10 AI Quality Analytics KPIs That Replace Manual QA Metrics
-
Bella Williams
- 10 min read
AI Quality KPIs play a pivotal role in modern Quality Analytics, marking a significant shift from traditional manual quality assessments. As organizations increasingly rely on data-driven insights, these KPIs provide a framework for evaluating and enhancing product quality through artificial intelligence. This evolution not only streamlines QA processes but also uncovers deeper insights, fostering continuous improvement and innovation.
Understanding AI Quality KPIs is essential for optimizing performance and ensuring products meet high standards. By focusing on these metrics, teams can effectively measure quality attributes such as predictive accuracy and cost efficiency. Ultimately, embracing AI Quality KPIs empowers organizations to elevate their quality assurance strategies and adapt to the dynamic needs of the market.
Analyze & Evaluate Calls. At Scale.
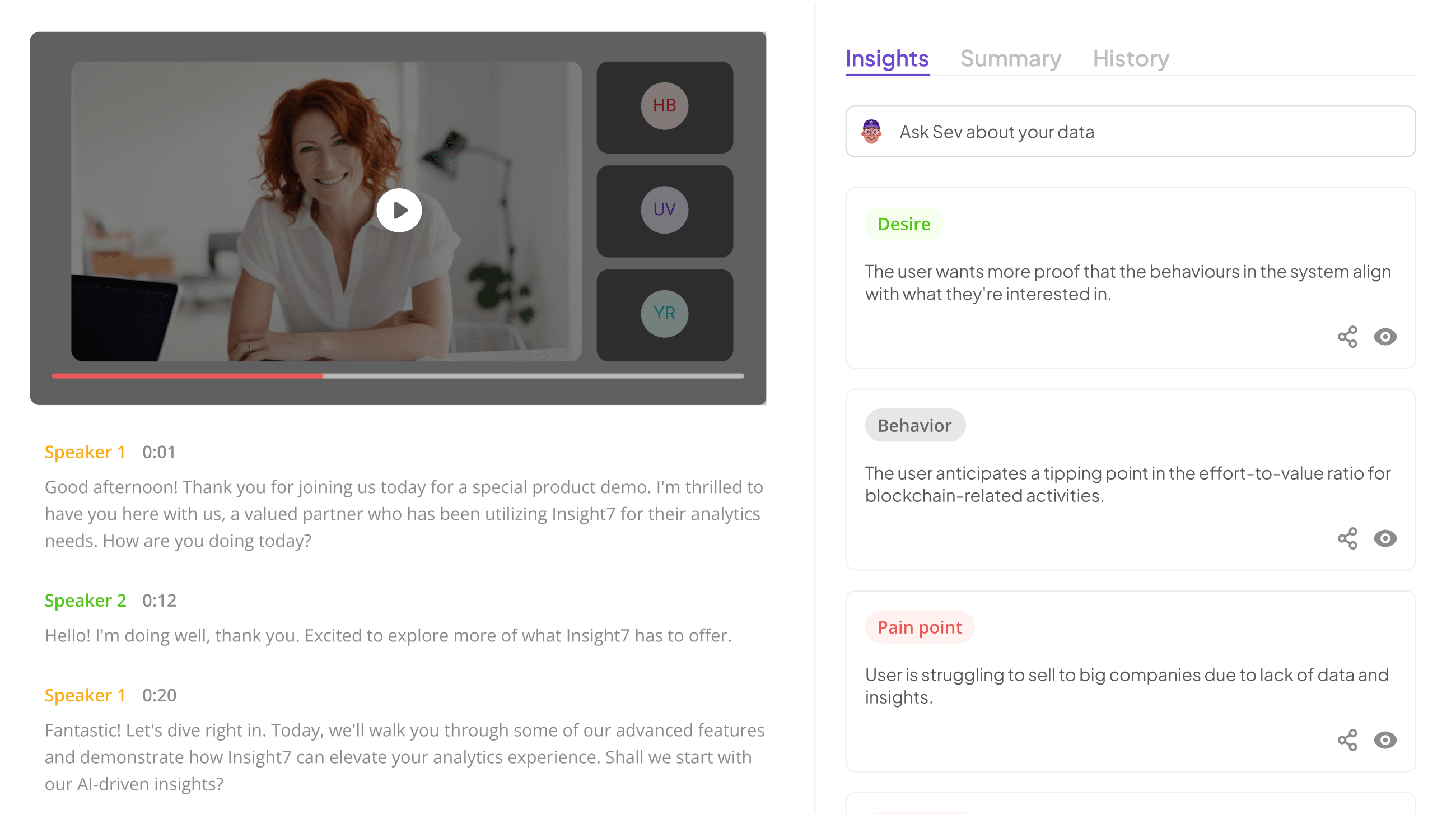
Transitioning from Manual to AI-Driven Metrics
Transitioning from manual metrics to AI-driven metrics marks a significant evolution in quality analytics. Traditional manual QA metrics often struggle with consistency and scalability, creating limitations in assessing product quality accurately. In contrast, AI quality KPIs provide a refined approach by leveraging data-driven insights, enabling teams to make informed decisions in real time.
