AI-Enhanced QA Training is reshaping the landscape of quality assurance, offering innovative approaches to train new analysts. As businesses increasingly adopt artificial intelligence, the need for effective training methodologies becomes vital. AI tools can analyze data, assess performance, and streamline processes, providing new analysts with meaningful insights that were once hard to obtain.
In this dynamic training environment, the traditional methods are enhanced by technology, allowing newcomers to quickly adapt and excel. By integrating AI into QA workflows, organizations can ensure that their teams possess a clear understanding of evaluation criteria and compliance standards. This introduction sets the foundation for exploring how AI can facilitate and enrich the training experience for future QA professionals.
Analyze qualitative data. At Scale.
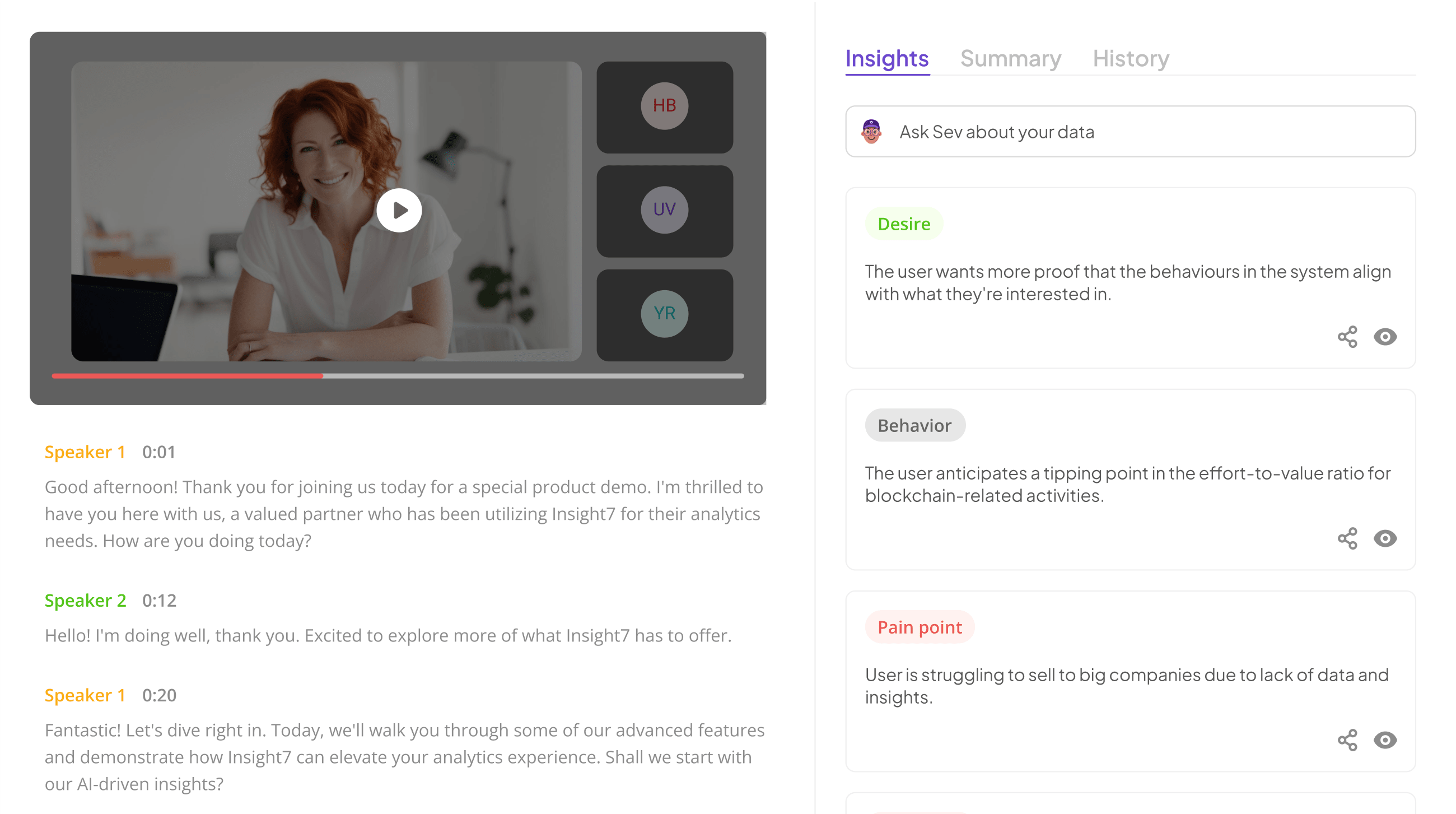
Understanding AI-Augmented Workflows in QA
AI-Enhanced QA Training is revolutionizing how quality assurance analysts are trained. The integration of AI into workflow processes significantly boosts efficiency and accuracy in testing scenarios. By automating repetitive tasks, AI allows new QA analysts to focus on more complex problem-solving and analytical thinking. This transformation not only enhances the learning experience but also equips trainees with the necessary skills to navigate modern QA challenges.
