How AI Predicts Call Center Agent Efficiency for Maximum Utilization
-
Bella Williams
- 10 min read
In an era where customer service demands are escalating, the role of call center agents has never been more critical. Predictive Agent Efficiency emerges as a key solution, utilizing advanced AI techniques to assess and enhance agent performance. This innovative approach enables organizations to analyze real-time data, forecast outcomes, and ultimately drive better service delivery.
Understanding how AI predicts agent efficiency is vital for maximizing utilization in call centers. By harnessing the power of historical data and machine learning, companies can gain insights into individual agent capabilities. This knowledge leads to informed decision-making, fostering an environment where agent strengths are matched to specific customer needs, thereby increasing overall satisfaction.
Analyze & Evaluate Calls. At Scale.
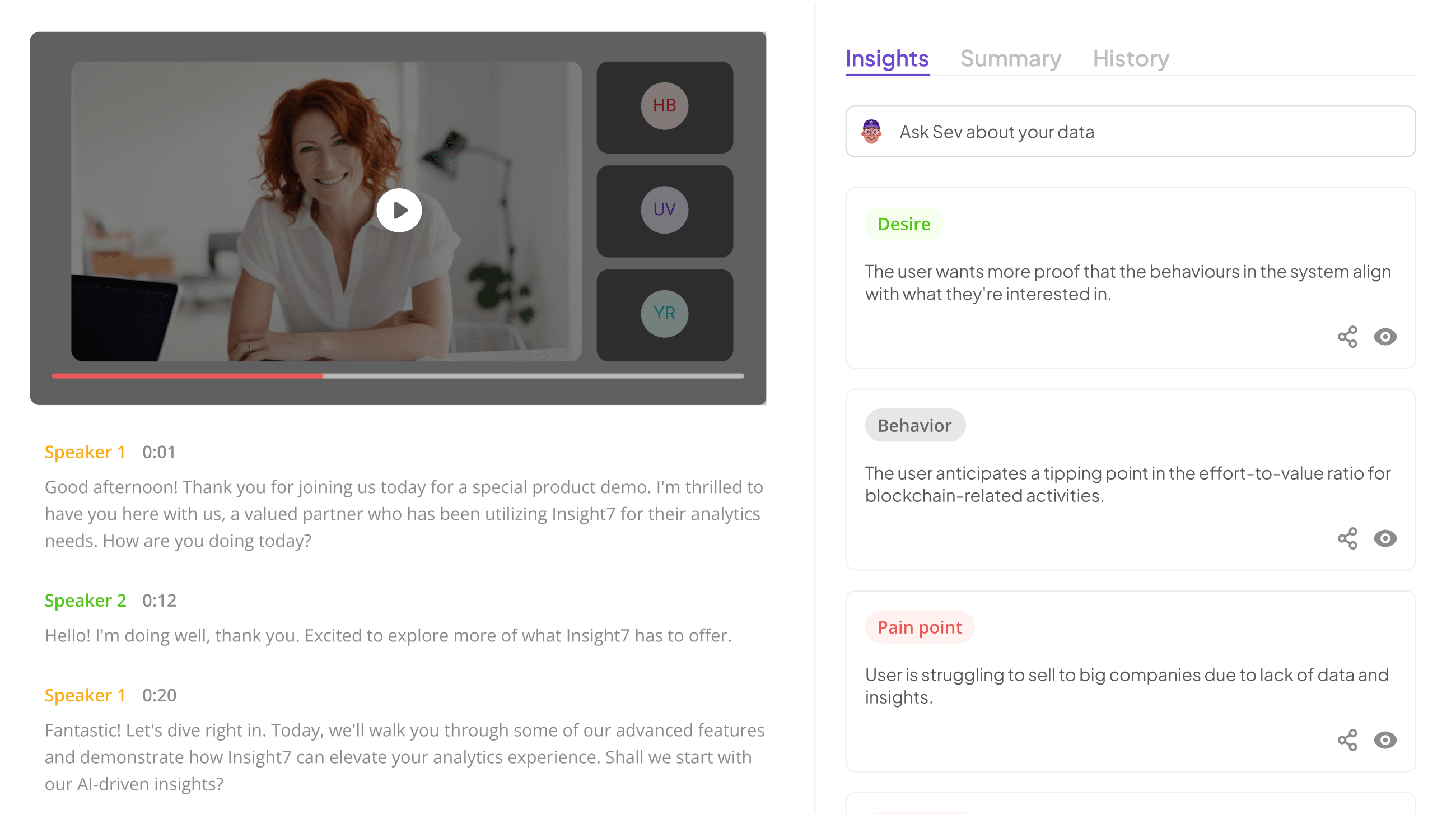
Understanding Predictive Agent Efficiency Analytics
Understanding Predictive Agent Efficiency Analytics is essential for optimizing call center operations. It involves using advanced data analysis techniques to assess an agentโs performance over time. By employing historical data, organizations can develop a clearer picture of individual agent strengths and areas for improvement. This information not only informs management strategies but also contributes to better resource allocation.
