AI-Powered Research Assistants are transforming qualitative research by streamlining data collection and analysis processes. With the growing complexity of qualitative inquiries, researchers often find themselves overwhelmed by vast amounts of information. This is where AI-Powered Research Assistants come into play, offering innovative solutions to extract meaningful insights from qualitative data quickly and efficiently. As research teams aim to enhance their methodologies, the integration of AI can significantly alleviate the challenges they face.
These advanced tools not only automate repetitive tasks but also enhance the accuracy of data interpretation. By leveraging machine learning algorithms, researchers can glean patterns and trends that may otherwise go unnoticed. The potential of AI-Powered Research Assistants extends beyond efficiency; they foster a deeper understanding of nuanced human experiences, enabling researchers to draw more reliable conclusions. As we delve into the process of building custom AI agents, we will explore how these technologies can be tailored to meet specific research objectives.
Analyze qualitative data. At Scale.
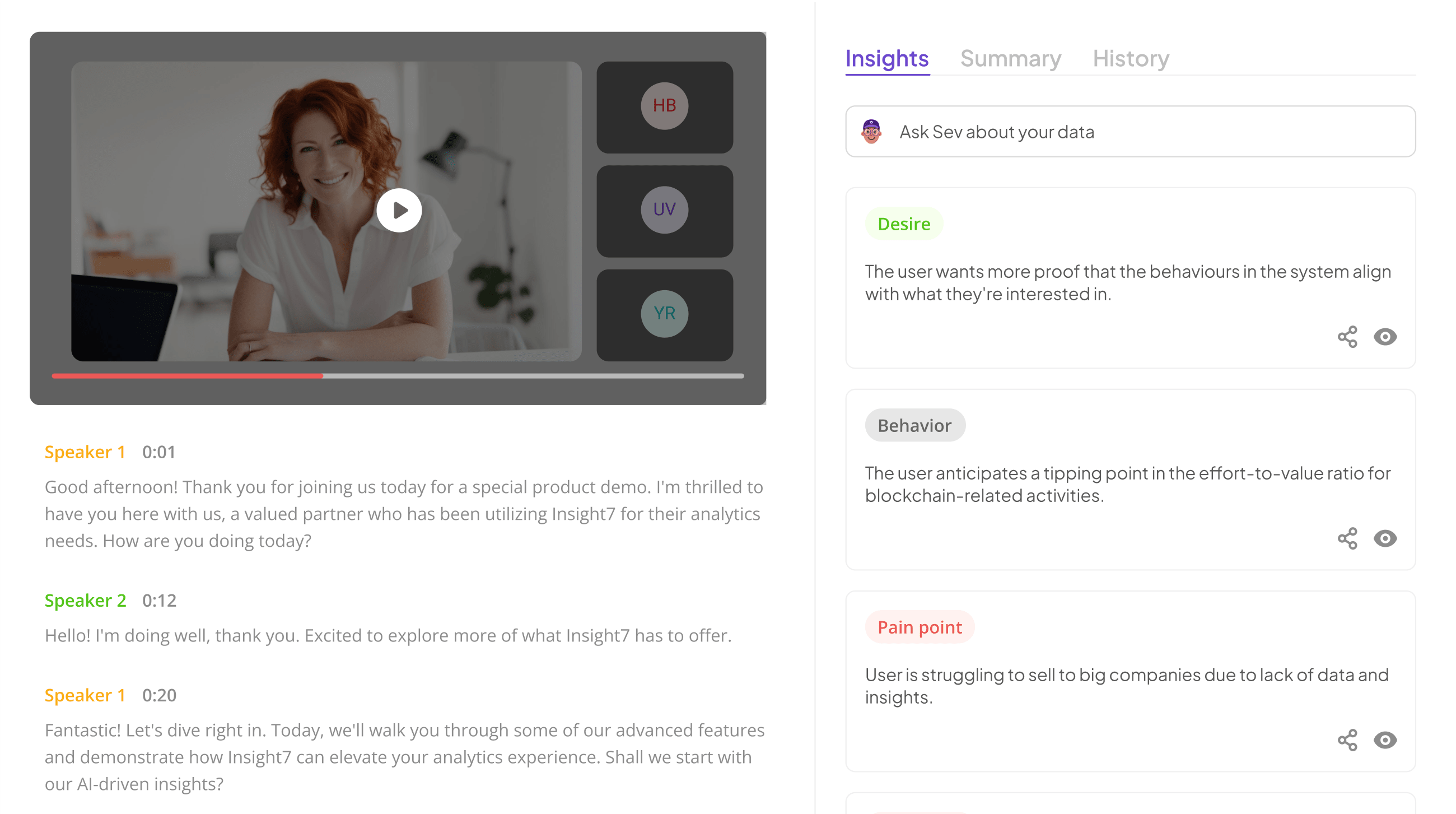
Understanding AI-Powered Research Assistants
AI-Powered Research Assistants are transformative tools designed to enhance qualitative research processes. They utilize advanced algorithms to analyze data, recognize patterns, and generate insights from various sources of information. This capability allows researchers to save time and effort while focusing on deeper analysis and interpretation of findings. By automating routine tasks, these assistants free researchers to concentrate on critical thinking and strategy, fostering a more reliable outcome.
