AI vs Human QA Scoring has emerged as a vital topic in today's data-driven world, where organizations strive for accuracy and efficiency. As technology advances, the debate over the effectiveness of AI in quality assurance versus traditional human scoring continues to gain prominence. Both methodologies offer distinct advantages, making it crucial for teams to understand their differences and implications.
In this section, we will explore the evolving landscape of QA scoring, highlighting how AI tools are transforming this critical aspect of evaluation. While AI provides speed and scalability, human reviewers add a layer of intuition and contextual understanding that machines currently cannot replicate. Ultimately, blending these approaches may lead to a more robust and reliable quality assurance process.
Analyze qualitative data. At Scale.
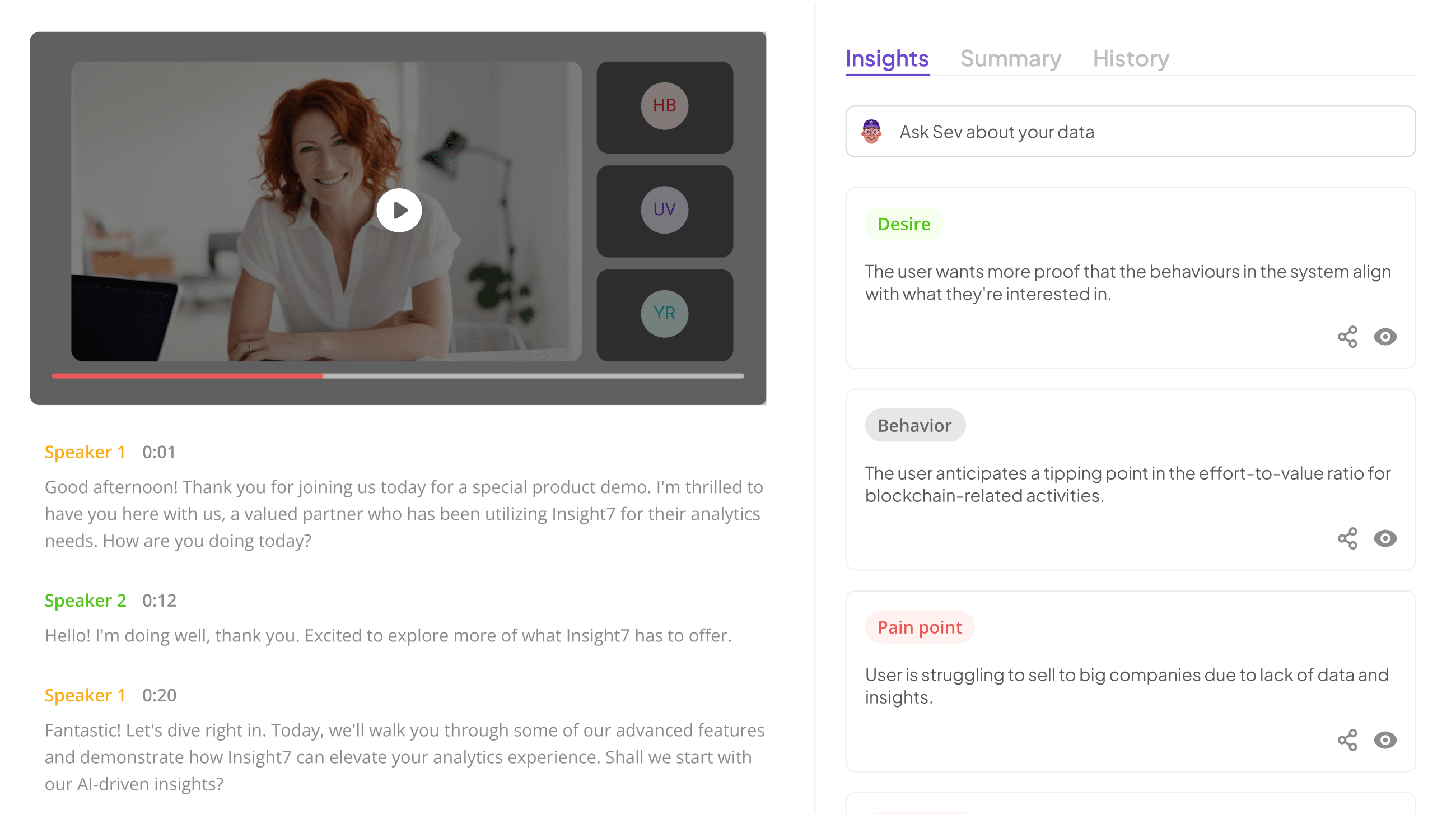
Understanding the Basics of AI vs Human Scoring
Understanding the basics of AI vs Human scoring entails delving into the characteristics that define each approach. AI scoring relies on algorithms and machine learning models to analyze data quickly and efficiently, making it ideal for large-scale assessments. However, while AI can handle vast amounts of data and provide consistency, it occasionally lacks the nuanced understanding that human evaluators naturally possess.