Understanding AI-augmented workflows involves recognizing the synergy between human expertise and advanced technology. AI tools provide data-driven insights, enabling analysts to assess quality metrics promptly and derive actionable conclusions. Additionally, these workflows foster a collaborative environment where seasoned professionals can mentor newcomers, sharing knowledge that complements AI-generated insights. Overall, the marriage of AI and QA workflows creates a solid foundation for training new analysts, preparing them for a dynamic and ever-evolving industry.
The Role of AI in Streamlining QA Processes
AI has a transformative role in streamlining QA processes, significantly enhancing how quality assurance analysts are trained. By integrating AI-driven tools into training programs, organizations can provide new analysts with real-time feedback and insights, thus accelerating their learning curve. This enables trainees to navigate complex data more efficiently, allowing for a smoother transition into their roles.
Moreover, AI can automate routine tasks, such as call evaluations and compliance checks, freeing analysts to focus on critical thinking and problem-solving. With the ability to analyze vast amounts of data, AI identifies patterns and trends that manual processes often overlook. This results in more informed decision-making and ultimately leads to improved quality assurance outcomes. By harnessing AI in training, organizations not only boost efficiency but also develop a deeper skill set in their QA teams, ensuring they are well-equipped for future challenges.
Benefits of AI-Enhanced QA Training for New Analysts
AI-Enhanced QA Training offers numerous benefits for new analysts, elevating their learning experience and efficiency. First, this approach utilizes advanced tools to create realistic, hands-on training scenarios. Analysts can practice their skills in a simulated environment that mimics actual quality assurance tasks. Consequently, they gain practical experience faster than traditional methods allow.
Moreover, AI-driven analytics provide personalized feedback for each analyst. By identifying strengths and weaknesses in real-time, the training can adapt to individual needs. This tailored approach helps analysts improve their skills more effectively. In addition, AI tools automate repetitive tasks, allowing analysts to focus on critical thinking and problem-solving. Ultimately, this enhances their confidence and readiness to tackle real-world challenges in quality assurance. By embracing AI-Enhanced QA Training, organizations can cultivate a new generation of analysts equipped to excel in dynamic work environments.
Extract insights from interviews, calls, surveys and reviews for insights in minutes
Implementing AI-Enhanced QA Training Programs
To successfully implement AI-Enhanced QA Training Programs, organizations must focus on integrating specific AI tools that support learning and development. Begin by identifying the core competencies required for QA analysts, such as testing methodologies, critical thinking, and familiarity with automated tools. Once these competencies are established, selecting the right AI tools becomes crucial. These tools can help facilitate interactive learning experiences and simulate real-world QA challenges, promoting hands-on experience.
Next, designing an AI-augmented training plan is essential. This plan should involve clear learning objectives tailored to each competency. Incorporating hands-on experience with AI tools allows trainees to engage actively, enhancing their understanding of automated processes and data analysis. Lastly, continuous monitoring and iteration of the training process ensure that the program remains effective and aligned with evolving QA standards. Adjustments based on feedback can significantly enhance the effectiveness of AI-Enhanced QA Training, fostering a more competent, agile workforce.
Step 1: Identifying Core Competencies and AI Tools
To effectively train new QA analysts using AI-enhanced workflows, the first step involves identifying core competencies and appropriate AI tools. Begin by assessing the essential skills required for a QA role, which typically include analytical thinking, attention to detail, and effective communication. These competencies serve as the foundational knowledge necessary for utilizing AI tools effectively. Understanding these skills helps in creating a structured framework for the training program.
Next, explore AI tools that can augment the workflows of QA analysts. Consider tools that assist in automating testing, managing test cases, and analyzing results. Selecting the right AI tools not only accelerates the training process but also enhances the efficiency of new analysts as they develop their skill sets. As you navigate this step, always align the chosen competencies and tools with the overall training objectives to ensure a coherent learning experience.