Understanding the journey from manual to AI-driven metrics involves a few essential steps. First, organizations must recognize and define key AI quality KPIs tailored to their specific needs. Then, they can systematically implement these metrics, gradually phasing out manual processes. This transition not only streamlines operations but also enhances the accuracy of performance evaluations. By embracing AI quality KPIs, businesses position themselves for increased efficiency and sustained product excellence. Ultimately, this transformation is crucial for organizations aiming to remain competitive in an increasingly data-centric marketplace.
The Limitations of Manual QA Metrics
Manual QA metrics pose significant limitations that can hinder the efficiency and effectiveness of quality assurance processes. Traditional methods often rely on subjective assessments, which can lead to inconsistencies in performance evaluations. Additionally, manual metrics typically focus on outputs rather than understanding the underlying issues, resulting in missed opportunities for improvement. This reactive approach can be time-consuming and may not provide holistic insights into the quality of products or services.
Moreover, manual QA metrics can lack real-time responsiveness. As businesses evolve rapidly, these metrics often become outdated, unable to keep pace with the dynamic nature of product development. This is where AI Quality KPIs come into play. By integrating AI technology, organizations can shift from static, manual assessments to dynamic, data-driven analysis. This transition not only enhances accuracy and consistency but also allows teams to identify areas for improvement proactively, revolutionizing quality assurance processes in the ever-changing marketplace.
Why AI Quality KPIs are Essential
AI Quality KPIs are essential for modern quality assurance, as they provide precise metrics for evaluating AI modelsโ effectiveness. Unlike traditional manual QA metrics, which can often be vague and inconsistent, AI Quality KPIs standardize the assessment process. This standardization is crucial, especially when aiming for accountability and reliability in AI systems.
By employing these KPIs, organizations can better understand model performance through various lenses, including predictive accuracy and resource optimization. Additionally, the flexibility of AI Quality KPIs allows for continual refinement and improvement, ensuring that models evolve according to changing needs. Ultimately, these KPIs not only enhance overall quality but also support strategic decision-making, allowing teams to allocate resources and prioritize initiatives more effectively. Thus, investing in AI Quality KPIs is vital for any organization seeking to thrive in a data-driven landscape.
Key AI Quality KPIs Transforming QA Processes
AI Quality KPIs are redefining quality assurance (QA) processes by offering precise insights that elevate operational efficiency. Traditional manual metrics often struggle to keep pace with the rapid evolution of technology, leading to delayed feedback and inaccuracies. By adopting key AI Quality KPIs, organizations can ensure quality metrics are not only reliable but also predictive, enabling proactive problem identification and resolution.
Key AI Quality KPIs focus on aspects such as predictive accuracy, model performance, and resource optimization. These metrics allow teams to analyze vast amounts of data quickly, turning insights into actionable improvements. Additionally, they streamline the tracking of compliance and customer engagement during interactions. By moving towards these AI-driven metrics, organizations will significantly improve their capacity to deliver high-quality products and services, ultimately enhancing customer satisfaction.