On the other hand, human scoring brings empathy, contextual awareness, and ethical considerations to the table, which are often crucial in quality assessments. Humans can interpret subtleties in tone, intent, and emotional cues that might escape an algorithm. For optimal results, recognizing the strengths and limitations of both AI vs Human scoring is essential. This dual approach allows organizations to harness the speed and efficiency of AI while still benefiting from the human touch in judgment and assessment.
The Rise of AI in Quality Assurance
AI is rapidly transforming the quality assurance (QA) landscape, providing new avenues for efficiency and accuracy. In the competition of AI vs Human scoring, AI technologies are increasingly capable of analyzing vast datasets with precision. This advancement is attracting businesses seeking to boost their QA processes and enhance overall productivity. Particularly in repetitive tasks, AI can significantly reduce processing time while maintaining consistency in evaluations.
The rise of AI in QA is also fueled by its adaptability and machine learning capabilities. As these systems improve, they can learn from past mistakes and refine their algorithms. However, understanding when to rely on AI or maintain human oversight is crucial. Human judgment remains invaluable, particularly in complex scenarios requiring empathy, contextual understanding, or nuanced decision-making that AI may not capture fully. Thus, brands are exploring effective integrations of AI with human QA efforts to maximize results and improve service quality.
- Define how AI is transforming quality assurance.
AI is transforming quality assurance by introducing unprecedented levels of efficiency and accuracy. Through advanced algorithms and machine learning, AI can analyze vast amounts of data swiftly, identifying patterns and anomalies recognized by human QA scorers. This shift enables organizations to perform continuous quality checks, increasing the reliability of outputs while reducing human error.
Moreover, AI can deliver more objective insights than human scorers, who may inadvertently be influenced by biases. Nonetheless, the role of human judgment remains essential, particularly in nuanced scenarios requiring emotional intelligence or contextual understanding. Understanding the balance between AI and human scoring is critical in this evolving landscape. Organizations must recognize how AI vs Human scoring can be structured to optimize their quality assurance processes while retaining the irreplaceable human touch where needed.
- Discuss the reasons for AIs growing presence in QA scoring.
The increasing presence of AI in quality assurance (QA) scoring can be attributed to several key factors. First, AI enhances efficiency by processing large volumes of data at remarkable speeds. This capability allows organizations to receive timely insights that are critical for performance evaluations. Second, AI systems are not only consistent but also minimize the subjectivity often seen in human evaluations. They are capable of identifying patterns that may go unnoticed, providing a data-driven approach that enhances the overall scoring accuracy.
Furthermore, AI's ability to adapt continuously through machine learning contributes to its growing relevance. As AI learns from new data, its scoring methods improve, helping organizations to refine their quality assessments. This evolving technology complements human judgment, leading to a balanced approach in AI vs Human scoring. In scenarios where emotional intelligence, contextual understanding, and intricate sets of criteria are vital, human insight remains invaluable. However, leveraging AI's strengths allows for a more comprehensive and efficient QA scoring system.
