AI-Enhanced Qualitative Research offers a transformative approach to understanding community dynamics. In today's fast-paced environment, traditional qualitative methods often struggle with biases and time constraints, making it challenging for teams to extract valuable insights effectively. With the integration of AI, researchers can streamline data collection and analysis, ensuring quicker, more reliable findings that resonate with community needs.
By adopting AI tools, projects can transcend the limitations of manual analysis, enabling teams to uncover deeper themes and patterns in qualitative data. This enhancement not only improves the overall research quality but also ensures that insights are actionable, helping communities address their unique challenges with precision and empathy.
Analyze qualitative data. At Scale.
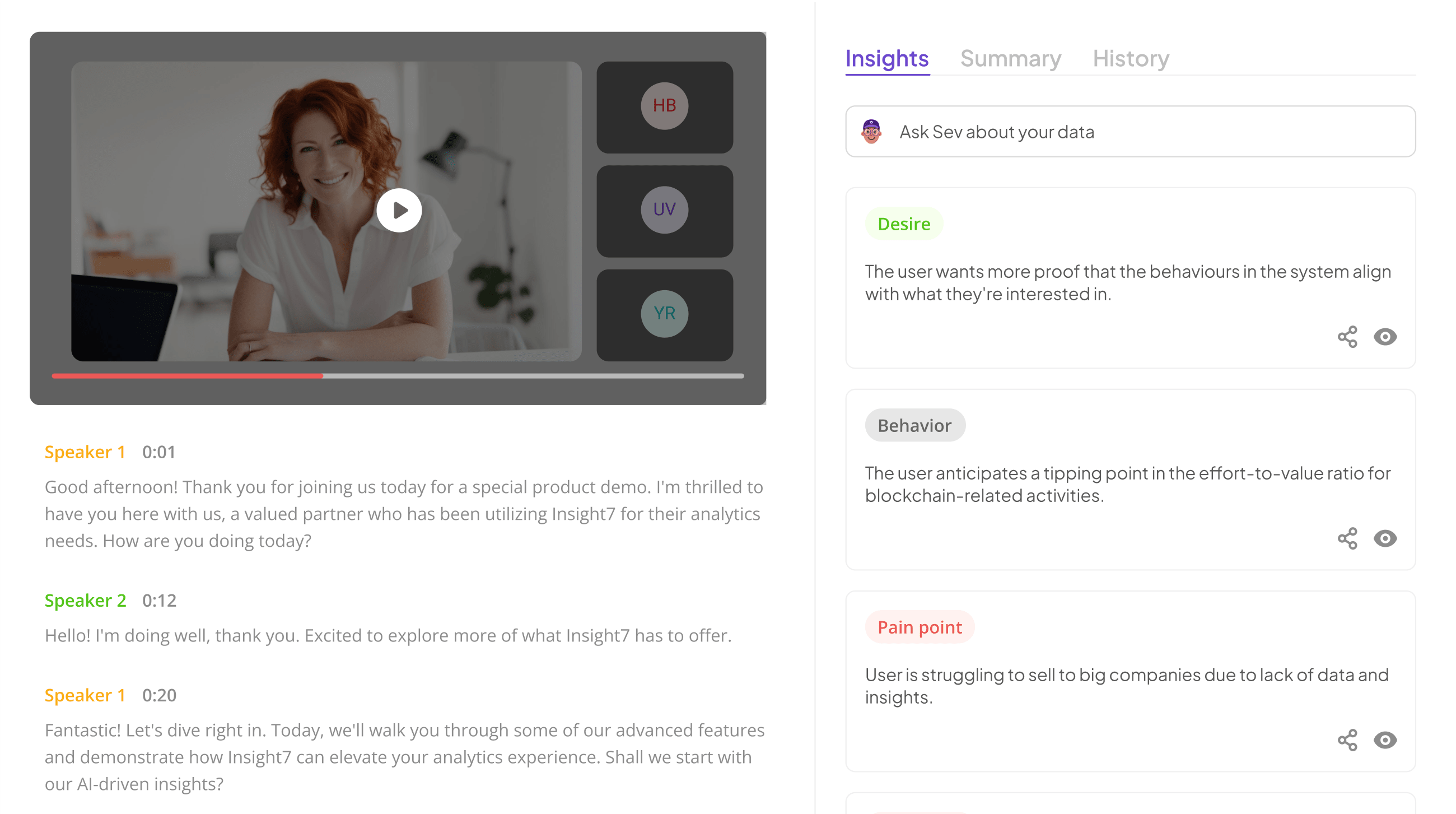
Understanding AI-Enhanced Qualitative Research
AI-Enhanced Qualitative Research revolutionizes traditional methods by integrating artificial intelligence to interpret complex data. This blending of technology and qualitative analysis helps researchers generate insights more efficiently and accurately. By automating tasks such as coding and theme identification, researchers can focus on deeper analysis and interpretation, thus enriching their findings.
