How to Incorporate Predictive Reporting into Coaching Models
-
Bella Williams
- 10 min read
Predictive Coaching Integration is revolutionizing the way coaches engage with their clients. Picture a coach reviewing data that reveals not just past performance, but also potential future outcomes. This data-driven approach enables coaches to tailor their strategies more effectively, personally addressing the specific needs and behaviors of each individual. By seamlessly incorporating predictive reporting into coaching models, the focus shifts from reactive to proactive development.
Understanding how to implement this integration is essential for coaches aspiring to enhance their practice. By analyzing key metrics and selecting appropriate tools, the coaching experience becomes data-informed and insightful. Coaches can empower their clients with strategies backed by predictive insights, ultimately fostering a more impactful coaching journey.
Analyze & Evaluate Calls. At Scale.
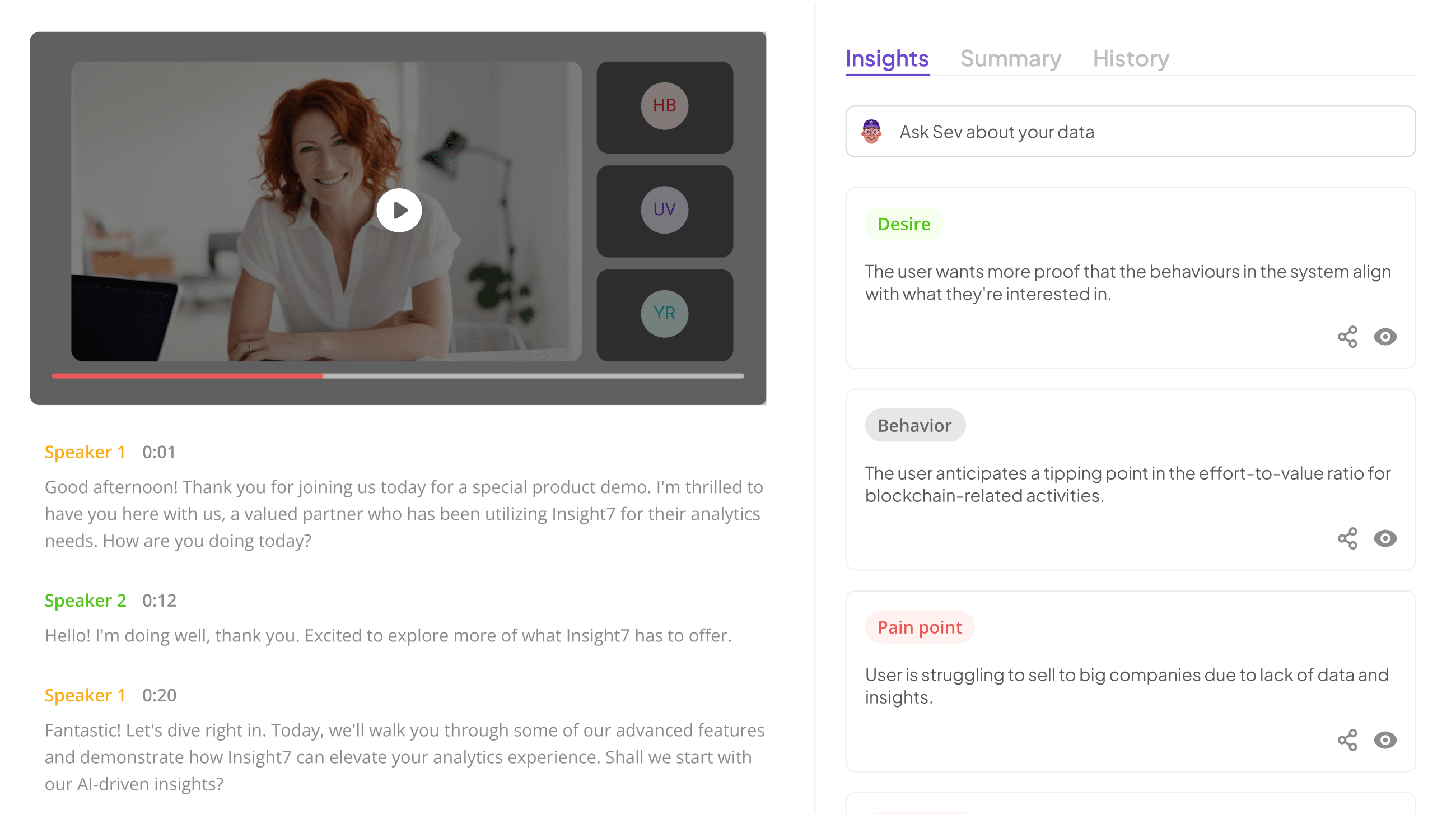
Understanding the Basics of Predictive Coaching Integration
Predictive Coaching Integration aims to blend data-driven insights into traditional coaching practices. Understanding its foundational elements is crucial for coaches eager to adopt this approach. At its core, predictive coaching utilizes analytics and forecasting to tailor coaching strategies, allowing for a more personalized experience. This kind of integration transforms how coaches interact with their clients, leading to improvements in goal-setting and performance tracking.
To effectively implement Predictive Coaching Integration, coaches should focus on the following key aspects. First, identifying essential metrics is vital, as it helps in determining which data points will offer the most valuable insights. Next, selecting the appropriate tools for predictive analysis is crucial for seamless data interpretation. By embracing these principles, coaches can enhance their overall methodology, making their coaching more impactful and client-focused. Adopting predictive reporting transforms the coaching process into a dynamic exchange backed by data, ultimately fostering better outcomes for both coaches and clients.
The Role of Predictive Reporting in Coaching
Predictive reporting plays a pivotal role in enhancing coaching models by transforming data into actionable insights. Through the lens of predictive coaching integration, coaches can assess patterns and trends, leading to more informed decisions. This proactive approach enables coaches to anticipate challenges and capitalize on opportunities, tailoring their strategies to better support individual and team development.
