Automated Qualitative Analysis marks a significant evolution in the realm of research methodologies. Traditionally, qualitative coding has been a time-consuming and often subjective process, reliant on human interpretation. However, advances in technology and artificial intelligence have sparked interest in whether this task can be effectively automated without sacrificing accuracy.
As we explore the potential of Automated Qualitative Analysis, it's essential to understand its promise. This system aims to enhance efficiency by swiftly processing large volumes of qualitative data. Simultaneously, it seeks to maintain consistency, reducing biases that often infiltrate manual coding. Yet, as automation takes center stage, we must also remain vigilant about its limitations and the nuances inherent in human language.
Analyze qualitative data. At Scale.
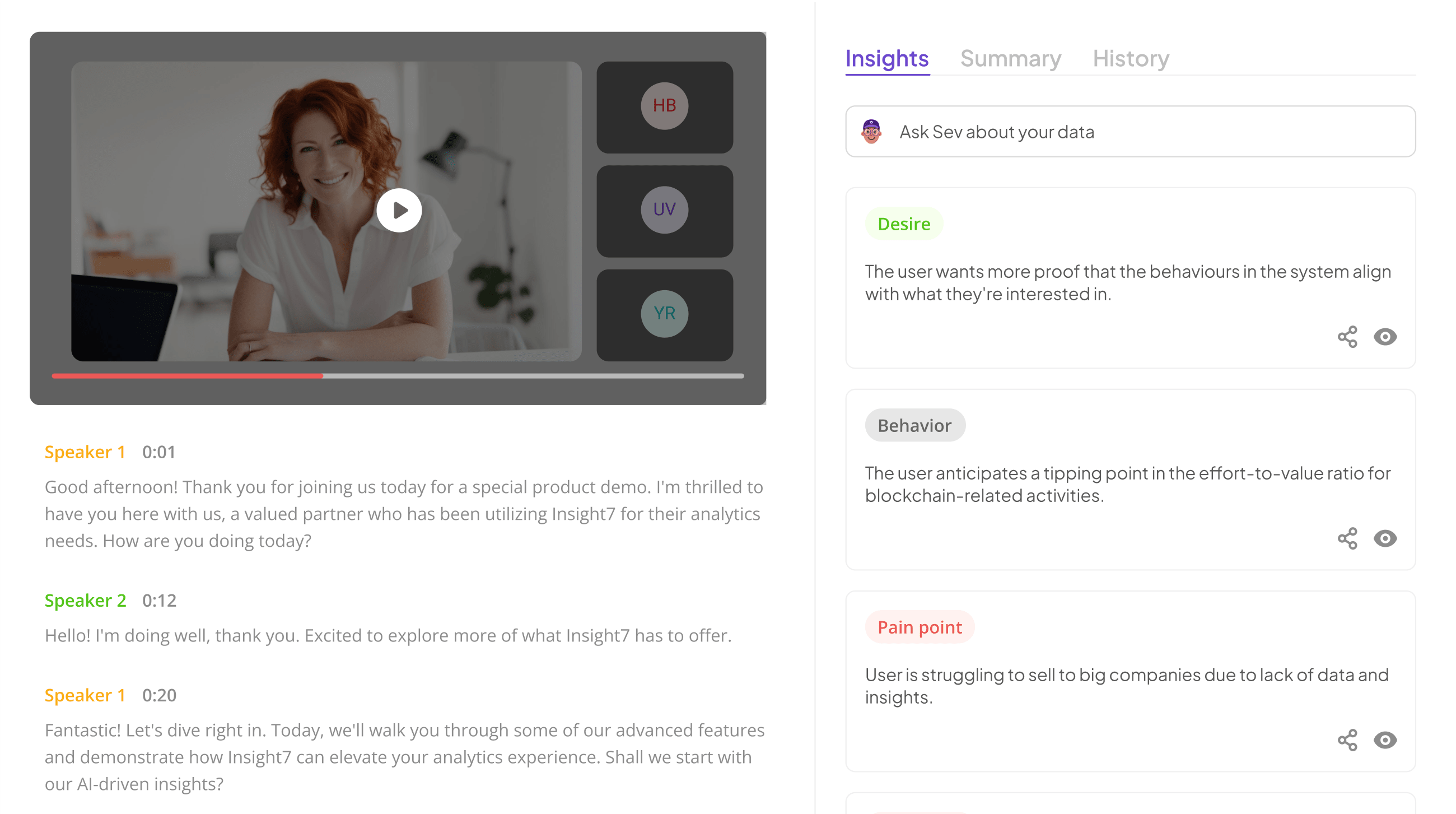
The Promise of Automated Qualitative Analysis
Automated Qualitative Analysis holds significant potential for transforming traditional research methodologies. As organizations increasingly generate vast amounts of customer data, the promise of automation becomes appealing. This shift can alleviate the burdens associated with manually analyzing qualitative data, which is often time-consuming and laden with potential biases. By integrating automated systems, researchers can analyze interviews and open-ended survey responses more efficiently, leading to quicker insights.
