AI-Driven Qualitative Insights are revolutionizing the way researchers uncover and interpret complex data. Imagine sifting through hundreds of interviews or focus group transcriptions without feeling overwhelmed. Traditional qualitative research can be labor-intensive and prone to bias, but the integration of AI can streamline this process, enabling researchers to gain valuable insights quickly and efficiently.
In this section, we will explore how AI enhances discovery in qualitative research projects. By employing advanced data analysis techniques, such as natural language processing, researchers can uncover trends and patterns that might go unnoticed using manual methods. With these AI-driven solutions, understanding participant sentiments and experiences becomes less daunting, allowing researchers to focus on creating actionable outcomes from their findings.
Analyze qualitative data. At Scale.
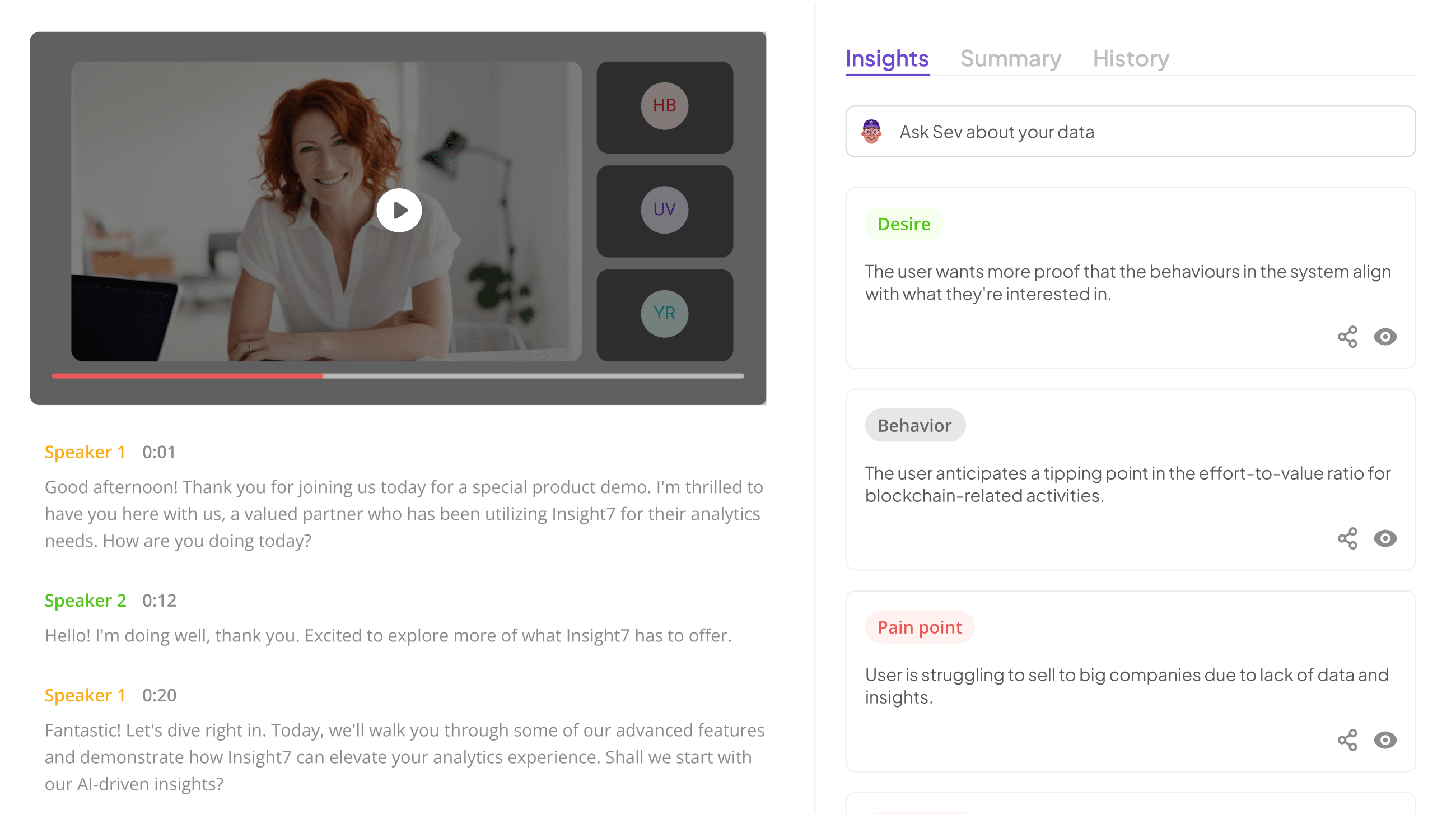
Transforming Traditional Methods with AI-Driven Qualitative Insights
Traditional methods of qualitative research often involve time-consuming processes and manual analysis that may hinder timely insights. With the introduction of AI-Driven Qualitative Insights, researchers can now enhance these methods significantly. AI technologies streamline the data analysis process, transforming how qualitative insights are gathered and interpreted.