Understanding how AI-Powered Research Assistants function is crucial for successful integration into research workflows. They rely on natural language processing and machine learning to interpret human language and context, providing accurate results that can inform decision-making. Moreover, they can adapt to the unique needs of each research project, allowing for customization that aligns with specific research goals. This adaptability makes them invaluable allies in navigating the complexities of qualitative research, ultimately enhancing reliability and depth in the insights derived.
What are AI-Powered Research Assistants?
AI-Powered Research Assistants are cutting-edge tools designed to streamline qualitative research. These advanced systems utilize artificial intelligence to automate various aspects of data collection, analysis, and interpretation. By leveraging natural language processing technologies, AI-powered assistants can efficiently parse through vast amounts of qualitative data, such as interviews and focus group discussions. This capability enables researchers to extract actionable insights with less manual effort.
The effectiveness of AI-Powered Research Assistants lies in their ability to learn from patterns in data. They assist researchers in identifying trends, sentiments, and key themes, thereby enhancing the depth of qualitative analysis. Furthermore, these tools can be customized to fit different research needs, allowing users to tailor their functionalities based on specific objectives. The combination of speed, efficiency, and adaptability makes AI-powered assistants invaluable in todayโs research environment, ultimately transforming how qualitative insights are gathered and interpreted.
The Role of AI in Qualitative Research
AI-Powered Research Assistants are transforming qualitative research by enhancing the efficiency and depth of analysis. They automate tedious tasks such as transcription, categorization, and thematic analysis, allowing researchers to focus on interpretation and strategy. Furthermore, these intelligent agents can provide insights by identifying patterns and trends from large datasets that might go unnoticed through traditional methods.
In addition, AI-Powered Research Assistants foster a more consultative approach to research. By generating specific prompts and guiding questions, they help researchers dig deeper into subjects and extract valuable insights. Their ability to adapt to varying research goals means they can be tailored for specific studies, facilitating a more nuanced understanding of the data. As qualitative researchers harness AI, they gain powerful tools that not only enhance productivity but also enrich the entire research process.
Building Your Custom AI-Powered Research Assistants
Building your custom AI-powered research assistants involves a thoughtful and structured approach. First, it's essential to define your research goals clearly. Knowing what insights or data you seek allows you to tailor the AI assistant's functionalities. This step sets the foundation for the entire project, ensuring that the assistant operates with a focused purpose.
Next, selecting the right machine learning models is crucial. There are various algorithms available, each serving different needs in qualitative research. Once you have chosen your model, gather and preprocess relevant data. This data must be cleaned and formatted properly to maximize the AI's effectiveness. Following this, you'll train and test your AI model to refine its performance. Regular evaluations of the model will help ensure it meets the desired standards and can adapt as more data becomes available. By following these steps, you can build a robust AI-powered research assistant that enhances your qualitative research efforts.
Extract insights from interviews, calls, surveys and reviews for insights in minutes
Steps to Create a Custom AI Agent
Creating a custom AI agent for qualitative research begins with defining specific research goals. This foundational step aligns your AI with targeted objectives that serve your overall strategy. Be explicit about what you want from the agent, including the types of insights you seek and how they will be utilized. This focus will guide every subsequent stage and ensure your AI-powered research assistants deliver meaningful contributions to your project.