Extract insights from interviews, calls, surveys and reviews for insights in minutes
Predictive Accuracy and Model Performance
Predictive accuracy and model performance are critical factors when evaluating AI quality KPIs. These aspects reveal how well a model can anticipate outcomes based on input data. In the realm of quality analytics, traditional metrics are often insufficient, making AI Quality KPIs vital for delivering precise insights. Understanding predictive accuracy allows organizations to gauge a model's ability to make correct predictions over time, while model performance encompasses a broader assessment of how effective those predictions are in real-world scenarios.
To enhance predictive accuracy, organizations should focus on setting up continuous model training and validation processes. This ensures that the system adapts to new data, maintaining high levels of performance. Additionally, regular monitoring of performance metrics can identify potential issues early, enabling swift adjustments. By integrating these practices, organizations can significantly improve their AI-driven QA processes, ultimately replacing outdated manual metrics with forward-thinking AI Quality KPIs that provide robust, actionable insights.
Cost Efficiency and Resource Optimization
In todayโs fast-paced business environment, achieving cost efficiency and resource optimization is essential. This is where AI Quality KPIs come into play, transforming the way quality assurance operates. By utilizing AI-driven metrics instead of traditional manual processes, businesses can significantly reduce operational costs. For instance, automated analytics tools help sift through vast amounts of data, making it easier to identify critical insights without extensive manual review.
Furthermore, AI Quality KPIs streamline resources by enabling teams to focus on high-impact areas. They facilitate better allocation of effort and time, ensuring that employees work on tasks that matter most. This shift allows organizations to enhance productivity and performance while minimizing wasted resources. Embracing these key performance indicators can lead to more informed decision-making, ultimately paving the way for greater success in quality assurance initiatives.
Implementing AI Quality KPIs: A Step-by-Step Guide
Implementing AI Quality KPIs is a structured, strategic process designed to enhance your quality assurance efforts. Begin by identifying key metrics that directly correlate with your AI systems' performance. These metrics should reflect the effectiveness, efficiency, and accuracy of your AI models and their outputs. For instance, predictive accuracy can serve as a benchmark for model performance while ensuring that your AI systems remain reliable and trustworthy.
Next, leverage the best AI tools available to facilitate the tracking and analysis of these metrics. Tools like IBM Watson Analytics or Sift Science provide valuable insights into the performance of your AI models. With accurate data at your disposal, you can continuously refine your approach and improve outcomes. The implementation of these KPIs marks a pivotal shift in your QA processes, allowing for real-time monitoring and adjustments, ultimately leading to a more robust, efficient quality assurance framework.
Step 1: Identifying Key Metrics
Identifying key metrics is the crucial first step in transitioning to AI quality KPIs from traditional manual quality assessment methods. This phase requires thoughtful consideration of the specific objectives you wish to achieve. Focusing on clear and measurable metrics allows teams to evaluate their AI systems effectively and gain insights into performance trends.
When determining which metrics to prioritize, consider both qualitative and quantitative data. For instance, look for predictive accuracy, which gauges how well the AI models forecast outcomes, and model performance, which assesses overall reliability. These key metrics not only provide a benchmark for improvements but also help uncover areas needing further training and refinement. By establishing relevant AI quality KPIs early on, organizations can ensure that their quality assurance processes evolve and meet the demands of a fast-paced, data-driven environment.
Step 2: Leveraging Top AI Tools
Incorporating advanced AI tools is a pivotal step toward evolving how organizations measure and optimize AI Quality KPIs. These tools automate processes that were once labor-intensive and prone to human error. By utilizing AI-driven solutions, businesses can rapidly analyze data, uncover insights, and gauge the effectiveness of their quality metrics with remarkable precision.
The journey involves selecting the right tools that align with specific operational needs. For instance, platforms like IBM Watson Analytics and Applitools offer tailored solutions that deeply analyze interactions and performance metrics. Other AI tools such as Neurala and Sift Science focus on real-time data analysis to enhance decision-making. Each tool provides unique features that streamline quality assurance, enabling organizations to transition from traditional metrics to a more dynamic, AI-enhanced framework. This careful selection and implementation of AI tools create a robust system for monitoring and improving project outcomes efficiently.
insight7
In the realm of quality analytics, understanding customer interactions is crucial. By effectively harnessing AI Quality KPIs, organizations can transition from traditional, manual QA practices to a more efficient, automated approach. The insights derived from customer interviews and interactions provide a wealth of data that, when analyzed properly, reveals valuable trends and patterns.