Additionally, AI tools provide ways to analyze large volumes of qualitative data, which can often be overwhelming. Researchers can utilize AI to mitigate biases and inconsistencies that may arise in manual analysis. For community-based projects, where timely insights are crucial, AI-enhanced methods streamline processes, ensuring that stakeholders receive actionable insights quickly. Understanding AI's role in qualitative research not only enhances the quality of analysis but also broadens researchers' capabilities to uncover valuable insights that might have gone unnoticed using traditional methods.
What is AI-Enhanced Qualitative Research?
AI-Enhanced Qualitative Research combines traditional qualitative methodologies with advanced artificial intelligence techniques. This innovative approach aims to streamline data collection and analysis, offering researchers a more efficient means to derive insights from community-based projects. By integrating AI tools, researchers can analyze transcripts, interviews, and open-ended survey responses swiftly, reducing biases and inconsistencies in findings.
Moreover, AI-Enhanced Qualitative Research allows for deeper exploration of data through automated thematic analysis. Researchers can harness machine learning algorithms to identify patterns and trends that may elude manual analysis. This capability not only accelerates the research process but also enhances the accuracy of insights generated. In a landscape where timely and reliable data is crucial, AI-enhanced methods promise to transform the effectiveness and accessibility of community-based qualitative research projects.
Benefits of Using AI in Community-Based Research
Using AI in community-based research offers transformative benefits that significantly enhance the research process. Firstly, AI-Enhanced Qualitative Research streamlines data collection and analysis, allowing researchers to gather insights more efficiently. This technology can analyze vast amounts of qualitative data rapidly, identifying key themes and patterns that might otherwise go unnoticed. As a result, researchers can dedicate more time to engaging with communities rather than sifting through data manually.
Moreover, AI tools can enhance the precision and reliability of research findings. They offer improved visibility into the data source and can filter insights based on specific criteria, which fosters trust and transparency among community stakeholders. By providing tailored insights and recommendations, AI can empower communities to make informed decisions based on accurate data. Ultimately, AI-Enhanced Qualitative Research not only elevates the quality of findings but also strengthens community involvement and collaboration in the research journey.
Applying AI to Community-Based Qualitative Research
AI-Enhanced Qualitative Research is transforming community-based projects by providing innovative methods for data collection and analysis. One of the primary advantages of using AI tools is their ability to streamline the analysis process, minimizing biases and inconsistencies that can occur when multiple researchers work with qualitative data. AI can analyze vast amounts of interview transcripts and open-ended survey responses quickly, enabling teams to generate insights faster than traditional methods.
Implementing AI in qualitative research involves several essential steps. First, researchers must clearly identify their research goals and needs, ensuring that any AI tool aligns with their objectives. Next, selecting the appropriate AI tools is critical, as different software may offer varied functionalities suited to specific research contexts. Finally, integrating these tools into the research process allows for seamless collaboration among team members, enhancing the efficiency and accuracy of data interpretation. By thoughtfully applying AI, community-based qualitative research can achieve more reliable and actionable insights.
Extract insights from interviews, calls, surveys and reviews for insights in minutes
Steps to Implement AI in Qualitative Research
To effectively implement AI in qualitative research, start by identifying your specific research goals and needs. This initial step involves clarifying what you hope to achieve and determining the type of insights that will benefit your community-based project. Whether you aim to analyze interview transcripts or survey feedback, understanding your objectives will guide your choice of AI tools.