The allure of this approach lies not only in its speed but also in its ability to ensure consistency across analyses. Automated systems can provide objectivity that human coders may inadvertently lack, especially in large-scale studies. Nevertheless, while the promise of automated qualitative analysis is enticing, it is essential to acknowledge both the technology's limitations and the nuances of human judgment that still play a crucial role in interpreting qualitative data. Thus, a balanced strategy that combines automation with human expertise may yield the best results.
Understanding Automated Qualitative Coding
Automated qualitative coding involves the process of using technology to analyze and categorize qualitative data, such as interviews or focus group discussions. This method seeks to replicate traditional coding processes, where human researchers identify themes or patterns, but does so through sophisticated algorithms. Understanding how automated qualitative coding works is vital for researchers looking to enhance their workflow, especially in areas requiring large-scale data analysis.
Automation comes into play by employing tools that can transcribe and analyze data efficiently. These technologies utilize algorithms that can identify keywords or themes within text, allowing researchers to uncover insights more rapidly than manual coding allows. Despite its potential, it's essential to acknowledge that automated qualitative coding may struggle with context and nuance, which are often critical in qualitative research. Thus, while it offers substantial benefits, understanding its limitations is equally important for effective application.
- Definition of Qualitative Coding and its Importance
Qualitative coding refers to the process of categorizing and analyzing unstructured data, such as interviews and open-ended survey responses. By identifying themes, patterns, and underlying meanings, researchers can derive insights that quantitative data often cannot provide. This nuanced approach is essential in understanding the complexities of human experiences and behaviors, particularly in fields like social science and market research.
The importance of qualitative coding cannot be understated, as it transforms raw data into actionable insights. Automated qualitative analysis emerges as a solution to enhance the efficiency of this process. By automating mundane tasks, researchers can focus on interpreting findings rather than getting lost in manual coding. This shift not only accelerates data analysis but also aims to improve consistency, reducing human error. Understanding these dynamics is crucial for evaluating the potential and limitations of automation in qualitative codingโpaving the way for more efficient research methodologies.
- How Automation Comes into Play
In the realm of qualitative research, automation introduces a transformative approach that streamlines coding processes. Automated qualitative analysis enables researchers to process large datasets efficiently, minimizing manual involvement. However, the true potential of automation lies in its ability to enhance accuracy and consistency, which are critical in qualitative coding.
To harness automation effectively, researchers often consider several factors. First, establishing clear coding guidelines ensures that the automated system can accurately interpret and classify data. Second, iterative testing allows researchers to refine the automation process, leading to improved reliability in results. Finally, itโs essential to balance automated coding with human oversight, as nuanced understanding remains vital in qualitative analysis. By combining these elements, automation comes into play as a practical solution, offering both speed and depth in qualitative research while maintaining integrity and ethical standards.
