Onboarding Drop-Off Detection plays a critical role in understanding user experiences during the initial stages of engagement. As companies strive to enhance their onboarding processes, identifying early signs of user disengagement becomes essential. By detecting potential drop-off signals, organizations can implement timely interventions, ultimately improving user retention and satisfaction.
Analyzing interview transcripts allows for a detailed examination of user sentiments and behaviors that may indicate disinterest. This section will provide insights into recognizing key indicators, such as communication breakdowns and misalignment of expectations, and outline effective steps to analyze transcripts. Understanding these patterns will empower teams to create a more engaging onboarding experience, ensuring users feel supported and valued from the start.
Analyze qualitative data. At Scale.
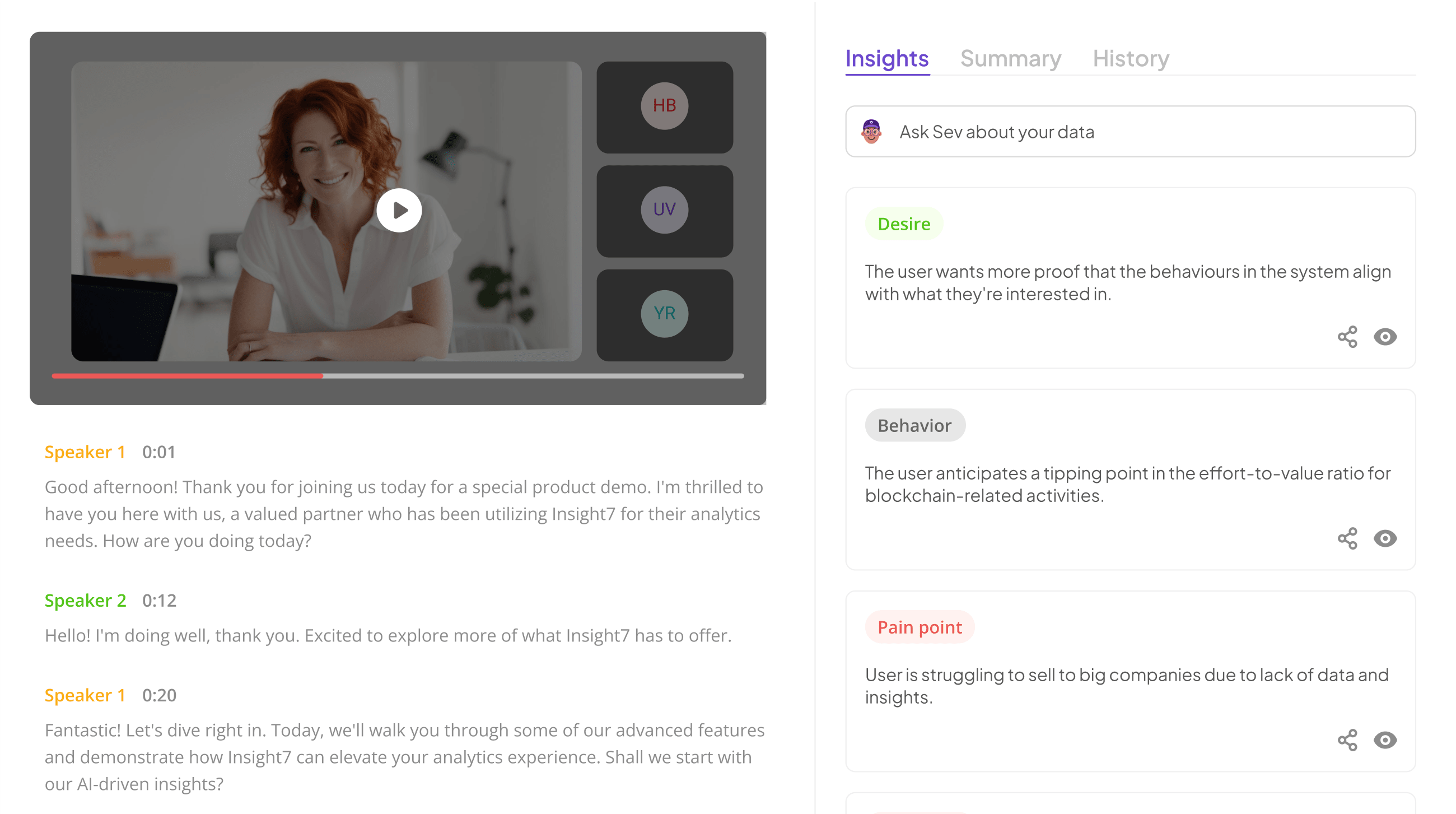
Understanding Onboarding Drop-Off Detection Through Interview Transcripts
Understanding onboarding drop-off detection through interview transcripts is crucial for enhancing the onboarding experience. By analyzing interview transcripts, organizations can uncover patterns and signals indicating where users might disengage during onboarding. This allows teams to proactively address concerns, aligning their offerings with user needs and expectations.