Predictive Agent Efficiency utilizes machine learning models that analyze patterns in call data. These models evaluate various performance metrics, such as average handling time and customer satisfaction. Consequently, managers receive insights that empower them to make data-driven decisions about training and scheduling. Furthermore, understanding these analytics can enhance communication, enabling agents to perform at their best, ultimately leading to improved overall efficiency within the call center.
The Importance of Historical Data
Historical data serves as the foundation upon which predictive agent efficiency is built. By analyzing past interactions, call centers can uncover patterns that inform decision-making. This data reveals agent performance metrics, customer sentiments, and operational trends, all of which are crucial for effective forecasting and efficiency enhancement.
Furthermore, historical data allows AI systems to learn from previous outcomes. For instance, by examining past call resolutions, the AI can identify optimal strategies and predict future performance. This predictive capability is vital for ensuring that agents are utilized to their maximum potential. As a result, historical data not only supports accurate predictions but also fosters a culture of continuous improvement within the call center environment. By harnessing this data, organizations can make informed adjustments that lead to greater efficiency and enhanced customer satisfaction.
Machine Learning Models for Predictive Analysis
Machine learning models play a crucial role in enhancing predictive agent efficiency within call centers. These models utilize vast amounts of historical data to identify patterns and trends that impact agent performance. By analyzing various metrics, including call handling times, customer satisfaction scores, and agent availability, organizations can gain valuable insights into effective resource allocation.
In developing predictive models, several approaches can be employed. Firstly, regression models can predict agent performance based on defined historical factors. Secondly, classification algorithms determine agent suitability for specific queries, optimizing customer interactions. Finally, ensemble methods can combine multiple model outputs for improved accuracy. By implementing these machine learning techniques, call centers can better understand agent efficiency, leading to maximized utilization and enhanced customer experiences.
Tools for Predictive Agent Efficiency
Understanding the tools for predictive agent efficiency is crucial to maximizing call center utilization. These tools leverage machine learning and advanced analytics to assess agent performance effectively. By analyzing past interactions and workload patterns, organizations can forecast future behavior, enabling better resource allocation and training. This proactive approach helps improve agents' effectiveness while enhancing customer satisfaction.
Key tools for enhancing predictive agent efficiency include specialized software solutions. These platforms typically provide insights into call volumes, agent availability, and customer interactions. As a result, managers can identify strengths and weaknesses in their teams, facilitating targeted coaching. Furthermore, these insights allow for timely adjustments in scheduling to meet the demands of peak call periods. Overall, adopting such tools positions call centers to optimize efficiency and maintain high standards of service.
insight7
To harness predictive agent efficiency, it is essential to analyze various factors impacting call center performance. Understanding the nuances of agent interactions and customer responses inform how AI models can be tailored for maximum utilization. By gathering data from numerous customer conversations, organizations can unveil patterns that highlight where improvements are necessary.
Effective predictive analysis begins with a comprehensive evaluation of historical performance metrics. These metrics guide machine learning models in identifying which agents excel in different scenarios. By implementing AI-driven insights, call centers can optimize scheduling, training, and resource allocation. As agents become equipped with more targeted tools and knowledge derived from predictive analysis, they can engage more effectively with customers, leading to increased satisfaction and performance. Ultimately, adopting robust predictive agent efficiency frameworks ensures that call center operations are both efficient and responsive, driving business success.
Five9
In the evolving realm of call centers, understanding predictive agent efficiency becomes paramount. This platform utilizes advanced algorithms to analyze historical call data, identifying key patterns that enhance agent performance. By embracing this technology, organizations can optimize their workforce and improve response times. The core principle here is maximizing the potential of each call center agent through data-driven insights derived from past interactions.
Predictive agent efficiency not only streamlines operations but also tailors coaching to individual agent needs. This approach allows supervisors to focus on specific skill gaps, fostering a culture of continuous improvement. Furthermore, the integration of such tools enables proactive engagement with customers, ensuring their needs are met even before they are explicitly stated. As businesses recognize the value of these insights, they are better equipped to meet customer expectations while maintaining high operational standards.
NICE inContact
NICE inContact serves as a pivotal player in understanding how AI can predict call center agent efficiency. By harnessing advanced analytics and AI algorithms, this system evaluates numerous factors that influence call center performance. The tool optimizes agent scheduling based on anticipated call volumes, thereby enhancing overall efficiency and productivity.
Through predictive agent efficiency capabilities, the system analyzes data trends and historical performance metrics, allowing for proactive management of resources. It informs management on agent strengths and areas for improvement, ensuring that training and development programs are aligned with organizational goals. This leads to maximized utilization of workforce capabilities, creating a more agile and responsive call center environment. With such tools, businesses can achieve better customer satisfaction and operational excellence.
Genesys
In the evolving landscape of call centers, understanding predictive agent efficiency can significantly enhance performance. This involves utilizing advanced technologies to analyze past interactions, thereby anticipating future agent requirements. By implementing robust analytics tools, call centers can identify patterns in agent performance and customer interactions, leading to improved decision-making.