Key Benefits of Automated Qualitative Analysis
Automated Qualitative Analysis offers several key benefits that can enhance the efficiency and accuracy of qualitative research. First, it significantly increases efficiency and speed. Traditional qualitative coding can be highly time-consuming, often requiring countless hours to analyze textual data manually. Automation streamlines this process, allowing researchers to process large volumes of data quickly and focus on deriving insights rather than getting bogged down in manual coding.
Second, consistency and objectivity in coding are crucial advantages. Automated systems apply predefined coding schemes uniformly across the dataset, reducing the risk of biases that can occur with human coders. This objective approach ensures that similar data is coded in the same manner, enhancing the reliability of findings. Together, these benefits make Automated Qualitative Analysis not only a promising tool but also a game-changer for researchers seeking to derive meaningful insights more effectively.
- Increased Efficiency and Speed
Automation in qualitative analysis is revolutionizing how researchers approach data. Increased efficiency and speed are two core advantages of this shift. With traditional methods, analyzing qualitative data can be slow and labor-intensive. By employing automated qualitative analysis tools, researchers can drastically reduce the time spent coding large volumes of text. This not only accelerates the research process but also helps teams respond to insights swiftly.
Moreover, faster processing means researchers can focus more on interpreting results rather than getting lost in the minutiae of manual coding. Enhanced speed allows for timely decision-making, enabling organizations to adjust strategies based on fresh insights. As qualitative insights are generated rapidly, collaboration among team members improves significantly. Stakeholders can access coded information and interpretations in real-time, fostering more dynamic discussions and innovative problem-solving. Overall, the promise of automated qualitative analysis lies in transforming a traditionally slow process into a swift, streamlined workflow, ultimately driving smarter business strategies.
- Consistency and Objectivity in Coding
Automated Qualitative Analysis aims to enhance consistency and objectivity within the coding process. Traditional qualitative coding relies heavily on human interpretation, which can lead to inconsistent results due to personal biases and varying perspectives. By implementing automated systems, researchers can ensure that their coding frameworks remain uniform and free from individual interpretation errors, fostering reliability in qualitative research.
Furthermore, objectivity in coding is critical for drawing valid conclusions from data. Automated systems enable coders to apply standardized criteria across entries, reducing the influence of personal judgment in analysis. With a well-defined set of criteria, these systems can quickly process data at scale, thereby allowing researchers to focus on interpreting results rather than getting entangled in the minutiae of coding decisions. Ultimately, safeguarding these elementsโconsistency and objectivityโreinforces the value of insights derived from automated qualitative analysis.
Extract insights from interviews, calls, surveys and reviews for insights in minutes
Challenges and Limitations of Automated Qualitative Analysis
Automated Qualitative Analysis presents a complex set of challenges and limitations that must be addressed for effective implementation. Technological constraints significantly impact the ability to handle nuance and context in qualitative data, as these elements are often subtle and intricate. Automated tools may struggle to interpret the varied meanings inherent in complex conversations, leading to potential misinterpretations that can skew insights.
Additionally, ethical and practical concerns arise from the use of automation in qualitative analysis. Issues concerning data privacy must be vigilantly managed, ensuring that personal information is protected. Moreover, the need for human oversight remains critical, as automated systems cannot fully replicate human intuition and understanding in qualitative contexts. While automation is a promising development, striking a balance between efficiency and accuracy is essential to harness the true value of qualitative insights.
Technological Challenges
Automated Qualitative Analysis faces significant technological challenges that can hinder its effective implementation. One major hurdle is effectively understanding the nuance and context within qualitative data. Traditional coding often relies on an interpretive process that captures subtle meanings, tones, and cultural references. Automating this task may result in oversimplification, leading to misinterpretations of the data. A machine can struggle to discern the emotional undertones or contextual implications that a trained coder would naturally pick up.
