AI Tagging Efficiency is transforming the quality assurance (QA) landscape, providing a powerful tool for enhancing workflow productivity and accuracy. As businesses increasingly rely on data-driven decisions, the ability to efficiently tag and categorize content becomes paramount. AI systems can process vast volumes of data, significantly reducing the time and effort traditionally required by human taggers.
Despite their capabilities, it's crucial to recognize the limitations of AI in tagging efficiency. While these tools can achieve impressive accuracy rates, they may still misidentify nuances that a human might catch. Therefore, understanding the balance between AI's efficiency and the invaluable insights offered by human intuition is essential for maximizing QA effectiveness. As we explore the specific tools and technologies available, we'll gain a clearer picture of how to best leverage AI tagging efficiency in QA processes.
Analyze qualitative data. At Scale.
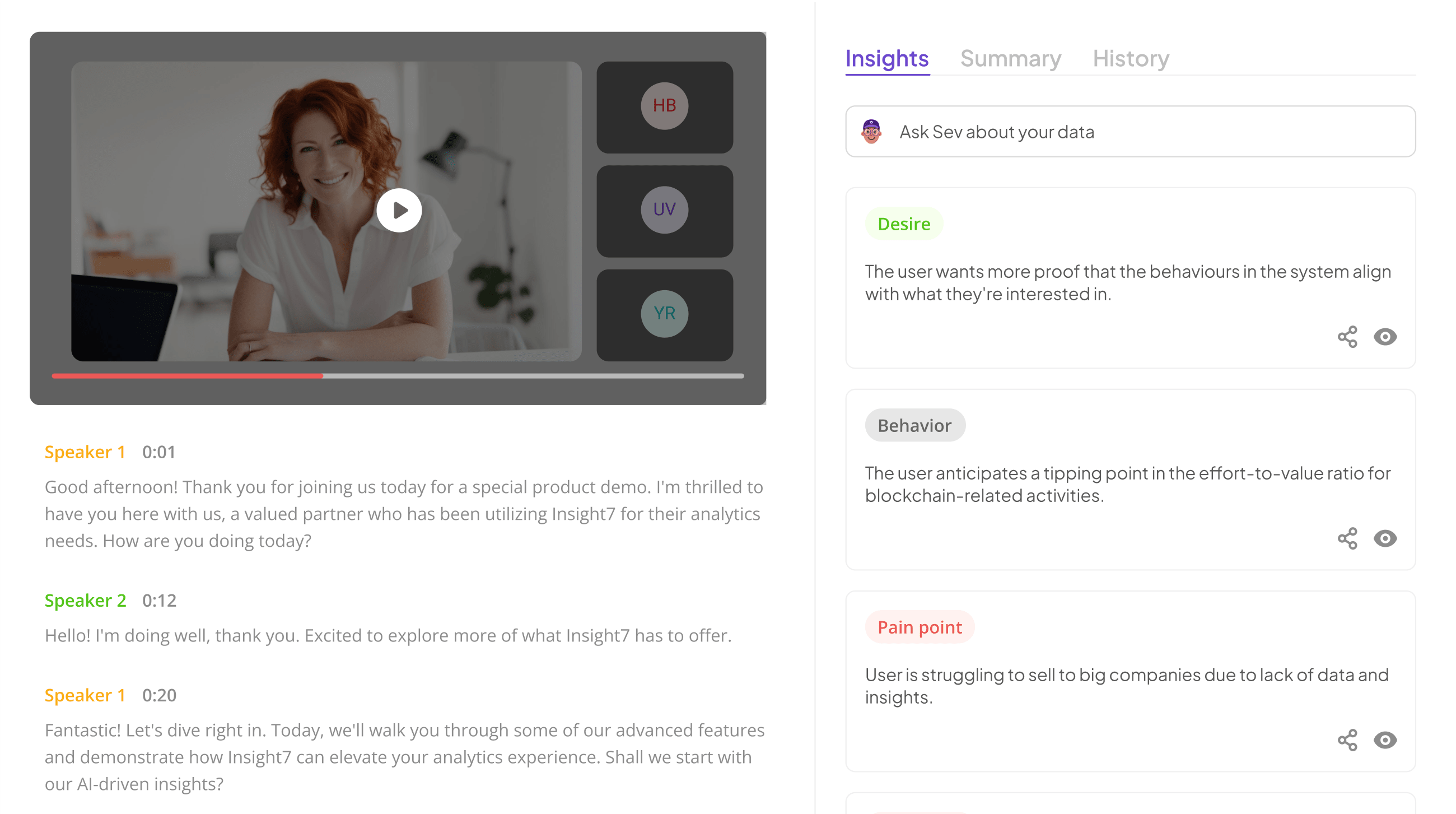
Advanced AI Tagging Efficiency and Their Benefits
Advanced AI tagging efficiency is revolutionizing quality assurance processes, allowing for remarkable improvements in accuracy and speed. By harnessing sophisticated algorithms, AI tools can process vast amounts of data and apply precise tags based on predefined criteria, minimizing human error. This enhances productivity, as teams can focus on interpretation rather than time-consuming manual tasks.
