AI Discourse Analysis has emerged as a transformative approach to understanding the nuances of communication in various contexts. By leveraging advanced tools, we can uncover patterns, sentiments, and themes that traditional analysis might overlook. This section introduces essential concepts that underpin effective AI discourse analysis, differentiating it from conventional methods.
Understanding the basic principles equips researchers and practitioners to harness the full potential of AI technology. Key components like discourse structure, coherence, sentiment analysis, and topic modeling play vital roles in interpreting language data. Familiarity with these concepts sets the stage for successfully applying AI discourse analysis tools in diverse fields, ultimately enhancing comprehension and fostering meaningful communication.
Analyze qualitative data. At Scale.
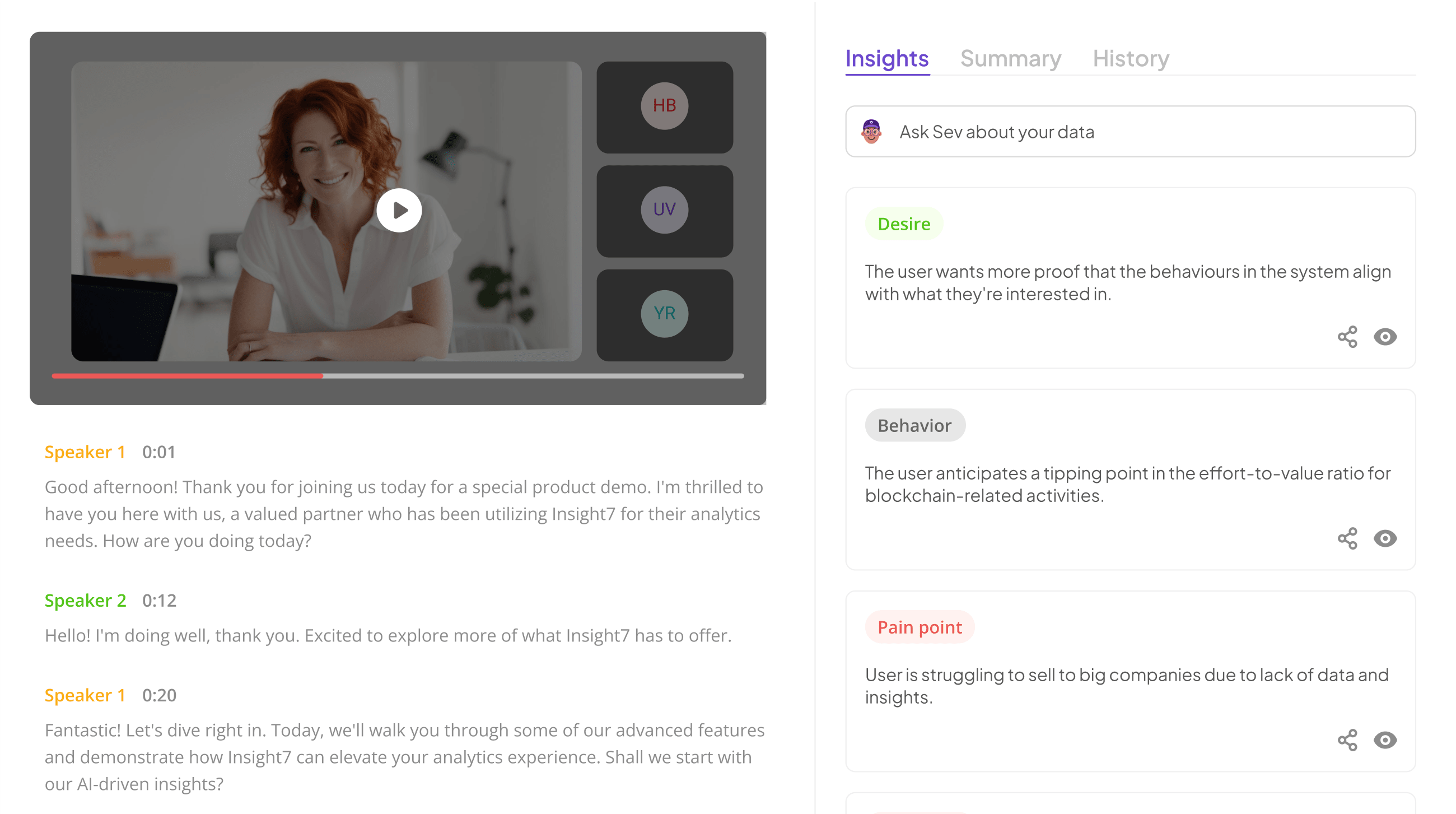
Understanding AI Discourse Analysis: Basics and Key Concepts
AI Discourse Analysis serves as a framework for understanding and interpreting communication patterns across various platforms. It encompasses the study of language usage in different contexts, revealing how words reflect thoughts, feelings, and social dynamics. This analytical approach is crucial for organizations aiming to derive insights from customer interactions and feedback.