Next, select appropriate AI tools that align with your research goals. There are various platforms available, each offering unique features tailored for qualitative analysis. Investigate tools like NVivo or Dedoose, which can help streamline data processing and insight generation. Lastly, integrate these AI tools into your research process seamlessly. Train your team on using the chosen platforms effectively to enhance the overall quality and efficiency of your qualitative research. By following these steps, you elevate your approach to AI-enhanced qualitative research, ultimately delivering faster, more reliable insights.
- Step 1: Identifying Research Goals and Needs
Identifying research goals and needs is an essential first step in any qualitative research project. In community-based contexts, clarity in objectives can significantly impact the outcomes of AI-enhanced qualitative research. Begin by engaging stakeholders to understand their priorities and concerns. What specific questions do they want answered? This interaction provides foundational insights that shape the direction of the research.
Next, it’s vital to define the key themes or areas of interest. Are you focused on understanding community needs, assessing program effectiveness, or exploring cultural contexts? By pinpointing these goals, the research process can be streamlined, ensuring that AI tools will analyze relevant data points effectively. This approach not only enhances the quality of insights gathered but also fosters a sense of ownership among community members, making the project more inclusive and impactful.
- Step 2: Selecting Appropriate AI Tools
Choosing the right AI tools is crucial for effective community-based qualitative research. First, it’s essential to identify specific research needs. Different AI tools cater to various aspects, such as data analysis, report generation, or sentiment analysis. For instance, tools like NVivo or MAXQDA specialize in handling large volumes of qualitative data. They can assist in thematic analysis and help extract valuable insights efficiently.
Next, consider the capabilities of each tool in relation to your research goals. Evaluate how user-friendly they are and whether they include features like customizable reporting or data visualization. Assessing these functionalities will allow you to select tools that align closely with your objectives. By making well-informed choices, you ensure that your AI-enhanced qualitative research can provide meaningful insights into community dynamics and preferences.
- Step 3: Integrating AI Tools into the Research Process
Integrating AI tools into the research process is crucial for enhancing the quality and efficiency of community-based qualitative research. To begin, researchers should identify specific areas where AI can add value, such as data analysis, thematic coding, or voice recognition. This integration can streamline data collection by automating routine tasks, allowing researchers to focus on interpreting findings and engaging with stakeholders.
Next, it's essential to select AI tools that align with your research needs. Popular options include software designed for qualitative analysis, which can help manage large data sets and extract insightful themes. These tools not only improve accuracy but also enhance the overall efficiency of AI-enhanced qualitative research. By adopting these technologies in your research process, you can gain deeper insights and present findings that resonate with the community, ultimately leading to more informed decision-making.
Top AI Tools for Qualitative Analysis
When considering AI tools for qualitative analysis, it's essential to recognize how they can revolutionize AI-Enhanced Qualitative Research. These tools allow researchers to efficiently analyze qualitative data, automate repetitive tasks, and extract deeper insights from interviews and open-ended survey responses. The use of AI tools can help mitigate bias and inconsistencies often present in manual analysis, ensuring more accurate findings.
Several notable AI tools stand out in this field. Insight7 offers robust text analysis capabilities, enabling researchers to identify emerging themes quickly. NVivo is well-known for its intuitive interface that supports various data types, including text and audio. Atlas.ti excels in managing large amounts of qualitative data, providing visual tools for better comprehension. Dedoose is designed for mixed-methods data analysis, which can be particularly beneficial for community-based projects. Lastly, MAXQDA offers powerful coding options, making it easy to organize and interpret qualitative data. Integrating these tools into research projects can significantly enhance the efficiency and effectiveness of qualitative analysis.
- insight7
AI-Enhanced Qualitative Research has the potential to revolutionize how we gather and interpret community-based insights. With the continuous evolution of AI technologies, there exists a remarkable opportunity to delve deeper into qualitative data. By integrating advanced algorithms and machine learning tools, researchers can sift through vast amounts of unstructured data—such as interviews and open-ended survey responses—to uncover patterns and themes that might otherwise go unnoticed.