The benefits of AI tagging efficiency extend beyond mere speed. These tools enable real-time insights into performance metrics, offering organizations the ability to make data-driven decisions quickly. Consistently high accuracy levels foster trust in the results, paving the way for deeper analysis and strategy development. Ultimately, the integration of AI tagging can lead to more informed, responsive, and agile quality assurance practices, enhancing overall operational effectiveness.
How AI Tools Achieve Unmatched Tagging Precision
AI tagging efficiency relies on sophisticated algorithms that analyze data patterns and context. Through machine learning and natural language processing, AI tools systematically identify relevant tags, achieving precision that often surpasses human capability. Unlike humans, who may be influenced by fatigue or bias, AI operates consistently and rapidly, providing reliable tagging for large volumes of data.
One key to this unmatched precision lies in the ability of AI tools to learn from vast datasets. By being trained on diverse examples, they can adapt and refine their tagging strategies. Furthermore, these systems continuously improve over time, learning from user feedback to enhance accuracy even more. With each analysis, AI tools become better equipped to handle intricate tagging requirements, ensuring that organizations can maintain high standards of quality assurance in their processes.
The Benefits of AI Tagging Efficiency Over Human Methods
AI Tagging Efficiency offers notable advantages over traditional human tagging methods. First, AI dramatically accelerates the tagging process, enabling rapid categorization of vast data sets. This efficiency is especially beneficial for businesses that require quick turnaround times in analyzing customer interactions or feedback. Unlike humans, AI systems can process and tag data at scale, significantly reducing bottlenecks in workflows.
Moreover, AI ensures a level of consistency that's challenging for human taggers to maintain. Human errors, whether due to fatigue or bias, can lead to inconsistent tagging outcomes. In contrast, AI employs algorithms designed to adhere to set rules without variation. This uniformity enhances the reliability of the insights generated from tagged data. Thus, while AI Tagging Efficiency brings scalability and consistency, it remains essential to consider the contexts in which it operates effectively. Understanding these benefits allows organizations to harness AI for improved data management while recognizing the need for human oversight in nuanced scenarios.
Extract insights from interviews, calls, surveys and reviews for insights in minutes
Top AI Tools That Enhance Tagging Efficiency
AI tagging efficiency is significantly boosted by innovative tools that streamline the tagging process. Among the top contenders are MonkeyLearn, Tagtog, Clarifai, Amazon Rekognition, and Google Cloud Vision. Each of these tools offers unique features that can enhance the accuracy and speed of tagging tasks. For instance, MonkeyLearn utilizes machine learning algorithms for text analysis, which allows users to create customized taggers tailored to specific needs.
Tagtog stands out for its collaborative approach, making tagging a shared experience that enhances team productivity. On the other hand, Amazon Rekognition excels in image analysis, automatically detecting and tagging objects within photos. Google Cloud Vision provides a robust solution with its powerful image recognition capabilities, allowing seamless integration into diverse applications. Collectively, these tools not only increase tagging efficiency but also minimize human error, creating a more reliable quality assurance process.
Insight7: Redefining AI Tagging Efficiency
The implementation of AI tagging efficiency radically transforms how we manage and utilize data. With these advanced tools, organizations can analyze vast amounts of data far more quickly than human taggers. This technology enables companies to extract meaningful insights from customer interactions, conversations, and feedback at an unprecedented scale. The efficiency gained through AI tools allows for faster decision-making, ensuring companies remain competitive in rapidly changing markets.