Key indicators of onboarding drop-off may include communication breakdowns or misalignments between user expectations and experiences. By closely examining interview transcripts, businesses can identify recurring themes and behaviors that signal potential drop-off points. This understanding not only strengthens the onboarding process but also fosters deeper relationships with users. Text analysis tools, such as Insight7 and others, can assist in efficiently extracting insights from transcripts. By following structured steps to analyze these interviews, organizations can transform qualitative feedback into actionable strategies to minimize drop-off rates, enhancing user satisfaction and retention.
Key Indicators of Onboarding Drop-Off Detection
Detecting drop-off signals during the onboarding process is crucial for retaining users. Key indicators of onboarding drop-off detection include communication breakdowns and misalignment of expectations. When users express confusion about the process or content, it is often a sign of inadequate communication. Analyzing interview transcripts can reveal these instances, allowing teams to address them promptly.
Misalignment of expectations occurs when users anticipate a different onboarding experience than what is delivered. Such discrepancies can lead to frustration and disengagement. Monitoring these key indicators helps identify risks early, enabling teams to enhance user experience. By focusing on these symptoms, organizations can implement changes that foster engagement and encourage completion of the onboarding process, ultimately reducing drop-off rates. Understanding these elements allows for a proactive approach in refining the onboarding journey, ensuring users receive a meaningful experience from the very start.
- Communication Breakdowns
Effective communication is vital in any onboarding process. However, communication breakdowns can occur, leading to confusion and disengagement among new users. These breakdowns often stem from unclear messaging or insufficiently documented insights from research. When project managers fail to communicate user needs effectively, the entire onboarding experience can be compromised, resulting in drop-offs.
To detect and address these communication issues, it is essential to focus on the following aspects. First, ensure all messaging is aligned with user expectations, which creates a seamless onboarding journey. Second, regularly document insights gathered during interviews, allowing insights to be shared effortlessly across teams. Third, leverage appropriate tools, such as Insight7, to analyze interview transcripts and identify key themes. By addressing these points, organizations can strengthen their onboarding drop-off detection strategies, fostering a more productive environment for users and project teams alike.
- Misalignment of Expectations
During the onboarding process, misalignment of expectations can lead to significant drop-off rates. This occurs when the assumptions of team members or users donโt align with the actual experiences they encounter. For example, a project manager might emphasize a specific feature without understanding usersโ core needs, leading to frustration and disengagement during onboarding. Such disconnects can be traced through interview transcripts, as they often reveal unmet expectations.
To mitigate these issues, itโs crucial to actively listen and document user feedback throughout the onboarding process. Consider the following aspects:
- Clarify Goals: Ensure all team members and users share a clear understanding of the project's objectives and user needs.
- Communicate Insights: Develop a structured approach to share insights gleaned from interviews to prevent misinterpretation or loss of information.
- Adjust Offerings: Gather feedback regularly, adjusting the onboarding experience to better align with usersโ expectations.
By addressing these areas, organizations can enhance onboarding drop-off detection and improve overall user engagement, ultimately fostering a more successful user experience.
Steps to Analyze Interview Transcripts for Early Signals
Analyzing interview transcripts for early signals requires a structured approach to effectively unveil potential onboarding drop-off indicators. Start by collecting and organizing the transcripts systematically. Ensure that these documents are easily accessible, as a well-structured dataset facilitates thorough analysis. You may want to include information such as participant demographics and their responses to open-ended questions for context.
Next, delve into identifying recurring patterns within the transcripts. Look for common phrases, themes, or concerns that may suggest friction points in the onboarding process. Finally, utilizing text analysis tools can significantly enhance your insights. These tools can help to quantify and visualize data, uncovering trends you might not readily see through manual inspection. By taking these steps, you can better detect early signals of potential drop-offs, ultimately refining the onboarding experience for users.