Moreover, the integration of predictive reporting fosters accountability and progress tracking. Coaches equipped with real-time data can identify key performance indicators and measure growth effectively. As a result, coaching becomes more personalized and relevant, addressing specific needs rather than applying a one-size-fits-all approach. Ultimately, predictive reporting not only streamlines the coaching process but also cultivates a culture of continuous improvement, empowering both coaches and coachees to thrive.
- Definition and Importance
Predictive Coaching Integration is defined as the incorporation of data-driven insights into coaching methodologies to enhance performance outcomes. It serves as a powerful tool for coaches to anticipate challenges and identify opportunities for growth. This integration leverages predictive reporting to analyze patterns and trends, ultimately equipping coaches with the knowledge necessary to make informed decisions and provide tailored guidance.
The importance of this approach cannot be overstated. By utilizing predictive reporting, coaches can gain a clearer understanding of an individual or team's unique needs. Furthermore, it allows for the tracking of progress over time, ensuring that coaching strategies evolve alongside the clientโs development. Ultimately, Predictive Coaching Integration fosters a proactive learning environment, enabling coaches to implement strategies that are not only reactive but also anticipatory, thus maximizing the potential for success.
- How Predictive Reporting Enhances Coaching Models
Predictive reporting plays a critical role in enhancing coaching models by offering data-driven insights that inform strategies and decision-making. By analyzing historical performance data, predictive reporting allows coaches to anticipate future outcomes and tailor their approaches to the unique needs of individual clients. This proactive method equips coaches with the information necessary to formulate effective training plans and set achievable goals that resonate with team members.
Moreover, integrating predictive reporting fosters a culture of accountability and continuous improvement. Coaches can identify patterns and trends, allowing them to adjust their methods promptly. This shift from a reactive to a proactive coaching stance ultimately enhances the coaching experience and the overall performance of teams. As a result, coaches and organizations are more equipped to nurture talent and promote development, leading to lasting success within teams and the larger organization.