Step 2: Designing an AI-Augmented Training Plan
Designing an AI-Augmented Training Plan involves creating a structured approach to integrating AI tools within the training of new QA analysts. The primary goal is to set clear learning objectives that will guide the training process. By outlining these objectives, trainers can ensure that all critical skills are covered, from understanding AI functionalities to applying practical testing techniques.
Incorporating hands-on experience with AI tools is essential for effective learning. Trainees should engage with real-world scenarios where they can apply their knowledge. This active involvement not only fosters a deeper understanding of the material but also enhances their ability to adapt to different testing environments. By focusing on these elements, organizations can create a robust training framework that supports new analysts in navigating the complexities of their roles, ultimately leading to improved efficiency and effectiveness in QA testing.
Sub-step A: Setting Learning Objectives
In AI-Enhanced QA Training, setting learning objectives is a fundamental step that aligns training with organizational goals. Begin by defining what new QA analysts need to learn. This involves recognizing the core competencies needed for effective quality assurance, such as understanding testing methodologies and familiarizing oneself with AI tools that can streamline processes. This clarity not only enhances the learning experience but also improves performance outcomes.
Once the core competencies are identified, develop specific, measurable objectives. These could include mastering AI-driven testing tools, executing test cases, or analyzing QA results effectively. It is essential to ensure that these objectives engage the analysts and provide a clear path for their progress. Revisit and revise these objectives periodically to accommodate evolving industry standards and technological advancements, thus ensuring that the training remains relevant and impactful.
Sub-step B: Incorporating Hands-On AI Tools
To effectively train new QA analysts, hands-on experience with AI tools is vital. Incorporating these tools into your training curriculum allows trainees to engage with technology actively rather than passively absorbing information. By providing practical exercises, analysts can better understand how AI enhances quality assurance processes. This experiential learning fosters confidence as they navigate AI applications relevant to their roles.
Consider implementing these approaches to enhance your training:
Interactive Simulations: Use AI-driven simulations that mirror real-world testing scenarios. This real-time feedback helps analysts grasp complex concepts swiftly.
Project-Based Learning: Assign projects that require the application of AI tools. Through collaborative efforts, analysts can learn from each other, further deepening their understanding.
Regular Assessments: Conduct assessments using these tools to evaluate both knowledge and practical skills. This ensures continuous improvement and prepares analysts for challenges in QA environments.
By embedding such strategies into your program, your team will benefit from a robust AI-enhanced QA training experience.
Step 3: Monitoring and Iterating the Training Process
Monitoring and iterating the training process is crucial in enhancing the effectiveness of AI-Enhanced QA Training. Start by establishing key performance indicators (KPIs) aligned with your training objectives. These metrics should reflect the progress of new QA analysts, including skills assessed, response times, and error rates. Regularly reviewing these KPIs allows trainers to identify strengths and areas needing improvement.
After data collection, it’s vital to iterate on your training methods. Gather feedback from trainees and incorporate it into training modules to ensure they remain relevant. Review and refine AI tools to enhance learning experiences continually. Be open to adjusting workflows as new challenges emerge. This adaptive approach not only enhances new QA analysts' capabilities but also solidifies the training program's foundation for ongoing development. By fostering an environment of continuous learning and adaptation, organizations can significantly improve QA processes and outcomes.
Top AI Tools for QA Training
In the quest for effective AI-enhanced QA training, selecting the right tools is crucial for empowering new analysts. These tools streamline processes, automate mundane tasks, and provide valuable insights, ultimately fostering a more efficient training environment. Some of the top AI tools to consider include Selenium, which excels in automated testing, and TestComplete, known for simplifying current testing protocols through user-friendly interfaces.
Moreover, platforms like QMetry offer advanced test management, enabling analysts to manage test cases effortlessly. Testim stands out for its AI-driven automation, helping teams quickly adapt to changes and improve accuracy. Using these AI tools not only enhances the learning experience for QA analysts but also creates workflows that support continuous skill development. By integrating these resources into training programs, organizations can ensure their QA teams are equipped with modern, industry-standard practices.
insight7: Leveraging AI for QA Insights
AI-Enhanced QA Training offers a transformative approach to onboarding new QA analysts. By integrating AI-driven tools into training workflows, organizations can significantly improve the learning experience. New analysts can benefit from real-time data analysis and feedback, empowering them to make informed decisions quickly. This shift not only boosts efficiency but also enhances the quality of their work.