Step 1: Collect and Organize Transcripts
To effectively approach onboarding drop-off detection, the first critical step is to collect and organize transcripts from user interviews. Start by ensuring you have all relevant transcripts gathered from various outreach efforts. Consider categorizing them based on user experiences, feedback, or specific onboarding phases. This organized framework becomes essential as you dive deeper into understanding the underlying patterns and issues within the onboarding process.
Post-collection, align your transcripts in a way that facilitates easy access and analysis. Digital tools can assist in this endeavor, allowing quick retrieval and meaningful comparisons. Remember, a well-organized transcript repository aids significantly when sifting through user sentiments, enabling you to pinpoint initial drop-off signals effectively. As you prepare for the next steps in analyzing these transcripts, the organization becomes the backbone for insightful onboarding drop-off detection. This structured setup empowers you to make informed decisions based on user experiences, ultimately leading to smoother onboarding processes.
Step 2: Identify Recurring Patterns
Identifying recurring patterns in interviews is a critical aspect of onboarding drop-off detection. Start by analyzing the transcripts for consistent themes or phrases that emerge across different discussions. Look for patterns in user sentiments, frustrations, or unmet expectations that may indicate a likelihood of disengagement early in the onboarding process.
Focus on key concerns, such as misunderstandings related to the product's functionality or confusion about next steps. Document these findings meticulously as they provide insight into user experiences. Additionally, recognize that these patterns will serve as powerful indicators to fine-tune your onboarding strategies. By synthesizing this information, you can develop targeted interventions that anticipate and address user pain points, ultimately enhancing retention and reducing drop-off rates in the onboarding journey.
Step 3: Use Text Analysis Tools
Analyzing interview transcripts becomes significantly more effective when utilizing text analysis tools. These tools help streamline the process of detecting onboarding drop-off signals by organizing and interpreting large volumes of textual data. By applying such technologies, you can identify recurring themes and sentiments that indicate where users might feel confused or disengaged. This analytical phase is crucial for comprehending the deeper motivations behind user feedback.
To maximize the benefits of text analysis, consider employing tools like Insight7, which can efficiently evaluate data and summarize key insights from interviews. Other viable options include IBM SPSS Text Analytics for Surveys, MonkeyLearn, NVivo, and Google Cloud Natural Language API. Each of these platforms offers unique features that can provide comprehensive insights into user patterns, facilitating proactive interventions. Ultimately, successful onboarding drop-off detection hinges on the effective use of these tools to transform raw data into actionable insights.
Extract insights from interviews, calls, surveys and reviews for insights in minutes
Tools for Onboarding Drop-Off Detection
Implementing effective tools for onboarding drop-off detection can significantly enhance user retention. These tools help identify critical moments in the onboarding process where users face challenges, potentially leading to drop-offs. By using technology, organizations can analyze user behavior more effectively, allowing for targeted improvements.
A variety of tools are available to assist in onboarding drop-off detection. For qualitative insights, tools like Browsee provide session recordings that highlight usersโ struggles and successes during navigation. Additionally, Insight7 offers a robust platform for quantitative analysis, enabling the evaluation of data gathered from user interviews. Other options include IBM SPSS Text Analytics, MonkeyLearn, NVivo, and Google Cloud Natural Language API, all of which can help uncover trends and patterns in user feedback. Leveraging these tools creates a data-driven approach to understand user behavior and improve the onboarding experience. As a result, organizations can proactively address potential drop-off points and better support their users throughout the onboarding journey.
Using Technology to Enhance Onboarding Drop-Off Detection
Utilizing technology is vital for effective onboarding drop-off detection. Tools such as session recording software provide invaluable insights into user behavior during the onboarding process. These recordings allow companies to observe where users encounter challenges, helping them to pinpoint specific pain points. By continuously analyzing these interactions, organizations can make data-driven decisions to enhance the onboarding experience.
In addition to session recording tools, text analysis platforms can be invaluable. Solutions like Insight7 are designed to evaluate interview transcripts effectively, identifying recurring patterns that signal potential drop-off risks. Other technology options include IBM SPSS Text Analytics and NVivo, which help in processing and analyzing large volumes of qualitative data. By combining various technological solutions, organizations can proactively address issues, ensuring a seamless onboarding journey for every user. This holistic approach not only minimizes drop-offs but also fosters user trust and satisfaction.