Human Element in QA Scoring: Why It Still Matters
In the evolving discourse of AI vs Human QA scoring, understanding the human element remains essential. Human judgment brings unique qualities such as empathy and intuition, which are difficult for AI to replicate. These traits enable nuanced evaluations that consider context, emotions, and customer experiences. For instance, in cases of complex interactions or sensitive situations, a human scorer can analyze subtleties that AI might overlook, ensuring a more comprehensive understanding.
Moreover, there are specific scenarios where human oversight is critical. When evaluating customer service interactions, the ability to perceive the emotional tone and respond accordingly can influence the scoring. Humans can assess not just what is said but also how it is said, capturing the essence of customer interactions. Therefore, while AI plays a significant role in streamlining processes and data handling, the human element in QA scoring remains invaluable and irreplaceable. Balancing both approaches enhances quality assurance efforts.
- Explore the unique qualities humans bring to QA scoring.
Human judgment offers remarkable qualities in the realm of QA scoring that AI has yet to replicate. The nuances of empathy, experience, and contextual understanding allow humans to interpret nuances in customer interactions, which machines may overlook. For example, a human scorer can discern the emotional tone of a conversation, a subtlety essential for gauging customer satisfaction, ensuring that the nuances of communication are accurately captured.
Moreover, human scorers bring critical thinking and adaptive problem-solving to the evaluation process. They can objectively assess the effectiveness of responses against evolving criteria, which is vital in industries where standards continually shift. While AI excels at processing large volumes of data, the human touch remains indispensable for nuanced evaluations. This interplay of strengths illustrates why combining AI and human scoring could lead to the most effective quality assurance practices. By integrating the unique qualities of humans with the efficiency of AI, companies can achieve a more holistic approach to QA scoring.
- Discuss scenarios where human judgment is crucial.
In the discussion of scenarios where human judgment is crucial, it becomes clear that certain situations demand the nuanced understanding and empathy only humans can provide. For instance, when assessing customer interactions, human scorers can recognize subtle tones, emotions, and context that AI may overlook. Human judgment plays an essential role in handling complex situations where the stakes are high, such as disputes or delicate customer concerns.
Moreover, AI vs Human scoring becomes particularly significant in creative evaluations. While AI can analyze data and trends, the subjective nature of creativity often requires a human touch. Individuals can offer unique insights based on personal experiences or cultural contexts. This interaction fosters a quality of evaluation that transcends mere data points, ensuring that the evaluations are both accurate and contextually relevant. Human scorers, therefore, complement AI's strengths, enhancing the overall quality assurance process, especially in intricate or emotionally charged scenarios.
Extract insights from interviews, calls, surveys and reviews for insights in minutes
AI vs Human Scoring: Comparative Analysis
In the analysis of AI vs Human Scoring, it is essential to evaluate both methodologies' unique advantages and limitations. AI scoring leverages data-driven algorithms, resulting in a faster and often consistent evaluation of performance metrics. This efficiency can be beneficial for organizations processing large volumes of data, as it provides a comprehensive overview in a shorter timeframe. However, AI lacks the nuanced understanding and contextual interpretation that human scorers possess, especially in complex situations.
Human scoring, on the other hand, brings invaluable expertise and empathy to quality assurance. The ability to read between the lines, understand emotional cues, and offer tailored feedback is something AI cannot replicate. Nonetheless, human scorers may introduce inconsistencies, influenced by subjective biases or fatigue. Therefore, a hybrid approach that combines the strengths of both AI and human scoring can ensure a more effective QA process. Embracing this balance allows organizations to harness automation's efficiency while maintaining the essential human touch in evaluations.
Making Sense of AI vs Human Scoring: Advantages and Limitations
AI vs Human Scoring presents a fascinating juxtaposition in quality assurance processes. The advantages of AI include speed, consistency, and the ability to analyze vast amounts of data in real-time. AI tools can quickly identify patterns and anomalies, enabling instant feedback without fatigue. However, they sometimes lack the nuanced understanding that human scorers can provide, particularly in complex or emotionally charged scenarios.
On the other hand, human scorers bring empathy, context, and judgment to the assessment process. They excel in interpreting subtleties that AI may overlook, making their insights invaluable in situations requiring a deeper understanding. Yet, human scoring can be time-consuming and prone to biases, potentially affecting consistency.
