Extracting Key Phrases That Predict Churn in Support Call Data
-
Bella Williams
- 10 min read
In the ever-evolving landscape of customer retention, understanding churn indicators is crucial for any organization. Identifying these indicators in support call data offers valuable insights into the underlying reasons for customer dissatisfaction. By analyzing conversations with support representatives, businesses can unveil patterns that lead to churn, empowering teams to take proactive measures.
Churn indicators identification involves utilizing qualitative insights derived from customer interactions. This process not only aids in pinpointing areas for improvement but also fosters a culture focused on customer satisfaction. With the right tools and methodologies in place, organizations can effectively address concerns before they escalate, turning potential churn into customer loyalty.
Analyze & Evaluate Calls. At Scale.
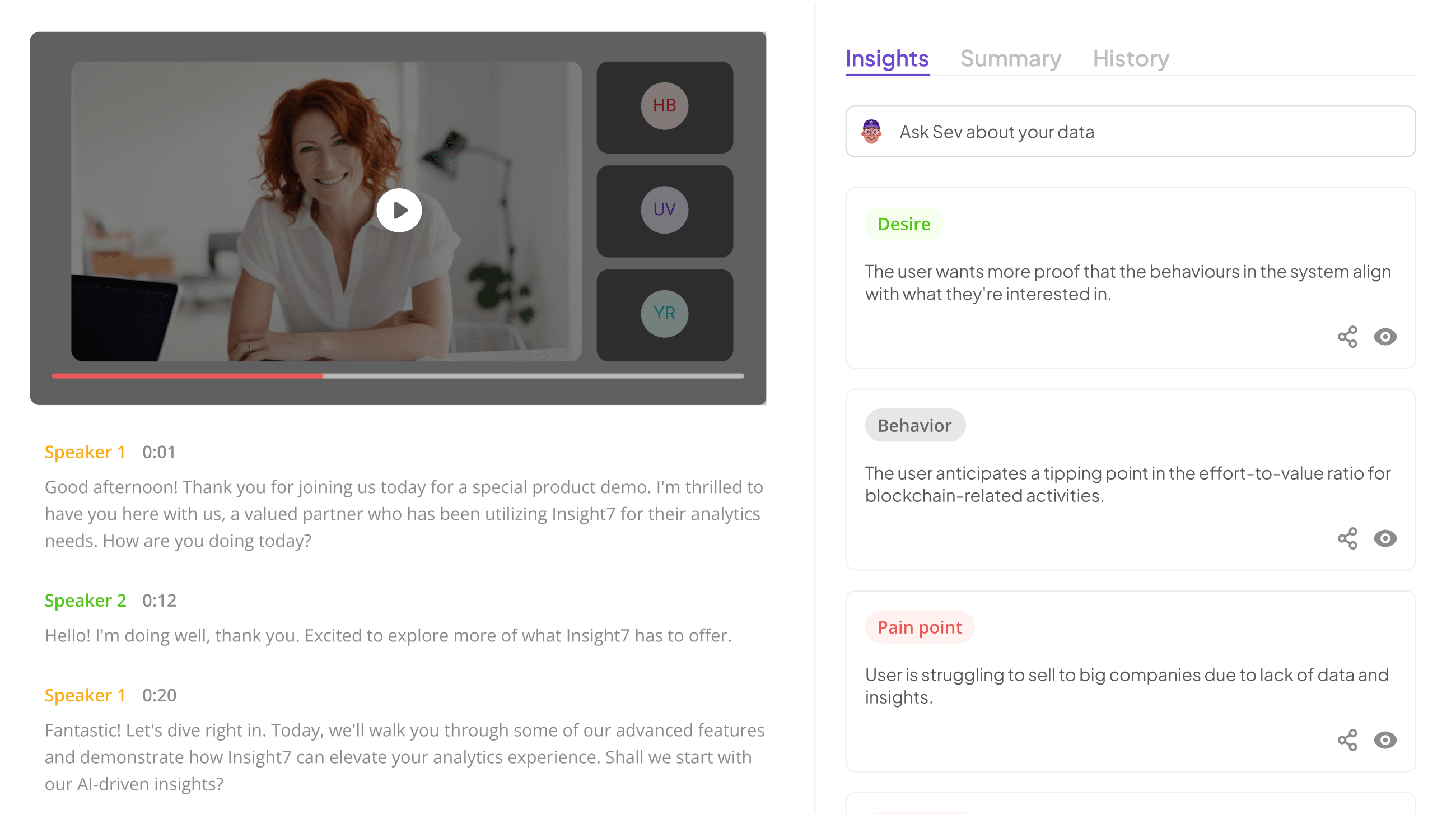
Understanding Churn Indicators Identification
Understanding churn indicators is a crucial first step in effectively managing customer retention. Churn indicators identification involves recognizing key phrases in support call data that suggest a customer may be considering leaving. By analyzing these phrases, businesses can gain insights into customer sentiment and dissatisfaction. Often, the language customers use during calls reveals underlying issues that might not be directly communicated.
