Bias in Research Interpretation: How to Mitigate It
-
Hello Insight
- 10 min read
Interpretation bias mitigation in research is essential for maintaining integrity and validity in findings. As researchers analyze data, personal beliefs and subjective interpretations can cloud judgment, leading to skewed results. By recognizing and addressing these biases early, researchers can improve the quality of their interpretations and ensure more reliable outcomes.
Bias in research interpretation affects how data is understood and communicated. Various strategies can help mitigate this bias, including fostering diverse research teams and incorporating peer reviews. Implementing these measures enhances transparency and encourages a more accurate understanding of the research subject, ultimately benefiting the entire research process.
Analyze & Evaluate Calls. At Scale.
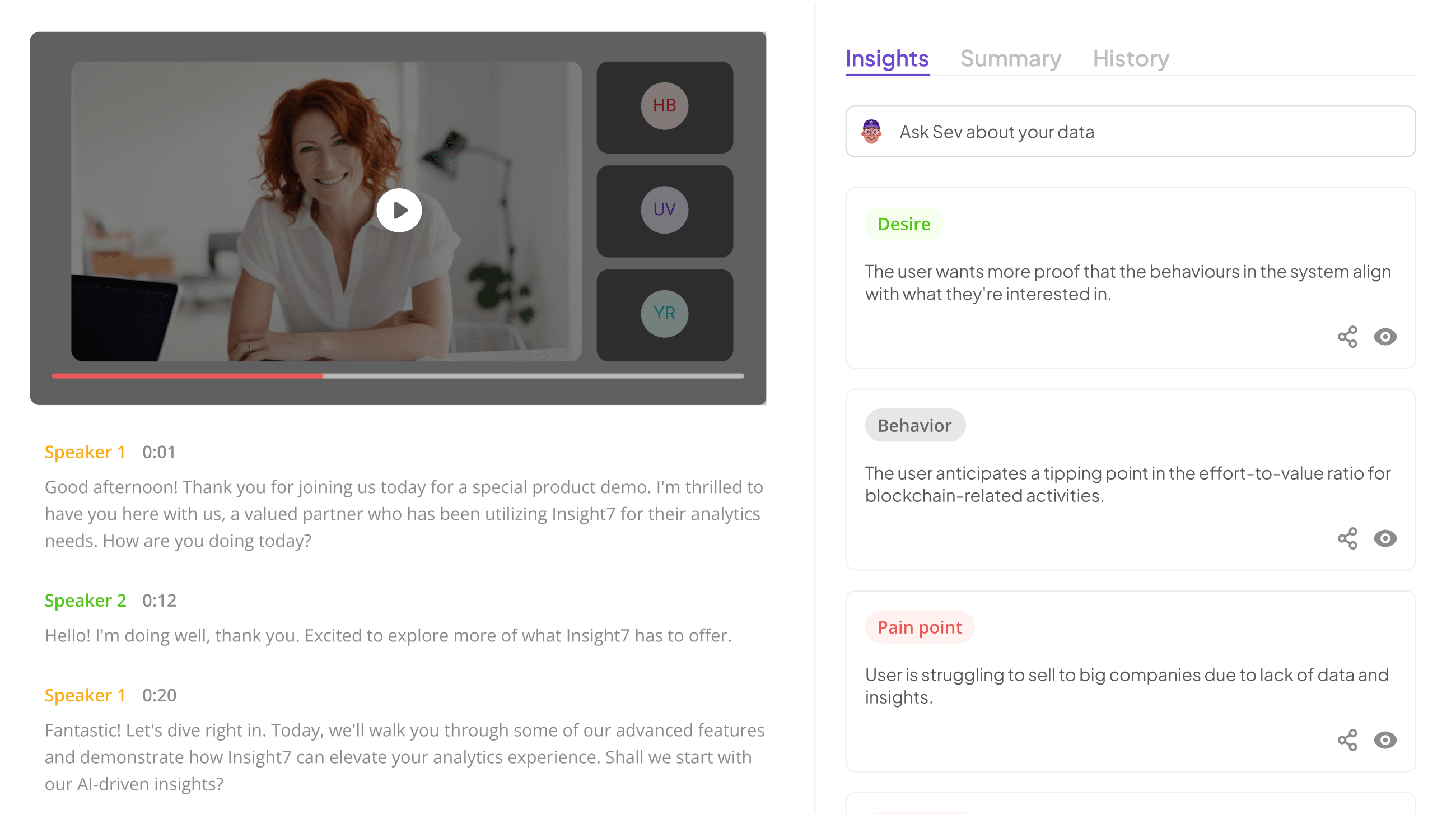
Understanding the Impact of Interpretation Bias in Research
Interpretation bias can significantly affect research outcomes, leading to misleading conclusions. Researchers often bring their own beliefs and experiences into their work, which can skew how they analyze data. For example, confirmation bias occurs when researchers prioritize information that supports their existing views, disregarding conflicting evidence. This type of bias can mislead stakeholders and distort the understanding of the research findings.