By integrating AI tools, researchers can quickly sift through vast amounts of data, identifying trends and patterns that may otherwise be overlooked. For instance, Natural Language Processing (NLP) enables automated text analysis, allowing teams to focus on strategic decision-making rather than data collection. Moreover, AI models enhance collaboration by providing centralized insight repositories, which facilitate efficient sharing and discussion among team members. Thus, AI not only transforms traditional research methodologies but also empowers teams to generate actionable insights that can drive business strategies forward.
AI Integration into Qualitative Research
Incorporating AI into qualitative research fundamentally reshapes how researchers uncover insights. Through AI integration, traditional methods are enhanced, allowing for a deeper understanding of participant perspectives. Automated data analysis tools can quickly process large volumes of qualitative data, revealing patterns that may go unnoticed by human analysts. This swift processing capability means researchers can derive AI-driven qualitative insights much faster, enabling timely decision-making.
The key benefits of this integration include improved accuracy in data interpretation, enhanced scalability for larger projects, and increased efficiency in research workflows. By automating repetitive tasks, researchers have more time to focus on refining their strategies and engaging with participants. This dynamic shift not only elevates the quality of insights generated but also fosters innovative approaches to qualitative research, paving the way for organizations to remain competitive in a continuously evolving landscape.
- Understanding the role of AI in enhancing traditional qualitative methodologies
AI-Driven Qualitative Insights can significantly reshape how researchers approach traditional qualitative methodologies. By integrating AI tools, researchers can enhance data collection and analysis, streamlining processes that previously required extensive manual effort. These intelligent technologies are adept at processing vast amounts of data, quickly identifying patterns and themes within qualitative feedback that human researchers might overlook.
The role of AI in enhancing qualitative methods can be understood through several key aspects. First, AI algorithms can analyze interview transcripts and open-ended survey responses with remarkable precision, saving researchers time. Second, AI-powered tools bring forth insights that contribute to more profound understanding and actionable findings. Lastly, the ability to adapt AI frameworks ensures that researchers can maintain specificity and relevance in their inquiries, fostering greater accountability and depth in qualitative research. Embracing AI can ultimately lead to richer, more insightful discoveries in qualitative projects.
- Key benefits of incorporating AI technologies in research processes
Incorporating AI technologies in research processes provides significant benefits that enhance qualitative analysis. First, AI-driven qualitative insights streamline data processing, allowing researchers to analyze vast amounts of qualitative data efficiently. This enhances the ability to identify patterns, themes, and anomalies more quickly than traditional methods. Second, the integration of AI tools can minimize biases in data interpretation, ensuring a more objective approach to qualitative research.
Furthermore, AI technologies can automate mundane tasks such as coding and categorizing responses, enabling researchers to focus on deeper analytical work. Automated summaries offer a high-level overview of insights derived from extensive interviews or focus groups. With these advantages, researchers can make informed decisions based on real-time data, ultimately accelerating the discovery process and enhancing the overall quality of their findings. By marrying technology with qualitative methodologies, the potential for innovative insights expands significantly.