Therefore, seeking a balance between AI and human scoring is crucial. Ultimately, leveraging the strengths of both can enhance the quality and effectiveness of QA scoring, creating a more robust evaluation process.
- Compare the benefits and drawbacks of AI in QA scoring.
AI in QA scoring offers several benefits and drawbacks, crucial for understanding the balance between AI vs Human scoring. One primary benefit is efficiency; AI can analyze vast amounts of data quickly, providing rapid insights that would take humans significantly longer. Additionally, AI systems can maintain consistent quality evaluations, reducing the risk of human error. However, AI lacks the critical thinking and contextual understanding that human scorers possess. This limitation may affect the quality of assessments during complex scenarios requiring subjective judgment.
On the downside, AI can struggle with nuances such as tone and emotion, which humans naturally interpret. Moreover, reliance on AI may lead to complacency among human evaluators, lowering their engagement and expertise. Balancing the strengths of AI’s speed and consistency with human evaluators' nuanced understanding remains pivotal to optimizing QA scoring. Hence, integrating both approaches can yield a more comprehensive evaluation strategy, taking advantage of AI capabilities while retaining human insights.
- Highlight the strengths and weaknesses of human scorers.
Human scorers bring a nuanced perspective to quality assurance that AI often struggles to replicate. Their ability to understand context, emotional nuances, and complex scenarios allows for deeper evaluations. This human touch is invaluable when assessing customer interactions, where empathy and critical thinking play key roles. Furthermore, human scorers can adapt to changing criteria and expectations dynamically, providing flexibility that AI might not achieve.
However, there are also notable weaknesses. Human scorers can be influenced by fatigue, bias, or inconsistent standards, which could impact scoring reliability. Additionally, the time taken for human evaluations can extend project timelines compared to the speed of AI processing. Therefore, organizations must weigh the strengths of human scorers against these weaknesses. Finding the balance between AI and human scoring methodologies is essential in optimizing quality assurance processes.
Steps to Integrate AI with Human QA Scoring
To effectively integrate AI with human QA scoring, a structured approach is essential. First, assess your current QA scoring process to identify strengths and weaknesses. This evaluation forms the foundation for integrating AI technologies. Understanding how your teams operate will help in determining the most suitable areas for enhancement.
Next, identify specific areas where AI can complement human efforts. This might involve automating data collection or scoring certain metrics while leaving subjective assessments to human evaluators. Implementing a hybrid model allows for the strengths of both AI and human scoring to be utilized effectively. It is vital to continuously evaluate and refine this integration to ensure optimum performance. Regular feedback loops will help address any challenges that arise, leading to improved outcomes and experiences in your quality assurance processes. By taking these steps, organizations can find a harmonious balance in the debate of AI vs Human QA scoring.
- Step 1: Assess your current QA scoring process.
To begin the assessment of your current QA scoring process, start by reviewing what criteria you’re currently using. Consider both the qualitative and quantitative aspects of the calls or interactions being scored. For instance, look at how you evaluate smoothness in conversations or how specific keywords are being addressed. By identifying these criteria, you can lay a solid foundation for understanding the effectiveness of your existing scoring methods.
Next, it’s essential to compare human judgments to AI-driven assessments. Analyze discrepancies in scores and determine where human intuition provides insight that AI might miss. Documenting these findings can illuminate areas of strength in human scoring, as well as potential gaps where AI can contribute. This step will set the stage for exploring how to integrate AI into your current process, combining the strengths of both AI and human scoring for optimal outcomes.
- Step 2: Identify areas where AI can enhance human effort.
Identifying areas where AI can enhance human effort is pivotal in optimizing QA scoring. In the evolving landscape of AI vs human scoring, it's essential to recognize specific tasks where artificial intelligence can provide significant support. For instance, AI excels in processing vast amounts of data quickly, enabling faster assessments and consistent scoring standards. By automating repetitive tasks, human scorers can devote more time to complex decision-making and nuanced evaluations, which require human insight and context.
