But first, why is ethnographic data analysis essential? Unlike other methodologies, ethnography centers on lived experiences. It uses participant observation, interviews, and cultural immersion to uncover insights that traditional data analysis might miss. This guide demystifies those methods and helps you answer questions such as, “What is ethnographic data?” and “How do I code ethnographic data effectively?”
Before exploring practical steps and tools to enhance your research, let’s dive into the foundational concepts.
Understanding the Basics of Ethnographic Data Analysis
Ethnographic data analysis refers to interpreting data collected during ethnographic research. Ethnography is a qualitative research method that studies people within their natural environments, often using participant observation, interviews, and field notes.
To start, it’s essential to understand what ethnographic data entails. Ethnographic data includes any information gathered from these immersive research practices, such as transcripts of interviews, observational notes, photographs, and even artifacts. But how do you make sense of all this data? That’s where analysis methods come into play.
One foundational approach is ethnographic coding. Coding ethnographic data involves identifying themes, patterns, and categories within the data, providing a framework for interpretation. For example, researchers may code for recurring words, phrases, or behaviors that signify underlying social norms or values when analyzing interview transcripts.
Another critical aspect is contextual analysis. Ethnographic analysis prioritizes understanding data within its cultural and social context. Unlike traditional data analysis focusing on numerical results, ethnography asks: What do these observations mean within this specific environment?
Finally, ethnographic content analysis (ECA) extends this concept by blending qualitative and quantitative techniques. ECA evaluates patterns while preserving the data’s context and meaning—a skill especially useful in interdisciplinary research.
These foundational concepts are essential for anyone looking to understand the nuances of ethnographic research methods.
Now that you’ve grasped the basics let’s move on to a step-by-step breakdown of how to analyze ethnographic data effectively.
Read: AI Comparative Analysis Software: Choosing the Right Tool
Key Ethnographic Data Analysis Techniques
Descriptive Coding Methods in Ethnographic Studies
Analytical Coding for Deeper Ethnographic Insights
Read: Top 7 Qualitative Market Research Tools
Step-by-Step Process for Ethnographic Data Analysis
Analyzing ethnographic data requires a structured approach to ensure that the rich, qualitative insights are fully understood and translated into actionable conclusions. Below, we outline a step-by-step process that demystifies the task of ethnographic data analysis, helping you move from raw data to meaningful results.
Step 1: Organize Your Data
The first step in analyzing ethnographic data is organizing your collected information. Ethnographic research often generates diverse data types, including interview transcripts, observational notes, photos, and videos. Start by categorizing and digitizing this data so that it’s easier to navigate.
For example, the participant can label interview transcripts, while field notes can be grouped by location or observation type. Using a research repository, such as Insight7, can streamline this process. Insight7 allows you to store all your data in a single, project-based repository that’s easy to search and share with your team. This organization is crucial for identifying patterns later.
Step 2: Familiarize Yourself with the Data
Before you can start coding or analyzing, it’s essential to immerse yourself in the data. Read through the transcripts, review your notes, and revisit your photos or videos multiple times. Look for repeated themes or interesting insights that stand out.
For instance, if your research focuses on consumer habits, you may notice recurring behaviors or shared cultural references. Highlight these during your review. This initial step helps you develop a mental map of the data, laying the groundwork for the following stages of analysis.
Step 3: Coding the Data
Coding is one of the most critical steps in ethnographic data analysis. This process involves assigning labels or codes to sections of your data that reflect key themes, concepts, or patterns. Coding allows you to break down large amounts of information into manageable pieces.
How to code ethnographic data effectively:
- Start with open coding, creating codes based on the data rather than pre-defined categories. For example, you might code interview excerpts with labels like “community dynamics” or “challenges in adoption.”
- Move to axial coding, where you group similar codes together into categories or themes. This step helps you identify relationships between the data.
- Finally, use selective coding to focus on the most relevant themes and insights that align with your research objectives.
If this sounds daunting, don’t worry—tools like Insight7 simplify the coding process with automated qualitative data analysis. The platform can generate codes and themes, saving time and ensuring consistency.
Step 4: Analyze Patterns and Relationships
Once you’ve coded the data, the next step is to analyze the patterns and relationships between different themes. This involves asking questions like:
- Are there recurring themes across different participants or contexts?
- How do the findings align with the cultural or social framework of the study?