The next step involves selecting the appropriate machine learning models tailored for your goals. Understanding the various algorithms and their strengths is crucial for optimizing your AI's performance. After that, gather and preprocess your data, ensuring that it is clean and relevant. Once your data is ready, train and test your AI model diligently, evaluating its effectiveness in generating qualitative insights. By following these structured steps, you can build a powerful AI agent that enhances your qualitative research endeavors.
Step 1: Define Your Research Goals
To effectively build custom AI agents for qualitative research, you must begin with a clear understanding of your research goals. This step is pivotal, as it outlines the direction and objectives of your project. Defining what you aim to achieve helps ensure that the AI-powered research assistants you create will effectively address your specific needs and questions. Think about the key insights you wish to uncover and the problems you want to solve with your AI agent.
Articulating your research goals involves asking critical questions. What type of data do you need to analyze? Who is your target audience, and what insights will benefit them? Additionally, consider how the AI-powered research assistants can enhance your data collection and interpretation processes. Creating well-defined and measurable goals will pave the way for the subsequent steps in the development process, ultimately resulting in a more efficient and effective research project.
Step 2: Select the Right Machine Learning Models
Choosing the right machine learning models is critical for developing effective AI-powered research assistants. The models you select will directly influence how well your assistant understands and processes qualitative data. Start by assessing the specific tasks you want your assistant to perform, such as sentiment analysis or topic modeling. Different tasks require different models, so clarity on your goals is essential.
Once you know the tasks at hand, consider popular machine learning models. For instance, natural language processing (NLP) models like BERT or GPT can be highly effective for text-based qualitative research. Additionally, you might explore supervised learning models for classification tasks or unsupervised learning for exploring patterns within your data. The right combination of models will ensure that your AI-powered assistant provides relevant and accurate insights, enhancing the overall quality of your research.
Step 3: Gather and Preprocess Data
Gathering and preprocessing data is a pivotal step in building your custom AI-powered research assistants. This phase lays the foundation for accurate analysis and insightful outcomes in qualitative research. Start by collecting relevant data from interviews, surveys, or other qualitative sources. It's crucial to ensure that the data is comprehensive and reflective of the research objectives.
Once you've collected sufficient data, the preprocessing stage begins. This involves cleaning the data, which means removing any irrelevant information, correcting errors, and handling missing values. You may also categorize your data to identify patterns and insights easily. Finally, employing tools to transform this cleaned data into a structured format can significantly streamline the analysis process. This ensures your AI-powered research assistants can function effectively, ultimately leading to more reliable and actionable insights in your qualitative research.
Step 4: Train and Test Your AI Model
Training and testing your AI model is a critical phase in creating effective AI-powered research assistants. After gathering and preprocessing your data, it is time to refine your model. This involves selecting a suitable training algorithm based on your research objectives. For instance, if your AI model is intended to analyze sentiment in qualitative data, specific supervised learning techniques may be essential for accurate outputs.
Once your model is trained, the next step is rigorous testing. Evaluate its performance using a separate dataset to ensure reliability. Techniques such as cross-validation can help gauge the modelโs effectiveness. During this phase, be prepared to make adjustments based on test results. Continuous refinement and iterations will enhance the model's performance, ultimately resulting in a more reliable AI-powered research assistant, capable of generating meaningful insights from qualitative data. Remember, success in this step leads to a more robust and intuitive research tool for your future projects.
Top Tools for Developing AI-Powered Research Assistants
AI-Powered Research Assistants are transforming the qualitative research field by streamlining data analysis and insight extraction. To develop effective assistants, it is essential to utilize advanced tools that facilitate data management, processing, and analysis. Various tools on the market can assist researchers by offering tailored functionalities based on specific needs.
First, consider tools like insight7 and MonkeyLearn, which specialize in automated data analysis and sentiment extraction. NVivo and MAXQDA, on the other hand, provide robust frameworks for qualitative coding and thematic analysis. Finally, Atlas.ti emerges as a versatile application, supporting complex data categorization and visualization. Each of these platforms caters to different research aspects, allowing you to choose one that best aligns with your project's goals and complexity. By selecting the appropriate tools, youโll not only enhance productivity but also improve the quality of insights derived from your research.
insight7
AI-Powered Research Assistants streamline the process of analyzing qualitative data, making it easier for researchers to derive insights. The effectiveness of these assistants lies in their ability to handle vast amounts of information rapidly. As organizations generate significant customer signals at scale, traditional methods of analysis often fall short. AI-powered tools automate menial tasks, allowing researchers to focus on deriving actionable insights from data.