Additionally, the constraints of AI and Natural Language Processing (NLP) technologies further contribute to these challenges. While advancements are continually being made in AI, issues like ambiguity in language and idiomatic expressions can disrupt the coding process. Consequently, even sophisticated algorithms may fail to achieve the desired accuracy and depth in data analysis. As we explore the feasibility of automating qualitative coding, it is essential to recognize these technological limitations while seeking ways to enhance the accuracy and reliability of the insights gained.
- Handling Nuance and Context in Data
The process of handling nuance and context in data is critical in automated qualitative analysis. While automation can process vast amounts of data quickly, it often struggles with the subtleties that human interpreters instinctively grasp. For example, different phrases may carry diverse meanings influenced by context, cultural background, or emotional tone. Without careful programming, an automated system may misinterpret these nuances, leading to flawed conclusions.
To address these challenges, it is essential to implement advanced natural language processing techniques. Such techniques should be designed to recognize context cues, idiomatic expressions, and emotional undertones. Additionally, integrating human oversight can significantly improve the accuracy of interpretations. A collaborative approach that combines automation's efficiency with human insight can pave the way for more reliable coding outcomes. As organizations embrace automated qualitative analysis, understanding and mitigating the complexities of data context becomes a vital aspect of their strategy.
- AI and Natural Language Processing Constraints
Automated Qualitative Analysis aims to streamline data interpretation, but several constraints hinder its effectiveness. The journey begins with understanding that AI and Natural Language Processing (NLP) systems struggle with nuances inherent in qualitative data. For example, human emotions, sarcasm, and cultural references often elude even advanced algorithms. Consequently, these limitations may lead to misinterpretations or superficial analyses that miss the depth of human sentiment.
Additionally, the dependence on large datasets presents challenges. AI models require extensive examples to learn effectively; however, qualitative data is often rich in variability. As a result, codifying complex themes may not align with automated approaches. Thus, while the notion of automating qualitative coding seems appealing, practitioners must remain aware of these AI and NLP constraints to ensure the analysis remains meaningful and accurate. A balanced approach that combines technology and human insight is essential for achieving reliable outcomes in Automated Qualitative Analysis.
Ethical and Practical Concerns
Automated Qualitative Analysis raises several ethical and practical concerns that deserve careful consideration. First, data privacy issues loom large. Automated systems often process sensitive information, which can lead to unintentional breaches of privacy if not managed properly. Researchers must ensure that any automated tools adhere to strict data protection regulations to maintain participant confidentiality.
Moreover, human oversight remains crucial. While automation can enhance efficiency, it lacks the nuanced understanding inherent to human analysis. A human touch not only interprets context but also addresses potential biases embedded within the coding algorithms. Thus, a collaborative approach that leverages both automated processes and human expertise is essential to cultivate ethical practices in qualitative research. Striking this balance can help ensure research integrity while maximizing the benefits of automation.
- Data Privacy Issues
Automated qualitative analysis carries significant data privacy considerations. As organizations strive to enhance their research capabilities, they must navigate the complexities of handling sensitive information. One primary concern revolves around protecting personally identifiable information (PII), such as social security numbers and dates of birth. Failing to secure these details can result in serious breaches of trust and legal repercussions.
Moreover, automated systems may inadvertently mishandle data if not designed with privacy in mind. It's vital for organizations to implement strict protocols around data management. They should ensure that consent is obtained for data collection and recording, maintaining transparency with users at all touchpoints. Human oversight remains essential in this context, as technology may not fully comprehend the nuances of ethical data handling. Ultimately, prioritizing data privacy will foster a safer environment for conducting automated qualitative analysis while cultivating trust with stakeholders.
- The Need for Human Oversight
Automated Qualitative Analysis holds promise for efficiency, yet it must be approached with caution. Relying solely on automated systems risks overlooking vital complexities inherent in qualitative data. Context, nuance, and the subtleties of human expression are aspects that often escape even the most advanced algorithms. Therefore, the need for human oversight is paramount in any automated qualitative coding process.