Steps to Integrate Predictive Reporting into Coaching Models
To effectively integrate predictive reporting into coaching models, begin by identifying key metrics. This entails selecting relevant data points that are aligned with your coaching objectives. Ensure that the metrics you choose are measurable and provide actionable insights. Establish clear objectives for what you hope to achieve through predictive reporting, guiding coaches in evaluating progress and areas for improvement.
Next, focus on choosing the right tools for predictive analysis. Utilizing advanced platforms like insight7, IBM SPSS Modeler, and RapidMiner can facilitate data processing and yield valuable insights. Each tool offers unique features supporting various aspects of predictive coaching integration. Finally, it's essential to train coaches on how to interpret and utilize predictive reports effectively. This creates a data-informed coaching culture, enabling continuous growth and adaptation. By following these steps, organizations can successfully incorporate predictive reporting into their coaching frameworks.
Step 1: Identifying Key Metrics
Identifying key metrics is crucial in the process of Predictive Coaching Integration. The initial step involves selecting relevant data points that directly align with the goals of your coaching model. This could involve defining performance indicators, customer feedback, and training outcomes that will guide your coaching strategies. Remember, the key metrics you choose should provide insights into both the quantitative and qualitative aspects of performance, such as training completion rates and participant satisfaction.
Once you have established your key metrics, itโs essential to set clear objectives for reporting these metrics. Define what success looks like, and ensure that these objectives are measurable. By establishing a transparent framework, you can effectively track progress over time. This enables continual improvement and helps customize coaching interventions based on data-driven insights, ultimately enhancing the efficacy of your coaching program.
- Selecting Relevant Data Points
To effectively incorporate predictive reporting into coaching models, selecting relevant data points is crucial. These data points should align with the coaching objectives and illustrate the performance trends that matter most. Begin by identifying key metrics that reflect the outcomes you aim to achieve, such as engagement levels and individual growth rates. Each data point must help coaches understand where improvement is needed and recognize patterns over time.
Next, consider the context in which the data will be analyzed. For instance, assessing performance across various coaching sessions allows for a deeper understanding of which strategies work best. Collaboratively define what constitutes success in your specific environment; this clarity will ensure that selected data points are meaningful and actionable. Remember, by focusing on relevant data, the integration of predictive coaching can deliver tailored insights, promoting a more effective coaching experience.
- Setting Clear Objectives for Reporting
Setting clear objectives for reporting is pivotal in effectively integrating predictive coaching. When coaches understand what they aim to achieve with their reports, it enhances the overall coaching process. Clear objectives allow for targeted data collection, which can identify trends and insights that may have otherwise gone unnoticed. This clarity ensures that resources are utilized efficiently and that the reporting serves its intended purpose.
To establish these objectives, focus on the following points:
Define Desired Outcomes: Clearly articulate the goals you wish to achieve, such as improving team performance or increasing customer satisfaction.
Identify Key Performance Indicators (KPIs): Select relevant metrics that will allow you to measure progress toward achieving your desired outcomes effectively.
Set a Reporting Cadence: Decide the frequency of reporting, ensuring it aligns with the coaching schedule and allows for timely adjustments based on the insights gathered.
By implementing these practices, coaches can align their reporting strategies with their broader objectives, effectively embracing Predictive Coaching Integration.
Step 2: Choosing the Right Tools for Predictive Analysis
Selecting the appropriate tools for predictive analysis is essential for successful Predictive Coaching Integration. The right tools not only provide valuable insights but also enhance the overall coaching experience. When choosing tools, consider data accessibility, ease of use, and compatibility with existing systems. Popular tools like insight7, IBM SPSS Modeler, and RapidMiner offer unique advantages. These tools can analyze data efficiently and deliver actionable insights to inform coaching strategies.