Moreover, AI can augment human efforts by offering analytical insights that may go unnoticed. Scoring patterns detected through AI can identify strengths and weaknesses in performance, allowing for more targeted training and support. This collaborative approach not only improves overall efficiency but also enhances the accuracy of evaluations. Thus, leveraging AI effectively can lead to a more balanced and insightful scoring process, making the integration of technology alongside human judgment truly transformative in QA settings.
- Step 3: Implement a hybrid model for QA scoring.
Implementing a hybrid model for QA scoring combines the strengths of both AI and human evaluation. Begin by defining clear criteria for assessment, which will guide both AI algorithms and human reviewers in their evaluations. AI can efficiently analyze vast amounts of data, identifying patterns and flagging potential issues. However, it is the nuanced understanding of human scorers that enriches this process, allowing for context-based judgments that AI may overlook.
Once the criteria are established, integrate AI tools that can assist human evaluators. For example, AI can handle routine checks and preliminary assessments, freeing up human scorers to focus on more complex evaluations. Continuous feedback loops between AI outputs and human insights help refine both the algorithms and the scoring process over time. This synergy enhances the reliability and accuracy of QA scoring, ensuring a balanced approach in the dynamic interplay of AI vs Human Scoring.
- Step 4: Continuously evaluate and refine the integration.
To ensure effective integration between AI and human QA scoring, continuous evaluation and refinement are essential. This process begins by assessing how both elements interact within your system and determining their collective effectiveness. Regular feedback loops can help identify strengths and weaknesses within the scoring framework. For instance, analyzing the discrepancies between AI-generated insights and human assessments can reveal areas requiring adjustment to harmonize both methods.
Moreover, the continuous evaluation should encompass data trends, user satisfaction, and overall scoring accuracy. This dynamic approach ensures that as both AI and human scorers evolve, their integration improves in quality and reliability. As practices and standards shift, refining the integration might lead to better outcomes, ultimately benefiting the organization. Investing in ongoing training for human scorers, alongside updates in AI algorithms, furthers the goal of achieving optimal AI vs Human scoring.
Top Tools for AI vs Human QA Scoring
When discussing the top tools for AI vs Human QA Scoring, it’s crucial to note several key technologies that enhance the evaluation process significantly. The first noteworthy tool is Natural Language Processing (NLP) software, which employs algorithms to assess spoken language and text patterns for quality analysis. NLP can save time and highlight vital areas for improvement, making it a valuable asset in any QA scoring toolkit.
Next, AI-based analytics platforms are instrumental in providing quantitative insights. These tools analyze large volumes of data swiftly, allowing for performance benchmarking and identifying trends that a human reviewer might miss. Human scorers bring empathy and contextual awareness, making them essential for interpreting nuanced responses and providing rich, qualitative feedback. Ultimately, the best approach involves integrating these AI tools alongside human judgment, combining speed and efficiency with essential human insight to enhance overall quality assurance outcomes.
Insights with insight7
The insights gained from quality assurance processes are becoming increasingly essential as businesses strive to remain competitive. Insight7 offers a platform that bridges the gap between traditional human QA scoring and the advancements brought by AI. By analyzing data from customer interactions and feedback, this tool enables organizations to extract valuable insights quickly and efficiently. This rapid analysis can be particularly beneficial in high-stakes environments where timely decisions are necessary.
Incorporating both AI and human scoring methodologies can create a robust system. While AI excels at processing large volumes of data and identifying patterns, humans bring valuable contextual judgment that machines may miss. Understanding the interplay between these approaches can streamline quality assurance processes and enhance overall decision-making. As businesses adapt, combining AI’s analytical capabilities with human intuition will redefine how QA scoring is executed in the future.
- Overview of how insight7 aids in QA scoring.
Insight7 is designed to enhance the QA scoring process by marrying the efficiency of AI with the nuance of human judgments. This platform provides users with a self-service model that facilitates large-scale data analysis. By simplifying how organizations conduct customer interviews and analyze feedback, it helps businesses overcome traditional challenges in extracting actionable insights.