Organizations can build customized AI agents by following a few steps. Begin by clearly defining your research goals to shape the AIโs functionality. Next, choose suitable machine learning models that align with your objectives. Data collection and preprocessing come next, ensuring the information is clean and usable. Finally, training and testing the model allow it to learn effectively, driving better analytical outcomes. By empowering your research with AI-Powered Research Assistants, you enhance efficiency and unlock deeper qualitative insights.
MonkeyLearn
In the realm of qualitative research, the integration of AI-powered research assistants can significantly streamline data processing and insight generation. These tools enable researchers to gather and analyze qualitative data from various sources quickly. For instance, a user-friendly platform allows anyone in an organization to access and utilize its features without extensive training. This simplifies the process of transforming raw data, such as interview transcripts, into actionable insights that inform strategic decisions.
The platform's robust capabilities include organizing information into a searchable library, where users can examine individual conversations and extract relevant insights. Pain points, customer desires, and feedback can be identified easily, enhancing the overall research experience. By combining intuitive design with powerful analytical tools, AI-powered research assistants can democratize data-driven insights, empowering teams to engage with qualitative research on a deeper level and drive informed decision-making within their organizations.
Atlas.ti
This powerful tool is designed to streamline qualitative research processes effectively. Users can organize and analyze rich data sets, including interviews, focus group transcripts, and open-ended survey responses. By harnessing its capabilities, researchers benefit from visuals that highlight patterns in their data, allowing for more insightful conclusions.
To build your AI-powered research assistants, first, create projects within this platform. Projects allow you to upload various data files, analyze them collectively, and run queries that summarize findings across different data sources. Through features like automated transcriptions and data matrices, researchers can quickly extract the information needed for comprehensive analyses or reporting. This efficiency in handling qualitative data makes it an essential component for any team looking to enhance their research quality.
NVivo
NVivo serves as an essential tool for developing AI-Powered Research Assistants tailored for qualitative research. This platform allows researchers to effortlessly manage and analyze complex data from interviews, focus groups, and surveys. By organizing qualitative data into projects, you can visually represent patterns and insights, facilitating a deeper understanding of your research questions.
One of NVivo's standout features is its ability to integrate various data sources, enabling the seamless import of audio transcripts and written files. This versatility helps you swiftly analyze customer feedback or product insights across multiple channels. The matrix analysis tool allows researchers to extract relevant quotes and summarize trends from numerous sources, maximizing your efficiency with qualitative data. By utilizing NVivo, you can create a foundation for your AI-Powered Research Assistants, ensuring your qualitative research is both comprehensive and meaningful.
MAXQDA
Creating AI-powered research assistants involves leveraging various tools that streamline qualitative research. MAXQDA stands out as a versatile application designed to handle data qualitatively, offering researchers a unique edge. This robust software allows for the importation of diverse data types, such as transcripts and audio files, making it easier to analyze and visualize findings. Researchers can initiate new projects effortlessly, thereby promoting efficient organizing and management of research material.
In the context of building custom AI agents, MAXQDA equips users with features to extract themes and recognize patterns quickly. Users can synthesize large amounts of qualitative data into actionable insights, essential for decision-making processes. The software also supports project collaboration, enabling teams to analyze and visualize historical queries collaboratively, thus fostering richer discussions and deeper understanding. When integrated thoughtfully, AI-powered research assistants can significantly enhance the depth and breadth of qualitative research, streamlining workflows and enriching research outputs.
Conclusion: Advancing Qualitative Research with AI-Powered Research Assistants
AI-Powered Research Assistants hold transformative potential for qualitative research. By seamlessly integrating advanced algorithms, these tools can analyze vast amounts of data, identify trends, and elevate insights. Researchers can focus more on interpreting findings rather than getting bogged down in data collection, allowing for deeper and more meaningful analyses.
Moreover, employing AI technology enriches the research experience by providing customizable features tailored to specific project needs. As we move forward, embracing AI-Powered Research Assistants can significantly enhance research methodologies and outcomes, ultimately fostering more informed decision-making in various fields.