Moreover, AI tagging efficiency can address challenges that human analysts face, such as inefficiency and inconsistency in categorizing data. While human judgment is invaluable, relying solely on it can lead to slow workflows and potential biases. By coupling the strengths of AI with human insight, companies can achieve a balanced approach to data analysis, enabling them to respond effectively to customer needs and market dynamics. The future of tagging lies in this synergy, where AI not only enhances performance but also supports human creativity and strategic thinking.
Other Leading Tools Enhancing Tagging Efficiency
In the evolving world of AI solutions, several advanced tools significantly enhance tagging efficiency. MonkeyLearn stands out as a user-friendly platform, enabling users to create custom models for text analysis and categorization. It offers intuitive functionality that allows users to integrate tagging seamlessly into their existing workflows. Next, Tagtog provides an effective way to annotate and tag textual data efficiently, catering to a range of industries that rely on precise categorization for improved data management.
Clarifai has carved a niche with its powerful image and video recognition capabilities. This tool can classify and tag multimedia content based on user-defined terms, significantly optimizing the content management process. Amazon Rekognition and Google Cloud Vision are also noteworthy; both facilitate visual data tagging through machine learning technologies. By automating and refining the tagging process, these tools collectively enhance AI tagging efficiency in various organizational contexts, paving the way for more streamlined operations.
- MonkeyLearn
AI tagging efficiency has seen remarkable improvements with the advent of advanced tools designed for data classification and analysis. One such tool exemplifies the capability to enhance tagging processes significantly, making them faster and more accurate than traditional methods. Its design leverages machine learning algorithms to automate the tagging of large datasets, reducing the time needed for human involvement.
However, itโs essential to recognize the limitations of such tools. While they excel in efficiently processing data, they may still struggle with contextual nuances that a human tagger would naturally understand. Issues can arise in interpreting language subtleties or recognizing sentiments, which highlights the importance of human oversight in critical tagging scenarios. Balancing AI tagging efficiency with human intuition creates a hybrid approach that can optimize data accuracy and relevance in various applications.
- Tagtog
AI Tagging Efficiency plays a crucial role in modern Quality Assurance (QA) processes, particularly in tagging audio, text, and visual content. One tool designed to optimize this efficiency offers a sophisticated method of organizing and categorizing data. This tool allows users to create specific tags that can identify various elements in a transcript, assisting in quickly locating relevant information. For instance, capturing details like service types or customer interactions can be streamlined, enabling faster decision-making through organized data.
Moreover, the tool facilitates filtering capabilities that enhance the quality of insights drawn from the tagging process. By specifying tags for various services, call outcomes, and customer responses, users can analyze trends much more effectively. Yet, like all AI-driven solutions, it brings its challenges. While the tagging efficiency is impressive, it still relies on human input to define context and nuances that a machine might miss. Emphasizing the need for a balanced approach, combining the robust tagging capabilities of AI with the essential human touch is key for optimal results.
- Clarifai
AI Tagging Efficiency in advanced systems is significantly enhanced to cater to various data quality assurance needs. One notable tool excels in accurately recognizing and categorizing images and text through its intuitive interface and robust algorithms. By employing sophisticated deep learning techniques, it improves the speed and accuracy of tagging, enabling businesses to process large volumes of data efficiently.
However, while AI offers remarkable tagging efficiency, it is crucial to acknowledge that it also has its limitations. The tool may struggle with nuanced context and cultural references that human taggers readily understand. Ultimately, combining this AI solution with human oversight can optimize data tagging processes, ensuring high quality while capitalizing on efficiency. This balance allows organizations to navigate the complexities of AI-enhanced workflows effectively.
- Amazon Rekognition
Amazon Rekognition exemplifies advanced AI tagging efficiency by utilizing sophisticated algorithms to analyze images and videos. This tool automatically identifies objects, scenes, and activities within media, enhancing tagging speed and accuracy. Users can gain insights from large datasets, enabling faster decision-making processes. Typically, its precision in detecting faces and emotions supports various applications, from security monitoring to customer behavior analysis.