- insight7: A comprehensive tool for identifying drop-off trends.
insight7 is designed to be an essential tool for identifying drop-off trends during onboarding processes. Through advanced data analysis capabilities, it helps organizations pinpoint areas where users may experience difficulties or disengage. By analyzing user behavior and feedback, insight7 enables onboarding teams to gain clear insights into user experiences, ensuring that they can address issues proactively.
One of the standout features of insight7 is its ability to analyze interview transcripts effectively. It highlights common pain points and recurring themes, which serve as critical indicators of potential drop-off signals. By leveraging this information, organizations can refine their onboarding strategies, ensuring a smoother experience for new users. Utilizing such a comprehensive tool not only aids in user retention but also fosters a deeper understanding of user needs, enhancing the overall onboarding experience.
- IBM SPSS Text Analytics for Surveys
IBM SPSS Text Analytics for Surveys provides valuable insights into understanding users' experiences during the onboarding process. This tool allows for the examination of interview transcripts by revealing patterns, sentiments, and themes that may signal early drop-off points. Effective analysis of these texts is crucial as it enables organizations to comprehensively evaluate what drives users away or keeps them engaged.
By employing this advanced text analytics system, organizations can analyze open-ended survey responses and interviews to uncover critical feedback. Subtle cues, such as communication breakdowns or misaligned expectations, may surface, providing actionable insights for improving the onboarding experience. The integration of text analytics not only aids in identifying potential drop-offs but also empowers teams to make data-driven decisions. Ultimately, IBM SPSS enables organizations to fine-tune their onboarding strategies, fostering an environment that supports user retention and satisfaction.
- MonkeyLearn
When detecting early drop-off signals in onboarding processes, employing advanced data analysis tools can greatly enhance insight extraction. Among these tools is a powerful platform designed to assist in identifying key trends and patterns in user interactions. By utilizing this technology, teams can efficiently analyze interview transcripts, pinpointing moments where users may feel disengaged or confused.
Harnessing text analysis features allows users to sift through large volumes of transcripts seamlessly. This process facilitates the recognition of recurring phrases or sentiments that indicate potential drop-off points. By understanding the language used by users, companies can better align their onboarding processes with expectations, ultimately leading to a more engaging experience. This method not only aids in detecting onboarding drop-off signals but also contributes to refining overall user experiences and improving retention rates.
- NVivo
NVivo is a robust tool designed to assist researchers in analyzing qualitative data, making it invaluable for onboarding drop-off detection. By utilizing NVivo, you can systematically organize and code interview transcripts, allowing for an in-depth analysis of user experiences during the onboarding process. This capability is particularly crucial for identifying recurring challenges or frustrations that may lead to drop-off.
In the context of onboarding, NVivo helps facilitate the extraction of meaningful insights from user interviews. It allows analysts to highlight specific phrases or themes that signal potential issues, such as communication breakdowns or misalignment of expectations. By focusing on these aspects, organizations can implement targeted strategies to enhance the onboarding experience and improve user retention. Utilizing NVivo ensures a thorough evaluation of qualitative data, ultimately leading to better-informed decisions and strategies for reducing onboarding drop-off rates.
- Google Cloud Natural Language API
The Google Cloud Natural Language API offers powerful text analysis capabilities that can significantly enhance onboarding drop-off detection. This tool utilizes natural language processing to extract insights from interview transcripts. It allows you to analyze sentiment, understand entities, and identify key phrases, which can help in pinpointing areas where users may feel lost or disengaged.
By integrating this API into your onboarding analysis process, you can efficiently uncover communication breakdowns and misalignments in expectations. The API provides real-time insights that make it easier to identify and address potential drop-off signals early. As a result, youโll be equipped with actionable data that informs your strategies for enhancing the onboarding experience. Ultimately, using the Google Cloud Natural Language API may lead to a more effective onboarding process by proactively addressing the issues that lead to user drop-off.
Conclusion on Effective Onboarding Drop-Off Detection
Effective Onboarding Drop-Off Detection is essential for enhancing user retention and product usage. By closely analyzing interview transcripts, organizations can identify early signals indicating potential drop-offs. Key insights from user behavior reveal communication breakdowns and misalignment of expectations, which often lead to disengagement. Understanding these patterns allows companies to adjust their onboarding processes to better meet user needs and foster a more supportive experience.
