enhancing their understanding of customer interactions and employee performance. By employing natural language processing algorithms, AI systems can analyze conversations and identify key themes, sentiment, and specific topics discussed.
The primary goal of AI Call Tagging is to streamline the analysis of call recordings, making it easier to evaluate agent performance and compliance. This method not only saves time compared to manual tagging but also increases accuracy, providing businesses with actionable insights. When implemented effectively, AI Call Tagging offers deeper visibility into communication patterns, ultimately supporting better decision-making and improved customer experiences. By integrating this innovative approach into their operations, companies can significantly elevate their customer service quality and operational efficiency.
Importance of AI Call Tagging in Business Communication
AI Call Tagging plays a vital role in enhancing business communication by systematically organizing call data. This process enables businesses to analyze interactions, revealing patterns in customer behavior and agent effectiveness. By tagging calls with specific identifiers, companies can streamline workflows, improve service delivery, and ensure tailored responses to customer needs.
Implementing AI Call Tagging facilitates better understanding of customer sentiment and key concerns during conversations. It enables organizations to gauge not only the topics discussed but also the tone and demeanor of interactions, helping to shape future strategies. Furthermore, effective tagging identifies outcomes such as successful appointments or missed opportunities, leading to data-driven decision-making. Understanding these elements is crucial for optimizing customer engagements and improving overall communication strategies.
Building an AI Call Tagging Framework
To build an effective AI call tagging framework, clarity in objectives is essential. Start by defining what you want to achieve with AI call tagging, such as improving customer service or enhancing sales strategy. Specific objectives will guide your tagging process, ensuring relevance and efficiency. This step provides a foundation for measuring success and aligning your AI tools to meet these goals effectively.
Next, develop tagging categories that are intuitive and easy to use. These categories should encompass various aspects of customer interactions, allowing for detailed analysis and insights. Consider including areas like customer inquiries, product feedback, and agent performance. Implementing AI tools optimized for call tagging is equally important. Well-chosen tools will automate the tagging process and generate actionable insights, streamlining communication and decision-making. This strategic approach transforms your AI call tagging framework into a valuable asset for improving business outcomes.
Extract insights from interviews, calls, surveys and reviews for insights in minutes
Step 1: Define Your Objectives
Establishing clear objectives is essential when initiating an AI Call Tagging framework. Identifying your goals helps streamline the tagging process, ensuring that every call is analyzed in a manner that aligns with your overall business strategy. Consider what insights you want to derive from the call data, whether it’s improving customer satisfaction, enhancing sales effectiveness, or optimizing service delivery. When you define these objectives, you create a focused roadmap that guides the entire framework.
Next, it’s vital to communicate these objectives to your team. Involving your colleagues from the start fosters a collaborative environment and encourages everyone to share their perspectives and ideas. This way, you not only clarify your intentions but also elevate the framework by integrating diverse experiences. Ultimately, a well-defined set of objectives for your AI Call Tagging strategy strengthens your capacity to derive actionable insights and make data-driven decisions.
Step 2: Select the Right Tools for AI Call Tagging
In this step, it’s essential to select the right tools for AI Call Tagging to optimize your framework. Begin by identifying your specific needs, as various tools offer unique capabilities tailored for different business contexts. Look for software that can seamlessly integrate with your existing systems while providing accurate speech recognition and tagging functionalities. A tool's ability to analyze conversation tone, sentiment, and key topics can significantly enhance your call evaluation process.
Consider factors such as scalability, pricing, and user-friendliness when exploring options. It’s essential to select tools that support comprehensive reporting features, allowing for better insights into team performance and customer interactions. Popular options include Gong for deep analysis of conversations and Invoca for enhanced tracking and attribution. By choosing the right tools, you ensure that your AI Call Tagging system remains efficient, effective, and aligned with your business goals.
Insight7
To effectively implement an AI Call Tagging framework, understanding the core elements is essential. Insight7 explores how to streamline your process, ensuring valuable insights are extracted from customer conversations. Fostering clarity around goals will guide your framework's design and execution. Start with a clear vision of what you want to achieve through AI Call Tagging, as this sets the foundation for effective categorization and analysis.
Next, select the appropriate tools that enhance your AI Call Tagging capabilities. The right tools facilitate real-time insights and automate the tagging process, saving time and resources. Furthermore, creating distinct tagging categories helps in organizing the data collected and allows for insightful analysis. Implementation requires rigorous testing to confirm that the framework works efficiently and accurately captures essential customer insights, driving better business outcomes. Prioritizing these stages will maximize the value gleaned from customer interactions.