Key concepts within AI Discourse Analysis include discourse structure, coherence, sentiment analysis, and topic modeling. Discourse structure refers to how statements and arguments are organized, facilitating effective communication. Coherence ensures that ideas flow logically, enhancing comprehension. Sentiment analysis evaluates emotional tones in dialogue, providing valuable insights into consumer sentiments. Lastly, topic modeling identifies prevalent themes in large datasets, allowing businesses to quickly grasp essential topics of discussion. By leveraging these concepts, organizations can transform raw data into actionable insights, enriching their understanding of audience behaviors and preferences.
What is AI Discourse Analysis?
AI Discourse Analysis is an innovative approach that combines artificial intelligence techniques with the study of language and communication. It explores how language shapes interactions, meanings, and social dynamics in various contexts. By analyzing spoken and written discourse, AI Discourse Analysis tools can identify patterns, themes, and insights that may not be immediately apparent.
The importance of AI Discourse Analysis lies in its ability to enhance our understanding of communication. For businesses and researchers, it offers a powerful means to assess customer interactions, gauge sentiment, and derive actionable insights. Whether used in customer service evaluations or social media monitoring, these tools provide a deeper understanding of how language influences perceptions and behaviors. Ultimately, AI Discourse Analysis empowers users to make informed decisions based on comprehensive language insights, shaping strategies that resonate with their audiences.
- Definition and scope
AI Discourse Analysis serves as a vital framework for understanding the complexities of communication in various contexts. At its core, this approach focuses on the systematic examination of spoken or written discourse, enabling users to extract meaningful insights from conversations or text. The scope of AI Discourse Analysis extends beyond mere content evaluation; it encompasses aspects such as sentiment analysis, coherence, and thematic identification, which facilitate nuanced understandings of communication patterns.
The application of AI Discourse Analysis can span numerous fields, including marketing, customer service, and social research. By identifying trends and themes, organizations can improve their interactions and enhance user experiences. Moreover, the importance of employing reliable tools in AI Discourse Analysis cannot be overstated, as they bridge the gap between data and actionable insights. This approach ultimately aims to foster improved communication strategies, illuminating how audiences perceive messages and interact with content.
- Importance in modern communication
In a world increasingly defined by digital interactions, the importance of AI Discourse Analysis in modern communication cannot be overstated. This innovative technology enables us to dissect and understand conversations at an unprecedented scale. By analyzing textual data from various sources like customer feedback and social media interactions, organizations can gain valuable insights into public sentiment and engagement.
AI Discourse Analysis enhances our ability to articulate thoughts and respond effectively in real-time. It aids in identifying patterns in communication, transforming raw data into actionable strategies. As teams become more adept at using these tools, they can foster collaboration and improve decision-making processes. Ultimately, utilizing AI Discourse Analysis can lead to more meaningful interactions, which are essential in todayโs fast-paced, information-driven environment. Understanding its significance will empower businesses to navigate communication challenges thoughtfully and effectively.