Key AI Quality KPIs to consider include predictive accuracy, which measures how well your models forecast outcomes based on historical data. Another important KPI is cost efficiency, focusing on how resource allocation can enhance overall performance. By prioritizing these metrics, businesses can avoid the pitfalls of scattered insights and ensure that their analytics drive actionable strategies. Embracing AI Quality KPIs not only improves decision-making but also promotes a collaborative work environment where data insights are more accessible and impactful.
Neurala
In a world increasingly driven by data, the need for innovative AI Quality KPIs is essential to enhance quality assurance processes. These metrics enable organizations to analyze and interpret vast amounts of customer feedback seamlessly. With advanced analytics, businesses can quickly identify patterns and insights that would take traditional methods much longer to uncover. This capability not only accelerates decision-making but also improves the overall customer experience by providing timely and relevant responses.
AI Quality KPIs can significantly transform how companies operate, leading to better resource utilization and increased productivity. By automating the data analysis process, businesses can shift from a reactive approach to a proactive one. This transition empowers teams to understand customer needs and expectations better, ultimately driving strategic initiatives more effectively. Embracing these AI-driven metrics is not just a necessity; itโs a competitive advantage in todayโs fast-paced business environment.
Sift Science
Sift Science represents a pivotal shift in the realm of AI Quality KPIs by enabling businesses to better manage their quality assurance processes. Through advanced data analysis and machine learning, organizations can replace outdated manual metrics. The result is increased efficiency and improved decision-making through real-time insights, which are essential in today's fast-paced market.
This approach allows companies to track potential fraud patterns and assess customer interactions more seamlessly. By harnessing the power of AI-driven analytics, businesses can focus on significant performance indicators that truly matter. Adopting such metrics leads to a proactive environment where issues can be addressed before they escalate. As organizations embrace these advancements, they become better equipped to meet customer expectations and stay competitive. Understanding and utilizing these AI Quality KPIs is vital for continued success in quality assurance.
Applitools
Incorporating AI Quality KPIs into your QA process can significantly enhance your evaluation metrics. Applitools exemplifies how AI-driven visual testing can automate and refine quality assurance. By relying on visual AI, teams can efficiently identify differences in user interfaces across multiple platforms. This technological advancement allows for rapid feedback cycles and reduces human error, ultimately ensuring a consistent user experience.
The core functionalities of Applitools prioritize essential metrics like accuracy and compliance in visual testing. Companies can adapt predefined templates to suit their specific quality criteria, whether for customer service interactions or sales calls. This not only facilitates thorough assessments but also aligns evaluations with strategic business goals. As organizations transition from manual metrics to AI Quality KPIs, Applitools stands as a valuable tool, driving higher standards in quality assurance practices and yielding measurable outcomes.
IBM Watson Analytics
IBM Watson Analytics serves as a powerful tool in modern quality analytics, streamlining the assessment of AI Quality KPIs. This platform enables users to analyze vast datasets quickly, aiding in the identification of key performance indicators that enhance quality assurance processes. By harnessing advanced data visualization techniques, it turns complex data into insights that are easily digestible.
Incorporating features such as natural language processing, users can pose queries and receive answers in real time. This ability to interact with data dynamically allows businesses to uncover patterns and trends that were previously obscured. Enhanced data interpretation means teams can make informed decisions, prioritize actions based on quantitative insights, and subsequently replace traditional manual metrics with more reliable, AI-driven alternatives. Overall, the insights derived from this tool can significantly transform how quality metrics are tracked and analyzed.
Conclusion: Embracing AI Quality KPIs for Enhanced QA
In conclusion, embracing AI Quality KPIs marks a significant shift towards enhanced quality assurance processes. By replacing traditional manual metrics with AI-driven insights, organizations can achieve greater precision and efficiency in evaluating their systems. This transformation not only streamlines operations but also enables teams to focus on impactful, data-informed decisions.
Adopting these innovative metrics fosters a proactive approach to quality assurance. With real-time analytics and automated evaluations, businesses can quickly identify issues, optimize resources, and improve compliance. Ultimately, the integration of AI Quality KPIs not only enhances quality assurance but also paves the way for an adaptive, future-ready organization.