One effective approach involves deploying AI-driven platforms that provide real-time insights into agent efficiency. These tools not only streamline the assessment process but also enable organizations to align their efforts with emerging trends. For instance, such systems can help optimize staff schedules based on predicted call volume or customer needs, ensuring resources are allocated efficiently. By harnessing predictive analytics, businesses can foster a proactive environment, transforming how they manage and utilize their workforce to maximize overall call center performance.
Talkdesk
In the realm of advancing call center operations, platform innovation plays a crucial role. This platform empowers organizations to maximize operational efficiency through predictive analytics. By leveraging historical data and machine learning, it gives insights that help optimize agent performance. With a user-friendly interface, it streamlines the process of transcribing and analyzing calls. Users can drop audio files for bulk transcription, making it easier to extract vital customer insights rapidly.
Understanding Predictive Agent Efficiency is central to improving service delivery. The platform allows real-time analysis of conversations, revealing trends and areas needing improvement. With tailored templates, organizations can focus on understanding customer pain points and agent responses. This holistic view not only enhances operator efficiency but also ensures a better customer experience. Overall, innovative tools in this space are reshaping how call centers approach efficiency, integrating predictive capabilities to foster growth and responsiveness.
Extract insights from interviews, calls, surveys and reviews for insights in minutes
Steps to Implementing Predictive Efficiency Models
To effectively implement predictive efficiency models in call centers, it's essential to follow structured steps that ensure accuracy and usability. The first step involves robust data collection and preprocessing. Gather historical call data, customer interactions, and agent performance metrics to form a comprehensive dataset. Clean and organize this information to eliminate any discrepancies and prepare it for analysis.
After data preparation, the next step is model training and validation. Using machine learning algorithms, develop predictive models that can analyze patterns in agent behavior and customer interactions. By applying various validation techniques, assess the models' reliability and ensure they accurately forecast agent efficiency.
Finally, integration and continuous improvement are vital for success. Seamlessly incorporate the predictive models into existing systems, allowing real-time insights for supervisors and agents. Regular assessment and updates of the models will ensure that they adapt to evolving conditions and maintain high utilization rates among call center agents.
Step 1: Data Collection and Preprocessing
To effectively predict agent efficiency, the initial step is gathering and preprocessing relevant data. This includes collecting call records, customer feedback, and performance metrics from various sources. Data must be comprehensive and encompass different elements such as call duration, resolution rates, and agent interactions. Aggregating this information allows for a clearer picture of agent performance and success rates, setting the stage for deeper analysis.
Once the initial data is gathered, preprocessing is vital. This involves cleaning the data to remove inconsistencies, such as incorrect entries or duplications, and standardizing formats for smooth analysis. Additionally, categorizing responsesโlike positive or negative customer feedbackโhelps in organizing the insights to be derived. Through this meticulous process, the framework for understanding predictive agent efficiency emerges. Such structured data ultimately empowers AI to uncover trends that can drive efficiency and optimize agent utilization.
Step 2: Model Training and Validation
In Step 2: Model Training and Validation, the focus shifts to the core processes of enhancing Predictive Agent Efficiency. Here, we utilize the clean and structured data gathered during the first step. The choice of algorithm plays a crucial role in the accuracy of predictions. Different machine learning models, such as regression analysis or decision trees, can be evaluated to determine which one best fits the data characteristics and business needs.
The validation phase ensures that the model's predictions can hold up under various scenarios. This step typically involves splitting the dataset into training and testing sets to assess the model's performance. Metrics like accuracy, precision, and recall help gauge effectiveness and guide further adjustments. By iterating through modeling and validation, organizations can refine their predictive models, ultimately leading to improved decision-making regarding agent training and resource allocation.
Step 3: Integration and Continuous Improvement
Integrating predictive agent efficiency models into a call center requires more than just implementation; it demands a commitment to continuous improvement. This means regularly assessing the effectiveness of the AI systems in predicting agent performance. By analyzing call metrics and agent feedback, organizations can identify areas for training and development, thereby enhancing overall agent productivity.
To ensure lasting success, it's crucial to create a feedback loop. Begin by reviewing actual performance against AI predictions, allowing adjustments to be made where necessary. Next, incorporate regular training sessions based on emerging patterns in call data to refine agent skills. Finally, foster a culture of open communication where agents can share their experiences, giving valuable insights that can drive further optimization. Through this cycle of evaluation and adaptation, call centers can realize the maximum potential of predictive agent efficiency, leading to improved service quality and customer satisfaction.
Conclusion: Maximizing Call Center Utilization with Predictive Agent Efficiency
By embracing predictive agent efficiency, call centers can transform their operations for optimal utilization. This approach not only streamlines agent performance but also anticipates customer needs, enabling a proactive response to inquiries. Data analytics enhances training methods, allowing centers to focus on relevant areas that improve employee engagement and satisfaction.
As organizations harness these insights, they can elevate customer service quality through tailored agent development. Ultimately, integrating predictive models leads to reduced operational costs and better resource allocation, ensuring that call centers operate at peak efficiency while meeting customer expectations. The journey towards excellence in call center management starts with adopting predictive agent efficiency.
๐ฌ Need Help? Chat with Support
Our team typically responds within minutes