Next, assess analytics capabilities and reporting features of each tool. Tools should allow coaches to customize reports, monitor key metrics, and visualize data trends. Ultimately, the right tools will support decision-making processes and actively engage team members in their growth journey. Remember, the goal is to create a seamlessly integrated coaching model that continuously evolves, leveraging the insights provided by these powerful platforms. Prioritize a user-friendly interface to ensure that all team members can effectively utilize the chosen tools.
- List of Top Tools for Predictive Coaching Integration
Incorporating predictive coaching integration into your coaching models necessitates the right set of tools to facilitate effective analysis. Here are five top tools that significantly enhance predictive coaching integration:
Insight7: This platform allows easy access to data insights, making it simple for coaches to analyze customer interactions without requiring advanced training.
IBM SPSS Modeler: Known for its robust predictive analytics capabilities, it empowers coaches to generate insights from their data efficiently, aiding in personalized coaching strategies.
RapidMiner: With its user-friendly interface, RapidMiner enables coaches to carry out predictive analysis and create reports without needing extensive coding skills.
H2O.ai: This tool offers advanced machine learning capabilities, allowing for the identification of trends and patterns crucial for data-driven coaching approaches.
KNIME: As an open-source data analytics platform, KNIME facilitates seamless data integration, transformation, and analysis, which are essential for effective predictive reporting.
By using these tools, coaches can better understand their audience, customize their strategies, and make informed decisions to enhance their coaching effectiveness. These technologies not only simplify data handling but also empower coaches to leverage insights that can lead to transformative results.
- insight7
Incorporating predictive coaching integration is crucial for enhancing coaching effectiveness. By utilizing predictive reporting, coaches can make informed decisions that drive better outcomes. This integration allows for real-time data analysis, offering coaches key insights into their clients' progress and areas needing improvement. As coaches analyze patterns and trends, they shift from a reactive approach to one that proactively addresses client needs, fostering a deeper connection.
To successfully implement predictive coaching integration, consider these steps:
- Identify Key Metrics: Determine which performance indicators will provide actionable insights.
- Select Appropriate Tools: Utilize effective software solutions that offer robust predictive analytics features.
- Establish Clear Goals: Set specific objectives that you aim to achieve through predictive reporting.
By focusing on these steps, you can enrich your coaching methodology. Such proactive engagement not only improves coaching outcomes but also enhances the overall experience for your clients.
- IBM SPSS Modeler
IBM SPSS Modeler is a powerful analytics tool essential for predictive coaching integration. Utilizing this software, coaches can explore vast data sets to uncover patterns and insights that drive their coaching decisions. The visual interface simplifies complex analyses, making it accessible for users at any experience level. By embracing this tool, coaches can quantify performance metrics and areas for improvement, ultimately leading to more personalized coaching strategies.
This tool empowers coaches to assess historical performance data, allowing them to make informed predictions about future outcomes. Through predictive modeling, coaches can tailor their methods to suit individual needs and team dynamics. The integration of such a robust analytical tool not only enhances the coaching experience but also fosters continuous improvement by identifying actionable insights. Embracing IBM SPSS Modeler can significantly bolster the effectiveness of coaching models, paving the way for data-driven decision-making.
- RapidMiner
Incorporating predictive coaching integration requires selecting effective tools that can streamline data analysis. Among these, RapidMiner stands out due to its user-friendly interface and powerful analytical capabilities. It enables coaches to gather data from diverse sources and visualize trends, making it easier to understand client needs and behavior patterns.
One significant feature of RapidMiner is its intuitive project setup, which allows users to upload and analyze multiple datasets efficiently. Coaches can perform in-depth analyses using simple queries to extract insights from client interactions. This process not only helps in identifying key performance indicators but also aids in crafting tailored coaching strategies based on concrete data. By integrating RapidMiner into predictive coaching models, coaches can significantly enhance their decision-making processes and deliver more personalized and effective guidance.
- H2O.ai
Predictive Coaching Integration involves the use of advanced data analytics tools to enhance coaching effectiveness. Among the various options available, several prominent platforms stand out. These tools utilize machine learning algorithms and data science techniques to process and analyze vast amounts of information, transforming raw data into actionable insights for coaches.