Key Concepts in AI Discourse Analysis
AI Discourse Analysis offers a systematic approach to understanding and interpreting communication. This field involves various concepts that enhance our grasp of how language shapes human interaction. One essential idea is discourse structure and coherence, which focuses on how the organization of text contributes to meaningful communication. Recognizing the relationship between parts of spoken or written discourse allows for deeper insights into its effectiveness.
Another important concept is sentiment analysis, which assesses the emotional tone in communication. By identifying sentiments, analysts can gauge responses to specific topics and understand the audience's feelings. Additionally, topic modeling and theme extraction are critical for categorizing discussions and pinpointing prevalent ideas. Collectively, these concepts create a robust foundation for executing AI Discourse Analysis, helping researchers and practitioners derive beneficial insights from dialogues and texts. Understanding these key concepts elevates our ability to analyze and engage in effective communication strategies.
- Discourse structure and coherence
Discourse structure and coherence are pivotal components in AI Discourse Analysis. Understanding how discourse is structured enables the effective organization of information, allowing for logical flow and clarity. Essential aspects include the sequence of ideas, the relationships between them, and the overall narrative coherence that guides readers. Each element plays a role in enhancing the reader's comprehension and engagement with the text.
Coherence is achieved when the discourse elements connect seamlessly. This connection helps convey meaning and allows readers to follow the argument or narrative without confusion. Employing AI tools can significantly bolster this process by analyzing patterns and identifying inconsistencies in discourse. Such analysis can provide insights into how ideas relate and contribute to a more organized presentation. By focusing on the structure and coherence of discourse, AI Discourse Analysis enhances communication effectiveness across various domains.
- Sentiment analysis and emotion detection
Sentiment analysis and emotion detection play a crucial role in AI Discourse Analysis, allowing systems to interpret the emotional tone of text data. By utilizing advanced algorithms, AI tools can categorize sentiments as positive, negative, or neutral, effectively gauging public opinion or customer satisfaction. This process involves analyzing word choices, expressions, and contextual meanings, enabling deeper insights into communication patterns.
Understanding these nuances goes beyond basic sentiment classification. Emotion detection can identify specific feelings such as joy, anger, or sadness within the text. This granularity provides valuable context for businesses and researchers, as it enhances their ability to respond to users' needs and concerns promptly. By integrating sentiment analysis and emotion detection into their communication strategies, organizations can foster more meaningful interactions, leading to improved customer relationships and informed decision-making. This dynamic approach ultimately transforms qualitative data into actionable insights, amplifying the efficacy of AI Discourse Analysis.
- Topic modeling and theme extraction
In AI Discourse Analysis, topic modeling and theme extraction play crucial roles in uncovering underlying patterns within large sets of textual data. Topic modeling allows analysts to identify clusters of words that frequently occur together, representing common themes present in conversations or documents. This method highlights the prominent issues and interests within the text while filtering out noise, enabling a clearer understanding of discourse.
Theme extraction goes a step further by summarizing these identified topics into key themes that capture the essence of the discussions. This process involves analyzing the context in which words and phrases appear to create insightful narratives that inform decision-making. By effectively employing topic modeling and theme extraction, researchers can gain valuable insights from qualitative data, facilitating a deeper understanding of customer sentiments and needs. Ultimately, these techniques enhance the power of AI Discourse Analysis, making it possible to derive meaningful conclusions from extensive datasets.
[ hfe_template id=22694]Exploring AI Discourse Analysis Tools and Techniques
AI Discourse Analysis offers a vital approach to understanding patterns in communication through sophisticated tools and techniques. Diving into this field reveals various methods for interpreting language, sentiment, and thematic structures within large datasets. These techniques empower researchers and businesses to extract meaningful insights from user interactions, enhancing their understanding of public perception and feedback.
Several effective tools take center stage in this analysis, each designed to cater to unique needs. For instance, Insight7 stands out for its comprehensive feature set that streamlines data analysis and visualization. Other noteworthy tools include MonkeyLearn, Lexalytics, Nvivo, the Natural Language Toolkit (NLTK), and Google Cloud Natural Language. Each tool provides distinct functionalities, such as sentiment analysis and topic modeling, essential for leveraging discourse analysis efficiently. Together, these methods and technologies pave the way for improved communication strategies and informed decision-making.