To address these challenges, it is essential to implement strategies for interpretation bias mitigation. Engaging diverse research teams can offer varying perspectives that counteract personal biases. Additionally, incorporating rigorous peer review processes allows for constructive feedback, which can enhance the integrity of the findings. By actively acknowledging the potential for bias and employing a thorough critique process, researchers can produce results that are more reliable and accurate. Ultimately, addressing interpretation bias within research is crucial to maintaining credibility and fostering trust in the findings.
Common Types of Interpretation Bias in Research
Interpretation bias in research can significantly influence findings. One common type is confirmation bias, which occurs when researchers favor information that supports their existing beliefs. This tendency can lead to selective data usage, ultimately skewing conclusions. Another prevalent form is publication bias, where studies with positive results are more likely to be published than those yielding negative or inconclusive outcomes. This imbalance can distort the scientific record, making certain findings seem more robust than they genuinely are.
Recognizing these biases is imperative for effective interpretation bias mitigation. By being aware of these patterns, researchers can take proactive measures to minimize their effects. Addressing these biases not only improves the integrity of research but also fosters a more nuanced understanding of data. Cultivating a critical mindset and employing diverse methodologies can help create a more balanced representation of findings, ultimately enhancing the quality and reliability of research interpretations.
- Confirmation Bias
Confirmation bias presents a significant challenge in the interpretation of research findings. This bias leads individuals to favor information that confirms their preexisting beliefs while disregarding contradictory evidence. Consequently, researchers might selectively interpret data in ways that align with their hypotheses, undermining the integrity of their conclusions. Understanding this bias is essential to conducting trustworthy research.
To mitigate confirmation bias, researchers should adopt several strategies. First, acknowledging the presence of bias is crucial in fostering objectivity. Next, employing diverse research teams can introduce various perspectives, reducing the likelihood of biased interpretation. Finally, incorporating peer reviews allows for critical evaluation, ensuring that multiple viewpoints are considered in the analysis. By focusing on these strategies, researchers can better navigate the complexities of confirmation bias, ultimately enhancing the reliability of their findings.
- Publication Bias
Publication bias occurs when the outcomes of research studies influence the likelihood of their publication. Often, studies that report positive or significant results are more likely to be published than those that yield neutral or negative findings. This selective publication can distort the overall understanding of a research topic, leading to exaggerated perceptions of effectiveness or importance.
Mitigating interpretation bias requires transparent reporting and a commitment to publishing all research findings, regardless of their nature. Researchers and journals should prioritize the dissemination of negative or inconclusive results through dedicated platforms or registers. By ensuring comprehensive representation of research outcomes, the scientific community can foster more robust interpretation and applications of findings. Investing in these practices enhances the credibility of research and supports more informed decision-making processes in various fields.
Real-world Examples of Interpretation Bias
In the realm of research, interpretation bias can significantly skew results, leading to flawed conclusions. A prime example is the misinterpretation of clinical trials. When data from trials are viewed through the lens of pre-existing beliefs, outcomes may be overstated or understated, affecting treatment protocols. For instance, if a pharmaceutical company emphasizes positive results while downplaying adverse effects, it can mislead stakeholders about a drug's efficacy. This highlights the necessity for robust interpretation bias mitigation strategies to ensure findings are accurate and trustworthy.
Another illustrative case is the bias in media reporting on scientific studies. When journalists cherry-pick data or sensationalize findings, the public may draw erroneous conclusions. For example, a study linking diet to health issues may be presented in a way that supports specific dietary trends, neglecting comprehensive context. Such reporting compromises the integrity of research and its applications. By recognizing these real-world issues, researchers can better employ strategies to mitigate interpretation bias, thereby fostering more reliable and balanced insights in their work.
Interpretation Bias Mitigation: Strategies and Tools
Interpretation Bias Mitigation is essential in ensuring the integrity of research findings. To effectively reduce biases, a structured approach can be beneficial. First, acknowledging the presence of bias is crucial; researchers should reflect on their perspectives and potential preconceived notions that may influence interpretations. This transparency not only enhances the validity of the research but also promotes a culture of openness.