Using an advanced analytical system can significantly streamline the coaching process. One important step in this integration is identifying key metrics relevant to the coaching goals. Once these metrics are established, the chosen tools can deliver customized predictive reports tailored to each coachโs needs.
Such reports provide a clear understanding of individual and team performance while highlighting areas that require further development. Coaches can leverage these insights to furnish targeted feedback, making coaching sessions more effective and aligned with the specific needs of their clients. Embracing this technological approach ensures that outcomes are not only measurable but also continuously optimized.
- KNIME
KNIME is a powerful open-source tool designed for data analytics, allowing users to create data workflows easily. Its visual programming interface makes it accessible for individuals without extensive technical expertise. This democratization of data analysis is essential for effective predictive coaching integration. Coaches can leverage KNIME to analyze data, identify key performance indicators, and extract actionable insights without needing advanced coding skills.
Integrating KNIME into coaching models begins with its ability to gather and process various data sources. Users can design workflows that pull in data and generate reports automatically, significantly reducing manual work. As a coach, you can utilize these insights to forecast trends and improve client outcomes. Establishing efficient data analysis practices with KNIME ensures that coaches stay informed and responsive to client needs, ultimately enhancing the coaching experience through informed decision-making.
Extract insights from interviews, calls, surveys and reviews for insights in minutes
Implementing Predictive Coaching Integration in Practice
Implementing Predictive Coaching Integration in Practice requires a systematic approach to enhance the coaching experience. Initially, it is essential to analyze case studies that showcase successful integration. These real-world examples provide valuable insights into effective strategies and the potential impact of predictive tools on coaching outcomes. Through detailed assessments, coaches can discover critical patterns and adapt their methodologies to meet specific client needs.
Furthermore, addressing common challenges during integration is crucial. Coaches often encounter obstacles such as data overload and resistance to new technologies. To overcome these hurdles, establishing a clear communication plan and providing adequate training to all users is essential. Emphasizing the benefits of predictive coaching not only aids in smooth integration but also encourages a culture of data-informed decision-making. Ultimately, by navigating challenges effectively, coaches can fully utilize predictive coaching integration to maximize their impact and facilitate transformative growth for their clients.
Case Studies of Successful Predictive Coaching Integration
Successful Predictive Coaching Integration has been showcased through various real-world examples where innovative practices led to significant improvements in coaching effectiveness. In one case, a coaching program implemented predictive analytics to analyze historical training data, which enhanced their understanding of participant performance. By identifying patterns and trends, coaches were able to tailor their approaches for individual needs, leading to improved outcomes and satisfaction among participants.
Another example involved the development of customized feedback loops that included predictive reporting. Coaches utilized these insights to adjust training strategies dynamically, responding to progress in real-time. Participants reported higher engagement, as the coaching sessions became increasingly relevant to their performance goals. The combination of historical data analysis and ongoing feedback fostered a more adaptive coaching model, serving as a valuable lesson in how Predictive Coaching Integration can enhance coaching practices.
- Real-world Examples of Effective Integration
Real-world examples highlight the tangible benefits of Predictive Coaching Integration in various industries. One notable instance involves a financial services company that utilized predictive analytics to enhance its coaching program for customer service agents. By analyzing past performance data and customer interactions, the coaches could tailor training sessions more effectively, leading to a significant increase in client satisfaction scores.
Another example is found in the healthcare sector, where predictive reporting enabled coaching around patient interaction. Coaching teams used data insights to identify common patient concerns and tailored their training to address these needs, resulting in improved patient outcomes. These real-world scenarios clearly demonstrate how predictive analytics can guide coaching practices, ensuring that training is not only relevant but also impactful in meeting organizational goals.
- Lessons Learned from Industry Leaders
The integration of predictive coaching has revealed valuable insights from industry leaders who have successfully adopted this innovative approach. One key lesson is the importance of data-driven decision-making in enhancing coaching effectiveness. Leaders emphasize the necessity of analyzing customer and performance metrics to tailor coaching strategies. By effectively using these insights, coaches can better address the specific needs of their teams and improve overall performance.