Top AI Discourse Analysis Tools
When discussing the top AI Discourse Analysis tools, itโs essential to recognize their significant role in understanding communication. These tools leverage sophisticated algorithms to analyze text and speech, providing insights into patterns and sentiments. This can enhance how organizations engage with their audience and improve their messaging strategies.
Among the most notable tools is Insight7, which offers comprehensive features catering to various analytical needs. Additionally, tools like MonkeyLearn and Lexalytics excel in sentiment analysis, allowing for the detection of nuanced emotional tones in dialogues. Nvivo is favorable for qualitative data analysis, while the Natural Language Toolkit (NLTK) serves as an excellent resource for developing custom solutions. Lastly, Google Cloud Natural Language provides robust capabilities for text analysis, making it a versatile choice for businesses looking to implement AI Discourse Analysis effectively. These tools collectively enhance communication strategies, ensuring a more nuanced understanding of interactions.
- Insight7
Understanding the nuances of AI Discourse Analysis is increasingly important in today's data-driven world. This method aids organizations in gaining timely insights from customer conversations, ensuring they leverage these insights for competitive advantage. Traditional analysis methods often struggle to keep pace with the vast amount of data generated through customer interactions, emphasizing the need for more effective tools.
AI Discourse Analysis empowers businesses to streamline the process of interpreting qualitative data, transforming it into meaningful strategies. This capability allows for a collaborative approach to insights, making it easier to translate findings into actionable business steps. Moreover, accessibility to self-service platforms enhances user engagement, fostering a culture of proactive inquiry rather than reactive responses. Understanding this dynamic is crucial for retaining a competitive edge in an ever-evolving market.
- Features and applications
AI Discourse Analysis tools offer a variety of features and applications that enhance communication insights. These tools enable users to easily access transcriptions of conversations and extract valuable information such as pain points, desires, and behaviors. With user-friendly interfaces, anyone can engage with the data without requiring specialized training. This democratization of insights allows businesses to understand customer experiences comprehensively and quickly.
In addition to processing individual calls, these tools can analyze large datasets, making it easier to identify trends and themes across multiple conversations. For instance, users can group numerous calls into projects and gain a holistic view of customer sentiments and feedback. This capability transforms raw data into actionable insights, empowering organizations to make informed decisions swiftly. Ultimately, the applications of AI Discourse Analysis extend beyond customer insights, providing advantages in research, strategy development, and improving overall communication efficiency.
- Other Notable Tools
When considering AI Discourse Analysis, there are several notable tools that have garnered attention for their unique capabilities. Tools like MonkeyLearn and Lexalytics stand out for their user-friendly interfaces, making them accessible to both beginners and experienced analysts. These platforms help extract insights from vast text data using advanced machine learning algorithms, facilitating a deeper understanding of public sentiment and discourse patterns.
Another prominent tool is Nvivo, which excels in qualitative data analysis and allows users to explore and visualize themes and patterns seamlessly. Additionally, Natural Language Toolkit (NLTK) provides robust libraries for text processing and analysis, catering to developers and researchers alike. Google Cloud Natural Language offers powerful APIs to analyze sentiment, entities, and syntax, contributing significantly to streamlined workflows in professional settings. Collectively, these tools support diverse applications, ensuring that stakeholders can effectively harness the power of AI discourse analysis for their specific needs.
- MonkeyLearn
AI Discourse Analysis is a transformative approach to extracting insights from textual data, and it requires powerful tools to function effectively. Among these tools is one that excels in providing intuitive data analysis capabilities. Its user-friendly interface allows both novice and experienced users to conduct comprehensive analyses of texts. By harnessing machine learning algorithms, you can analyze various types of data from customer feedback to social media interactions, promoting better understanding of user sentiments.