The technology accelerates the process of identifying trends and patterns in customer data, which is often tedious for human scorers. Yet, it doesn’t replace the human touch; rather, it augments it, allowing human evaluators to focus on interpreting data in ways that AI alone cannot. This blend of AI technology and human insight enables organizations to respond more quickly to customer needs, ultimately gaining a competitive edge in the market. As businesses navigate the balance of AI vs Human scoring, tools like Insight7 play a pivotal role in making the process smoother and more effective.
Other Key Tools for QA Scoring
In the discussion of AI vs Human QA Scoring, it’s essential to recognize the pivotal tools that enhance the quality assurance process. First, consider analytics platforms that provide detailed insights into performance metrics. These tools are vital for identifying trends over time, helping both AI and human scorers understand how changes impact quality assurance outcomes.
Next, evaluation templates are significant assets. They allow users to establish clear criteria for scoring, ensuring consistency across evaluations. With a defined framework, both AI and human evaluators can benchmark performance against established standards. Additionally, call monitoring tools are crucial for real-time evaluation and provide constructive feedback. By integrating these tools, organizations can achieve a well-rounded approach that enhances the effectiveness of both AI and human scoring methods. Collectively, these key tools facilitate achieving optimal quality assurance, combining technology's efficiency with human insight.
- Tool 1: Tool description and benefit.
In the evolving field of QA scoring, one tool stands out for its ability to bridge the gap between AI and human expertise. This tool simplifies data analysis and enhances decision-making by providing clear insights from various interactions. Users can quickly access information, making it beneficial for teams aiming to improve their quality assurance processes.
Utilizing this tool empowers users to identify pain points and customer desires, turning raw data into actionable insights. It facilitates the entire workflow, allowing multiple files and calls to be analyzed simultaneously. This capability enriches the combination of AI vs Human scoring, as it underscores the importance of human intuition in interpreting data trends and dynamics. Ultimately, leveraging such tools can streamline the QA scoring process, enhancing efficiency while retaining the irreplaceable human element in quality assessments.
- Tool 2: Tool description and benefit.
The next tool in our exploration of AI vs Human QA scoring is designed to streamline data analysis and enhance decision-making. This tool simplifies the process of gathering valuable insights from various data sources, providing users with an intuitive platform. With its user-friendly interface, anyone can engage with the tool effortlessly, eliminating the need for specialized training.
One significant benefit of this tool is its ability to transform raw data into actionable intelligence. It efficiently identifies key insights, including customer pain points and preferences, facilitating informed decision-making. Additionally, by visualizing data in a comprehensible manner, it allows users to easily identify trends and discrepancies. Ultimately, this tool bridges the gap between automated AI scoring and human judgment, improving the overall quality assurance process and enabling teams to respond quickly to customer needs.
- Tool 3: Tool description and benefit.
In the evolving conversation around AI vs Human QA Scoring, understanding effective tools is essential. Tool 3 focuses on a platform that simplifies the quality assurance process and encourages user accessibility. This tool can analyze conversation data and produce actionable insights without requiring extensive training or expertise. This user-friendly approach democratizes insights, allowing anyone in a business to leverage the data efficiently.
The benefits extend beyond mere accessibility. By utilizing this platform, users can quickly identify pain points, desires, and behaviors from customer interactions. This focus on data visualization enables teams to gather evidence-based insights that drive better decision-making. Therefore, the integration of AI tools fosters a synergy with human intuition, ensuring that critical nuances do not get lost, thus enhancing overall QA efforts. This shared space between AI and human oversight can streamline operations and improve the overall quality of outputs.
Conclusion: The Future of AI vs Human Scoring
As we look to the future, the ongoing evolution of AI vs human scoring in quality assurance will shape how organizations assess performance. AI offers remarkable efficiency, scalability, and a data-driven approach, reducing the time needed to evaluate large volumes of interactions. However, it is essential to recognize that human scorers possess invaluable insights and contextual understanding that AI cannot fully replicate.
The optimal solution may lie in a hybrid approach, combining AI's strengths with the nuanced judgment of human evaluators. Organizations must focus on refining this integration, ensuring that technology complements human expertise rather than replacing it. As AI continues to advance, maintaining a balance between automation and human insight will be crucial for achieving the highest quality in scoring methodologies.