First, human oversight ensures that the interpretation of data remains grounded in real-world understanding. Automated systems may misinterpret tone or intent, leading to incorrect coding results. Second, it safeguards against biases that automated analysis might introduce. Humans can apply critical thinking and contextual awareness, refining analyses for accuracy. Lastly, oversight allows for iterative feedback loops, enabling continuous improvement in both the automation process and the overall insights derived. Thus, blending human intelligence with automation can lead to more reliable outcomes in qualitative research.
Tools for Automated Qualitative Analysis
Automated Qualitative Analysis is becoming increasingly feasible thanks to various innovative tools designed for this purpose. A core element of this technological shift involves harnessing software that can efficiently categorize and analyze qualitative data. Tools like Atlas.ti and NVivo have emerged as industry leaders, offering robust functionalities for coding and theme analysis. These platforms assist researchers by streamlining the evaluation process, allowing for more organized data management.
Each tool presents unique benefits and features. For instance, Atlas.ti excels in visualizing complex data relationships, making it easier to derive insights. NVivo, on the other hand, highlights the integration of various sources, including text and audio, which can enrich the analysis. Quirkos and Dedoose further diversify the options by providing user-friendly interfaces ideal for both novice users and seasoned researchers. As the landscape of Automated Qualitative Analysis continues to evolve, so too does the capacity for researchers to gain meaningful insights efficiently.
Insight7: Leading the Way in Innovation
As industries increasingly seek timely insights from massive amounts of data, automated qualitative analysis emerges as a key player in navigating this complexity. The promise of this innovation lies in its ability to swiftly interpret customer conversations, transforming raw data into actionable intelligence. Organizations are now poised to embrace tools that provide advanced analysis, driving efficiency in understanding consumer sentiments.
Leading the way in innovation, top-notch automated qualitative analysis tools offer self-service options that enhance data scalability. These platforms not only ensure high-speed processing but also maintain objectivity and consistency in coding. By streamlining the analysis process, businesses can focus on strategic decisions, resulting in a competitive edge. As customer signals continue to grow, the adoption of innovative technologies becomes essential for effective insight generation and business success. Embracing automated qualitative analysis may very well shape the future of data-driven decision-making.
- Key Features and Capabilities
Automated Qualitative Analysis offers a range of features and capabilities designed to streamline the coding process. First, advanced algorithms can efficiently identify themes and patterns within large datasets, which enhances analysis speed and reduces manual effort. This capability is essential for researchers aiming to draw insights quickly from extensive qualitative data.
Moreover, consistency and objectivity in coding are among the standout features of automated coding systems. These systems minimize the influence of human bias, ensuring that analysis remains objective across various data sets. Additionally, user-friendly interfaces often allow researchers to visualize coding results, making it easier to interpret findings.
Furthermore, integrating feedback loops within these tools can lead to continuous improvement. By evaluating prior analyses, automated systems can refine their coding accuracy over time. This iterative process ultimately enhances the quality and reliability of insights drawn from qualitative research.
Other Noteworthy Tools
In the realm of Automated Qualitative Analysis, various tools have emerged that enhance coding processes and provide valuable insights. These noteworthy tools serve different needs while streamlining qualitative research. One such tool, Atlas.ti, offers a robust environment for data organization and visualization. Users can annotate, code, and analyze data with ease, making it invaluable for researchers.
Another popular option is NVivo, known for its advanced analytical capabilities and compatibility with various data sources. This tool allows for a deeper understanding of patterns and themes within qualitative datasets. Quirkos stands out with its user-friendly interface, simplifying the coding process for individuals less familiar with software. Dedoose, on the other hand, facilitates collaborative coding, making it ideal for teams working on qualitative research together. In a landscape where speed and accuracy are vital, these tools significantly contribute to effective automated qualitative analysis.