AI-Driven Data Analysis Techniques
AI-driven data analysis techniques significantly enhance qualitative research by uncovering valuable insights from extensive datasets. Utilizing Natural Language Processing (NLP), researchers can analyze text, identify patterns, and extract key themes from transcriptions efficiently. This technology accelerates the process of digesting interviews or focus group discussions, turning overwhelming amounts of raw data into actionable insights.
Additionally, machine learning models play a crucial role in recognizing data patterns that may go unnoticed in traditional qualitative analysis. By applying these models, researchers can identify trends across numerous conversations, such as common pain points or frequently mentioned themes. This not only aids in summarizing findings but also provides a deeper understanding of participant experiences. With the capacity to transform qualitative data into structured reports, these AI-driven qualitative insights offer researchers a practical approach to informing decision-making and refining research strategies.
- Natural Language Processing (NLP) for text analysis
Natural Language Processing (NLP) plays a critical role in transforming how we analyze text in qualitative research. This technology allows researchers to analyze large volumes of unstructured data, which traditional methods struggle to manage. By utilizing NLP, teams can identify themes, sentiments, and nuances in conversations more efficiently. This AI-driven approach enhances qualitative insights, leading to faster and more actionable results.
When integrating NLP into text analysis, several key benefits emerge. First, it accelerates the data analysis process, enabling researchers to extract insights quickly. Second, it improves the accuracy of identifying subtle patterns and sentiments within customer conversations. Lastly, it fosters better collaboration by centralizing insights in a systematic way, reducing the chaos of scattered information. With AI-driven insights at the forefront, qualitative research can evolve, empowering teams to make informed, strategic decisions swiftly.
- Machine learning models for data pattern recognition
Machine learning models for data pattern recognition are essential for unlocking AI-driven qualitative insights. These models analyze large datasets efficiently, identifying patterns that might not be visible through traditional methods. By employing algorithms, researchers can automatically classify and interpret qualitative data, which accelerates the discovery of significant themes and trends.
The automation of pattern recognition leads to more accurate analyses and helps highlight relationships between variables. For instance, researchers can assess customer feedback from various sources, identifying sentiment trends across multiple datasets. This approach saves time and resources while enhancing research depth. As AI continues to evolve, integrating machine learning models into qualitative research processes will become increasingly beneficial, enabling more profound insights that lead to informed decision-making and innovative solutions.
Extract insights from interviews, calls, surveys and reviews for insights in minutes
Top AI Tools for Enhancing Discovery in AI-Driven Qualitative Insights
In the world of AI-driven qualitative insights, various tools can significantly improve the discovery process. These tools leverage advanced technologies to transform data into meaningful insights that guide decision-making. Each tool comes with unique features designed to simplify the analysis of qualitative data, making it accessible for researchers at all levels.
Quirkos offers an intuitive interface that facilitates thematic analysis, allowing users to visualize data trends effectively. NVivo is known for its ability to provide advanced data visualization and organization capabilities, supporting detailed qualitative research. Atlas.ti emphasizes comprehensive text-based research analysis, making it easier to understand complex data. Dedoose integrates both qualitative and mixed-method research approaches, enhancing the research flexibility. Lastly, MAXQDA stands out with its versatile data analysis options, catering to various research needs. These AI tools collectively enhance discovery in qualitative insights, driving more informed outcomes in research projects.
Insight7: Leading the Way in AI-Enhanced Research
AI-driven qualitative insights are transforming how researchers approach and execute their qualitative projects. The integration of artificial intelligence significantly enhances traditional qualitative methodologies by streamlining data collection and analysis processes. With sophisticated algorithms in place, researchers can quickly transform vast amounts of interview and survey data into actionable insights.