To successfully identify these churn indicators, itโs essential to evaluate common customer concerns, such as product usability or service quality. By prioritizing these issues, organizations can implement proactive measures, addressing the root causes of churn. Collecting and analyzing support call data allows for identifying patterns in customer behavior. This understanding not only fosters customer loyalty but also enhances the overall service experience, ultimately reducing churn rates and leading to increased customer satisfaction.
The Importance of Identifying Key Phrases
Identifying key phrases in support call data is crucial for understanding customer sentiment and predicting churn. Properly analyzing these phrases can reveal insights into the issues that customers face. This identification helps businesses proactively address concerns and enhance customer experiences, ultimately leading to retention.
Engaging with churn indicators allows organizations to delve deeper into customer feedback. By focusing on language patterns, businesses can better identify common pain points and recurrent themes. This understanding not only aids in refining support strategies but also fosters a culture of continuous improvement. In the long run, addressing these phrases can significantly reduce churn rates and improve customer satisfaction, making key phrase identification a vital component of any successful customer retention strategy.
How Key Phrases Predict Customer Churn
Identifying key phrases in support call data plays a crucial role in predicting customer churn. These phrases serve as indicators that reveal underlying sentiments and issues customers face. Recognizing patterns in the language used by customers when expressing dissatisfaction can provide invaluable insights. By analyzing these key phrases, organizations can pinpoint specific problems contributing to churn, allowing for targeted interventions.
To effectively predict customer churn, it's essential to focus on the sentiment expressed in these key phrases. For instance, phrases indicating frustration, disappointment, or confusion may highlight critical areas for improvement. Additionally, categorizing these phrases helps in understanding which aspects of the service are most likely to cause churn. By integrating this knowledge, teams can proactively address concerns, enhancing customer retention and loyalty.
Extract insights from interviews, calls, surveys and reviews for insights in minutes
Techniques for Churn Indicators Identification
Identifying churn indicators requires a systematic approach to analyzing support call data. First, it's essential to prepare this data effectively, ensuring that it is clean and organized for meaningful analysis. This preparation stage sets the foundation for further exploration of patterns that indicate potential churn risk among customers. Accurate data preparation involves filtering out irrelevant information, normalizing formats, and categorizing calls based on key attributes.
Next, applying text mining techniques for key phrase extraction is crucial. This process enables the identification of specific phrases or terms frequently associated with customer dissatisfaction or complaints. By scrutinizing these phrases, organizations can uncover underlying sentiments and trends that signal an increased likelihood of churn. Finally, analyzing these key phrases in the context of customer interactions provides actionable insights. Understanding the language and concerns raised by customers during support calls can guide proactive measures to enhance retention strategies and improve overall customer experience.