Next, employing diverse research teams can significantly improve the richness of interpretations. Diverse perspectives enable the exploration of various angles, reducing the risk of a singular worldview shaping the findings. Lastly, utilizing peer review and critiques helps uncover hidden biases, ensuring that interpretations withstand scrutiny. Tools such as insight7, Nvivo, Dedoose, MAXQDA, and Atlas.ti can facilitate this process by providing frameworks for qualitative analysis, thus supporting researchers in their efforts toward Interpretation Bias Mitigation. This comprehensive view not only aids in better decision-making but also fosters trust in research outcomes.
Extract insights from interviews, calls, surveys and reviews for insights in minutes
Interpretation Bias Mitigation: Best Practices
To effectively address interpretation bias, it's crucial to implement solid best practices that support interpretation bias mitigation within research. First, acknowledging the presence of bias is vital. Researchers must recognize their own biases and how they may affect findings and conclusions. This awareness fosters transparency and better decision-making throughout the research process.
Next, employing diverse research teams can significantly contribute to minimizing bias. When individuals from different backgrounds, experiences, and expertise collaborate, their varied perspectives enhance the quality of interpretation and analysis. Lastly, utilizing peer review processes ensures that research findings are evaluated by independent experts, who can challenge assumptions and provide critical feedback. These steps create a more robust framework for interpretation bias mitigation, ultimately leading to more reliable and credible research outcomes. By systematically incorporating these strategies, researchers can significantly reduce the impact of bias on their interpretations.
- Step 1: Acknowledge Bias
Recognizing bias is the crucial starting point in the journey toward interpretation bias mitigation. Every researcher brings personal experiences and beliefs that can subtly influence how they interpret data. This influence can lead to skewed results, undermining the integrity of the research process. Acknowledging these biases requires a courageous and honest evaluation of one’s perspectives, as well as how they might shape findings. Understanding one’s own biases is not merely an academic exercise; it is an essential practice for producing credible, objective research outcomes.
Furthermore, recognizing bias sets the foundation for creating a more systematic approach to interpretation. It encourages researchers to challenge their viewpoints and seek contrary evidence, which can help minimize the risk of confirmation bias. This proactive stance paves the way for employing diverse research teams and other strategies, creating a more balanced discussion around the data. Ultimately, acknowledging bias allows researchers to engage in a more reflective and thorough analysis, resulting in more trustworthy conclusions.
- Step 2: Employ Diverse Research Teams
Diverse research teams are crucial to mitigating interpretation bias, as they bring varied perspectives and experiences to the table. By assembling teams that include individuals from different backgrounds, genetic heritages, genders, and disciplines, researchers can challenge prevailing assumptions and minimize cognitive biases. This diversity fuels richer discussions, resulting in more balanced interpretations of research findings.
To employ diverse research teams effectively, organizations should prioritize inclusive hiring practices and foster an environment where contributions from all members are valued. Team members should also be encouraged to express differing viewpoints, as this can lead to more thorough vetting of ideas and conclusions. Moreover, conducting regular training on bias awareness can further enhance the team's ability to recognize and address interpretation bias. Embracing diverse perspectives will ultimately enhance research quality and integrity, making the findings more robust and applicable across various contexts.
- Step 3: Utilize Peer Review and Critique
Engaging in peer review and critique is a crucial strategy in the journey of interpretation bias mitigation. This process invites fellow researchers to examine your work, offering insights that may illuminate biases you may have overlooked. When colleagues analyze your data and interpretations, they can provide diverse perspectives and highlight potential pitfalls in reasoning that you might not have recognized on your own.
To maximize the benefits of peer review, consider implementing a structured approach. Start by selecting reviewers who possess expertise in relevant areas but also come from varied academic backgrounds. This diversity in viewpoints can significantly enhance the critique process. Secondly, create an open environment where constructive feedback is welcomed and encouraged. Finally, be willing to revise your work based on the critique received, which promotes accuracy and thoroughness in your research. Such diligence not only enhances the robustness of your findings but also cultivates a culture of transparency and integrity in research.
Tools for Interpretation Bias Mitigation
To effectively address interpretation bias in research, it’s crucial to utilize various tools designed for interpretation bias mitigation. These tools serve to streamline data analysis, enhance collaboration, and encourage critical thinking. For instance, software like insight7 allows researchers to manage vast amounts of data efficiently, turning interviews and customer conversations into actionable insights.
Additionally, tools such as NVivo and MAXQDA facilitate qualitative analysis by enabling researchers to categorize and interpret data systematically. Dedoose and Atlas.ti similarly help in organizing research findings, making collaboration more efficient among diverse teams. Integrating various platforms can significantly minimize the risks associated with personal bias, ensuring that results reflect true data rather than personal interpretations. By embracing these innovative solutions, researchers can foster a more balanced and objective approach when interpreting findings, ultimately leading to better decision-making and outcomes.