Moreover, AI facilitates customized training experiences. Each analyst can engage with personalized modules that cater to their unique learning pace and style. With access to AI-driven insights, trainers can better assess individual progress and adapt content as needed. Implementing such workflows fosters a collaborative environment, ensuring that insights drawn from various analyses can be seamlessly integrated into ongoing training efforts. This holistic approach prepares new QA analysts to excel, ultimately leading to a more effective and agile QA team.
Selenium: Automation in QA Testing
Selenium plays a pivotal role in automating quality assurance testing, providing a powerful tool for QA analysts. With its capability to interact with web applications, Selenium enables testers to automate repetitive tasks, ensuring consistency and efficiency in the testing process. This automation is crucial in today's fast-paced software development environments, where speed and accuracy are vital.
Incorporating Selenium into AI-enhanced QA training allows new analysts to gain hands-on experience with automated testing frameworks. By utilizing AI-driven workflows, training becomes more interactive and engaging. Analysts can analyze real-time data and utilize automation scripts to validate application functionality. This practical exposure not only enhances their technical skills, but also builds confidence in managing complex testing scenarios. Embracing automation through Selenium ultimately empowers QA teams to achieve higher levels of productivity and accuracy in their testing efforts.
Testim: AI-Driven Test Automation Platform
In the realm of AI-Driven Test Automation, modern QA training programs can significantly benefit from introducing new analysts to advanced technologies. This type of platform automates many repetitive tasks that were once manual, allowing novice analysts to focus on deeper analytical skills. As they engage with these tools, they gain hands-on experience with real-time feedback, which enhances their learning journey effectively.
By utilizing such a platform, organizations can facilitate an AI-Enhanced QA Training that not only accelerates the onboarding process but also empowers new team members to become proficient more quickly. A guided approach helps in promoting problem-solving capabilities, all while providing valuable insights into test results and quality assurance metrics. Consequently, this can lead to greater confidence among new analysts as they transition into their roles, fostering a culture of continuous learning.
TestComplete: Simplifying Automated Testing
Automated testing has revolutionized quality assurance by reducing the time and effort required for test execution. TestComplete simplifies the process, offering a user-friendly interface that enables new QA analysts to quickly adapt to automated testing. By integrating AI-enhanced workflows, this tool streamlines repetitive tasks, allowing analysts to focus on more critical judgment-based activities. New analysts can benefit significantly, as they can witness firsthand how AI can optimize their testing processes, ultimately enhancing their competency.
Moreover, TestComplete supports various testing types, including functional, regression, and performance testing. This flexibility allows teams to tailor their testing strategy according to specific project needs. Additionally, its advanced analytics features enable analysts to gain deeper insights from test results, making it easier to identify areas for improvement. Embracing TestComplete within AI-enhanced QA training programs fosters a culture of efficiency, learning, and continuous improvement among new analysts.
QMetry: AI-Enhanced Test Management
QMetry enhances test management through the integration of artificial intelligence, transforming the traditional QA training landscape. By automating repetitive tasks, it allows new analysts to focus on critical thinking and complex problem-solving. This platform employs AI-driven insights, offering real-time data analysis that fosters a more interactive learning experience. New QA analysts can leverage this technology to gain a deeper understanding of testing methodologies and quality assurance protocols.
Moreover, the structured environment of AI-enhanced test management supports new analysts in mastering essential skills quickly. With easy access to templates and evaluation criteria, trainees can engage in practical exercises that simulate real-world scenarios. By bridging the gap between theory and application, this approach not only boosts confidence but also accelerates the onboarding process. Ultimately, understanding AI-enhanced test management can significantly contribute to developing proficient QA analysts equipped for today's dynamic testing environments.
Conclusion: The Future of AI-Enhanced QA Training
The future of AI-Enhanced QA Training holds tremendous potential for transforming the development of new QA analysts. As artificial intelligence continues to evolve, training programs will become more effective, engaging, and tailored to individual learner needs. This technological advancement enables the incorporation of real-time feedback and adaptive learning paths, making the training process not only faster but more impactful for new analysts embarking on their careers.
Moreover, as AI tools become increasingly sophisticated, they will help analysts refine their skills through data-driven insights and performance evaluations. By integrating these innovative approaches, organizations can enhance their quality assurance teams, ensuring higher efficiency and accuracy in their workflows. Ultimately, embracing AI in QA training will empower analysts, equipping them with the necessary tools to navigate an ever-changing work environment.