Moreover, utilizing tools like Insight7 for quantitative analysis can streamline the identification of these drop-off signs. It is crucial to shift focus from mere data collection to actionable insights that can improve user experiences over time. Therefore, integrating findings from user interviews into onboarding strategies is vital to minimize drop-off rates and maximize user satisfaction.
Analyze qualitative data. At Scale.
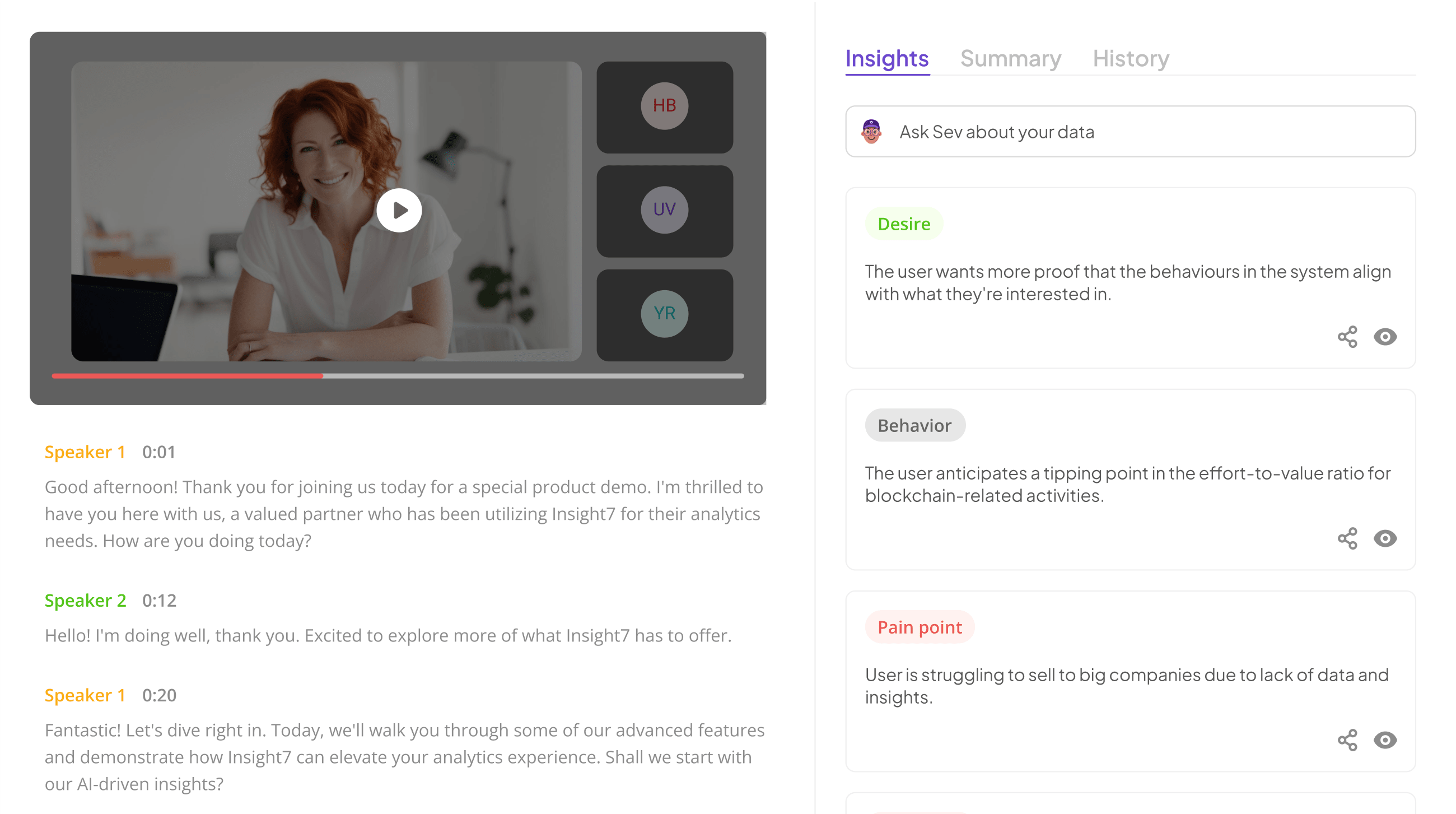