Step 1: Preparing the Support Call Data
In preparing the support call data, the first and crucial step involves thorough data collection and organization. Begin by gathering all relevant support call transcripts, noting specific interactions that may indicate potential customer dissatisfaction. This initial set of data serves as a foundation for identifying patterns and trends. Each transcript should be cleaned and structured, ensuring clarity in the text and consistency in formatting.
Once you have organized the data, it's essential to identify key phrases that might operate as churn indicators. During this phase, consider utilizing tools like Insight7 for effective data transcription and analysis. By accurately preparing your support call data, you enhance the potential for meaningful insights, guiding further analysis. The eventual goal is to synthesize this information and apply it toward predicting customer churn effectively.
Step 2: Text Mining for Key Phrases
Text mining for key phrases is a critical step in the process of churn indicators identification. To successfully extract meaningful phrases, various natural language processing techniques come into play. By analyzing customer support call transcripts, you can uncover recurring themes, sentiments, and language patterns that signal potential customer dissatisfaction. The insights derived from these phrases enable organizations to better understand their customers' needs and concerns, which is essential for reducing churn rates.
Several techniques can facilitate effective text mining. First, tokenization breaks text into manageable pieces, allowing for easier analysis. Next, sentiment analysis assesses the emotional tone of the conversations. Following this, frequency analysis highlights the most common phrases used, pinpointing key topics of discussion. Lastly, contextual analysis helps in understanding the sentiments in relation to specific phrases. These processes combine to create a comprehensive view of customer sentiment and behavior, informing strategies to improve customer retention and satisfaction.
Step 3: Analyzing Key Phrases for Churn Prediction
Analyzing key phrases for churn prediction involves a detailed investigation of customer interactions during support calls. This step is crucial for uncovering churn indicators that might be hidden within the conversations. By pinpointing phrases that frequently appear in calls from customers who eventually churn, businesses can gain valuable insights into pain points and expectations.
๐ฌ Questions about Extracting Key Phrases That Predict Churn in Support Call Data?
Our team typically responds within minutes
Understanding these phrases allows organizations to shift their focus from reactive measures to proactive strategies that address customer concerns. For instance, if repeated phrases indicate confusion about pricing, that could signal a need for clearer communication in sales materials or customer support training. Tools like Insight7 can assist in analyzing large volumes of support data, enabling teams to identify these critical phrases more effectively. Ultimately, this analysis empowers companies to mitigate churn by addressing concerns before they lead to customer attrition.
Tools for Extracting Churn Indicators
Identifying churn indicators is essential for improving customer retention and enhancing service strategies. Various tools facilitate churn indicators identification by analyzing support call data and extracting key phrases that signal customer dissatisfaction. Each tool serves a unique purpose in the analytical process, ensuring a comprehensive approach to understanding why customers leave.
One effective tool is Insight7, which allows for quantitative analysis and efficient data evaluation. Alternatively, MonkeyLearn provides text analysis capabilities helping to uncover sentiments and trends embedded in customer conversations. IBM Watson employs advanced AI to understand context and predict churn based on call data. RapidMiner offers powerful analytics features while SAS Text Miner excels in managing unstructured text data. Leveraging these tools not only enhances understanding of churn drivers but also equips businesses with actionable insights for mitigating future churn risks. By actively engaging with these technologies, organizations can systematically address customer concerns and foster loyalty.
insight7
Identifying churn indicators is crucial for businesses seeking to improve customer retention. By analyzing support call data, organizations can uncover key phrases that repeatedly occur before a customer decides to leave. This process allows companies to understand the underlying issues causing dissatisfaction and proactively address them.
Churn indicators can be found through several effective techniques. Preparing the support call data involves organizing the information so it is ready for analysis. Next, text mining is performed to extract and categorize key phrases from the conversations. This step is essential because it highlights recurring themes that may signal customer frustration or dissatisfaction. Finally, analyzing these key phrases helps in predicting churn, providing valuable insights that can inform strategic improvements. By focusing on common concerns, companies can enhance customer experience and lower the rate of churn.