Another critical takeaway is the significance of collaboration and communication in predictive coaching integration. Leaders often highlight that fostering a culture of open feedback leads to enhanced learning outcomes. Coaches are encouraged to engage actively with team members, utilizing predictive reporting tools to create a transparent environment where insights are shared and acted upon collectively. This collaborative approach not only streamlines the integration process but also empowers coaches to adapt their methods continuously, ensuring sustained success in their coaching endeavors.
Challenges and Solutions in Predictive Coaching Integration
Integrating predictive coaching into existing frameworks presents several challenges that can hinder effectiveness. One of the most pressing issues is the resistance to change among coaches and clients alike. Many may feel uneasy about relying on data-driven insights instead of their instinct or experience. Additionally, inadequate technical skills can limit a coach's ability to engage with predictive tools effectively, posing a barrier to implementation.
Addressing these challenges requires a multi-faceted approach. Firstly, education around the benefits of predictive coaching integration is essential; helping both coaches and clients understand data's value encourages buy-in. Secondly, investing in training sessions equips coaches to utilize analytical tools confidently. Lastly, establishing a clear roadmap for implementation can guide stakeholders through the transition, ensuring a smoother adaptation process. By tackling these obstacles head-on, predictive coaching integration can enhance the coaching process and drive impactful results.
- Common Obstacles Coaches Face
Coaches often encounter various obstacles when trying to implement Predictive Coaching Integration effectively. One common challenge is the resistance to change within an organization. Many team members may be accustomed to traditional coaching methods and may hesitate to adopt new data-driven approaches. Addressing these concerns requires strong communication and reassurance about the benefits of predictive reporting in improving coaching effectiveness.
Another significant obstacle is the availability and accuracy of data. Coaches may struggle with incomplete or inconsistent data sets, which can hinder meaningful analysis. Moreover, the selection of appropriate metrics that align with coaching goals is crucial. Coaches must clearly define success metrics and ensure that the data collected is relevant and reliable. Overcoming these challenges often involves ongoing training and support, allowing coaches to become comfortable with metrics and data analysis tools. By addressing these common obstacles, coaches can successfully integrate predictive reporting into their coaching models, ultimately enhancing their impact.
- Strategies to Overcome Integration Challenges
Integration of predictive coaching poses unique challenges that require thoughtful strategies to address. One essential tactic is to ensure open communication among all stakeholders involved. By fostering a culture of collaboration, coaches can identify resistance points and address them promptly. Emphasizing the benefits of predictive reporting, such as increased efficiency and better decision-making, also helps to motivate teams to embrace new practices.
Another critical strategy involves providing targeted training to improve comfort with new tools. Workshops, role-playing, and hands-on sessions ensure that coaches feel equipped to utilize predictive coaching integration effectively. Implementing pilot programs allows for initial testing, whereby feedback can be gathered to refine processes further. By approaching these challenges with a comprehensive plan, organizations can successfully embed predictive reporting into their coaching models, ultimately transforming their coaching practices into data-driven success stories.
Conclusion: Achieving Success with Predictive Coaching Integration
Success in Predictive Coaching Integration hinges on a proactive approach to understanding data and its impact on coaching effectiveness. By leveraging predictive analytics, coaches can identify patterns that enhance decision-making and drive meaningful outcomes. The seamless integration of data-driven insights allows coaches to tailor their strategies, making their efforts more effective and focused on individual needs.
Moreover, fostering a culture of data literacy within coaching teams is essential. This not only empowers coaches to utilize predictive reporting efficiently but also encourages continuous improvement across all levels. Ultimately, embracing Predictive Coaching Integration can lead to remarkable advancements in coaching practices, enhancing overall performance and achieving transformative success.
๐ฌ Need Help? Chat with Support
Our team typically responds within minutes