- Atlas.ti
Automated qualitative analysis relies on tools that streamline data coding for researchers. One notable example is a robust software that enhances efficiency in managing qualitative data. This tool offers a unique project management system, allowing users to compile, transcribe, and analyze audio files efficiently. By enabling the import of various data formats, researchers can initiate projects with a few clicks and focus on extracting key insights.
Moreover, the platform's analytical capabilities stand out. Users can easily create matrices to examine multiple data points, identifying patterns and trends across responses. This simplification not only saves time but also minimizes human error, contributing to more reliable outcomes. With the potential to automate repetitive tasks, this tool exemplifies how automated qualitative analysis can drive valuable insights and foster better decision-making in research. Additionally, understanding the nuances of user feedback is crucial in maintaining the quality of insights derived from coded data.
- NVivo
With advancements in technology, NVivo emerges as a noteworthy tool in the realm of automated qualitative analysis. This software enables researchers to organize and analyze complex data with greater efficiency, automating many tedious aspects of qualitative coding. By allowing users to create projects and upload various data types, NVivo streamlines the process of extracting insights from qualitative data.
When using NVivo, users benefit from features that enhance data interpretation. The software's ability to visualize historical queries can quickly extract recurring themes, enabling researchers to draw insights without manual effort. Additionally, its project-based approach facilitates the aggregation of data, permitting deeper analysis through direct comparisons across different sources. This integration of automation does not eliminate the need for human oversight but certainly enhances the speed and coherence of the qualitative analysis process, making it a valuable asset in researchers' toolkits.
- Quirkos
Quirkos offers an intuitive approach to Automated Qualitative Analysis by simplifying the complexity of coding qualitative data. It integrates visual elements that allow researchers to organize and categorize their data interactively. This visual-centric design is particularly beneficial for teams seeking to facilitate collaboration and streamline their data analysis processes. It empowers researchers to extract meaningful insights efficiently while maintaining focus on the themes that emerge from their data sets.
Moreover, the platform addresses common challenges faced in traditional qualitative coding. It accommodates coding nuances and context by allowing users to easily adjust codes and themes as needed. This capability fosters a dynamic coding environment, ensuring that analysis is both thorough and adaptable. By leveraging such tools, organizations can not only save time but also enhance the accuracy and depth of their insights, making them better equipped to inform decision-making processes.
- Dedoose
Dedoose is a valuable tool for researchers looking to streamline their qualitative analysis processes. With features designed for enhanced collaboration, it allows researchers to analyze large volumes of qualitative data efficiently. Users appreciate its intuitive interface, which simplifies coding and facilitates the exploration of relationships among various themes within datasets. By employing cloud-based technology, it ensures that teams can access data from anywhere, thus promoting teamwork across diverse locations.
One of the core advantages of using Dedoose for automated qualitative analysis is its ability to generate visualizations that aid in understanding complex data sets. Such features not only save time but also enable researchers to dive deeper into insights that may otherwise go unnoticed. While the tool streamlines many coding processes, it remains essential to provide human oversight to ensure nuanced interpretations of data. Ultimately, embracing Dedoose can enhance the quality and efficiency of research efforts in an increasingly data-driven world.
Conclusion: Is Automated Qualitative Analysis Truly Feasible?
The feasibility of Automated Qualitative Analysis remains a complex topic for researchers and practitioners alike. While advances in technology have made it possible to automate certain aspects of qualitative coding, the nuances inherent in human communication present significant challenges. Automated systems can struggle to interpret context, tone, and emotional subtleties, which often play crucial roles in qualitative research outcomes.
Moreover, ethical considerations, such as data privacy and the necessity of human oversight, further complicate the scenario. Although automated tools can enhance efficiency and consistency, they cannot fully replace the human touch required for in-depth analysis. Therefore, a hybrid approach, combining automated technologies with expert human insight, may be the most viable path forward for effective qualitative research.