To effectively apply AI to qualitative research projects, it's essential to adopt a structured approach. Start by clearly identifying your research objectives and the community needs you're addressing. Next, choose appropriate AI tools that align with these goals, ensuring they enhance data collection and analysis processes. Finally, seamlessly integrate these tools into your research methodologies, allowing AI algorithms to streamline the interpretation and synthesis of qualitative insights, making the findings more accessible and actionable for community stakeholders.
- NVivo
In the realm of AI-Enhanced Qualitative Research, NVivo serves as a critical tool for analyzing qualitative data. It offers advanced capabilities for coding, sorting, and visualizing themes, making it easier to extract meaningful insights. Researchers can upload various data types, such as interviews, focus group discussions, and open-ended survey responses, allowing for comprehensive analysis within a single platform.
Furthermore, NVivo integrates AI features to enhance the qualitative analysis process. For example, its AI coding suggestions can help identify patterns and themes automatically, saving time and improving productivity. Additionally, visual tools such as charts and models make it easier to communicate findings to stakeholders clearly. As community-based projects grow increasingly complex, using NVivo can bridge the gap between qualitative insights and actionable outcomes through AI-driven capabilities, ensuring that researchers can address the diverse needs within their communities effectively.
- Atlas.ti
In the realm of AI-enhanced qualitative research, certain tools stand out for their capabilities in analyzing and interpreting complex data. One such tool allows researchers to pinpoint specific themes and patterns within their data, making the entire analysis process more efficient. This tool can swiftly audit data through predefined analysis kits, which assist in segmenting responses according to predefined goals, such as improving member experience or identifying risks.
Moreover, it enables users to define customizable tags and themes based on their project needs. By clustering insights into distinct categories, researchers can extract meaningful narratives from raw data. In addition, it offers sentiment analysis, allowing users to gauge emotions underlying the participants' feedback. Harnessing these features not only enhances the quality of community-based qualitative research but also optimizes time spent analyzing, delivering valuable insights that can drive impactful decisions.
- Dedoose
Dedoose plays a crucial role in AI-Enhanced Qualitative Research, especially within community-based projects. It is designed to streamline the analysis of qualitative data collected from various sources, such as interviews and surveys. The platform allows users to upload data, fostering collaboration among team members while ensuring efficient management of insights and findings.
One of the standout features of Dedoose is its ability to conduct real-time data analysis. Researchers can quickly identify themes, quotes, and trends, significantly reducing the time spent on manual data processing. With a user-friendly interface, Dedoose makes it easier to transcribe and analyze data across multiple channels, providing a comprehensive view of the research findings. This capability is invaluable for community-based projects, where collaboration and swift decision-making are essential for success.
- MAXQDA
In the realm of AI-Enhanced Qualitative Research, the MAXQDA software stands as a powerful tool for researchers aiming to streamline their analysis. With its user-friendly design, it enables seamless qualitative and mixed-methods research. This software allows users to organize qualitative data, analyze interviews, and visualize findings, making it ideal for community-based projects that require in-depth understanding.
Researchers often face challenges when sifting through large volumes of qualitative data. The MAXQDA tool significantly alleviates this burden by automating various aspects of data coding and analysis. Its features also facilitate collaboration among team members, enhancing communication and ensuring uniformity in data interpretation. In a rapidly evolving research environment, leveraging such tools is crucial for gaining comprehensive insights that can drive meaningful change in communities.
Conclusion: The Future of AI-Enhanced Qualitative Research
The future of AI-Enhanced Qualitative Research holds significant promise for community-based projects. As AI tools become increasingly sophisticated, they will revolutionize data collection and analysis, allowing researchers to gain deeper insights more efficiently. By automating time-consuming tasks like transcribing interviews or extracting themes from open-ended survey responses, researchers can focus on interpreting data and applying findings to real-world challenges.
Moving forward, embracing AI will foster enhanced collaboration among researchers and community stakeholders. It can minimize bias, standardize analysis, and ultimately lead to more reliable insights. This evolution in qualitative research empowers communities to leverage data-driven strategies for improved outcomes, paving the way for transformative progress in understanding and addressing social issues.