- What outliers or exceptions exist, and what might they signify?
For example, if you’re analyzing ethnographic data from a workplace setting, you might notice themes like “team collaboration” or “communication challenges.” Exploring how these themes intersect can reveal deeper insights into organizational culture.
At this stage, many researchers rely on visualizations such as mind maps or thematic charts to identify connections. Insight7’s customizable insight deliverables feature can help you create compelling visuals for presentations or reports.
Step 5: Interpret the Findings
Interpreting ethnographic data is where the researcher translates raw insights into meaningful conclusions. This process requires you to consider the themes and patterns identified during coding and analysis within your research’s cultural and social context.
Key considerations during interpretation:
- Contextual meaning: Ask yourself, What do these behaviors, attitudes, or themes mean within the participants’ cultural framework?
- Divergent perspectives: Acknowledge and explore outliers or contradictory data points. They often reveal hidden insights or unique viewpoints.
- Research objectives: Relate your findings back to your research questions or objectives. For example, if your goal is to understand consumer buying behavior, ensure your interpretations align with this focus.
For instance, if you notice that participants in a study on rural healthcare frequently mention “distance to clinics” and “trust in community healers,” these findings highlight logistical and cultural factors affecting healthcare access. You can craft actionable recommendations by linking these insights to your study goals.
Step 6: Validate Your Analysis
Validation is a crucial step to ensure the credibility of your findings. Ethnographic data is often subjective, so cross-referencing and peer review can help confirm your interpretations.
Ways to validate your ethnographic analysis:
- Triangulation: Use multiple data sources (e.g., interviews, observations, and surveys) to confirm recurring themes.
- Member checking: Share your findings with participants to confirm accuracy and ensure their perspectives are represented correctly.
- Peer review: Collaborate with colleagues or experts to discuss your conclusions and refine them.
By validating your analysis, you ensure your insights are reliable and defensible.
Step 7: Present Your Findings
The final step in ethnographic data analysis is presenting your findings. Your presentation should effectively communicate the insights, themes, and patterns uncovered during your research and include actionable recommendations.
Key elements of presenting ethnographic findings:
- Narrative storytelling: Use vivid descriptions, quotes, or anecdotes from participants to bring your findings to life.
- Visualizations: Include thematic charts, word clouds, or infographics to help your audience quickly grasp key insights.
- Actionable insights: Tie your findings to clear recommendations or next steps.
Insight7’s comprehensive reporting feature is a game-changer here. The platform allows you to generate detailed, visually appealing reports tailored to your audience. Whether you’re presenting to stakeholders or academic peers, this feature ensures your findings make a lasting impact.
Applying Ethnographic Analysis Techniques in Fieldwork
Integrating Participant Observation Data
Analyzing Interviews and Narrative Data
Extract insights from interviews, calls, surveys and reviews for insights in minutes
Read: Best Free AI Data Analysis Tools in 2025
Expert Tips & Best Practices for Ethnographic Data Analysis
Mastering ethnographic data analysis requires more than just following steps—refining your approach to ensure accuracy, depth, and reliability. Here are some expert tips and best practices to help you excel:
1. Start with a Clear Research Question
Before data collection or analysis, ensure your research questions are well-defined. A focused question will guide your analysis and help you prioritize relevant themes and insights. For example, ask: How do cultural norms influence purchasing decisions? What barriers exist in rural communities to accessing healthcare?
2. Embrace Reflexivity
Ethnographic research is inherently subjective, so be aware of your biases and how they might influence your interpretation. Document your thought process and decisions throughout the analysis to maintain transparency and credibility.
3. Use Triangulation
To cross-validate your findings, combine multiple data sources—such as interviews, field notes, and photos. Triangulation enhances the reliability of your analysis and ensures no key insights are overlooked.
4. Stay Close to the Data
Avoid rushing to conclusions. Spend ample time reviewing and re-reviewing your data. For example, revisit interview transcripts to uncover subtle nuances or patterns you might have missed in earlier readings.
5. Leverage Technology
Modern tools like Insight7, NVivo, or MAXQDA can simplify time-consuming tasks like transcription, coding, and visualization. Using these tools lets you focus on interpreting the data rather than getting bogged down by logistics.
6. Use Quotes and Stories
Ethnographic research thrives on storytelling. Use direct quotes, anecdotes, or vivid participant descriptions to bring your findings to life. These narratives help your audience connect with the data on a human level.