CallRail
In the journey of implementing AI call tagging, the tools you choose play a pivotal role in the overall effectiveness of your framework. Call tagging aids businesses in understanding customer interactions through insights drawn from conversations. One vital tool in this domain simplifies the process of tagging calls and offers a user-friendly interface for all employees, regardless of their technical expertise. This empowerment democratizes access to critical insights, ensuring that everyone in the organization can contribute to enhancing the customer experience.
As you build your AI call tagging framework, consider the features of this tool, which includes call library and transcript functionalities. The ability to visually analyze conversations and extract insights such as pain points or customer desires helps pinpoint specific areas for improvement. With this tool, you can efficiently manage various projects, analyzing multiple calls simultaneously to glean actionable data. In essence, integrating such a powerful tool can significantly enhance your AI call tagging initiatives while streamlining your workflow.
Gong
Incorporating AI Call Tagging through efficient tools can significantly enhance customer interactions. One such tool offers a structured approach to evaluate key aspects of sales calls, ensuring consistent communication standards across teams. By analyzing call transcripts, it assesses elements like greetings, engagement levels, and resolution effectiveness.
The framework enables businesses to develop tagging criteria tailored to their needs. For instance, you can break down the evaluation process into four core areas: introductions, engagement, product knowledge, and closure. Each area is scored, providing clear insights into representative performance. Such detailed breakdowns help pinpoint training needs and highlight areas for improvement, fostering a culture of continuous learning. By integrating these practices, you can refine your call strategies and improve overall customer satisfaction.
Invoca
When exploring AI call tagging, it's crucial to consider its role in enhancing call evaluation efficiency. This tool enables businesses to categorize and analyze calls effectively, allowing for better coaching and training of agents. By implementing a systematic tagging framework, organizations can ensure that the most relevant calls are scrutinized, leading to improved service quality and customer satisfaction.
Utilizing advanced AI-driven solutions allows teams to capture key insights during conversations. These insights can facilitate persistent performance improvements and help uncover trends that might otherwise go unnoticed. Businesses that adopt a comprehensive approach to AI call tagging can not only streamline their operations but also enhance their overall communication strategy. Therefore, investing in a robust tagging system is not just a technical preference but a strategic decision that can significantly impact customer engagement and retention.
Step 3: Develop Tagging Categories
Developing tagging categories is a crucial step in building an effective AI call tagging framework. This process involves identifying key areas or themes based on the type of calls being analyzed, ensuring that you can categorize and retrieve information efficiently. It begins with a thorough review of the conversations to pinpoint recurring topics, sentiments, and concerns raised by the callers. By focusing on these elements, you can create a structured system that enables easy access to valuable insights.
Once you have outlined your categories, define clear labels and criteria for each to streamline the tagging process. For instance, categories could include inquiries about specific products, customer feedback on service quality, or requests for support. This structured approach not only facilitates better data organization but also enhances the overall efficiency of AI call tagging, allowing businesses to make data-driven decisions with precision and confidence.
Step 4: Implementation and Testing
In the process of building an AI call tagging framework, Step 4 involves both implementation and testing, which are crucial stages for success. Once the tagging categories and tools are established, it’s essential to integrate these elements into the existing systems. This might include training staff on how to use the AI tools effectively and ensuring that the tagging aligns with organizational goals. During this stage, clear communication is vital to facilitate smooth transitions and encourage user adoption.
Testing the framework is equally important, as it helps identify any gaps or areas for improvement. Conducting trial runs with actual call data allows for adjustments based on real-world performance. Analyze the outcomes to validate accuracy and effectiveness. By iterating through this implementation and testing phase, businesses can refine their AI call tagging strategy, ultimately leading to improved insights and decision-making that enhance customer interactions.
Conclusion: Achieving Success with an AI Call Tagging Framework
Achieving success with an AI call tagging framework involves understanding its critical role in enhancing business communication. By effectively categorizing call data, organizations can identify customer needs and improve their services over time. This process empowers teams to gain actionable insights that foster continuous improvement and ultimately drive business growth.
Incorporating an AI call tagging framework allows for streamlined analysis of customer interactions, leading to improved training for customer service representatives. As businesses start to implement this framework, tracking the right metrics will also support ongoing optimization efforts. By embracing these strategies, organizations can ensure they remain responsive to customer feedback and improve their overall performance.