Analyze & Evaluate Calls. At Scale.
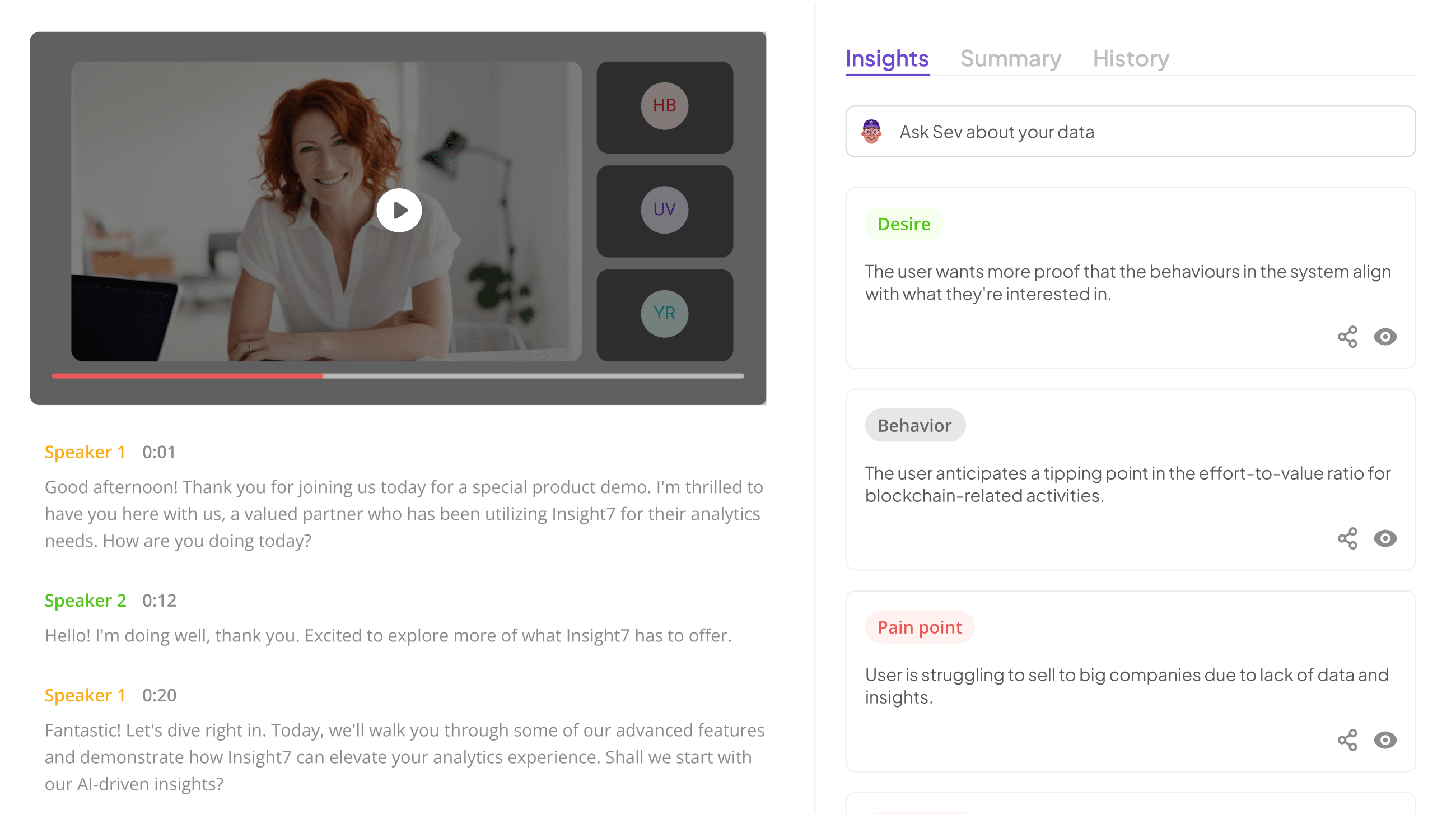
๐ฌ Need Help? Chat with Support
Our team typically responds within minutes