- insight7
Facing bias in research interpretation is an increasingly critical issue. By recognizing its existence, researchers can adopt practical strategies for interpretation bias mitigation. A systematic approach can include acknowledging personal biases, employing diverse research teams, and promoting peer review processes. Each of these steps helps create clarity and aids in the accurate analysis of data.
To further detail this approach, starting with awareness is essential. When researchers actively acknowledge their biases, it opens the door to more objective analysis. Next, employing diverse teams not only enhances various perspectives but also enriches discussions, leading to a more balanced interpretation of findings. Lastly, utilizing peer review fosters accountability, ensuring that conclusions drawn from research are scrutinized before being finalized. The commitment to interpretation bias mitigation is vital for producing reliable, actionable insights that can positively impact decision-making processes.
- Tool 2: Nvivo
Nvivo serves as a robust tool for researchers aiming to mitigate interpretation bias. This software provides a structured environment for organizing qualitative data, enhancing the clarity of insights derived from research. By allowing users to code and categorize information, Nvivo enables researchers to spot trends and patterns in data, reducing subjective interpretations that can skew results.
To maximize the benefits of Nvivo in interpretation bias mitigation, researchers can follow several key steps. First, they should import diverse data sources, including transcripts and audio recordings, allowing comprehensive analysis. Next, employing the software's matrix feature helps in analyzing responses systematically, providing clear summaries that identify customer sentiments effectively. Lastly, collaborative features enable feedback from peers, fostering an environment of shared insights and critical discussions, ultimately leading to more balanced interpretations. By integrating these features, Nvivo helps safeguard against the pitfalls of bias in research interpretation.
- Tool 3: Dedoose
Dedoose serves as a powerful platform to support interpretation bias mitigation in research. Its user-friendly interface allows researchers to seamlessly analyze qualitative and quantitative data without requiring extensive training or expertise. By democratizing access to insights, Dedoose enables teams to engage more fully with their datasets, which helps identify patterns and biases that may otherwise go unnoticed.
One of the key benefits of Dedoose is its ability to visualize conversations and extract meaningful insights from customer feedback. Users can analyze multiple calls at once, highlighting pain points, desires, and behavioral trends. This functionality ensures that researchers can make data-informed decisions that reflect a comprehensive understanding of participant perspectives. Furthermore, Dedoose promotes collaboration among team members, fostering an environment where diverse viewpoints can collectively contribute to lowering interpretation bias in research. By harnessing its capabilities, researchers can work towards a more accurate and nuanced understanding of data-driven insights.
- Tool 4: MAXQDA
MAXQDA serves as a powerful tool for researchers striving to address interpretation bias in their work. By organizing and analyzing qualitative data, researchers can gain clearer insights that reduce bias. The software allows for easy integration of various data types, transforming chaotic information into coherent projects that highlight patterns and trends.
To effectively mitigate interpretation bias, researchers can utilize MAXQDA’s features in several key ways. First, it enables comprehensive data organization, allowing for streamlined access to critical information. Second, the matrix analysis feature helps identify recurring themes in qualitative feedback. Finally, the visualization tools aid in understanding complex data relationships, empowering researchers to draw more reliable conclusions. Through these functionalities, MAXQDA fosters an environment conducive to unbiased research interpretation.
- Tool 5: Atlas.ti
Atlas.ti offers a robust framework for managing qualitative data, making it an excellent tool for addressing interpretation bias. By enabling researchers to organize, analyze, and visualize data systematically, this software fosters a deeper understanding of complex narratives within the information. Utilization of Atlas.ti can significantly contribute to interpretation bias mitigation by facilitating a structured approach to data analysis, ensuring that insights are drawn from a comprehensive and diverse set of data points.
In practice, Atlas.ti allows researchers to integrate various types of data, including interview transcripts and audio recordings. The platform supports intuitive organization through projects, enhancing the ability to explore different themes and patterns. These features empower research teams to collaboratively analyze findings and encourage rigorous questioning of interpretations. Consequently, by leveraging its organizational capabilities, researchers can reduce the likelihood of subjective biases influencing their conclusions, ultimately leading to more reliable and transparent research outcomes.
Conclusion: The Path Forward for Interpretation Bias Mitigation
The path forward for interpretation bias mitigation requires a committed effort toward transparency and collaboration in research. Acknowledging existing biases is crucial, as it enables researchers to develop strategies that promote objective findings. Engaging diverse research teams can enhance perspectives, leading to more comprehensive evaluations.
Additionally, employing peer review processes fosters accountability and enriches insights through constructive criticism. By prioritizing these inclusive practices, we can create a more equitable research environment that minimizes interpretation bias, ultimately resulting in richer, more reliable insights across various fields. Addressing these biases is essential for advancing research integrity and fostering trust among stakeholders.