Moreover, this tool incorporates features like sentiment analysis and topic modeling, enhancing your ability to identify key themes within large volumes of text. It also streamlines the process of evaluating discourse structures, ensuring coherent and meaningful insights. Users benefit from customizable dashboards that visualize data trends in real-time, making it easier to derive actionable strategies. Utilizing such a tool can greatly enhance your understanding of AI Discourse Analysis, guiding you toward informed decision-making based on rich textual insights.
- Lexalytics
This tool stands out in the realm of AI discourse analysis due to its user-friendly platform, designed to democratize the process of gaining insights from conversations. Users can easily access various functionalities directly from the homepage, eliminating the need for extensive training. This accessibility empowers businesses to efficiently generate reports or analyze customer experiences, helping identify friction points.
The platform integrates features such as a library for organizing conversations and an analytical dashboard that extracts valuable insights. By highlighting key themes like pain points and customer desires, it aids in transforming raw data into actionable information. The system captures real-time quotes and evidence from customer interactions, making findings more trustworthy. Overall, this tool enhances AI discourse analysis by enabling teams to access pertinent insights quickly, fostering a more informed decision-making process.
- Nvivo
Nvivo is a software tool widely used for qualitative data analysis, particularly in the realm of AI Discourse Analysis. It offers researchers the ability to organize and analyze large amounts of textual data effectively. With its intuitive interface, users can code text and reveal patterns within the discourse by drawing connections between themes and insights.
The software provides several key features crucial for discourse analysis. Firstly, it allows for the coding of data, enabling users to tag or highlight important sections, which can later be retrieved for in-depth examination. Secondly, Nvivo supports various data types, including text, video, and audio, increasing its versatility in research contexts. Lastly, advanced query functions facilitate the extraction of significant trends and relationships, making it an invaluable asset in understanding communication dynamics in various fields. By utilizing these strengths, researchers can produce richer interpretations of discourse than manual analysis alone would permit.
- Natural Language Toolkit (NLTK)
Natural Language Toolkit (NLTK) serves as a foundational resource in AI Discourse Analysis. It provides a suite of libraries and programs designed specifically for working with human language data. This toolkit effectively supports tasks such as tokenization, stemming, and tagging, making it easier for researchers to analyze language patterns and context.
One of the standout features of NLTK is its ability to manage complex linguistic structures. It allows users to dissect conversations and texts to extract meaningful insights. For instance, researchers can utilize NLTK to conduct sentiment analysis, assessing how emotional tones influence communication. Additionally, the integration of powerful machine learning models enhances the toolkit's effectiveness in processing and analyzing large text corpora. Thus, using NLTK not only streamlines the workflow of AI Discourse Analysis but also elevates the quality of insights drawn from language data.
- Google Cloud Natural Language
Google Cloud Natural Language offers advanced capabilities for analyzing text data, which is essential in AI Discourse Analysis. This tool can identify sentiment, extract entities, and analyze syntax, making it invaluable for understanding the nuances of human communication. By leveraging these features, users can gain insights into how language conveys meaning and emotion, ultimately enhancing their communication strategies.
Moreover, the platform supports multiple languages, broadening its applicability across diverse contexts. It utilizes machine learning to improve its analysis, becoming more effective with usage. This adaptability allows organizations to fine-tune their messaging and respond better to customer needs. Integrating such a tool into discourse analysis practices can significantly enrich the quality of insights generated, driving informed decision-making and strategic planning in various sectors.
Implementing AI Discourse Analysis in Practice
Implementing AI Discourse Analysis in practice requires a clear strategy to effectively utilize the available tools. The initial step involves selecting the right framework for your needs, considering various tools that fit your objectives. Each tool serves specific functions, so understanding their capabilities is essential. Once a selection is made, the next phase focuses on preparing and processing the data. This includes collecting relevant text samples and ensuring the data is clean and organized for analysis.
Finally, after the analysis is conducted, interpreting the outcomes plays a crucial role. It is important to translate the results into actionable insights that can inform decision-making or enhance communication strategies. By following these steps, organizations can effectively harness AI Discourse Analysis for improved understanding in their interactions. Ultimately, implementing these practices enhances overall communication effectiveness and provides valuable insights into audience perceptions and responses.