Additionally, AI tools empower researchers to identify patterns and themes within qualitative data that may not be immediately apparent through manual analysis. By automating data processing, these tools reduce the time spent on analysis, allowing researchers to focus on interpreting results and strategizing effectively. As this technology evolves, organizations embracing AI-enhanced research will likely gain a competitive edge, enabling informed decision-making and driving impactful outcomes in their projects.
- Overview of features and benefits specific to Insight7
The integration of advanced AI technologies into qualitative research brings forth a suite of powerful features tailored to enhance the discovery process. Key features include sophisticated Natural Language Processing (NLP) capabilities for analyzing large volumes of text data quickly and accurately. This technology enables researchers to identify patterns and themes that may be easily overlooked in traditional methods. Additionally, AI algorithms can automate repetitive tasks, freeing up researchers to focus on deeper insights and critical analysis.
The benefits associated with these features are substantial. AI-driven qualitative insights provide enhanced accuracy and speed, allowing for quicker turnaround times on research findings. This efficiency not only accelerates decision-making but also supports a more agile research environment. Furthermore, the ability to handle multi-language analysis opens doors to more comprehensive insights across diverse markets, ultimately fostering better understanding and richer interpretation of qualitative data. This transformative impact positions AI as a pivotal element in elevating qualitative research projects to new heights.
- Case studies illustrating the impact of Insight7 in qualitative research
In the realm of qualitative research, AI-Driven Qualitative Insights have become a transformative force. Case studies showcasing the effectiveness of Insight7 reveal how organizations are overcoming traditional data analysis hurdles. Initially, many teams struggled with the sheer volume of customer interviews and conversation data. The manual analysis often proved time-consuming, leading to delayed insights and ineffective decision-making.
By integrating Insight7, teams experienced a significant acceleration in their research processes. The platform's AI capabilities facilitated swift data analysis, enabling real-time insights that empowered businesses to act promptly. In one prominent case, a consulting firm was able to synthesize client feedback into actionable strategies in a fraction of the time previously required. This effectiveness not only improved internal collaboration but also helped the firm strengthen its competitive edge in a rapidly evolving market. These examples underscore the profound impact of leveraging AI for qualitative research, leading to more efficient operations and enhanced decision-making capabilities.
Other Essential AI Tools for Qualitative Insights
In addition to popular platforms like Insight7, various other AI tools enhance the discovery process by providing vital qualitative insights. Each tool offers unique functionalities that help researchers better understand their data and uncover meaningful patterns. For instance, Quirkos facilitates a user-friendly thematic analysis, making it easier for researchers to visualize codes and themes. This intuitive interface allows a more interactive approach to qualitative analysis.
Similarly, NVivo differentiates itself with advanced data visualization features, enabling researchers to organize and present their findings more effectively. Atlas.ti offers comprehensive capabilities for text-based research analysis, including coding and detailed data management options. Tools like Dedoose excel in integrating qualitative and mixed-method research, broadening the scope of analysis. Lastly, MAXQDA provides flexible data analysis options, appealing to various research needs.
These tools collectively enhance AI-driven qualitative insights, ensuring researchers can navigate complex data landscapes with greater ease and accuracy.
- Quirkos: User-friendly interface for thematic analysis
A user-friendly interface for thematic analysis revolutionizes how researchers conduct qualitative studies. By simplifying the research process, it enables users, regardless of their technical expertise, to seamlessly navigate through data. This platform provides intuitive tools that facilitate data entry and analysis while ensuring that AI-Driven Qualitative Insights are readily accessible to everyone involved.
Key features include efficient library management, where research files and transcripts are organized effectively. Users can quickly extract meaningful insights from conversations with just a few clicks. The interface’s visual aids help in identifying patterns, pain points, and customer sentiments, making it easier to summarize findings. Moreover, the ability to analyze multiple projects simultaneously amplifies the researcher's capacity to derive comprehensive insights, ensuring that data-driven decisions can be made efficiently. Ultimately, this approach not only democratizes data insights but also enhances the overall quality of qualitative research.