While it significantly outperforms manual tagging in terms of speed, the tool is not without its limitations. Factors like lighting conditions, image quality, and diverse perspectives can hinder accuracy. Additionally, it lacks the human touch when interpreting context or nuance in images. Thus, while AI tagging efficiency can streamline operations greatly, integrating human oversight remains essential to optimize results. Balancing these elements ensures a comprehensive approach to media analysis and tagging, leading to more reliable outcomes.
- Google Cloud Vision
Google Cloud Vision offers robust AI tagging efficiency, enabling businesses to automate the image labeling process. This tool leverages machine learning to analyze the content of images, identifying objects, text, and even the mood depicted in visuals. By integrating such technology, organizations can significantly reduce the time and effort traditionally associated with manual tagging.
However, while AI-enhanced tools can outperform human taggers in speed and volume, they come with limitations. For instance, the accuracy of tagging can vary based on image quality and the complexity of visual elements. Furthermore, the subtleties of context or cultural references may elude an AI's understanding. Thus, combining AI tagging efficiency with human oversight is essential for achieving optimal results in any project that relies on image analysis. This partnership can result in improved accuracy and relevancy in tagging over time.
Challenges and Limitations of AI QA Tools in Tagging
AI Tagging Efficiency often faces significant challenges and limitations when employed in quality assurance processes. One major obstacle is the reliance on historical data. AI models learn patterns and associations from previous information, which can result in outdated or biased tagging when exposed to new or evolving contexts. This issue highlights the need for continuous training and updates to maintain accuracy.
Another critical limitation lies in the lack of human intuition. Unlike human taggers who can consider nuanced meanings and contextual subtleties, AI tools may struggle with ambiguous content. This shortcoming can lead to misinterpretations, undermining the overall effectiveness of AI in complex scenarios. Therefore, while AI tools enhance tagging efficiency, they cannot entirely replace the nuanced understanding that human cognition provides. Addressing these challenges is essential for maximizing AI's potential in tagging roles while ensuring reliability in various applications.
Understanding the Boundaries of AI Tagging Efficiency
AI Tagging Efficiency has made significant strides, yet understanding its boundaries is crucial for effective implementation. While AI-driven tools excel at processing vast amounts of data quickly, they can struggle with nuanced contexts. For instance, AI systems may misinterpret slang or cultural references, resulting in inaccuracies that could impact analysis.
One key limitation involves the AI's reliance on training data. If this data is biased or lacks diversity, the AI's tagging could reflect these biases, potentially skewing results. Additionally, while AI excels in pattern recognition, it may not grasp the intricate subtleties that a human tagger could easily identify. Hence, combining AI efficiency with human insight is essential to achieve the most reliable outcomes. By recognizing these limitations, users can harness the strengths of AI tools while remaining vigilant about their shortcomings, ultimately leading to more balanced and accurate tagging.
Human Intuition: Where AI Tools Still Fall Short
AI Tagging Efficiency is significantly enhanced by advanced algorithms, yet there are areas where human intuition remains unmatched. One critical aspect is the ability to understand nuance and context in language. While AI tools are effective at processing data quickly, they often struggle with the subtleties that human taggers intuitively grasp. This results in errors or misinterpretations, particularly in complex, nuanced conversations where tone and intent play vital roles.
Furthermore, human taggers can adapt to evolving situations and environmental cues more seamlessly than AI tools. This adaptability allows them to consider factors that algorithms may overlook. As a result, while AI can boost tagging efficiency dramatically, it still lacks the emotional intelligence and contextual background that humans inherently possess. Balancing AI's speed with human insight can create a more comprehensive approach to tagging, ensuring accuracy alongside efficiency.
Conclusion: Balancing AI Tagging Efficiency with Human Insight
AI Tagging Efficiency presents a unique solution for organizations seeking precision and speed in data management. However, while AI systems excel in processing large amounts of data quickly, they lack the nuanced understanding that human insight provides. Therefore, integrating AI capabilities with human oversight can create a more balanced and effective tagging approach.
As such, ensuring that AI tools function as supportive allies, rather than stand-alone solutions, is essential. By combining the efficiency of AI with the critical thinking and contextual understanding of human taggers, organizations can improve overall accuracy while benefiting from faster workflows. This collaborative approach harnesses the best of both worlds, enhancing data quality in ways neither can achieve alone.