- Step-by-step guide to using discourse analysis tools
To effectively implement AI Discourse Analysis, start by selecting the most suitable tools for your needs. Analyze the features of various platforms, like Insight7 or Nvivo, to determine their compatibility with your goals. Consider the type of data you will be dealing with and the insights you hope to extract.
Next, prepare and process your data carefully. This involves gathering dialogue, transcripts, or other text formats and ensuring they are clean and organized for analysis. A well-structured dataset enables the software to function optimally, revealing key insights more accurately.
Finally, interpreting your analysis outcomes is crucial. Understand the trends and patterns identified by the tool, and relate them back to your initial objectives. This thorough approach will ensure you gain actionable insights from your AI Discourse Analysis efforts, enhancing your understanding of communication dynamics.
- Selecting appropriate tools
When selecting appropriate tools for AI discourse analysis, it is vital to consider your specific needs and context. Start by identifying the key features that will enhance your analysis, such as sentiment detection, topic modeling, and coherence assessment. The chosen tool should seamlessly integrate with your existing workflow, enabling effective data preparation and interpretation.
Next, evaluate various options based on usability and scalability. Some tools offer user-friendly interfaces ideal for beginners, while others cater to advanced analytics, suited for specialized teams. Additionally, consider the cost-effectiveness of each solution and any support services that accompany them. Ultimately, the right tool will empower your research and provide insights that drive informed decisions. Remember, a thoughtful selection process enhances the overall impact of your AI discourse analysis efforts.
- Data preparation and processing
Data preparation and processing are crucial steps in AI Discourse Analysis as they establish a solid foundation for subsequent interpretations. Initially, data must be collected, often from various sources such as interviews, surveys, or online platforms. Once gathered, the data needs to be organized and pre-processed to ensure accuracy, consistency, and readiness for analysis. This may involve cleaning the data by removing irrelevant or erroneous information, as well as addressing issues like formatting discrepancies.
Next, the analysis phase utilizes tools designed to extract meaningful insights from the prepared data. Techniques such as topic modeling, sentiment analysis, and theme extraction are commonly employed. Each technique reveals specific patterns and trends, allowing for a comprehensive understanding of the discourse. The effectiveness of these insights relies heavily on meticulous data preparation and processing, making this stage a cornerstone of successful AI Discourse Analysis.
- Interpreting analysis outcomes
When exploring AI Discourse Analysis, interpreting analysis outcomes is essential for extracting meaningful insights from the data. By reviewing the findings, researchers can identify patterns, trends, and notable themes that emerge from discourse interactions. This phase allows practitioners to connect specific data points to broader narratives, enabling informed decision-making based on customer sentiments and opinions.
To effectively interpret analysis outcomes, consider these key steps:
Summarization: Begin by creating summaries of the findings, highlighting significant data points and trends. Utilize clear visuals to present this information effectively.
Thematic Identification: Look for recurring themes or topics in the discourse data. Understanding these themes helps to contextualize the data and draw connections among insights.
Quantitative Analysis: Assess the data quantitatively to determine the frequency and relevance of specific sentiments expressed, which can clarify prevailing customer attitudes.
Through these steps, AI Discourse Analysis becomes a powerful tool, facilitating a deeper understanding of communication dynamics within various contexts.
Conclusion: Advancing Communication with AI Discourse Analysis Tools
AI Discourse Analysis tools hold significant promise for enhancing communication in diverse settings. They enable organizations to systematically analyze conversations, offering insights into the effectiveness of dialogue. By harnessing these tools, businesses can uncover underlying themes and sentiments, ultimately refining their communication strategies to better connect with their audience.
Moreover, as technology evolves, the accuracy and capabilities of AI Discourse Analysis tools improve. This advancement empowers users to derive actionable insights swiftly, enabling timely enhancements to messaging. In this way, AI Discourse Analysis is not just a means of understanding communication but a pathway to fostering meaningful interactions and driving engagement.