MonkeyLearn
MonkeyLearn offers powerful capabilities for extracting churn indicators from support call data, helping organizations gain critical insights into customer behavior. This tool utilizes machine learning to analyze text, enabling the identification of key phrases that correlate with customer churn. By processing support call transcripts, it filters through vast data volumes to highlight patterns and signals that may predict churn, allowing businesses to be proactive in their customer retention strategies.
The ability to automate this analysis reduces manual effort and ensures higher accuracy in identifying churn indicators. By integrating MonkeyLearn with existing customer relationship management systems, organizations can continuously monitor customer sentiment and flag significant issues as they arise. This streamlined approach not only enhances the understanding of customer needs but also paves the way for more effective support strategies, ultimately fostering long-term loyalty and satisfaction.
IBM Watson
IBM Watson serves as a powerful tool for extracting valuable insights from support call data, playing a critical role in Churn Indicators Identification. By leveraging advanced natural language processing capabilities, this tool can analyze customer interactions and detect patterns associated with potential churn. The technologyโs ability to process vast amounts of text data enables businesses to identify key phrases that signal dissatisfaction or intent to leave.
To optimize its effectiveness, itโs vital to prepare the support call data appropriately and utilize text mining techniques to unveil influential keywords. Once these key phrases are identified, businesses can analyze them to predict customer churn accurately. This proactive approach not only enhances customer engagements but also informs strategies that improve retention rates, ultimately fostering stronger client relationships. As businesses harness the power of AI, tools like this can transform customer support dynamics and predict churn more effectively.
RapidMiner
RapidMiner provides a robust platform for extracting meaningful insights from support call data, aiding organizations in churn indicators identification. By utilizing its advanced machine learning capabilities and intuitive interface, users can effectively parse through vast datasets to uncover critical phrases signaling customer dissatisfaction or potential disengagement. The platform's versatility allows users to seamlessly integrate support call transcriptions, enabling a comprehensive analysis of conversational data.
The process begins with data preprocessing, where users can clean and structure the input data, enhancing accuracy in subsequent analyses. Following this, RapidMiner facilitates text mining techniques that identify recurring themes or phrases linked to customer churn. Once these key phrases are uncovered, they can be analyzed in-depth to develop predictive models tailored to anticipating churn. Ultimately, leveraging RapidMiner not only streamlines the identification of churn indicators but also empowers organizations to take proactive steps in retaining valuable customers.
SAS Text Miner
SAS Text Miner offers a robust framework for extracting insights from unstructured data, particularly in analyzing customer support calls. By applying advanced text mining techniques, this tool helps in identifying crucial churn indicators that may otherwise go unnoticed. It employs algorithms to process large volumes of text, distilling key phrases that reflect customer sentiments and potential issues leading to churn.
Using SAS Text Miner, organizations can enhance their customer retention strategies significantly. The tool enables analysts to pinpoint recurring phrases and sentiments that indicate dissatisfaction or potential churn signals. Through a detailed examination of support call data, businesses gain actionable insights into customer experience, allowing for proactive measures to address concerns before they escalate. This strategic approach not only bolsters customer satisfaction but also drives long-term loyalty by anticipating issues effectively.
Conclusion: Synthesizing Churn Indicators Identification Results
In synthesizing the results of Churn Indicators Identification, we uncover essential insights that can significantly impact customer retention strategies. By analyzing support call data, we've identified patterns and phrases that directly correlate with customer dissatisfaction and potential churn. This analysis not only highlights critical touchpoints where intervention is needed but also empowers teams to refine their support processes.
Ultimately, understanding and acting upon these churn indicators is vital for maintaining customer loyalty. The results emphasize the importance of proactive measures, such as improved onboarding and enhanced customer communication. By addressing these indicators, organizations can better serve their customers and curb churn effectively.
๐ฌ Questions about Extracting Key Phrases That Predict Churn in Support Call Data?
Our team typically responds within minutes