7. Regularly Review with Peers
Collaborate with other researchers or team members to review your codes, themes, and interpretations. Peer feedback can challenge your assumptions and improve the quality of your analysis.
Conclusion: Mastering Ethnographic Analysis Techniques for Comprehensive Understanding
Analyze qualitative data. At Scale.
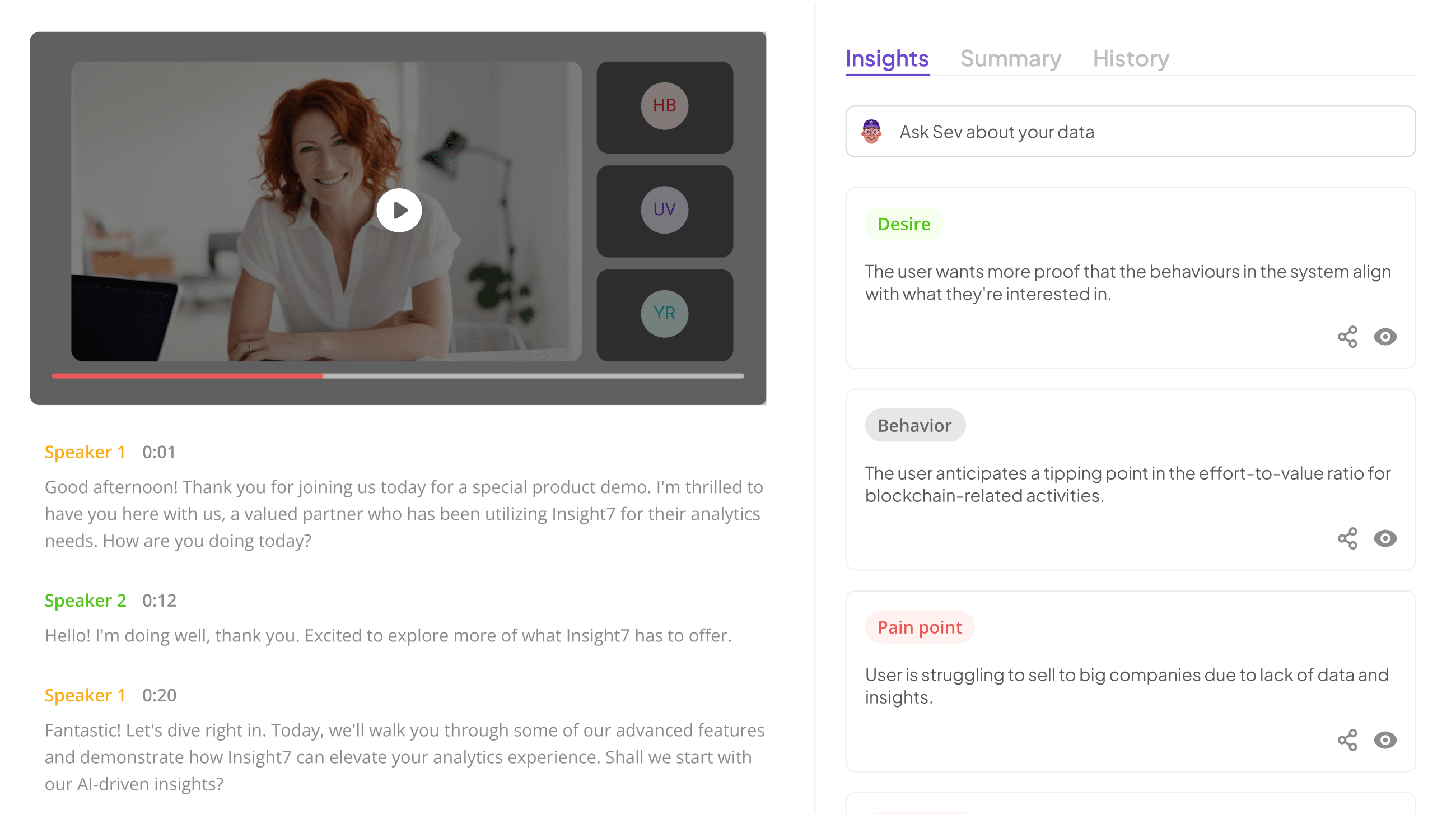