- NVivo: Advanced data visualization and organization capabilities
Advanced data visualization and organization capabilities significantly enhance qualitative research projects. By streamlining data management, researchers can effortlessly sort and analyze vast amounts of qualitative data. This process allows for quick identification of themes, trends, and connections within the data, essential in deriving AI-driven qualitative insights that were previously time-consuming to uncover.
One notable feature involves the ability to create projects effortlessly. Researchers can upload various data types, including transcripts and audio files, which can be integrated from multiple sources. Concurrently, advanced tools allow for visualizing historical queries, making it easier to extract relevant quotes or testimonials efficiently. Utilizing matrix analysis capabilities simplifies complex data interpretations, enabling researchers to answer specific questions and discover valuable insights. This capability ensures that qualitative research remains organized and easily accessible, fostering deeper analysis and informed decision-making.
- Atlas.ti: Comprehensive text-based research analysis
In qualitative research, comprehensive text-based analysis is essential for distilling rich insights from diverse data sources. AI-driven qualitative insights provide researchers with the tools to synthesize vast amounts of text quickly, identifying themes and patterns that might otherwise remain hidden. By employing advanced natural language processing techniques, researchers can extract meaningful data from interviews, focus groups, or even social media content without the traditional manual effort.
Utilizing dynamic project settings facilitates the organization and analysis of qualitative data. Researchers can easily upload and manage multiple files, allowing them to draw comprehensive insights from a unified source. The platform encourages collaboration and provides an intuitive interface that streamlines the analysis process. This leads to timely and effective decision-making, enhancing the overall quality of research outcomes. Empowered by these capabilities, researchers can derive AI-driven qualitative insights to inform strategies and address specific research challenges more effectively.
- Dedoose: Integrating qualitative and mixed-method research
Integrating qualitative and mixed-method research enhances the value of AI-driven qualitative insights. By focusing on various data sources, researchers can gather richer, more nuanced information. This integration allows for the combination of qualitative data, such as interviews and open-ended survey responses, with quantitative findings, enabling a more comprehensive analysis. Engaging multiple methods improves the validity of research outcomes and supports the identification of complex patterns within data.
Moreover, mixed-method research offers flexibility in analysis. Researchers can explore initial qualitative findings, then test those insights through quantitative measures. This iterative process leads to robust, data-driven conclusions. As AI tools facilitate real-time data analysis, researchers can quickly iterate and refine their research strategies. Ultimately, this approach not only strengthens the findings but also contributes to a deeper understanding of research subjects, paving the way for more informed decision-making in various fields.
- MAXQDA: Flexible and versatile data analysis options
Data analysis in qualitative research often requires adaptability and sophistication, which is where MAXQDA excels with its flexible and versatile options. This software allows researchers to seamlessly compile various data, such as interviews, surveys, and audio recordings, into cohesive projects. Whether importing files from Google Drive or transcribing audio into text, the user-friendly interface invites researchers to efficiently manage their data.
Within these projects, researchers can ask specific questions to extract meaningful insights. For instance, by utilizing matrix tools, they can detail customer sentiments regarding a product. This targeted analysis swiftly reveals trends and patterns across the collected data. The integration of AI-driven features within MAXQDA enhances the depth of qualitative analysis, making it an invaluable tool for uncovering AI-driven qualitative insights that drive informed decision-making in research projects.
Conclusion: The Future of AI-Driven Qualitative Insights in Research
The future of AI-driven qualitative insights promises to revolutionize the landscape of research projects. As organizations increasingly adopt these advanced technologies, they will unlock new dimensions of understanding from vast amounts of qualitative data. Researchers will benefit from enhanced accuracy and efficiency, allowing them to focus on deeper analyses and creative solutions.
Moreover, AI’s ability to process and analyze multiple data sources simultaneously will encourage more informed decision-making. The integration of user-friendly tools will empower professionals across all levels, enabling everyone to extract meaningful insights. Ultimately, AI-driven qualitative insights will reshape how we perceive and utilize research in various fields, enhancing the overall impact of qualitative methodologies.